- Contact Us: [email protected]


Social Network Analysis 101: Ultimate Guide
Comprehensive introduction for beginners.
Social network analysis is a powerful tool for visualizing, understanding, and harnessing the power of networks and relationships. At Visible Network Labs, we use our network science and mapping tools and expertise to track collaborative ecosystems and strengthen systems change initiatives. In this Comprehensive Guide, we’ll introduce key principles, theories, terms, and tools for practitioners framed around social impact, systems change, and community health improvement. Let’s dig in!
Learn more and get started with the tools below in our complete Guide.
Table of Contents
You can read this guide from start-to-finish or use the table of contents to fast forward to a topic or section of interest to you. The guide is yours to use as you see fit.
Introduction
Let’s start by reviewing the basics, like a definition, why SNA is important, and the history of the practice. If you want a quick intro to this methodology, download our Social Network Analysis Brief .
Definition of Social Network Analysis (SNA)
Social Network Analysis , or SNA, is a research method used to visualize and analyze relationships and connections between entities or individuals within a network. Imagine mapping the relationships between different departments in a corporation. The outcome would be a vivid picture of how each department interacts with others, allowing us to see communication patterns, influential entities, and bottlenecks
The Importance of SNA
SNA is a powerful tool. It allows us to explore the underlying structure of an organization or network, identifying the formal and informal relationships that drive the formal processes and outcomes. This insight can enable better communication, facilitate change management, and inspire more efficient collaboration.
This methodology also helps demonstrate the impact of relationship-building and systems change efforts by documenting the changes in the quality and quantity of relationships before and after the initiative. The maps and visualizations produced by SNA are an engaging way to share your progress and impact with stakeholders, donors, and the community at large.
Brief Historical Overview of SNA
The concept of SNA emerged in the 1930s within the field of sociology. Its roots, however, trace back to graph theory in mathematics. It was not until the advent of computers and digital data in the 1980s and 1990s that SNA became widely used, revealing new insights about organizational dynamics, community structures, and social phenomena.
While it originated as an academic research tool, it is increasingly used to inform real-world practice. Today, it is used in a broad variety of industries, fields, and sectors, including business, web development, public health, foundations and philanthropy , telecommunications, law enforcement, academia, and systems change initiatives, to name a few.
Fundamentals of SNA
SNA is a broad topic, but these are some of the essential terms, concepts, and theories you need to know to understand how it works.
Nodes and Edges
In SNA, nodes represent individuals or entities while edges symbolize the relationships between them. For example, in an inter-organizational network, nodes might be companies, and edges could represent communication, collaboration, or competition.
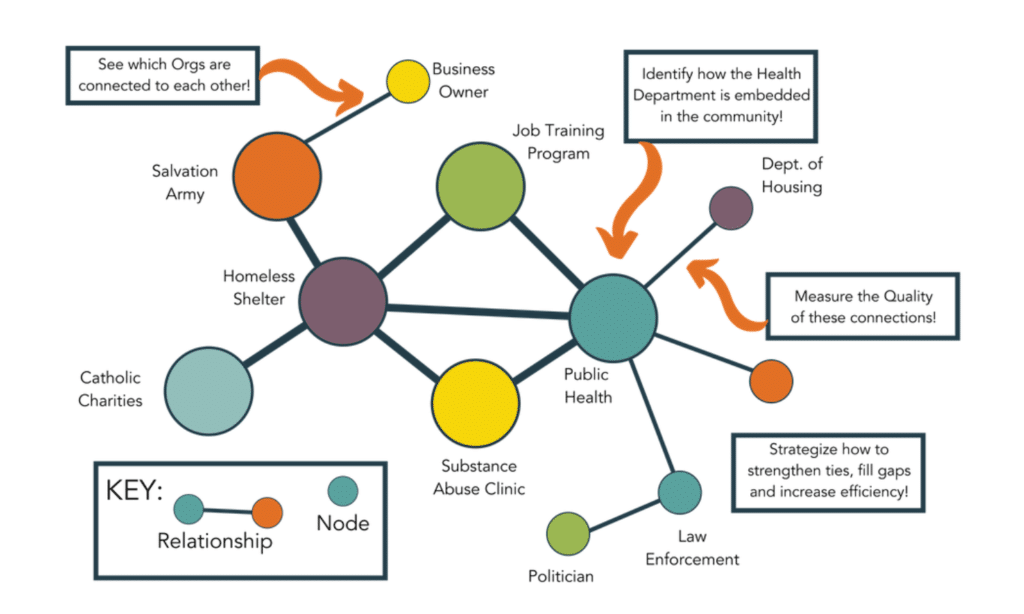
Network Types
Different types of networks serve different purposes. ‘Ego Networks’ focus on one node and its direct connections, revealing its immediate network. ‘Whole Networks’, on the other hand, capture a broader picture, encompassing an entire organization or system. Open networks are loosely connected, with many opportunities to build new connections, ideal for innovation and idea generation – while closed networks are densely interconnected, better for refining ideas amongst a group who all know each other.
Network Properties
Properties such as density (the proportion of potential connections that are actual connections), diameter (the longest distance between two nodes), and centrality (the importance of a node within the network) allow us to understand the network’s structure and function. Metrics also can measure relationship quality across the network, like our validated trust and value scores.
Dyadic and Triadic Relationships
Dyadic relationships involve two nodes, like a partnership between two companies. Triadic relationships, involving three nodes, are more complex but can offer richer insights. For instance, it might show how a third company influences the relationship between two others, or which members of your network are the best at building new relationships between their peers.
Homophily and Heterophily
Homophily refers to the tendency of similar nodes to connect, while heterophily is the opposite. In a business context, we might see homophily between companies in the same industry and heterophily when seeking diversity in a supply chain. Many networks aim to be diverse but get stuck talking to the same, similar partners. These network concepts underly many strategies promoting network innovation to avoid group-think among likeminded partners.
Network Topologies
Lastly, the layout or pattern of a network, its topology, can reveal much about its function. For instance, a centralized topology, where one node is connected to all others, may indicate a hierarchical organization, while a decentralized topology suggests a more collaborative and flexible environment. This is also referred to as the structure of the network. Read more.
Theoretical Background of SNA
Many different theories have developed to explain how certain network properties, like their topology, centrality, or type, lead to different outcomes. Here are several key theories relevant to SNA.
Strength of Weak Ties Theory
This theory postulates that weak ties or connections often provide more novel information and resources compared to strong ties. These “weak” relationships, which may seem less important, can serve as important bridges between different clusters within a network. Read more.
Structural Hole Theory
This theory posits that individuals who span the structural holes, or gaps, in a network—acting as a bridge between different groups—hold a strategic advantage. They can control and manipulate information and resources flowing between the groups, making their position more influential. Read more
Small World Network Theory
This theory emphasizes the interconnectedness of nodes within a network. It suggests that most nodes can be reached from any other node through a relatively short path of connections. This property leads to the famous phenomenon of “six degrees of separation,” indicating efficient information transfer and connectivity in a network.
Barabási–Albert (Scale-Free Network) Model
This model suggests that networks evolve over time through the process of preferential attachment, where new nodes are more likely to connect to already well-connected nodes. This results in “scale-free” networks, where a few nodes (“hubs”) have many connections while the majority of nodes have few.
Data Collection and Preparation
Every network mapping begins by collecting and preparing data before it can be analyzed. This data varies widely, but at a basic level, they must include data on nodes (the entities in the network) and data on edges (the lines between nodes representing a relationship or connection). Additional data on the attributes of the nodes or edges add more levels of analysis and insight but are not strictly necessary.
Primary Methods for Collecting SNA Data
This can be as simple as conducting interviews or surveys within an organization. The more complex the network, the more difficult it is to collect good primary data: If you have more than 5-10 partners, interviews and surveys are hard to conduct by hand.
Network survey tools like PARTNER collect relational data by asking respondents who they are connected to, and then asking them about aspects of their relationships to provide trust, value, and network structure scores. This is impossible to do using most survey software like Google Forms without hours of cleaning by hand.
Response rates are an important consideration if using surveys for data collection. Unlike a typical survey where a small sample is representative, a network survey requires a high response rate – 80% and above are considered the gold standard.
In an inter-organizational context where surveys are impossible, or you cannot achieve a valid response rate, one might gather data through business reports, contracts, or publicly available data on partnerships and affiliations. For example, you could visit an organization’s website to note who they list as a partner – and do the same for others – to generate a basic SNA map.
Secondary Sources of SNA Data
Secondary sources include data that was already collected but can be used again, often to complement your use of primary data you collect yourself. This might include academic databases, industry reports, or social media data. It’s important to ensure the accuracy and reliability of these sources.
You can also conduct interviews or focus groups with network members to add a qualitative perspective to your results. These mixed-method SNA projects provide a great deal more depth to their network maps through their conversations with numerous network representatives to explore deeper themes and perspectives.
Ethical Considerations in Data Collection
When collecting data, it’s crucial to ensure privacy, obtain necessary permissions, and anonymize data where necessary. Respecting these ethical boundaries is critical for maintaining trust and integrity in your work.
Consider also how your SNA results will be used. For example, network analysis can help assess how isolated an individual is to target them for interventions. Still, it could also be abused by insurance companies to charge these individuals a higher rate (loneliness increases your risk of death).
Lastly, consider ways to involve the communities with stake in your SNA using approaches like community-based participatory research. Bring in representatives from target populations to help co-design your initiative or innovation as partners, rather than patients or research subjects.
Preparing Data for Analysis
Data needs to be formatted correctly for analysis, often as adjacency matrices or edgelists. Depending on the size and complexity of your network, this can be a complex process but is crucial for meaningful analysis.
If you are new to SNA, you can start by laying out your data in tables. For example, the table below shows a relational data set for a set of partners within a public health coalition. The first column shows the survey respondent (Partner 1), the second shows who they reported as a partner, the third shows their reported level of trust, and the fourth their reported level of collaboration intensity. This is just one of many ways to lay out and organize network data.
Depending on which analysis tool you choose, a varying degree of data preparation and cleaning will be required. Usually, free tools require the most work, while software with subscriptions do a lot of it for you.
Network Analysis Methods & Techniques
There are many ways to analyze a network or set of entities using SNA. Here are some of basic and advanced techniques, along with info on network visualization – a major component and common output of SNA projects.
Basic Technique: Network Centrality
One of the most common ways to analyze a network is to look at the centrality of various nodes to identify key players, information hubs, and gatekeepers across the network. There are three types of centrality, each corresponding to a different aspect of connectivity and centrality. Degree, Betweenness, and Closeness Centrality are measures of a node’s importance.
Degree Centrality
Can be used to identify the most connected actors in the network. These actors are considered “popular” or “active” and they often have a strong influence within the network due to their numerous direct connections. In a coalition or network, these nodes could be the organizations or individuals that are most active in participating or the most engaged in the network activities. They may be the ‘go-to’ people for information or resources and have a significant impact on shaping the group’s agenda.
Betweenness Centrality
A useful for identifying the “brokers” or “gatekeepers” in the network. These actors have a unique position where they connect different parts of the network, facilitating or controlling the flow of information between others. In a coalition context, these could be the organizations or individuals who have influence over how information, resources, or support flow within the network, by virtue of their position between other key actors. These actors could play crucial roles in collaboration, negotiation, and conflict resolution within the network.
Closeness Centrality
A measure of how quickly a node can reach every other node in the network via the shortest paths. In a coalition, these nodes can disseminate information or exert influence quickly due to their close proximity to all other nodes. These ‘efficient connectors’ are beneficial for the rapid spread of information, resources, or innovations across the network. They could play a vital role during times of rapid change or when swift collective action is required.
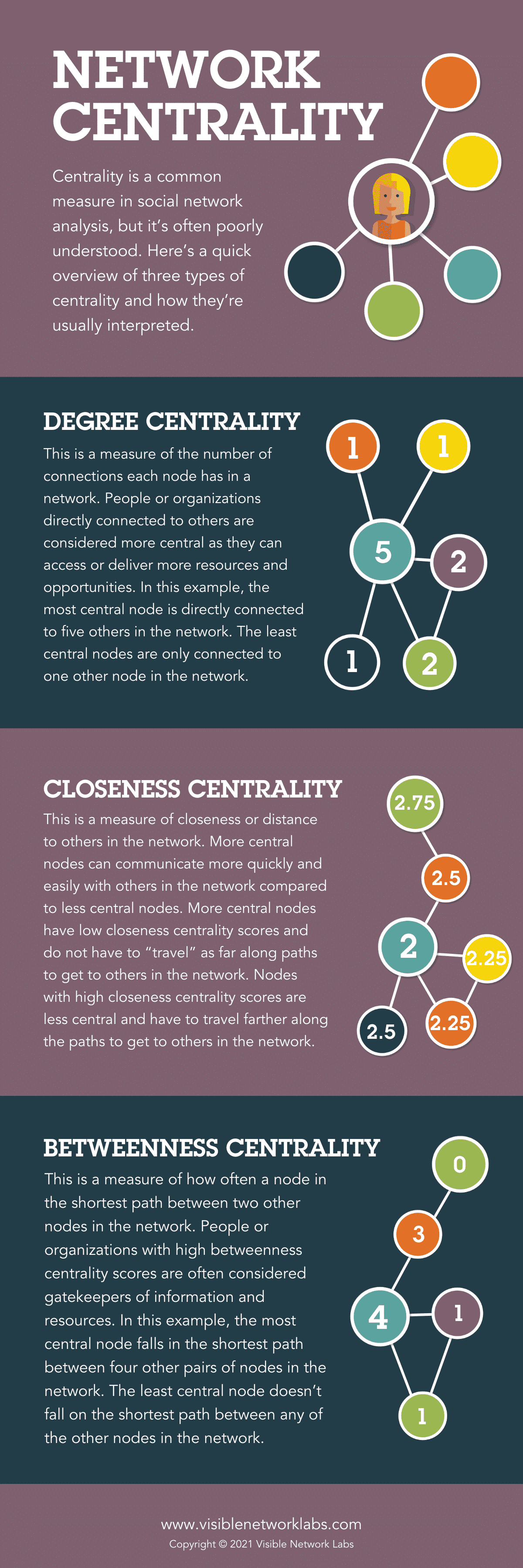
Advanced Techniques: Clusters and Equivalence
Clustering Coefficients
The Clustering Coefficient provides insights into the “cliquishness” or local cohesion of the network around specific nodes. In a coalition or inter-organizational network, a high clustering coefficient may indicate that a node’s connections are also directly connected to each other, forming tight-knit groups or sub-communities within the larger network. These groups often share common interests or objectives, and they might collaborate or share resources more intensively. Understanding these clusters can be crucial for coalition management as it can highlight potential subgroups that may need to be engaged differently, or that might possess different levels of influence or commitment to the coalition’s overarching goals.
Structural Equivalence
Structural Equivalence is used to identify nodes that have similar patterns of connections, even if they do not share a direct link. In a coalition context, structurally equivalent organizations or individuals often occupy similar roles or positions within the network, and thus may have similar interests, influence, or responsibilities. They may be competing or collaborating entities within the same sectors or areas of work. Understanding structural equivalence can provide insights into the dynamics of the network, such as potential redundancies, competition, or opportunities for collaboration. It can also reveal how changes in one part of the network may impact other, structurally equivalent parts of the network.
Visualizing Networks
Network visualization is a key tool in Social Network Analysis (SNA) that allows researchers and stakeholders to see the ‘big picture’ of the network structure, as well as discern patterns and details that may not be immediately evident from numerical data. Here are some key aspects and benefits of network visualization in the context of a coalition or inter-organizational network:
Overview of Network Structure: Visualizations provide a snapshot of the entire network structure, including nodes (individuals or organizations) and edges (relationships or interactions). This helps to comprehend the overall size, density, and complexity of the network. Seeing these relationships mapped out can often make the network’s structure more tangible and easier to understand.
Identification of Key Actors: Centrality measures can be represented visually, making it easier to identify key actors or organizations within the network. High degree nodes, gatekeepers, and efficient connectors will stand out visually, which can assist in identifying who holds influence or power within the network.
Detecting Subgroups and Communities: Visualization can also highlight clusters or subgroups within the network. These might be based on shared interests, common goals, or frequent interaction. Understanding these subgroups is crucial for coalition management and strategic planning, as different groups might have unique needs, concerns, or levels of engagement.
Identifying Outliers and Peripheral Nodes: Network visualizations can also help in identifying outliers or peripheral nodes – those who are less engaged or connected within the network. These actors might represent opportunities for further engagement or potential risks for network cohesion.
Highlighting Network Dynamics: Visualizations can be used to show changes in the network over time, such as the formation or dissolution of ties, the entry or exit of nodes, or changes in nodes’ centrality. These dynamics can provide valuable insights into the evolution of the coalition or network and the impact of various interventions or events.
Software and Tools for SNA
SNA software helps you collect, clean, analyze, and visualize network data to simplify the process of of analyzing social networks. Some tools are free with limited functionality and support, while others require a subscription but are easier to use and come with support. Here are some popular s tools used across many application
Introduction to Popular SNA Tools
Tools like UCINet, Gephi, and Pajek are popular for SNA. They offer a variety of functions for analyzing and visualizing networks, accommodating users of varying skill levels. Here are ten tools for use in different contexts and applications.
- UCINet: A comprehensive software package for the analysis of social network data as well as other 1-mode and 2-mode data.
- NetDraw: A tool usually used in tandem with UCINet to visualize networks.
- Gephi: An open-source network analysis and visualization software package written in Java.
- NodeXL: A free and open-source network analysis and visualization software package for Microsoft Excel.
- Kumu: A powerful visualization platform for mapping systems and better understanding relationships.
- Pajek: Software for analysis and visualization of large networks, it’s particularly good for handling large network datasets.
- SocNetV (Social Networks Visualizer): A user-friendly, free and open-source tool.
- Cytoscape: A bioinformatics software platform for visualizing molecular interaction networks.
- Graph-tool: An efficient Python module for manipulation and statistical analysis of graphs.
- Polinode: Tools for network analysis, both for analyzing your own network data and for collecting new network data.
Choosing the Right Tool for Your Analysis:
The right tool depends on your needs. For beginners, a user-friendly interface might be a priority, while experienced analysts may prefer more advanced functions. The size and complexity of your network, as well as your budget, are also important considerations.
PARTNER CPRM: A Community Partner Relationship Management System for Network Mapping
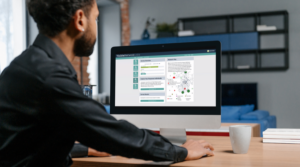
For example, we created PARTNER CPRM, a Community Partner Relationship Management System, to replace the CRMs used by most organizations to manage their relationships with their network of strategic partners. Incorporating data collecting, analysis, and visualization features alongside CRM tools like contact management and email tracking, the result is a powerful and easy-to-use network mapping tool.
SNA Case Studies
Looking for a real-world example of a social network analysis project? Here are three examples from recent projects here at Visible Network Labs.
Case Study 1: Leveraging SNA for Program Evaluation
SNA is increasingly becoming a vital tool for program evaluation across various sectors including public health, psychology, early childhood, education, and philanthropy. Its potency is particularly pronounced in initiatives centered around network-building.
Take for instance the Networks for School Improvement Portfolio by the Gates Foundation. The Foundation employed PARTNER, an SNA tool, to assess the growth and development of their educator communities over time. The SNA revealed robust networks that offer valuable benefits to members by fostering information exchange and relationship development. By repeating the SNA process at different stages, they could verify their ongoing success and evaluate the effectiveness of their actions and adjustments.
Read the Complete Case Study Here
Case Study 2: Empowering Coalition-building
In the realm of policy change, building a coalition of partners who share a common goal can be pivotal in overturning the status quo. SNA serves as a strategic tool for developing a coalition structure and optimizing pre-existing relationships among the members.
The Fix CRUS Coalition in Colorado, formulated in response to the closure of five major peaks to public access, is a prime example of this. With the aim of strengthening state liability protections for landowners, the coalition employed PARTNER to evaluate their network and identify key players. Their future plans involve mapping connections to important legislators as their bill progresses through the state legislature. Additionally, their network maps and reports will prove instrumental in acquiring grants and funding.
Case Study 3: Boosting Employee Engagement
In the private sector, businesses are increasingly harnessing SNA to optimize their employee networks, both formal and informal, with the goal of enhancing engagement, productivity, and morale.
Consider the case of Acuity Insurance. In response to a transition to a Hybrid-model amid the COVID-19 pandemic, the company started using PARTNER to gather network data from their employees. Their aim was to maintain their organizational culture and keep employee engagement intact despite the model change. Their ongoing SNA will reveal the level of connectedness within their team, identify employees who are over-networked (and hence at risk of burnout), and pinpoint those who are under-networked and could be missing crucial information or opportunities.
Read More About the Project Here
Challenges and Future Directions in Network Analysis
Like all fields and practices, social network analysis faces certain limitations. Practitioners are constantly innovating to find better ways to conduct projects. Here are some barriers in the field and current trends and predictions about the future of SNA.
The Limitations of SNA
SNA is a powerful tool, but it’s not without limitations. It can be time-consuming and complex, particularly with larger networks. Response rates are important to ensure accuracy, which makes data collection more difficult and time-consuming. SNA also requires quality, validated data, and the interpretation of results can be subjective. Software that helps to address these problems requires a significant investment, but the results are often worth it.
Lastly, SNA is a skill that takes time and effort to learn. If you do not have someone in-house with network analysis skills, you may need to hire someone to carry out the analysis or spend time training an employee to build the capacity internally.
Current Trends and Future Predictions
One emerging trend is the increased application of SNA in mapping inter-organizational networks such as strategic partnerships, community health ecosystems, or policy change coalitions. Organizations are realizing the power of these networks and using SNA to navigate them more strategically. With SNA, they can identify key players, assess the strength of relationships, and strategize on how to optimize their network for maximum benefit.
In line with the rise of data science, another trend is the integration of advanced analytics and machine learning with SNA. This fusion allows for the prediction of network behaviors, identification of influential nodes, and discovery of previously unnoticed patterns, significantly boosting the value derived from network data.
The future of SNA is likely to see a greater emphasis on dynamic networks – those that change and evolve over time. With increasingly sophisticated tools and methods, analysts will be better equipped to track network changes and adapt strategies accordingly.
In addition, there is a growing focus on inter-organizational network resilience. As global challenges such as pandemics and climate change underscore the need for collaborative solutions, understanding how these networks can withstand shocks and adapt becomes crucial. SNA will play an instrumental role in identifying weak spots and strengthening the resilience of these networks.
Conclusion: Social Network Analysis 101
SNA offers a unique way to visualize and analyze relationships within a network, be it within an organization or between organizations. It provides valuable insights that can enhance communication, improve efficiency, and inform strategic decisions.
This guide provides an overview of SNA, but there is much more to learn. Whether you’re interested in the theoretical underpinnings, advanced techniques, or the latest developments, we encourage you to delve deeper into this fascinating field.
Resources and Further Reading
For those who want to build more SNA skills and learn more about network science, check out these recommendations for further reading and exploration from the Visible Network Labs team of network science experts.
Recommended Books on SNA
- “Network Science” by Albert-László Barabási – A comprehensive introduction to the theory and applications of network science from a leading expert in the field.
- “Analyzing Social Networks” by Steve Borgatti, Martin Everett, and Jeffrey Johnson – An accessible introduction, complete with software instructions for carrying out analyses.
- “Social Network Analysis: Methods and Applications” by Stanley Wasserman and Katherine Faust – A more advanced, methodological book for those interested in a deep dive into the methods of SNA.
- “Connected: The Surprising Power of Our Social Networks and How They Shape Our Lives” by Nicholas Christakis and James Fowler – An engaging exploration of how social networks influence everything from our health to our political views.
- “The Network Imperative: How to Survive and Grow in the Age of Digital Business Models” by Barry Libert, Megan Beck, and Jerry Wind – An excellent book for those interested in applying network science in a business context.
- “Networks, Crowds, and Markets: Reasoning About a Highly Connected World” by David Easley and Jon Kleinberg – An interdisciplinary approach to understanding networks in social and economic systems. This book combines graph theory, game theory, and market models.
Online Resources and Courses
Here are some online learning opportunities, including online courses, communities, resources hubs, and other places to learn about social network analysis.
- Social Network Analysis by Lada Adamic from the University of Michigan
- Social and Economic Networks: Models and Analysis by Matthew O. Jackson from Stanford University
- Introduction to Social Network Analysis by Dr. Jennifer Golbeck from the University of Maryland, College Park
- Statistics.com : Statistics.com offers a free online course called Introduction to SNA taught by Dr. Jennifer Golbeck.
- The Social Network Analysis Network: This website provides a directory of resources on network methods, including courses, books, articles, and software.
- The SNA Society: This organization provides a forum for social network analysts to share ideas and collaborate on research. They also offer a number of resources on their website, including a list of online courses.
Journals and Research Papers on SNA
These are a few of the most influential cornerstone research papers in network science and analysis methods:
- “The Strength of Weak Ties” by Mark Granovetter (1973)
- “Structural Holes and Good Ideas” by Ronald Burt (2004)
- “ Collective dynamics of ‘small-world’ networks” by Duncan Watts & Steven Strogatz (1998)
- “The structure and function of complex networks.” by M.E. Newman (2003).
- “Emergence of scaling in random networks” by A. Barabasi (1999).
Check out these peer-reviewed journals for lots of network science content and information:
- Social Networks : This is an interdisciplinary and international quarterly journal dedicated to the development and application of network analysis.
- Network Science : A cross-disciplinary journal providing a unified platform for both theorists and practitioners working on network-centric problems.
- Journal of Social Structure (JoSS) : An electronic journal dedicated to the publication of network analysis research and theory.
- Connections : Published by the International Network for Social Network Analysis (INSNA), this journal covers a wide range of social network topics.
- Journal of Complex Networks : This journal covers theoretical and computational aspects of complex networks across diverse fields, including sociology.
Frequently Asked Questions about SNA
A: SNA is a research method used to visualize and analyze relationships and connections within a network. In an organizational context, SNA can be used to explore the structure and dynamics of an organization, such as the informal connections that drive formal processes. It can reveal patterns of communication, identify influential entities, and detect potential bottlenecks or gaps.
A: The primary purpose of SNA is to uncover and visualize the relationships between entities within a network. By doing so, it allows us to understand the network’s structure and dynamics. This insight can inform strategic decision-making, facilitate change management, and enhance overall efficiency within an organization.
A: SNA allows researchers to examine the relationships between entities, the overall structure of the network, and the roles and importance of individual entities within it. This can involve studying patterns of communication, collaboration, competition, or any other type of relationship that exists within the network.
A: SNA has a wide range of applications across various fields. In business, it’s used to analyze organizational structures, supply chains, and market dynamics. In public health, it can map the spread of diseases. In sociology and anthropology, SNA is used to study social structures and relationships. Online, SNA is used to study social media dynamics and digital marketing strategies.
A: Key concepts in SNA include nodes (entities) and edges (relationships), network properties like density and centrality, and theories such as the Strength of Weak Ties and Structural Hole Theory. It also encompasses concepts like homophily and heterophily, which describe the tendency for similar or dissimilar nodes to connect.
A: An example of SNA could be a study of communication within a corporation. By treating departments as nodes and communication channels as edges, analysts could visualize the communication network, identify key players, detect potential bottlenecks, and suggest improvements.
A: Social Network Analysis refers to the method of studying the relationships and interactions between entities within a network. It involves mapping out these relationships and applying various analytical techniques to understand the structure, dynamics, and implications of the network.
A: In psychology, SNA can be used to study the social relationships between individuals or groups. It might be used to understand the spread of information, the formation of social groups, the dynamics of social influence, or the impact of social networks on individual behavior and well-being.
A: SNA can be conducted at different levels, depending on the focus of the study. The individual level focuses on a single node and its direct connections (ego networks). The dyadic level looks at the relationship between pairs of nodes, while the triadic level involves three nodes. The global level (whole network) considers the entire network.
A: There are several types of networks in SNA, including ego networks (focused on a single node), dyadic and triadic networks (focused on pairs or trios of nodes), and whole networks. Networks can also be categorized by their structure (like centralized or decentralized), by the type of relationships they represent, or by their application domain (such as organizational, social, or online networks).
A: SNA is used to visualize and analyze the relationships within a network. Its insights can inform strategic decisions, identify influential entities, detect potential weaknesses or vulnerabilities, and enhance the efficiency of communication or processes within an organization or system. It’s also an essential tool for research in fields like sociology, anthropology, business, public health, and digital marketing.
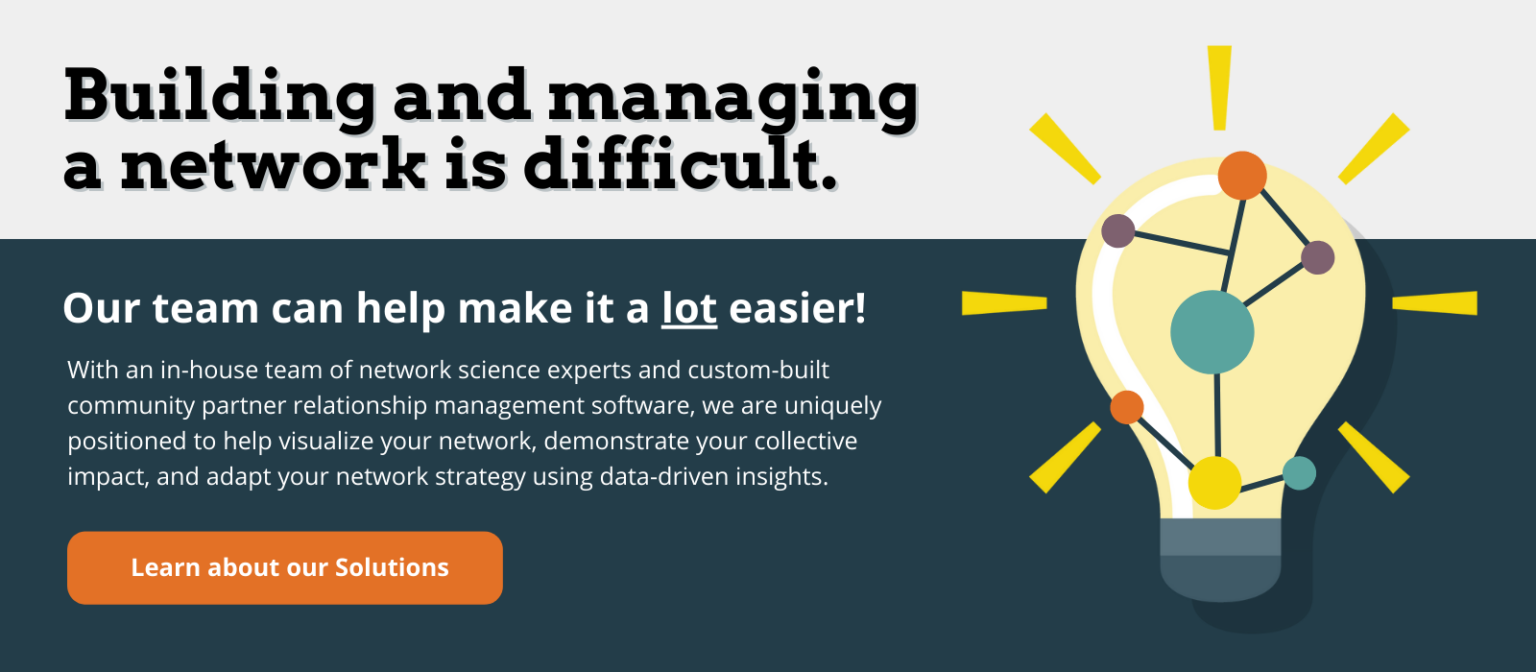
Connect with our Team!
Contact the VNL team to demo PARTNER™ or discuss a research or evaluation project. We can help you learn more about our services, help brainstorm project designs, and provide a custom scope based on your budget and needs. We look forward to connecting!
Email our team: [email protected]
Send a message: Contact Us Here
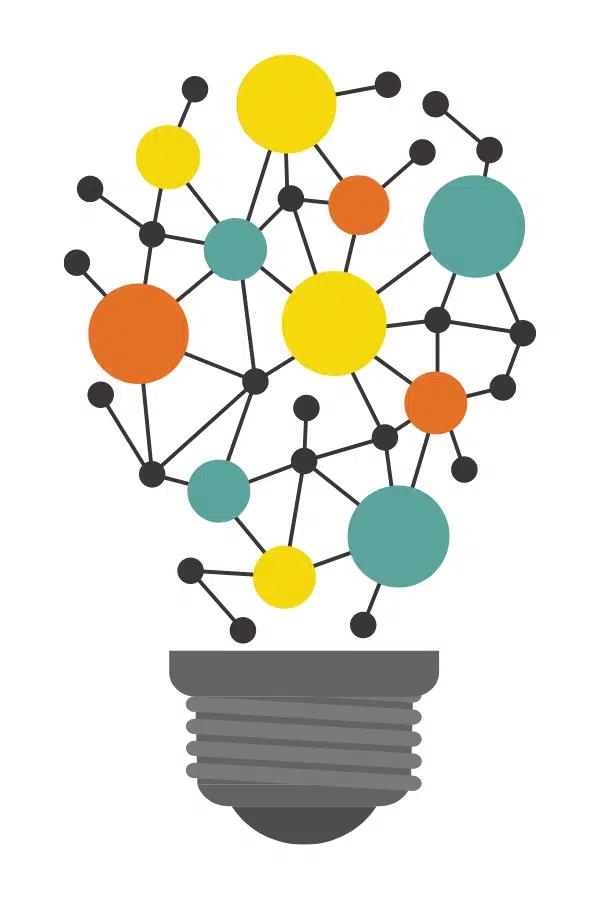
Get Involved!
Let us know how you’d like to get involved with the Jeffco PARTNER CPRM by choosing from the options below.
Join our next webinar: Marketing & Communication Strategies & Tactics for Networks & Coalitions
Choose a free gift.
Click one of the links below to download a free resource to strengthen your community partnerships, collaborative network, and strategic ecosystem.
Network Leadership Guide
Advice for building, managing, and assessing cross-sector networks or coalitions of partners.
Ecosystem Mapping Template
A template to map the connections and interactions between key stakeholders in your community.
Network Strategy Planner
A worksheet and guide to help you think through and develop your network or ecosystem strategy.
Subscribe to our Network Science Newsletter!
Get monthly updates on VNL news, new research, funding opportunities, and other resources related to network and ecosystem mapping and management.
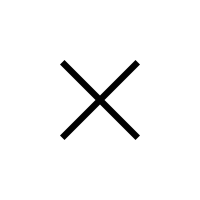
A Guide to Social Network Analysis and its Use Cases
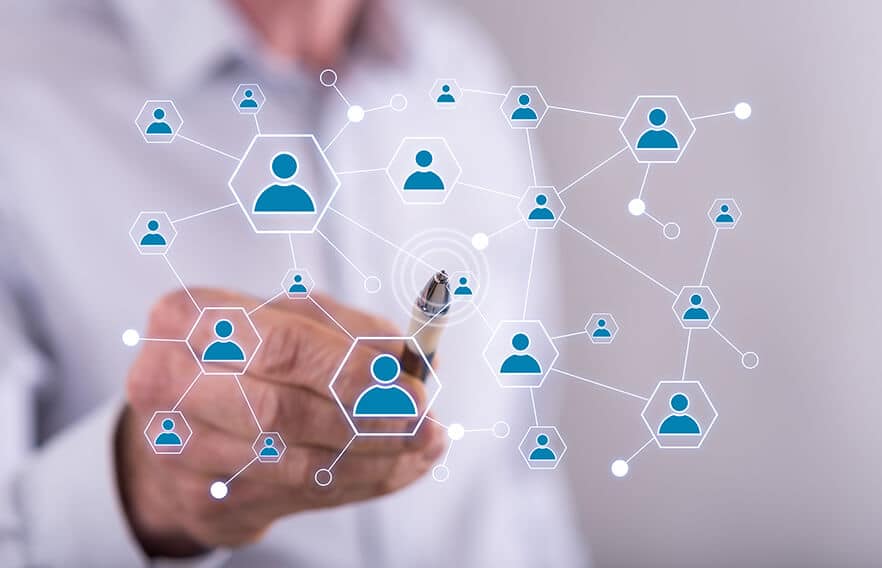
- Last Updated on April 23, 2021
In today’s world of limitless connectivity, multiple devices, unlimited choices, several individual personas, there is something sublime unifying all of the above. There is an invisible thread connecting all the dots despite the digital growth happening every day. According to the Chaos Theory, something as small as the flutter of a butterfly’s wing can ultimately cause a typhoon halfway around the world.
In other words, we are a part of a network in all stages of our lives, be it a social network like friends or family, an organization network like an educational institution or workplace. The networks we are a part of also include a social media network where we connect with people across the world or even a consumer network as users of various brands. Thus, networks are all around us.
The concept of networks and extracting information has untapped potential, be it a social setting, consumer behavior, health management, education, politics. Though intellectuals have started seeing the benefits of identifying social groups for various applications, this concept has not become mainstream in the business world. This blog delves into SNA (Social Network Analysis) and how it can be used to analyze and solve business-related problems.
What is SNA?
Social Network Analysis (SNA), also known as network science, is a general study of the social network utilizing network and graph theory concepts. It explores the behavior of individuals at the micro-level, their relationships (social structure) at the macro level, and the connection between the two.
SNA uses several methods and tools to study the relationships, interactions, and communications in a network. This study is key to procedures and initiatives involving problem-solving, administration, and operations of that network.
The basic entities required for building a network are nodes and the edges connecting the nodes. Let us try and understand this with the help of a most common application of SNA, the Internet. Webpages are often linked to other web pages on their own page or other pages. In SNA language, these pages are nodes, and the links between the pages are the edges. In this way, we can interpret the entire internet as one large graph.
SNA is a commonly used approach for analyzing interpersonal connections on the internet due to the boom of social media networking. But this concept is not limited to online social networks; it can be used for any application that can be modeled as a network.
A Guide to the Most Used SNA Terminologies
As established earlier, nodes and edges are the building blocks for SNA. Few characteristics of the edges that define the features of a network are shown below.
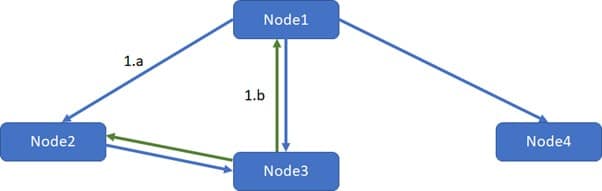
The Edges connect the Nodes. The direction of connections determines the Edge type.
1.a Directed Edge: The nodes connected by this edge are ordered, that is, the connection between the nodes is one way. For example, Twitter, Instagram are predominantly directed edge networks. You can follow someone without them following you back.
1.b Undirected Edge: The relationship between the nodes connected by this edge is mutual, i.e., the connection is applicable both ways. E.g., Befriending a person on Facebook, LinkedIn automatically creates a two-way connection.
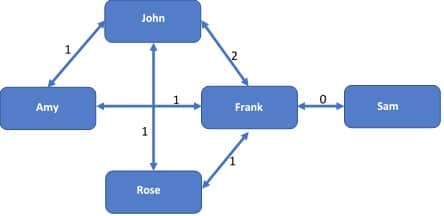
2. Weight: In a weighted network, an edge carries a label (weight) between the nodes. Different applications can have their own definition of weight. In social media analysis, a weight can define the number of mutual connections between the nodes connected by that edge.
In Figure 2 , John and Frank have two mutual friends, Rose and Amy. Thus, the edge connecting John and Frank carries a weight of 2.
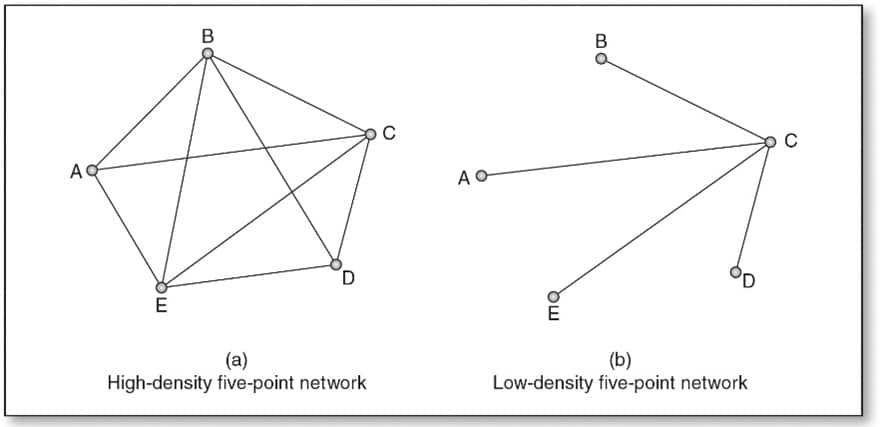
3. Density : The relation between the number of existing connections in a network and all possible connections in the network is calculated as follows:
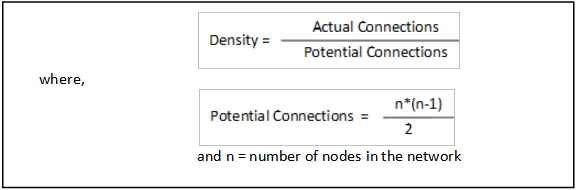
In Figure 3 , we have a five-point/node network. The total possible connections in this network are 10. Figure 3.a has nine edges; its density is 90%. Hence it is a high-density network. Whereas Figure 3.b has only four edges, it has a low density of 40%.
Centrality Measures:
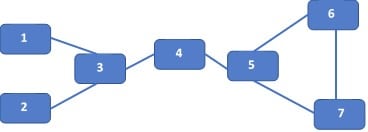
a) Degree Centrality: Measures the number of direct ties to a node; this will indicate the most connected node in the group.
Let’s consider the network in Figure 4 . The degree centrality score of a network is the sum of edges connected to that node. For Node 1, the degree centrality is 1, and for Nodes 3 and 5, the score is 3.
The standardized score is calculated by dividing the score by (n-1), where n is the number of nodes in the network.

We can see that nodes 3 and 5 have a high degree centrality of 0.5, i.e., they are the most well-connected nodes in the network.
b) Closeness Centrality: Closeness measures how close a node is to the rest of the network. It is the ability of the node to reach the other nodes in the network. It is calculated as the inverse of the sum of the distance between a node and other nodes in the network.
Let us take node 1 from Figure 4 ; the sum of distances from node 1 to all other nodes is 16.

Hence the Closeness score for node 1 will be 1/16. The standardized score is calculated by multiplying the score by (n-1).

We can conclude that node 4 is the closest/central node in the network with the highest closeness score of 0.6.
c) Betweenness Centrality: It is a measure of how often a node appears in the shortest path connecting two other nodes. Let us take node 5 in Figure 4 . Node 5 occurs in 9 shortest paths between a pair of nodes (as shown in Table 4 ).

If node 5 is the only node in the path, then the path value is 1. If it is one of the ‘n’ nodes in the shortest path, then the path value is 1/n. The sum of path values for node 5 for all nine pairs of nodes is its betweenness score. These values are then standardized by dividing the score by (n-1)*(n-2)/2

Nodes with high betweenness centrality are critical in controlling and maintaining flow in the network; hence these are critical nodes in the network
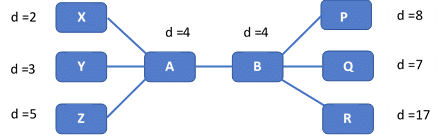
. d) Eigenvector Centrality: A relative measure of the importance of the node in the network. Each node is assigned a value or score depending upon the number of other prominent/ high scoring nodes it is connected to.
Why do we need such a relative measure? Consider the network in Figure 5 . Here ‘d’ represents the degree centrality score. Nodes A and B are connected to 4 nodes each, and hence both have a degree centrality score of 4. But when we look at their neighbors, we can see that node B is connected to nodes with a high degree. Hence, node B can be preferred over node A when we have to choose based on connectivity.
Real-world use cases of Social Network Analysis:
1. Supply Chain Management: A supply chain can be modeled into a network of supplier/consumer relations. Network analysis on the supply chain helps us improve the operation efficiency by identifying and eliminating less important nodes (suppliers/warehouses). It can help identify crucial nodes in the network and create a standby in crises or emergencies.
Nodes include Retailers, Suppliers, Warehouses, Transporters, Regulatory agencies.
SNA applications can help manufacturers identify more operationally critical nodes and identify potential sources to increase the number of connections to suppliers. This can also help identify any bottlenecks in the supply process and inventory management.
2. Human Resources: HRM often strives to identify critical resources and understand their contribution to the organization flow, collaboration, participation, and information flow. By following the Organizational Network Analysis (ONA), an organization will optimize the talent connections, productivity, and utilization.
It will also help identify the reach of an individual, identify accelerators of growth and poorly connected resources, and decide whom to give more opportunity.
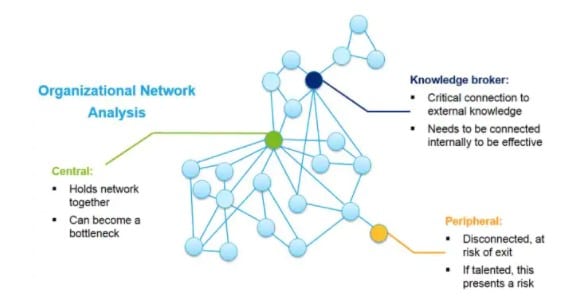
3. Transmission of Infectious Diseases: SNA could help identify and isolate individuals and groups with high betweenness and out-degree centrality (transmitters of disease) and implement sound contact tracing activities to mellow the impact.
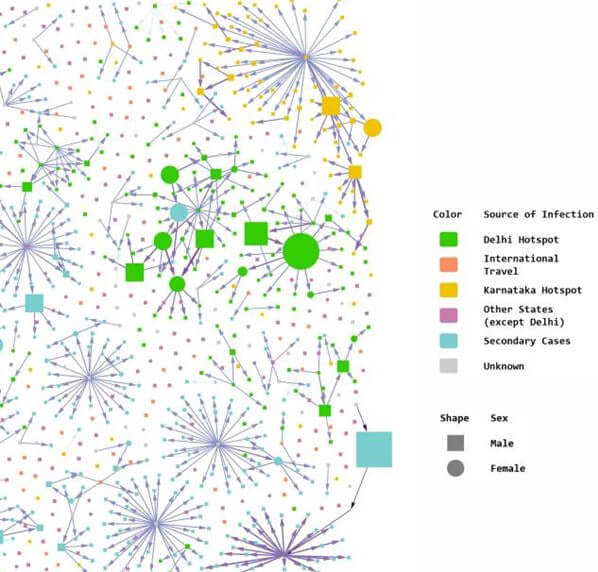
Apart from Contact tracing, SNA can also identify dominant themes and relations between keywords and identify the sentiment. Here is the connection between the top 10 words for COVID-19 themes:
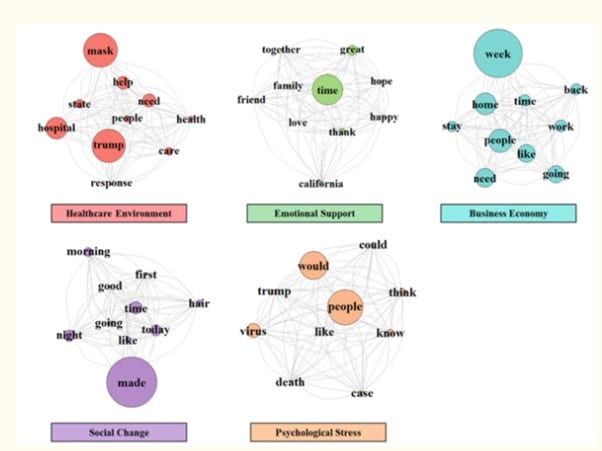
4. Finance, Fraud detection : Financial organizations can use SNA for fraud detection. Fraud is often organized by groups of people loosely connected to each other. Such a network mapping will enable financial institutions to identify customers who may have relations to individuals or organizations on their criminal watchlist (network) and take precautionary measures.
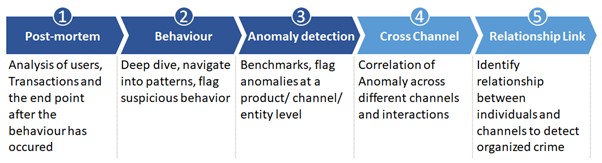
SNA can also be used to deny access to potential hacking networks, identify a fraud ring, and series of money transactions that could be linked to Money Laundering activities.
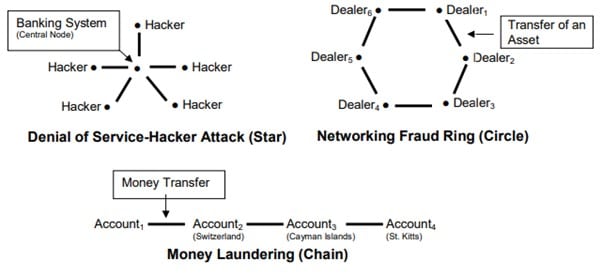
As a Business Leader, you will have to make many critical decisions regarding effective employee performance, supply chain management , and eliminating bottlenecks in an operation process, contact tracking, credit risks, and several other use cases. SNA has immense potential to elevate existing analysis, given there is information flow and connections.
Get in touch with us or mail us at [email protected] to know how SNA can be applied to add value to your business.
References:
- https://towardsdatascience.com/how-to-get-started-with-social-network-analysis-6d527685d374
- https://www.sciencedirect.com/science/article/pii/S2212017315001528
- https://bmcmedresmethodol.biomedcentral.com/articles/10.1186/s12874-020-01119-3
- https://www.cgi.com/sites/default/files/white-papers/Implementing-social-network-analysis-for-fraud-prevention.pdf
- https://www.mphasis.com/content/dam/mphasis-com/global/en/nextlabs/resources/home/whitepapers/Social-Network-Analytics-for-Fraud-Detection.pdf
POPULAR BLOGS
Data warehousing vs data engineering: which is right for your business, role of artificial intelligence in supply chain analytics, mitigating risks in financial services using predictive analytics, related blogs.
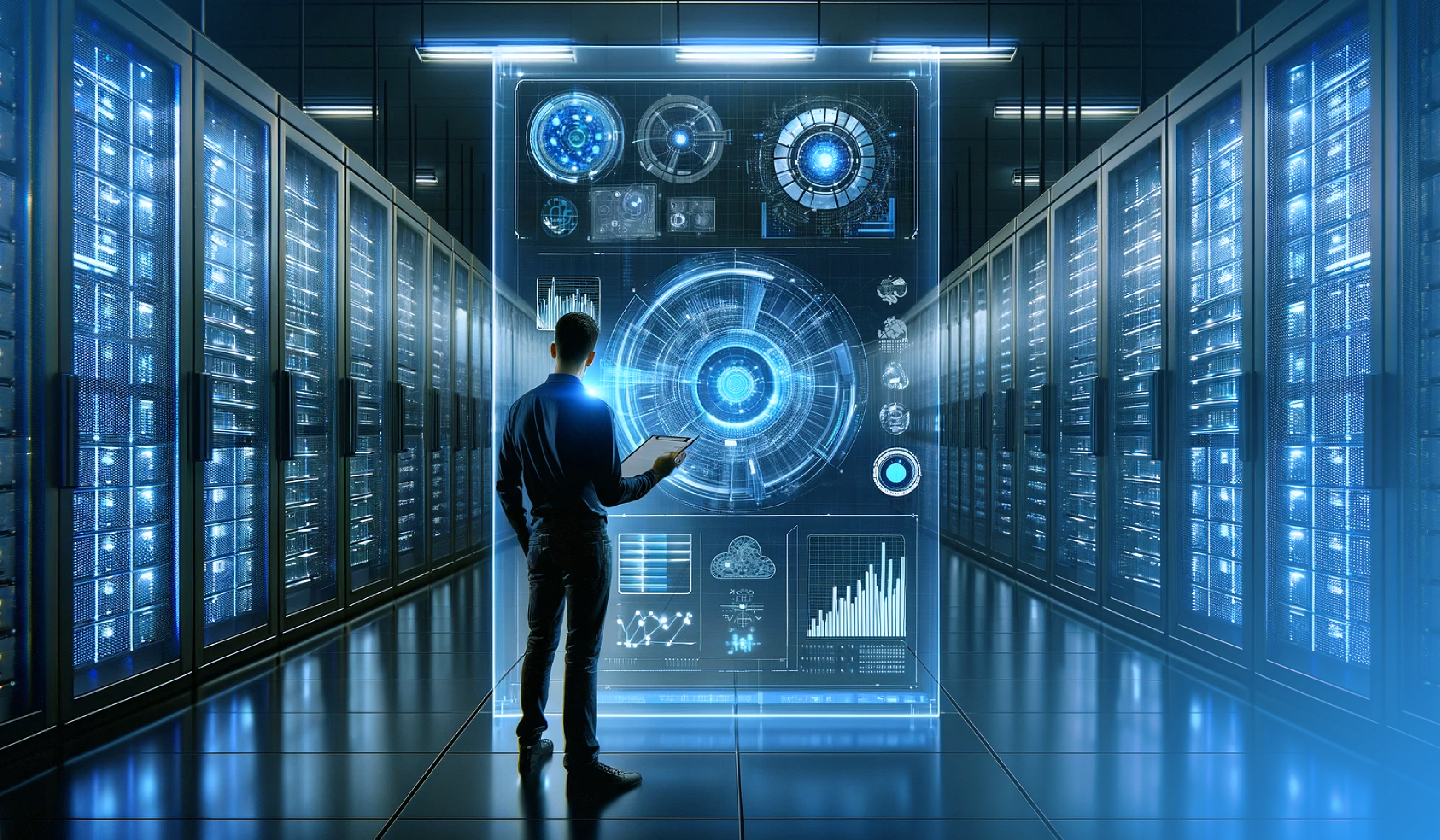
- May 9, 2024
Is Unity Catalog Right for You? A Guide to Databricks’ Next-Gen Data Governance
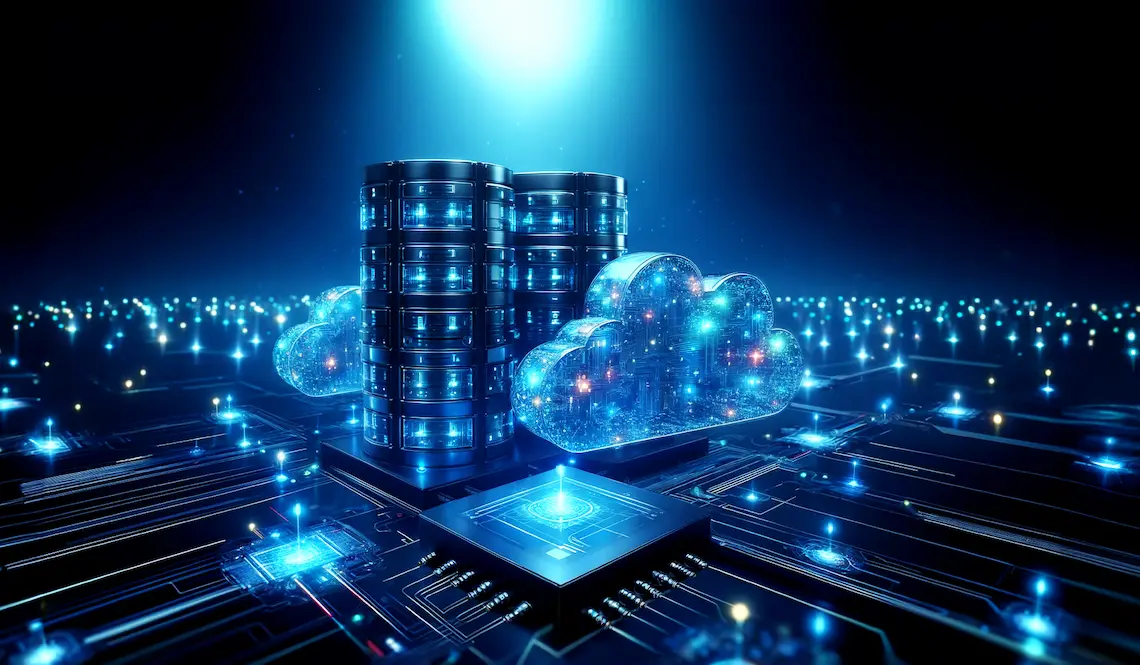
- April 5, 2024
Migrating Data Back from Cloud to On-Premise with Java and Talend
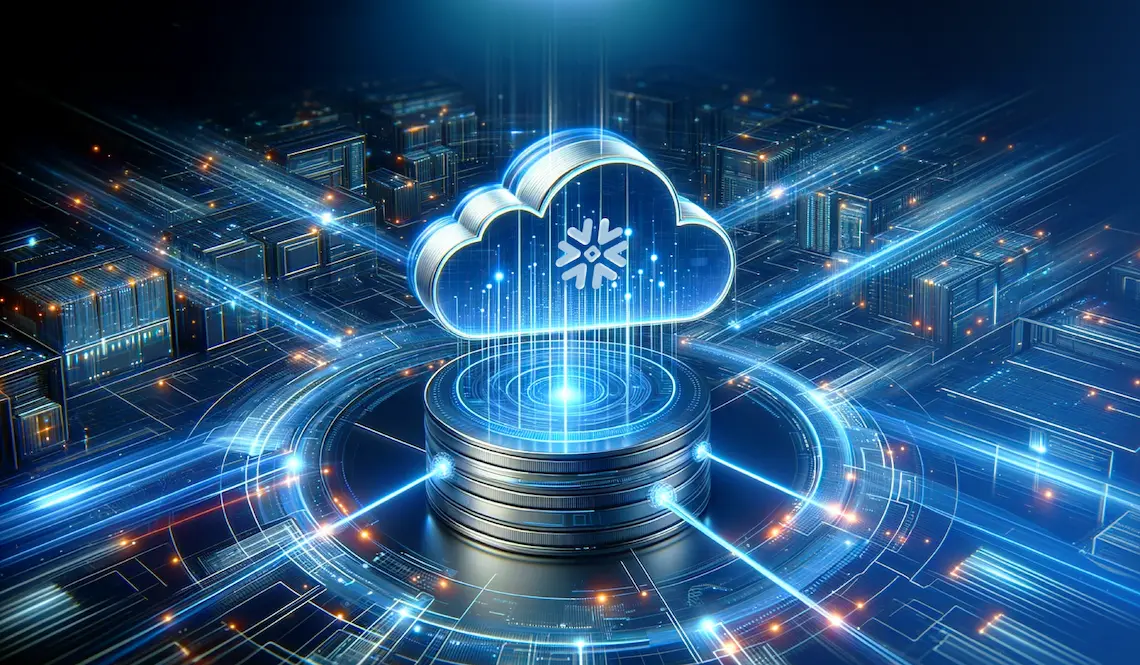
- March 27, 2024
Automate, Integrate, and Empower: Data Migration Made Easy with Streamlit and Snowpark
(re)think data think latentview, technical expertise, domain expertise, advisory services.
- Financial Services
- Industrials
- Case Studies
- Infographics
Whitepapers
- Leadership Team
- Corporate Social Responsibility
- Advisory Council
- Alumni Portal
Privacy Policy
- Partnerships
- Data Engineering
- Data Science
- Data Visualization
- Customer Analytics
- Marketing Analytics
- Supply Chain Analytics
- HR Analytics
- Financial Analytics
- Analytics Roadmap
- GenAI Readiness
- ConnectedView
- InsightLens
- MigrateMate
- OneCustomerView
- Smart Innovation
- Investor Relations
- Tools and Resources
- Customer Services
- Business Education
- Business Law
- Business Policy and Strategy
- Entrepreneurship
- Human Resource Management
- Information Systems
- International Business
- Negotiations and Bargaining
- Operations Management
- Organization Theory
- Organizational Behavior
- Problem Solving and Creativity
- Research Methods
- Social Issues
- Technology and Innovation Management
- Share This Facebook LinkedIn Twitter
Article contents
Social network analysis in organizations.
- Jessica R. Methot , Jessica R. Methot School of Management and Labor Relations, Rutgers University; Exeter Business School, University of Exeter
- Nazifa Zaman Nazifa Zaman School of Management and Labor Relations, Rutgers University
- and Hanbo Shim Hanbo Shim School of Management and Labor Relations, Rutgers University
- https://doi.org/10.1093/acrefore/9780190224851.013.228
- Published online: 23 March 2022
A social network is a set of actors—that is, any discrete entity in a network, such as a person, team, organization, place, or collective social unit—and the ties connecting them—that is, some type of relationship, exchange, or interaction between actors that serves as a conduit through which resources such as information, trust, goodwill, advice, and support flow. Social network analysis (SNA) is the use of graph-theoretic and matrix algebraic techniques to study the social structure, interactions, and strategic positions of actors in social networks. As a methodological tool, SNA allows scholars to visualize and analyze webs of ties to pinpoint the composition, content, and structure of organizational networks, as well as to identify their origins and dynamics, and then link these features to actors’ attitudes and behaviors. Social network analysis is a valuable and unique lens for management research; there has been a marked shift toward the use of social network analysis to understand a host of organizational phenomena. To this end, organizational network analysis (ONA) is centered on how employees, groups, and organizations are connected and how these connections provide a quantifiable return on human capital investments. Although criticisms have traditionally been leveled against social network analysis, the foundations of network science have a rich history, and ONA has evolved into a well-established paradigm and a modern-day trend in management research and practice.
- social networks
- social network analysis
- organizational networks
- network theory
- social capital
- network dynamics
- multiplexity
You do not currently have access to this article
Please login to access the full content.
Access to the full content requires a subscription
Printed from Oxford Research Encyclopedias, Business and Management. Under the terms of the licence agreement, an individual user may print out a single article for personal use (for details see Privacy Policy and Legal Notice).
date: 27 May 2024
- Cookie Policy
- Privacy Policy
- Legal Notice
- Accessibility
- [66.249.64.20|195.158.225.244]
- 195.158.225.244
Character limit 500 /500

What do you think about us?
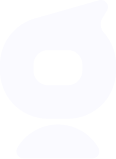
Social Network Analysis in Business Studies
Concept map.
Social Network Analysis (SNA) is an interdisciplinary approach that applies network and graph theory to analyze social structures in business. It helps in mapping connections to understand organizational behavior, strategic decision-making, and performance. SNA techniques like Centrality Measures and Clique Analysis offer insights into network influence and cohesion, aiding in resource allocation, innovation, and competitive positioning.
Definition and Importance of Social Network Analysis
Interdisciplinary approach.
Social Network Analysis (SNA) is an interdisciplinary approach that uses network and graph theory to analyze social structures in business
Strategic Insights and Benefits
Resource Allocation and Collaboration
SNA provides strategic insights for informed decision-making in business, including resource allocation and promoting collaboration
Innovation and Competitive Advantage
SNA can drive innovation and secure a competitive advantage for businesses
Risk Identification and Organizational Resilience
SNA plays a crucial role in identifying potential risks and exposing interdependencies that may affect organizational resilience
Applications in Digital Marketing and Knowledge Management
SNA is valuable in understanding consumer behavior and guiding marketing strategies, as well as revealing hidden information pathways and promoting interdisciplinary collaboration in knowledge management
Analytical Techniques in Social Network Analysis
Centrality measures.
Centrality measures, such as Degree, Closeness, Betweenness, and Eigenvector, are used to quantify the influence of nodes within a network
Cohesion Measures
Cohesion measures evaluate the solidarity of a network and are essential for analyzing social ties
Other Analytical Techniques
Other techniques, such as Clique Analysis and Ego Network Analysis, are used to dissect and interpret complex social networks
Practical Applications of Social Network Analysis
Corporate sector.
SNA can expose communication patterns and informal networks that are pivotal for organizational effectiveness in the corporate sector
SNA helps identify influencers and connectors within consumer networks, guiding targeted marketing campaigns
Knowledge Management
SNA is instrumental in revealing hidden information pathways and promoting interdisciplinary collaboration in knowledge management
Challenges and Limitations of Social Network Analysis
Data collection and analysis.
The intricacies of data collection and the fluid nature of social networks can make analysis daunting
Privacy and Misinterpretation Concerns
Careful management of data privacy and the risk of misinterpretation is necessary when using SNA
Effectiveness and Adaptability
The visualization tools and adaptability of SNA across disciplines make it an invaluable analytical instrument, but its effectiveness depends on recognizing and mitigating its limitations
Want to create maps from your material?
Enter text, upload a photo, or audio to Algor. In a few seconds, Algorino will transform it into a conceptual map, summary, and much more!
Learn with Algor Education flashcards
Click on each card to learn more about the topic.
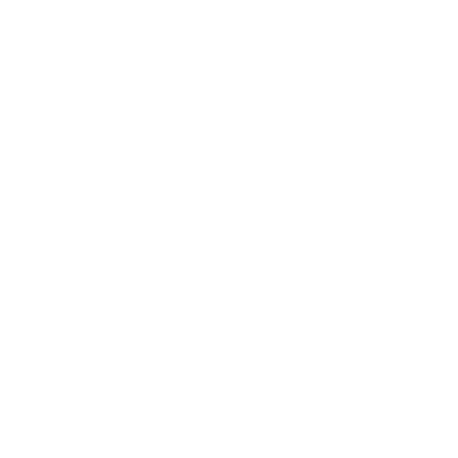
Disciplines involved in SNA
Interdisciplinary approach combining network and graph theory.
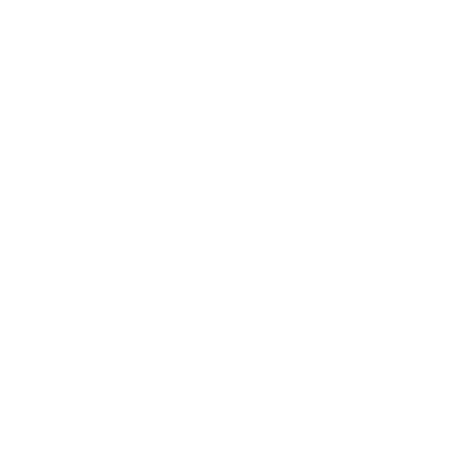
SNA's function in organizational strategy
Analyzes relational dynamics to influence strategic business decisions.
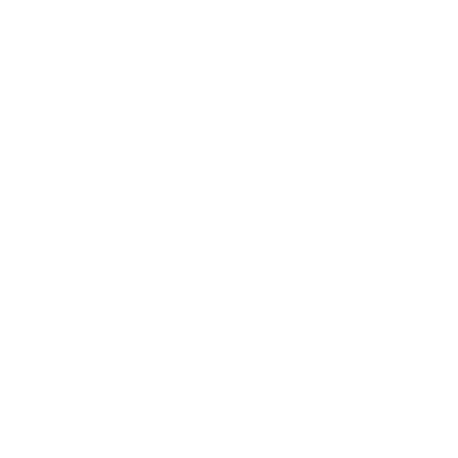
SNA's impact on understanding organizational behavior
Reveals informal and formal networks that determine organizational success.
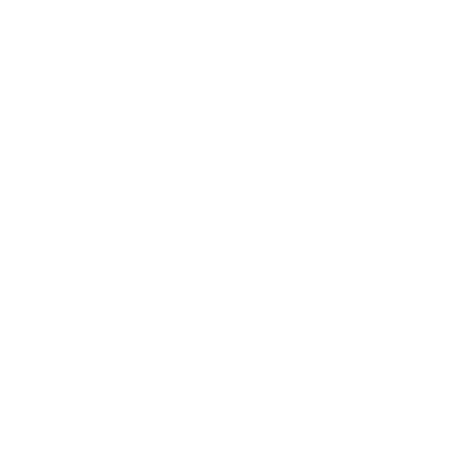
______ in businesses provides key insights for ______ decision-making.
Social Network Analysis informed
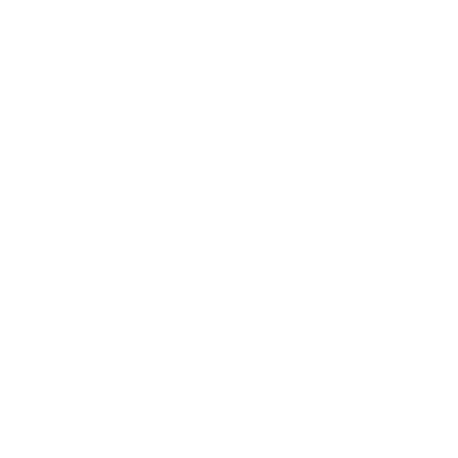
In ______ ______, SNA helps comprehend consumer behavior and the influence of ______ interactions on marketing approaches.
digital marketing online
Centrality Measures Purpose
Identify key influencers within a network.
Clique Analysis Focus
Detect cohesive subgroups within a network.
Cohesion Measures Evaluation
Assess the solidarity and unity of the entire network.
In Social Network Analysis (SNA), ______ Centrality measures a node's influence by the number of its direct connections.
______ Centrality is a metric in SNA that reflects a node's ability to spread information efficiently, based on its average distance to other nodes.
SNA in Corporate Sector
Reveals informal networks and communication patterns, crucial for organizational effectiveness.
SNA Role in Marketing
Identifies influencers and connectors, aids in crafting targeted campaigns.
SNA in Knowledge Management
Uncovers hidden information pathways, fosters interdisciplinary collaboration.
______ Analysis can uncover hidden connections and enhance ______ abilities but is complicated by data gathering and changing social structures.
Social Network predictive
SNA Research Methodology
Analyzes dynamics of networks in social/organizational contexts to understand patterns and interactions.
SNA in Strategic Decision-Making
Provides insights for strategy by revealing trends in relationships and aiding in prediction and innovation.
SNA Visualization and Predictive Power
Enables depiction of complex networks and forecasts future patterns, crucial for business and academic research.
Here's a list of frequently asked questions on this topic
What is the role of social network analysis in business studies, how does social network analysis benefit enterprises strategically, what are some analytical techniques used in social network analysis, why are centrality measures important in sna, can you name some fields where social network analysis is applied, what are the benefits and challenges of using social network analysis, what is the overall significance of social network analysis, similar contents, explore other maps on similar topics.
Communication Channels in Organizational Settings
The Communication Process
Decision-Making in Organizations
Oral Communication
Ethical Decision Making in Business
Transactional Leadership
Organizational Leadership
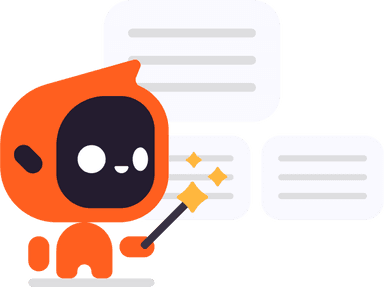
Can't find what you were looking for?
Search for a topic by entering a phrase or keyword
Exploring the Fundamentals of Social Network Analysis in Business
Strategic Implications of Social Network Analysis in Enterprises
The importance of centrality measures in assessing network influence, diverse applications of social network analysis, benefits and limitations of social network analysis, concluding insights on social network analysis.
Edit available

An official website of the United States government
The .gov means it’s official. Federal government websites often end in .gov or .mil. Before sharing sensitive information, make sure you’re on a federal government site.
The site is secure. The https:// ensures that you are connecting to the official website and that any information you provide is encrypted and transmitted securely.
- Publications
- Account settings
Preview improvements coming to the PMC website in October 2024. Learn More or Try it out now .
- Advanced Search
- Journal List
- v.8(4); 2022 Apr

Social network analysis in business and management research: A bibliometric analysis of the research trend and performance from 2001 to 2020
Adhe rizky anugerah.
a Bioresource Management Lab, Institute of Tropical Forestry and Forest Products (INTROP), Universiti Putra Malaysia, 43400 Serdang, Selangor, Malaysia
Prafajar Suksessanno Muttaqin
b Department of Logistics Engineering, School of Industrial and System Engineering, Telkom University, 40257 Bandung, Indonesia
Wahyu Trinarningsih
c Faculty of Economics and Business, Universitas Sebelas Maret, 57126 Surakarta, Indonesia
Associated Data
Data included in article/supplementary material/referenced in article.
In the past years, research in Social Network Analysis (SNA) has increased. Initially, the research area was limited to sociology and anthropology but has now been used in numerous disciplines. The business and management discipline has many potentials in employing the SNA approach due to enormous relational data, ranging from employees, stakeholders to organisations. The study aims to analyse the research trend, performance, and the utilisation of the SNA approach in business and management research. Bibliometric analysis was conducted by employing 2,158 research data from the Scopus database published from 2001 to 2020. Next, the research quantity and quality were calculated using Harzing's Publish or Perish while VOSviewer visualised research topics and cluster analysis. The study found an upward trend pattern in SNA research since 2005 and reached the peak in 2020. Generally, six subjects under the business and management discipline have used SNA as a methodology tool, including risk management, project management, supply chain management (SCM), tourism, technology and innovation management, and knowledge management. To the best of the authors' knowledge, the study is the first to examine the performance and analysis of SNA in the overall business and management disciplines. The findings provide insight to researchers, academicians, consultants, and other stakeholders on the practical use of SNA in business and management research.
Social network analysis; Bibliometrics; Clustering analysis; Business and management; Literature.
1. Introduction
The SNA is a theory investigating the relations and interactions based on anthropology, sociology, and social psychology to assess social structures ( Erçetin and Neyişci, 2014 ). The social structure in a network theory comprises individuals or organisations named nodes linked through one or more types of interdependencies, such as friendship, kinship, financial exchange, knowledge or prestige ( Parell, 2012 ). The actors range across different levels, from individuals, web pages, families, large organisations, and nations. Nowadays, SNA usage has grown, utilised in anthropology and sociology and several fields of science, including business and management disciplines.
However, studies on the SNA trends and applications in business and management are limited. Although published articles provide a catalogue of SNA concepts, they lack explanatory mechanisms on its application ( Borgatti and Li, 2009 ). Thus, the study aims to assess publication performances and explore SNA usage in business and management studies using Bibliometric analysis. The bibliometric methodology has been widely used to provide quantitative analysis of written publications using statistical tools ( Ellegaard and Wallin, 2015 ). It can help detect established and emergent topical areas, research clusters and scholars, and others ( Fahimnia et al., 2015 ). This analysis reveals important publications and objectively depicts the linkages between and among articles about a specific research topic or field by examining how frequently they have been co-cited by other published articles ( Fetscherin and Usunier, 2012 ).
Bibliometric analysis has at least two primary objectives: 1) to quantitatively measure the quality of journals or authors using statistical indicators such as citations rates ( Vieira et al., 2021 ), and 2) to analyse the knowledge structure and development of specific research fields ( Jing et al., 2015 ). Hence, the study addresses the following research questions: RQ1: What is the current research trend of SNA in business and management research ? RQ2: What is the most productive year of SNA in the business and management discipline ? RQ3: What are the most influential and productive institutions, authors, journals, and countries ? RQ4: What is the use of SNA in the business and management discipline and their cluster topics in the past 20 years ?
Several literature reviews and bibliometric papers on the use of SNA in general business and management areas have been published, but the number is limited. Monaghan, Lavelle, & Gunnigle (2017) analysed SNA usage in management research and practice to discuss the critical dimensions for handling and analysing network data for business research. The authors discovered four dimensions in initial engagement with SNA in business and management research: structure of research design, data collection, handling of data and data interpretation. Nonetheless, studies did not explain the distribution of research clusters and how SNA can be used in practical business and management research. Specifically, Su et al. (2019) conducted a Bibliometric analysis on SNA literature with no limitation of subject discipline and collected the data from Web of Science (WoS), covering 20 publication years from 1999 to 2018. Nevertheless, Su et al. (2019) mainly discussed the SNA publication performance but not how the approach was used previously.
In the more specific subject area of business and management, SNA has been explored to unveil the relationship between organisations, as conducted by Sozen et al. (2009) . The SNA has been used to measure the organisations' social capital, map resource dependency relations, and discover coalitions and cliques between organisations. Kurt and Kurt (2020) have explored the potential of SNA in international business (IB) research, because of two fundamental phemomena: firm internationalisation and multinational enterprises (MNEs). From the marketing perspective, SNA could detect the most influential actors to efficiently spread a message in online communities for marketing purposes ( Litterio et al., 2017 ).
The current study conducted a clustering analysis to identify and analyse SNA performance and its application in general business and management discipline using bibliometrics information. Thus, academicians, managers, consultants, and other stakeholders could understand when and how to apply the SNA approach. For instance, SNA can identify potential risks contributing to schedule delays in project risk management ( Li et al., 2016 ). The discussion section explores how SNA has been previously used in business and management research. Besides, the study addresses the problem in Borgatti and Li (2009) , exploring the actual application of SNA in management and business research.
2.1. Data sources and search strategy
The primary study objective is to analyse the research trend and explore the SNA approach in business and management research. A Bibliometric analysis was employed due to its accuracy in quantifying and evaluating scientific publications ( Carmona-Serrano et al., 2020 ). Additionally, the data were collected through the Scopus database. Although Scopus and WoS are the main and most comprehensive sources for Bibliometric analysis, Scopus has more advantages: more inclusive content coverage, more openness to society, and available individual profiles for all authors, institutions, and serial sources. Additionally, many papers have confirmed that Scopus provides wider overall coverage and Scopus indexing a greater amount of unique sources not covered by WoS ( Pranckutė, 2021 ). In the business, economics, and management area, 89% of articles listed in WoS are listed in Scopus. Hence, the study area (business and management) chose the Scopus database for further analysis.
The next step involved determining the search string, including all documents with the title, abstract, and keywords containing "network analysis" or "Social Network Analysis". These two main keywords are representative enough to reach the objective; they are not too wide and specific. The main goal is the utilisation of SNA as a concept and as a methodology can be widely captured. These two versions of keywords “Social Network Analysis” and “Network Analysis” without the “social” has a significant impact. Some articles did not put the complete sentence of SNA, although the articles mainly discussed the concept of network analysis. One of the examples is the ownership structure related research developed by Vitali et al. (2011) which changed the word "social network analysis" to "corporate network analysis". The term “social” in SNA refers to people interaction, while in the operation research, the relationship could be between airport, stakeholders, corporation, etc.
In the first run, 113,945 research related to SNA was found in the Scopus database, mainly Engineering and Computer Science fields. Besides, the search results were limited to the subject area in business, management, and accounting and covered publication from 2001 to 2020 (20 years). The study also excluded non-journal articles, such as conference proceedings, trade reports, book chapters, and others.
The search limitations have resulted in 2,881 articles, but many were still not related to network analysis or business and management. Further, 723 articles were excluded, covering articles in neuroscience, bibliometric, circuit network (engineering), earth and planetary science, chemistry, etc., although the articles employed network analysis as a methodology. The exclusion was also applied to articles that use SNA in multi-subject journals with little or no explanation in business, management, and accounting perspectives. One example is the Journal of Cleaner Production listed in four subject areas: business, management and accounting; energy; industrial and manufacturing engineering; and environmental science. In this journal, SNA theory is used to identify the relationship between ecosystems by measuring the flow of energy or material between organisms, which has little or no explanation from business and management perspectives. At the end of the search, 2,158 articles were extracted for further analysis. The flow chart on the data collection strategy is presented in Figure 1 .

The search strategy flow diagram (adopted from ( Zakaria et al., 2021 )).
2.2. Data analysis
The first stage involved analysing the data descriptively to identify the quality and quantity using standard Bibliometric measures ( Hirsch, 2005 ). The total number of publications (TP) assessed the quantity dimension, whereas other metrics assessed the quality dimension, such as total citation (TC), number of cited publications (NCP), average citation per publication (C/P), average citations per cited publication (C/CP) ( Hirsch, 2007 ). Additionally, the g-index ( g ) and h-index ( h ) are usually included in the Bibliometric measures to predict future achievement rather than standard measures. The indicators are applied to various levels: country-level, organisation-level, journal-level, and author-level. The information is processed and analysed using Harzing publish or perish (PoP) software by extracting Research Information System (RIS) data from the Scopus database.
The second stage visualised the research network to understand the relationship between nodes, including authors, affiliations (organisations), countries, citations, and keywords. Nonetheless, only keywords co-occurrence was carried out to examine the past, current, and future potential of SNA in business and management research. The study analysed the keywords based on the frequency, edges, and clusters. The combination between nodes (keywords) and edges (the relationship between keywords) form clusters with numerous research themes ( Dhamija and Bag, 2020 ). The bigger nodes show a higher occurrence in the keyword visualisations, and the thicker edges show the higher link strength. Meanwhile, cluster analysis in the study represents a set of similar keywords in one group, different in other groups to identify the research interest and keywords combination within the group. The cluster mapping was performed by VOSviewer, an open-access programme to construct and view Bibliometric maps ( van Eck and Waltman, 2010 ). Besides, the study developed an overlay visualisation to explore research evolution in SNA over time.
3.1. Description of retrieved literature
The study is limited to SNA research in business and management research published between 2001 to 2020. The study also excluded review papers, conference papers, editorials, and other documents besides journal articles for further analysis. Ultimately, the study retrieved a total of 2,158 articles. Although the search was limited to only English articles, the study identified seven bilingual articles in Spanish (3 articles), Chinese (2 articles), Lithuanian (1 article), and Portuguese (1 article). The articles had 58,522 citations, an average of 2,926 citations per year, and 27 citations per paper. The complete citation metrics for the articles are shown in Table 1 .
Table 1
Citations metrics.
Besides SNA as a primary keyword, the top keywords were "innovation" (6.16%), "project management" (3.48%), "knowledge management" (3.29%), "decision making" (2.97%), "complex networks" (2.69%), and others. The top keywords are listed in Table 2 . High-frequency keywords show the popularity of a specific topic ( Pesta et al., 2018 ). The listed keywords in the study usually appear together in SNA and are used to explore the potential use of SNA in business and management research.
Table 2
Top keywords.
3.2. Research Growth
Although SNA research productivity presented the ups and downs throughout the year, a consistent upward trend was found in the pattern. Research in the first five years (2001–2005) was limited and never reached 30 publications per year. In 2002, only 10 articles were published, increasing almost five times in 2007 (n = 49 documents). The number increased until 2020, slightly decreasing in 2011, 2015, and 2019. Meanwhile, the most productive year was in 2020, with 334 published articles.
The articles published in 2001 had the highest average citation per publication (c/p = 186.69). However, the highest h-index were in 2010 ( h = 43) and 2014 ( h = 36) which indicate high cumulative impact of the articles measured by its quantity with quality. Low citations per publication in recent years were expected due to increasing citation counts over time. The publication trend and average citations per publication are presented in Figure 2 .

Total publications and citations by year.
3.3. Top countries, institutions, and authors in SNA business and management research
The United States (US), the United Kingdom (UK), and China were the most prolific countries with 605, 230 and 215 articles, respectively. The study discovered no dominating continent that produced SNA business and management research, and all were equally distributed except for Africa. The top ten countries list (see Table 3 ) showed one North American, four Asian and Oceanian, and five European countries. As the top most productive countries, the United States and the United Kingdom published quality articles with highest average citation per publication of 39.34 and 32.80, respectively. Meanwhile, Asian countries had small citations (based on the top ten most productive countries): South Korea (c/p = 21.84) and China (c/p = 27.08) were at the bottom of the ranking according to the c/p calculation.
Table 3
The top ten countries contributed to the publications.
Notes: TP = total number of publications; NCP = number of cited publications; TC = total citations; C/P = average citations per publication; C/CP = average citations per cited publication; h = h-index; and g = g-index.
Hong Kong Polytechnic University was the most productive institution with 45 published articles and had the highest h- and g- index (see Table 4 ). The publication number was higher than the second most productive, Università Bocconi (n = 25). However, the top ten list showed that the University of Arizona had the highest c/p with 102.47, followed by the University of Kentucky (c/p = 58.40) and the Università Bocconi (c/p = 58.36). As the most productive country, there were four United States universities listed as the top most productive institutions but it only covered 10.9% from the total US articles. This indicates that the publications were distributed to other US institutions. Nevertheless, articles from two Hong Kong insitutions, Hong Kong Polytechic University and City University Hongkong accumulated 57 articles (82.61% of the country's total articles).
Table 4
Top 10 most influential institutions in SNA (business and management) research.
The two most productive institutions conducted different research themes. Figure 3 shows that Hong Kong Polytechnic University use SNA in numerous areas, such as "supply chain management", "transportation", "big data", "knowledge management", “stakeholder analysis in construction project” and others. Nonetheless, Università Bocconi research mostly involved tourism; the keywords were "tourist destination", "tourism management", "stakeholder", and “hospitality”. Besides, 17 of the 25 articles (68.0%) from Università Bocconi were written by Rodolfo Baggio, the author with the most article in SNA business and management. Table 5 presents the most productive authors with at least six published articles in SNA.

Research topic comparison between A) Hong Kong Polytechnic University and B) Università Bocconi.
Table 5
Most productive authors with a minimum of six articles.
Rodolfo Baggio was the most productive author with the most publications, followed by Noel Scott (n = 8) from Australia and Andrea Fronzetti Colladon (n = 7) from Italy. Based on the average citations per document, Carlos Casanueva from the Universidad de Sevilla, Spain, had the highest score with an average of 95.33 citations per document. Baggio, R. and Scott, N, as the most productive authors have similar research interest, which is in tourism management. Based on the study database, they had written four articles together using a network analysis approach in tourism management. Fronzetti Colladon, A. is an expert in big data, creativity and innovation management while Hossain L., utilising SNA in organisational communication network during crisis or emergency events.
3.4. Most active journals
The majority of the articles were mostly published in Elsevier's journals. Specifically, seven out of 12 source titles were Elsevier's journals; two journals were published by the American Society of Civil Engineers (ASCE), two journals by Taylor's and Francis, and one by Emerald. The Journal of Technological Forecasting and Social Change was the most active source with 84 articles, followed by Transportation Research Part E: Logistics and Transportation Review and Knowledge based Systems with 45 and 44 articles, respectively. Based on the average citations per publication, Construction Management and Economics had the highest score (c/p = 78.50), followed by Decision Support Systems (c/p = 55.33) and Transportation Research Part E (c/p = 50.51). Table 6 demonstrates a list of the most active sources in publishing research in SNA (business and management) and its impact score (cite score and SCImago Journal Rank (SJR) 2019).
Table 6
Most active source title.
Notes: TP = total number of publications; C/P = average citations per publication.
The SNA usage in business and management varies and depends on the journal scope. Particularly, SNA is used to study the interaction between people in the social environment and in various other subjects. The use of SNA in specific journals was explored by visualising the network of keywords relationship, as presented in Figure 4 . The selected three journals publishing research in SNA (business and management) had a different perspective in employing SNA as a tool to analyse the relationship between nodes.

Most frequent keywords in a) Technological Forecasting and Social Change; b) Transportation Research Part E: Logistics and Transportation Review; and c) Journal of Business Research.
Journal of Technological Forecasting and Social Change primarily utilised "innovation", "technological development", "patents and inventions", "technology adoption", "emerging technology", and others. SNA can also be used for transportation research as published in Transportation Research Part E: Logistics and Transportation Review with keywords "numerical model", "transportation planning", "air transportation", "optimisation", "freight transport", and others. Meanwhile, research published in Journal of Business Research published articles with keywords “business networks”, “social closure”, “collaboration”, “diffusion”, and others. The SNA can also be used in construction and project management, tourism management, urban planning and development, and organisational study (coordination and competition).
3.5. Highly-cited articles
Tsai (2002) published the top-cited article in SNA business and management research titled " Social structure of "coopetition" within a multiunit organisation: Coordination, Competition, and Intra organisational Knowledge Sharing" . The publication had 1,124 or 56.2 citations per year. Besides, the article explored another use of SNA, explained in the "most active journals" section in the organisational study. The study revealed that most of the top 15 articles related to inter- or intra- organisational networks, and some papers explored the use of SNA in social communication, supply chain, tourism marketing, and others. Table 7 shows the top 15 highly-cited articles.
Table 7
Top 15 Highly-cited articles.
3.6. The use of SNA in business and management research
The study conducted a keywords cluster analysis to highlight SNA usage in business and management research and identify how keywords are linked. The keywords cluster analysis was presented in two ways; the first is based on the occurrence level (see Figure 5 ), and the second is based on the year of publications (see Figure 6 ). Based on the level of keywords occurrence, SNA research was classified into six clusters: cluster 1 (red nodes) covered research in construction, project management, and information management; cluster 2 (green nodes) covered research in transportation and tourism management; cluster 3 (dark blue nodes) covered research in semantic, big data, and decision support system; cluster 4 (yellow nodes) included research in innovation, international trade, and globalisation; cluster 5 (purple node) explored research in knowledge management and knowledge sharing; cluster 6 (light blue nodes) included research in social capital, and financial performance and management.

Keywords analysis of SNA in business and management publications.

Keywords evolution of SNA in business and management research.
According to publication years, the study discovered that from 2012 to 2014, the most frequent keywords were "project management", "optimisation", "technology transfer", and "construction industry". From 2015 to 2016, the keywords shifted to "data mining", "information management", "decision-making", "tourist destination", "air transportation", "airline industry", and "innovation". Recently, "sentiment analysis", "text mining", and "big data" became popular in SNA research.
4. Discussion
The study was conducted to analyse SNA research in business and management subjects. Generally, an upward trend was found in the number of publications, significantly increasing since 2005. A significant increase was also discovered in 2020, a 40.3% increase from the previous year, the second-highest increase in the past 20 years. A similar Bibliometric analysis on SNA research without subject limitation had a similar pattern with the study, whereby SNA publications increased gradually since 2005 Based on quality metrics, articles published in 2001 and 2002 had the highest average citations per publication. Moreover, several articles published in those years also had the highest number of citations published in organisational science.
Tsai (2002) articles had the highest citation number (c = 1,124), followed by Reagans and Zuckerman (2001) , with 1009 citations. Tsai (2002) displayed intra organisational as a set of social networks and examined networks of collaborative and competitive ties within the organisation. Each unit collaborates for knowledge sharing and competes for resources and market share. Additionally, the centrality concept was used to measure the ability of intra organisational units in the knowledge sharing behaviour. In the same journal, Reagans and Zuckerman (2001) employed the SNA approach to examine the relationship between team density and heterogeneity to its performance using two network metrics: network density to assess communication frequency between team members, and network heterogeneity to explore time allocation of scientists to colleagues far removed in the team tenure distribution. According to above explanation, SNA can be used at different organisation levels, one in unit levels-organisation, and another in person-level interactions under one team.
The study revealed the US, the UK, and China as the most productive countries. Moreover, the study had similar findings as in Su et al. (2019) ; they found that the US had dominated the research in SNA, UK ranked second, and followed by China. Based on the average c/p, institutions from Asia such as South Korea, China, and Taiwan (average c/p = 23.77) received lower scores than European institutions (average c/p = 28.31). Better quality and impactful research are needed for authors from Asian institutions. The following section describes SNA usage in business and management themes.
4.1. Research themes
The SNA is a set of formal methods for studying social structures according to graph theory. Individuals and social actors, such as groups and organisations, are shown in points and their social relations in lines ( Korom, 2015 ). Meanwhile, the structure relations and the location of individual actors have substantial behavioural consequences for individuals and social structure as a whole ( Wellman, 1988 ). The SNA has become a multidisciplinary endeavour extending beyond sociology and social anthropology sciences and to many other disciplines, such as politics, epidemiology, communication science, and others. The next section explores the use of SNA in business and management discipline from publication records between 2001 to 2020 by analysing the keywords co-occurrences.
4.2. Project management
The first paper that employed SNA in project management was published in 1997 by Loosemore, believing that construction project participants are embedded in complex social networks that are constantly changing. Furthermore, Loosemore (1997) analysed communication efficiency in the engineering project organisation during crises. Besides, Pryke (2004) had the highest citations in the construction and project management area. Contrary to Loosemore, who applied network analysis at the individual level, Pryke examined the relationship between project actors at the firm level. Pryke also used network analysis in the comparative analysis of procurement and project management of construction projects. Subsequently, SNA publication in project management literature increased substantially.
Chinowsky, Diekmann, & O'Brien (2010) summarised the use of network analysis in project management research. After examining communication efficiency, network analysis analysed networks in relationship-based procurement, the effect of centrality on project coordination, the effect of cultural diversity on project performance, collaboration effectiveness to achieve high-performance teams, and others. The study discovered several popular keywords in project management research, such as "communication", "decision-making", "stakeholder", "accident prevention", "scheduling", "information exchange", "collaborative projects" and others, which explained the use of SNA in this subject. The top three journals publishing SNA usage in project and construction management were the Journal of Construction Engineering and Management (ASCE), International Journal of Project Management (Elsevier), and Construction Management and Economics (Taylor & Francis).
4.3. Risk management
The SNA publications in risk assessment and management usually relate to other subjects, such as project management, SCM, knowledge management, and others. The study found 42 related articles, mostly on construction and project management. Notably, SNA improved the effectiveness and accuracy of stakeholder and risk analysis in green building projects ( Yang et al., 2016 ). The model considered the risk associated with stakeholders and the interdependencies of risks for better decision-making. Additionally, Li et al. (2016) employed SNA for risk evaluation and risk response processes in construction projects.
The SNA effectively evaluates the potential risk contributing to schedule delays in project processes by removing key nodes and links, ultimately removing stakeholder risk that is highly interconnected to another risk. Similar to Li, Yu et al. (2017) specifically utilised SNA for social risk in urban redevelopment projects during the housing demolition stage with an identical process. The approach extends beyond construction and project management subject and any other subjects that employed risk assessment or evaluation in their risk management process.
4.4. tourism management
The SNA approach in tourism-related subjects was widespread, with "tourist destinations" and “tourism” among the most frequent and top 25 keywords (see Table 2 ). Besides, Baggio, R. was the most productive author who used SNA in tourism research. Three research streams are related to network analysis in the travel industry setting: the Bibliometric analysis on research collaboration and knowledge creation; network analysis on the travel industry supply, destination, and policy systems; and tourist movements and behavioural patterns ( Liu et al., 2017 ). Besides, the study discovered three significant journals with the most publications in tourism research: Annals of Tourism Research, Tourism Management, and Current Issues in Tourism, similar to Casanueva et al. (2016) ; whereby tourism management was the most productive journal. Recently, the Annals of Tourism Research surpassed Tourism Management.
Most tourism supply and destination research highlighted collaboration and partnership among tourism stakeholders. Baggio, Scott and Cooper (2010) applied network analysis to explain the topology of stakeholders in Elba, Italy's tourism organisations (hotels, travel agencies, associations, public bodies, and others). The tourism stakeholders were described in quantitative (network metrics) and qualitative (figure) ways. For instance, the percentage of non-connected networks described the sparseness of a network and showed a low degree of collaboration or cooperation between stakeholders. Nonetheless, Leung et al. (2012) and Asero et al. (2016) , and others studied tourist movement and behavioural patterns by analysing tourists' itineraries with traditional (interview or online travel diaries) or more-advanced technologies (geographic information system (GIS), global positioning system (GPS), timing systems, camera-based systems, and others). The primary objective is to analyse the main tourist attraction, main tourism movement patterns and change patterns in tourist attractions.
4.5. Supply chain management
The use of SNA in supply chain management research had developed in 2010, and numerous scholars were unaware of the possibilities of the SNA approach in the SCM field ( Wichmann and Kaufmann, 2016 ). The supply chain is a network of companies comprising interconnected actors, such as suppliers, manufacturers, logistic providers, and customers ( Bellamy et al., 2014 ). Three journals with the most SNA articles in SCM are the International Journal of Production Economics, International Journal of Production Research, and Journal of Operations Management.
Y. Kim et al. (2011) employed SNA to analyse the structural characteristics of supply networks in a buyers-suppliers network of automotive industries. The networks in the supply chain were classified into the material flow (supply load, demand load, and operational criticality) and the contractual relationship between actors (influential scope, informational independence, and relational mediation). The study discovered that network metrics could be used to analyse the characteristic of supply network structures.
The SNA can also measure and reduce supply chain complexity ( Allesina et al., 2010 ), a concept based on ecological theory. Additionally, eight entropic performance indexes were used: total system throughput, average mutual information, development capacity, overhead in input, export, and dissipation, etc. Contrarily, Ting & Tsang (2014) utilised SNA to identify the possibility of counterfeit products from infiltrating into the supply chain using the transaction records history to detect problematic parties and their suspicious trails. Three SNA measures were included in the study: degree centrality, betweenness centrality, and closeness centrality.
4.6. Knowledge management
The SNA usage in knowledge management varies; for instance, Parise (2007) used SNA for knowledge management of human resources, including knowledge creation and innovation, knowledge transfer and retention, and job succession planning. The SNA in knowledge creation and innovation is used to identify the flow of ideas and bottlenecks in the decision-making process. The SNA is also applied to analyse the structure of regional knowledge in the technology specialisation ( Cantner et al., 2010 ), knowledge transfer analysis on sustainable construction projects ( Schröpfer et al., 2017 ), predicting and evaluating future knowledge flows in insurance organisations ( Leon et al., 2017 ) and knowledge transfer from experts to newcomers ( Guechtouli et al., 2013 ), and others. The top productive journals are the Journal of Knowledge Management, Technological Forecasting and Social Change, and the Journal of Construction Engineering and Management.
4.7. Technology and innovation management
The SNA is used for innovation and technology transfer. Keywords under this subject include "citation "innovation", "patents and inventions", "technological development", and others. The SNA metrics utilised to assess the performance and centrality of individuals in virtual research and design (R&D) groups by analysing their e-mails ( Ahuja et al., 2003 ), identifying the position and relationships between innovators ( Cantner and Graf, 2006 ), research collaboration network between university-industry ( Balconi and Laboranti, 2006 ), and others.
The SNA in patents and inventions identify companies with a significant legal influence on the applied technologies by analysing intellectual property lawsuits between companies (H. Kim and Song, 2013 ). Patents data were also popular to study technological innovation of electronic companies; SNA was employed to cluster the patents and find vacant technology domains ( Jun and Sung Park, 2013 ). Furthermore, patent data in SNA enables exploring the technology evolution of certain products ( Lee et al., 2010 ). Specifically, the top three journals were: Technological Forecasting and Social Change, Technological Analysis and Strategic Management, and Industry and Innovation.
5. Conclusion
After SNA was introduced in 1969 by Mitchell, many researchers from various fields were interested in studying the relationship between nodes. The most frequent disciplines that used SNA are sociology, anthropology, social psychology, and communication. Nevertheless, SNA usage in business and management discipline was limited. Hence, the study analysed the trend and performance of SNA in the business and management discipline from 2001 to 2020. The study revealed a steady upward trend of publications in this field and increased significantly since 2005. The US, the UK, and China were the most productive countries. Although the study found three Asian institutions as the most productive countries, the average c/p was lower than the European and American countries. Besides, SNA as a research tool has been published in multidisciplinary journals, ranging from Journal of Management in Engineering to Journal of Knowledge Management, depending on the subject of investigation.
The study also performed a co-occurrence keywords analysis to examine the research cluster and emerging research topics in SNA, especially in business and management studies. The study revealed six clusters, each containing one to two research disciplines. The SNA has been employed in numerous topics, including project management, risk management, tourism management, supply chain, knowledge management, and technological management. Observably, big data, social media and sentiment analysis are the trending topic in SNA.
The research contributions include: first, the publication trend and research productivity show the current issue and development of SNA in the business and management discipline; second, the data on most productive authors and institutions academic communication and cooperation among scholars in related fields; and lastly, the visualisation of research topics mapping and the cluster analysis explored the current use of SNA in different discipline and formulated future research agenda.
The study limitations are: first, the study only considered the Scopus database and SNA literature could be more extensive. Other significant databases, such as WoS and CNKI (Chinese National Knowledge Infrastructure) should be considered for future research. Second, in the co-occurrence keywords analysis, a threshold was set to limit important keywords; thus, the study might not include several research topics using SNA. Third, although the study has cleaned the database, titles not purely from business and management disciplines might be included due to journal sources with multi subject classification. Lastly, we only employed standard bibliometric measures as a quantitative assessment in this research; the inclusion of SNA’ centrality index can be considered in future study to assess the power and importance of authors, institutions, countries, and journals.
Institutional review board statement
Not applicable.
Informed consent statement
Decalarations, author contribution statement.
All authors listed have significantly contributed to the development and the writing of this article.
Funding statement
This research did not receive any specific grant from funding agencies in the public, commercial, or not-for-profit sectors.
Data availability statement
Declaration of interests statement.
The authors declare no conflict of interest.
Additional information
No additional information is available for this paper.
- Acedo F.J., Barroso C., Casanueva C., Galán J.L. Co-authorship in management and organizational studies: an empirical and network analysis. J. Manag. Stud. 2006; 43 (5):957–983. [ Google Scholar ]
- Ahuja M.K., Galletta D.F., Carley K.M. Individual centrality and performance in virtual R& D groups: an empirical study. Manag. Sci. 2003; 49 (1):21–38. [ Google Scholar ]
- Allesina S., Azzi A., Battini D., Regattieri A. Performance measurement in supply chains: new network analysis and entropic indexes. Int. J. Prod. Res. 2010; 48 (8):2297–2321. [ Google Scholar ]
- Asero V., Gozzo S., Tomaselli V. Building tourism networks through tourist mobility. J. Trav. Res. 2016; 55 (6):751–763. [ Google Scholar ]
- Baggio R., Scott N., Cooper C. Network science: a review focused on tourism. Ann. Tourism Res. 2010; 37 (3):802–827. [ Google Scholar ]
- Balconi M., Laboranti A. University–industry interactions in applied research: the case of microelectronics. Res. Pol. 2006; 35 (10):1616–1630. [ Google Scholar ]
- Bellamy M.A., Ghosh S., Hora M. The influence of supply network structure on firm innovation. J. Oper. Manag. 2014; 32 (6):357–373. [ Google Scholar ]
- Borgatti S.P., Li X. On social network analysis in a supply chain context. J. Supply Chain Manag. 2009; 45 (2):5–22. [ Google Scholar ]
- Boschma R.A., ter Wal A.L.J. Knowledge networks and innovative performance in an industrial district: the case of a footwear district in the South of Italy. Ind. Innovat. 2007; 14 (2):177–199. [ Google Scholar ]
- Brown J., Broderick A.J., Lee N. Word of mouth communication within online communities: conceptualizing the online social network. J. Interact. Market. 2007; 21 (3):2–20. [ Google Scholar ]
- Cantner U., Graf H. The network of innovators in Jena: an application of social network analysis. Res. Pol. 2006; 35 (4):463–480. [ Google Scholar ]
- Cantner U., Meder A., ter Wal A.L.J. Innovator networks and regional knowledge base. Technovation. 2010; 30 (9–10):496–507. [ Google Scholar ]
- Carmona-Serrano N., López-Belmonte J., Cuesta-Gómez J.-L., Moreno-Guerrero A.-J. Documentary analysis of the scientific literature on autism and technology in web of science. Brain Sci. 2020; 10 (12):985. [ PMC free article ] [ PubMed ] [ Google Scholar ]
- Casanueva C., Gallego Á., García-Sánchez M.-R. Social network analysis in tourism. Curr. Issues Tourism. 2016; 19 (12):1190–1209. [ Google Scholar ]
- Chinowsky P.S., Diekmann J., O’Brien J. Project organizations as social networks. J. Construct. Eng. Manag. 2010; 136 (4):452–458. [ Google Scholar ]
- Dhamija P., Bag S. Role of artificial intelligence in operations environment: a review and bibliometric analysis. The TQM Journal. 2020; 32 (4):869–896. [ Google Scholar ]
- Ellegaard O., Wallin J.A. The bibliometric analysis of scholarly production: how great is the impact? Scientometrics. 2015; 105 (3):1809–1831. [ PMC free article ] [ PubMed ] [ Google Scholar ]
- Erçetin Ş.Ş., Neyişci N.B. Chaos, Complexity and Leadership. 2014. Social network analysis : a brief introduction; pp. 167–171. [ Google Scholar ]
- Fahimnia B., Sarkis J., Davarzani H. Green supply chain management: a review and bibliometric analysis. Int. J. Prod. Econ. 2015; 162 :101–114. [ Google Scholar ]
- Fetscherin M., Usunier J.C. Corporate branding: an interdisciplinary literature review. Eur. J. Market. 2012; 46 (5):733–753. [ Google Scholar ]
- Guechtouli W., Rouchier J., Orillard M. Structuring knowledge transfer from experts to newcomers. J. Knowl. Manag. 2013; 17 (1):47–68. [ Google Scholar ]
- Hafner-Burton E.M., Kahler M., Montgomery A.H. Network analysis for international relations. Int. Organ. 2009; 63 (3):559–592. [ Google Scholar ]
- Hirsch J.E. An index to quantify an individual’s scientific research output. Proc. Natl. Acad. Sci. Unit. States Am. 2005; 102 (46):16569–16572. [ PMC free article ] [ PubMed ] [ Google Scholar ]
- Hirsch J.E. Does the h index have predictive power? Proc. Natl. Acad. Sci. Unit. States Am. 2007; 104 (49):19193–19198. [ PMC free article ] [ PubMed ] [ Google Scholar ]
- Jing S., Qinghua Z., Landström H. Handbook of Research on Global Competitive Advantage through Innovation and Entrepreneurship. 2015. Entrepreneurship across regions: internationalization and/or contextualization? pp. 372–392. [ Google Scholar ]
- Jun S., Sung Park S. Examining technological innovation of Apple using patent analysis. Ind. Manag. Data Syst. 2013; 113 (6):890–907. [ Google Scholar ]
- Kim H., Song J. Social network analysis of patent infringement lawsuits. Technol. Forecast. Soc. Change. 2013; 80 (5):944–955. [ Google Scholar ]
- Kim Y., Choi T.Y., Yan T., Dooley K. Structural investigation of supply networks: a social network analysis approach. J. Oper. Manag. 2011; 29 (3):194–211. [ Google Scholar ]
- Korom P. International Encyclopedia of the Social & Behavioral Sciences. Vol. 16. 2015. Network analysis, history of; pp. 524–531. Second Edi. [ Google Scholar ]
- Kurt Y., Kurt M. Social network analysis in international business research: an assessment of the current state of play and future research directions. Int. Bus. Rev. 2020; 29 (2):101633. [ Google Scholar ]
- Lee P.-C., Su H.-N., Wu F.-S. Quantitative mapping of patented technology — the case of electrical conducting polymer nanocomposite. Technol. Forecast. Soc. Change. 2010; 77 (3):466–478. [ PMC free article ] [ PubMed ] [ Google Scholar ]
- Leon R.–D., Rodríguez-Rodríguez R., Gómez-Gasquet P., Mula J. Social network analysis: a tool for evaluating and predicting future knowledge flows from an insurance organization. Technol. Forecast. Soc. Change. 2017; 114 :103–118. [ Google Scholar ]
- Leung X.Y., Wang F., Wu B., Bai B., Stahura K.A., Xie Z. A social network analysis of overseas tourist movement patterns in beijing: the impact of the olympic games. Int. J. Tourism Res. 2012; 14 (5):469–484. [ Google Scholar ]
- Li C.Z., Hong J., Xue F., Shen G.Q., Xu X., Mok M.K. Schedule risks in prefabrication housing production in Hong Kong: a social network analysis. J. Clean. Prod. 2016; 134 (Part B):482–494. [ Google Scholar ]
- Li N., Wu D.D. Using text mining and sentiment analysis for online forums hotspot detection and forecast. Decis. Support Syst. 2010; 48 (2):354–368. [ Google Scholar ]
- Litterio A.M., Nantes E.A., Larrosa J.M., Gómez L.J. Marketing and social networks: a criterion for detecting opinion leaders. Eur. J. Manag. Bus. Econ. 2017; 26 (3):347–366. [ Google Scholar ]
- Liu B., Huang S., Fu H. An application of network analysis on tourist attractions: the case of Xinjiang, China. Tourism Manag. 2017; 58 :132–141. [ Google Scholar ]
- Loosemore M. Construction crises as periods of social adjustment. J. Manag. Eng. 1997; 13 (4):30–37. [ Google Scholar ]
- Monaghan S., Lavelle J., Gunnigle P. Mapping networks: exploring the utility of social network analysis in management research and practice. J. Bus. Res. 2017; 76 :136–144. [ Google Scholar ]
- Nagurney A., Dong J., Zhang D. A supply chain network equilibrium model. Transport. Res. E Logist. Transport. Rev. 2002; 38 (5):281–303. [ Google Scholar ]
- Netzer O., Feldman R., Goldenberg J., Fresko M. Mine your own business: market-structure surveillance through text mining. Market. Sci. 2012; 31 (3):521–543. [ Google Scholar ]
- Pan B., MacLaurin T., Crotts J.C. Travel blogs and the implications for destination marketing. J. Trav. Res. 2007; 46 (1):35–45. [ Google Scholar ]
- Parell C. SAGE Publication; Chennai: 2012. Social Network Analysis: History, Theory, and Methodology. [ Google Scholar ]
- Parise S. Knowledge management and human resource development: an application in social network analysis methods. Adv. Develop. Hum. Resour. 2007; 9 (3):359–383. [ Google Scholar ]
- Pesta B., Fuerst J., Kirkegaard E. Bibliometric keyword analysis across seventeen years (2000–2016) of intelligence articles. J. Intell. 2018; 6 (4):46. [ PMC free article ] [ PubMed ] [ Google Scholar ]
- Pranckutė R. Web of science (WoS) and Scopus: the titans of bibliographic information in today’s academic world. Publications. 2021; 9 (1):12. [ Google Scholar ]
- Provan K.G., Milward H.B. Do networks really work? A framework for evaluating public-sector organizational networks. Publ. Adm. Rev. 2001; 61 (4):414–423. [ Google Scholar ]
- Pryke S.D. Analysing construction project coalitions: exploring the application of social network analysis. Construct. Manag. Econ. 2004; 22 (8):787–797. [ Google Scholar ]
- Reagans R., Zuckerman E.W. Networks, diversity, and productivity: the social capital of corporate R&D teams. Organ. Sci. 2001; 12 (4):502–517. [ Google Scholar ]
- Schröpfer V.L.M., Tah J., Kurul E. Mapping the knowledge flow in sustainable construction project teams using social network analysis. Eng. Construct. Architect. Manag. 2017; 24 (2):229–259. [ Google Scholar ]
- Sosa M.E., Eppinger S.D., Rowles C.M. The misalignment of product architecture and organizational structure in complex product development. Manag. Sci. 2004; 50 (12):1674–1689. [ Google Scholar ]
- Sozen C., Basim N., Hazir K. Social network analysis in organizational studies. Int. J. Bus. Manag. 2009; 1 (1):21–35. [ Google Scholar ]
- Stephen A.T., Toubia O. Deriving value from social commerce networks. J. Market. Res. 2010; 47 (2):215–228. [ Google Scholar ]
- Su Y.-S., Lin C.-L., Chen S.-Y., Lai C.-F. Bibliometric study of social network analysis literature. Libr. Hi Technol. 2019; 38 (2):420–433. [ Google Scholar ]
- Ting S.L., Tsang A.H.C. Using social network analysis to combat counterfeiting. Int. J. Prod. Res. 2014; 52 (15):4456–4468. [ Google Scholar ]
- Tsai W. Social structure of “coopetition” within a multiunit organization: coordination, competition, and intraorganizational knowledge sharing. Organ. Sci. 2002; 13 (2):179–190. [ Google Scholar ]
- van Eck N.J., Waltman L. Software survey: VOSviewer, a computer program for bibliometric mapping. Scientometrics. 2010; 84 (2):523–538. [ PMC free article ] [ PubMed ] [ Google Scholar ]
- Vieira E.S., Madaleno M., Azevedo G. In: Comparative Research on Earnings Management, Corporate Governance, and Economic Value. Vieira E.S., Madaleno M., Azevedo G., editors. 2021. Research on earnings management: bibliometric analysis; pp. 1–26. [ Google Scholar ]
- Vitali S., Glattfelder J.B., Battiston S. The network of global corporate control. PLoS One. 2011; 6 (10) [ PMC free article ] [ PubMed ] [ Google Scholar ]
- Wellman B. Social Structures: A Network Approach. Cambridge University Press; 1988. Structural analysis: from method and metaphor to theory and substance. [ Google Scholar ]
- Wichmann B.K., Kaufmann L. Social network analysis in supply chain management research. Int. J. Phys. Distrib. Logist. Manag. 2016; 46 (8):740–762. [ Google Scholar ]
- Yang R.J., Zou P.X.W., Wang J. Modelling stakeholder-associated risk networks in green building projects. Int. J. Proj. Manag. 2016; 34 (1):66–81. [ Google Scholar ]
- Yu T., Shen G.Q., Shi Q., Lai X., Li C.Z., Xu K. Managing social risks at the housing demolition stage of urban redevelopment projects: a stakeholder-oriented study using social network analysis. Int. J. Proj. Manag. 2017; 35 (6):925–941. [ Google Scholar ]
- Zakaria R., Ahmi A., Ahmad A.H., Othman Z. Worldwide melatonin research: a bibliometric analysis of the published literature between 2015 and 2019. Chronobiol. Int. 2021; 38 (1):27–37. [ PubMed ] [ Google Scholar ]
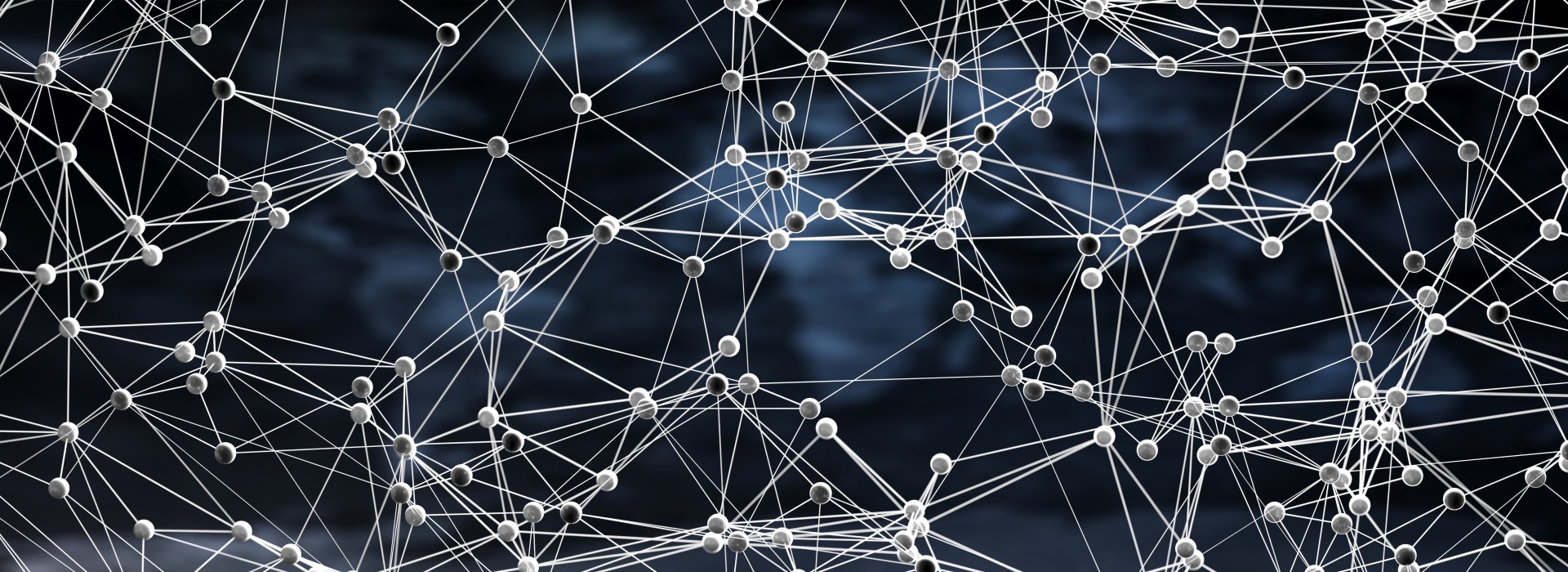
Using Social Network Analysis to Assess Collaborative Networks: A Case Study from the Genebank Platform Evaluation
What is social network analysis (sna).
Social Network Analysis (SNA) is an established method in sociology since the early 20th century that has gained prominence in recent decades due to technological advances. It is versatile and can be applied in a wide range of fields—including economics, biology, medicine, communications, and more—by identifying key actors within a social framework and decoding their interconnections. The approach offers a systematic methodology that employs graph theory to visually represent the structure of connections among entities.
In impact evaluations, SNA can usefully quantify the collaborative efforts of various stakeholders to achieve shared objectives. It can enhance evaluation processes by capturing and visualizing relationship nuances in interventions or programs, revealing insights into collaboration dynamics, identifying strong connections, and pinpointing gaps where interactions can be improved or established—thus boosting a group's overall effectiveness.
In SNA, networked structures are mapped out, with individual actors—such as people, groups, and organizations—referred to as ‘nodes’, and the relationships or interactions between them considered ‘edges’. These connections can be of any kind, such as family ties, friendships, professional, geographical, institutional, health-related, etc. Several tools can then be employed to study and interpret the mapped structures.
Several CGIAR studies have used SNA as a powerful way to measure the involvement of multiple stakeholders, including an evaluation of the influence and reach of the CGIAR Research Program on Climate Change, Agriculture and Food Security (CCAFS), and a systematic portfolio review of the International Maize and Wheat Improvement Center (CIMMYT)’s climate change research portfolio.
This blog shares key learnings from the CGIAR’s recent Genebank Platform evaluation . It demonstrates the as-yet-untapped potential of integrating SNA to generate and visualize evidence based on Quality of Science (QoS) evaluation criteria in process and performance evaluations.
SNA in the CGIAR Genebank Platform evaluation
The 2023 CGIAR Genebank Platform evaluation used SNA to gather evaluative evidence about the Platform (see SNA Report ) to support the institutional learning of CGIAR and Crop Trust. SNA helped address key evaluation criteria such as relevance, effectiveness, coherence, and QoS. It also contributed to the assessment of three Genebank Platform modules, on policy, conservation, and use.
During the evaluation, SNA was employed to identify key players and examine their relationships, both within their groups and with other Genebank Platform stakeholders. From the total 186 responses received from an online survey, a refined subset of 122 was selected after data cleaning and processing. These responses were then transformed into matrices, highlighting connections and other network characteristics. The evaluators then used the open-source software program Gephi to depict the network’s spatial layout using force-directed algorithms that brought linked nodes together and separated unrelated ones, thus facilitating the data’s interpretability and clarity.
The SNA generated four visualizations: a network of professional collaborations, a communication and interaction network encompassing various stakeholders, a leadership network, and a network representing management decision-making and funding needs. Analysis of clusters formed in these networks showed that partners and users interacting through the Genebank Platform were moderately interconnected.
Key takeaways from using SNA in the Genebank Platform evaluation include:
- CGIAR should continue its efforts to strengthen its relationship with National Agricultural Research (and Extension) Systems (NARS) across countries.
- Although the professional network of the Genebank Platform includes CGIAR centers and genebanks with important roles in the conservation and policy modules, partners outside the CGIAR system can play a crucial role in meeting the needs of farmers and other user groups.
- CGIAR centers have worked hand-in-hand with non-CGIAR partners. The flow of communication between different stakeholders reiterated the significant value of the professional ties these partners developed and positioned the Genebank Platform as a central space where relevant information was exchanged.
- Internal CGIAR stakeholders play a crucial role in disseminating information about access to and availability of plant genetic materials and accessions. However, the network of non-CGIAR partners offers an opportunity to extend CGIAR's reach to end users, including farmers and community-based organizations.
- Non-CGIAR partners, especially NARS and academic and research institutions, serve as pivotal connectors for region-specific user subgroups, providing access to vital information on plant and crop diversity and conservation. This enables CGIAR to meet its objective of enhancing the reach and timely accessibility of germplasm by the ultimate user groups (i.e. farmers).
- Lastly, leveraging the broadcast potential of influential partners ensures the cost-effectiveness of future interventions. Strategies aimed at empowering local and influential partners can motivate them to strengthen their networks and implement projects independently at the ground level, thereby enhancing the overall effectiveness of CGIAR's developmental efforts.
Figure: Communication Patterns and Interactions Between Nodes or Partners on the Genebank Platform (Source: SNA Report )
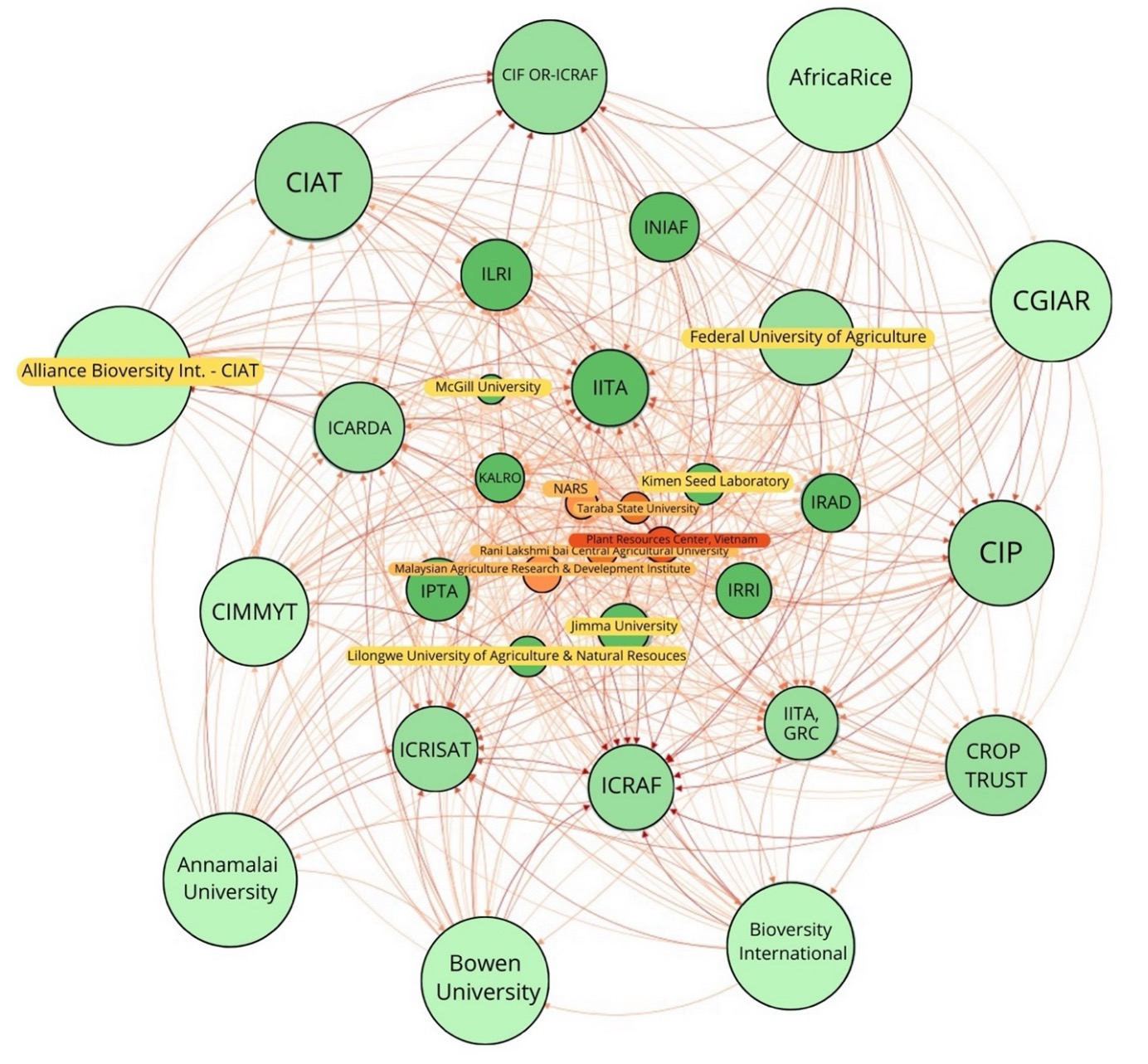
Source: Sociographs were created using Gephi (an online software for SNA). The responses received for section on ‘partnerships and interactions’ from the online survey were utilized to create these maps.
Role and utility of SNA for CGIAR’s organizational learning
In the context of CGIAR's mission to advance global agriculture and food security, SNA emerges as a powerful tool that complements and supplements the data obtained from other quantitative and qualitative tools, thereby contributing to the subsequent set of learnings obtained during any evaluative study. This analytical method delves into the intricacies of network relationships and interactions, answering pivotal questions that can significantly influence CGIAR's strategies and outcomes.
SNA’s ability to identify key interconnected individuals and organizations is particularly relevant for CGIAR, which operates in a complex landscape of stakeholders, including researchers, farmers, governments, and NGOs. Understanding the role of these actors in collaborative networks—as well as the strength of their relationships—can guide CGIAR in forging effective collaborations and partnerships, which are critical for driving agricultural innovation and policy development. By analyzing how strong these connections are and whether they are unidirectional or bidirectional, CGIAR can identify not just the main participants in a network but also those deviating from the norm.
SNA also provides insights into the dynamics of information diffusion. By enabling the identification of potential gaps in interconnections among actors, the method can point to where CGIAR might strategically direct resources to strengthen those relationships. Such insights are invaluable in designing more inclusive and effective strategies that take into account the diverse range of stakeholders in the agricultural sector.
As SNA centers on actors and their relationships, rather than traditional indicators such as scientific outputs or outcome statements, results from this approach uncover insights that cannot be derived from evaluations that focus only on implementation, monitoring, and reporting mechanisms. SNA provides a methodological framework to understand and optimize the dynamics of the complex networks that CGIAR programs and initiatives operate within—thereby supporting its mission to transform global food and agriculture systems.
- Report: Social Network Analysis: Evaluation of the CGIAR Genebank Platform
- Blog: 'Alone We Can do so Little; Together We Can do so Much'

Related Publications
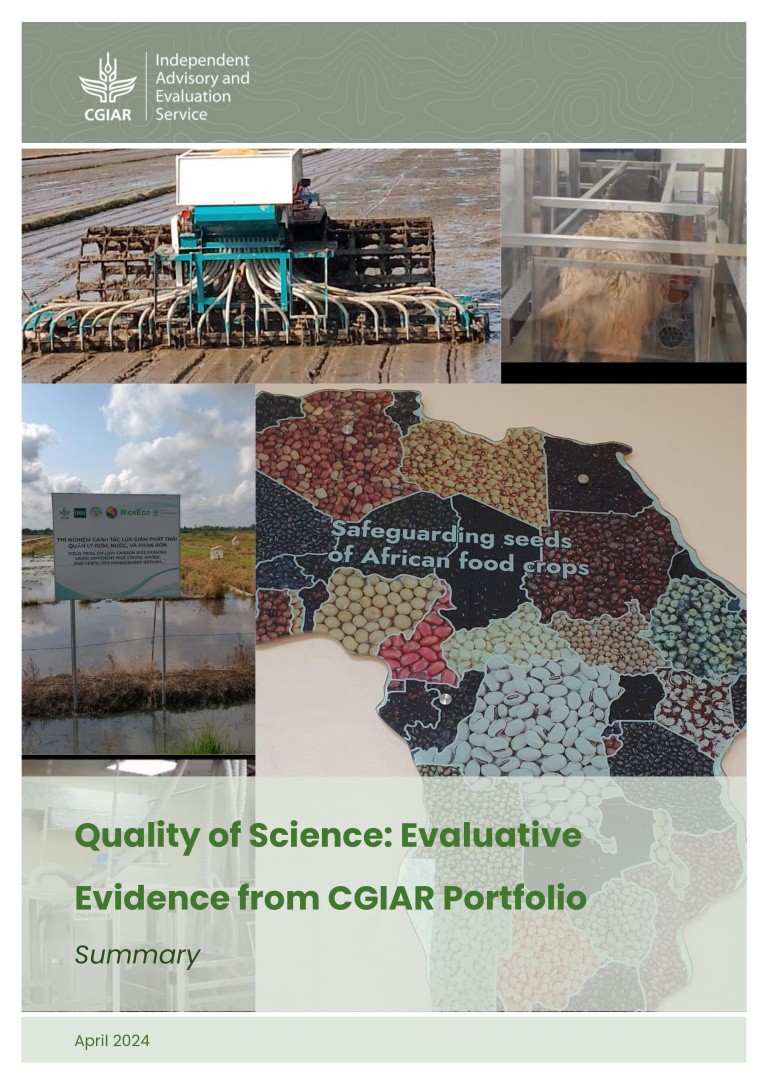
Quality of Science: Evaluative Evidence from CGIAR Portfolio Summary
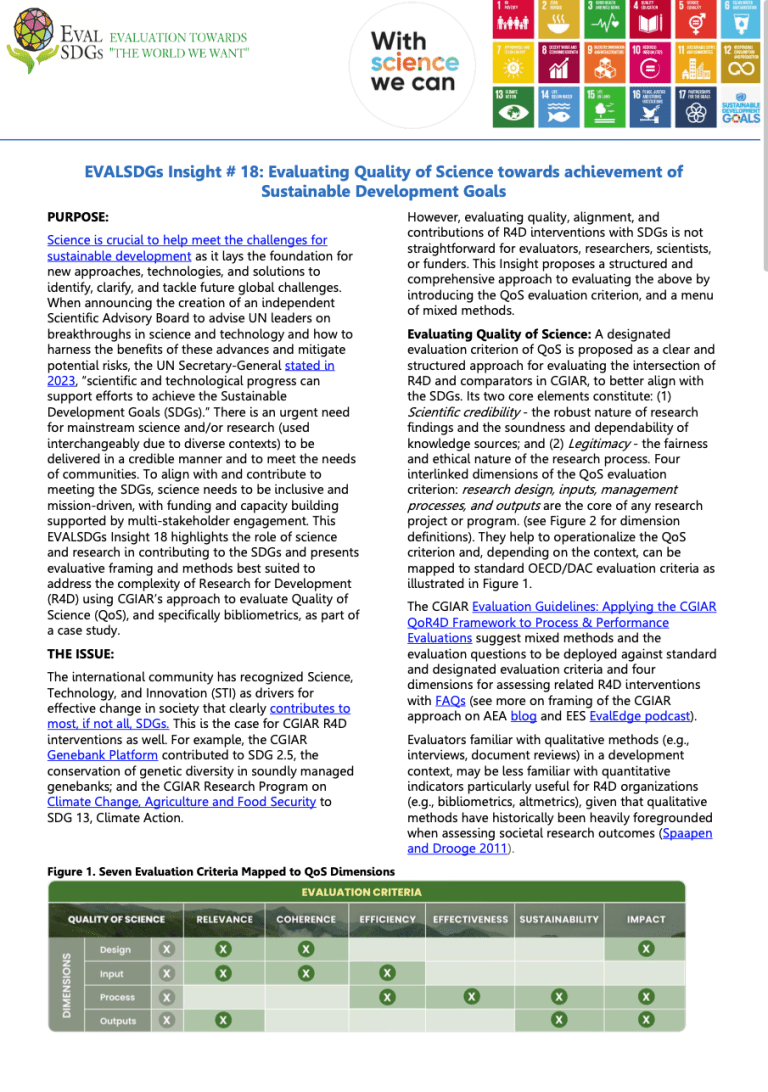
EVALSDGs Insight #18: Evaluating Quality of Science towards achievement of the SDGs
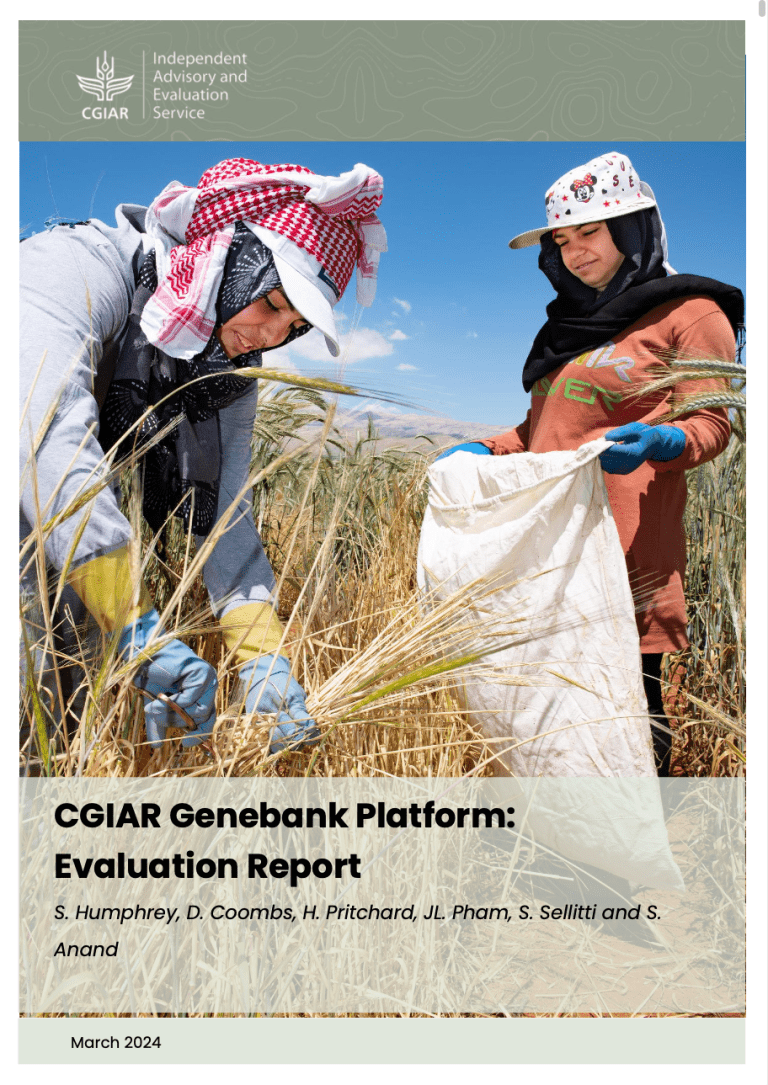
CGIAR Genebank Platform: Evaluation Report
Join our mailing list.
Social Network Analysis: How to Get Started

Networks are all around us — road networks, internet networks and online social networks like Facebook. While this article focuses on social network analysis (SNA), these techniques will give you valuable tools to gain insight on a variety of data sources.
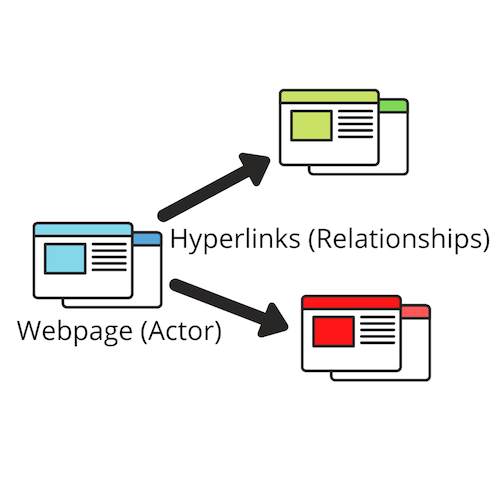
In order to build SNA graphs, we need two key components: actors and relationships. We commonly use SNA techniques with the internet. Web pages often link to other sites — either on their own website or an external page. These links can be considered relationships between actors (web pages) and this is a key component of search engine architecture.
What Is Social Network Analysis?
What does a social network graph look like.
A social network graph contains both points and lines connecting those points — similar to a connect-the-dot puzzle. The points represent the actors and the lines represent the relationships. An example of a social network graph would be this one, which demonstrates community detection of ISIS Twitter accounts .
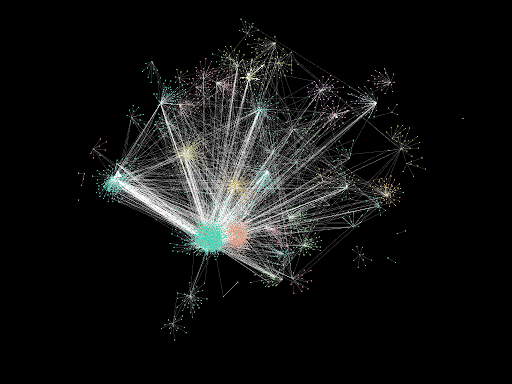
More From Mitch Ditch Your Passwords — They’re Only Hurting You
What Tools Do I Need to Get Started?
Like many things in data science, there are a variety of tools you can use to conduct SNA. This guide focuses on a specific set of tools in order to get you started making network graphs and conducting analysis on them. In no way are these the only or best tools available.
We’ll use Gephi , a free software for Mac, PC, and Linux, in order to build network graphs and run some analytics on them. Gephi provides a GUI interface (seen below) and will not require any coding.
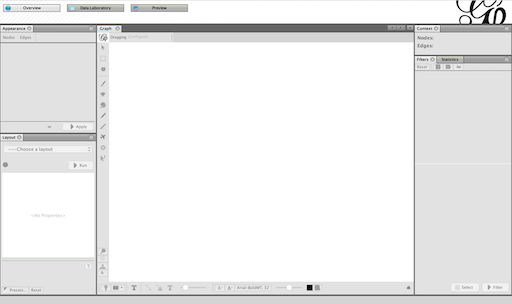
Python/Excel
In order to build network graphs in Gephi, we’ll need to use a specific data format and we’ll need to fit our data in the correct format (CSV files). With simple data, Excel should suffice. However, when using large amounts of data or data that must have its relationships extracted, I recommend Python. Don’t fret if you don’t have any Python skills — you should still be able to build basic networks.
Data Source
You’ll also need a data source for your network. Network data have two requirements: actors and relationships. Some data will require these relationships to be extracted, and others will be more explicit. I recommend using data sets from Kaggle to get started, such as:
Marvel Universe Social Network
Wikipedia Article Network
Deezer Social Network
Defining Our Terms
Nodes and edges.
Up until now, I’ve referred to both actors and relationships. In network science, actors are referred to as nodes (the dots on the graph) and relationships as edges (the lines on the graph).
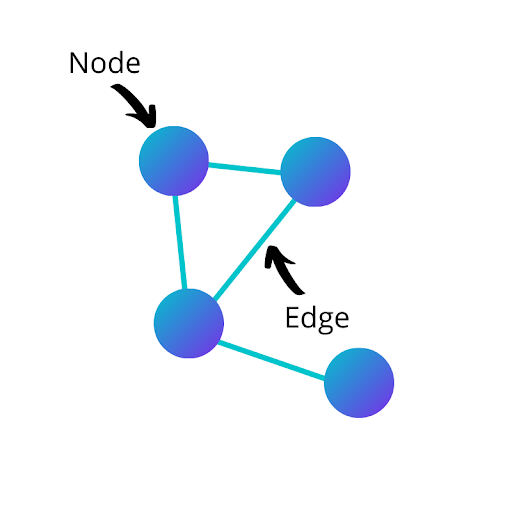
Nodes can represent a variety of actors. For example, in internet networks nodes can represent web pages while in social networks nodes can represent people. While nodes can represent a variety of things, each node always has a relationship with another thing.
Edges can represent a variety of relationships. In internet networks, edges can represent hyperlinks and in social networks edges can represent connections. Nodes and edges are a key concept in networks, so make sure you have a good understanding of them before tackling the other concepts.
Edge Direction
There are two types of edges: directed and undirected. It will be necessary to decipher what type of edge your data contains when building a network graph.
Directed edges are applied from one node to another with a starting node and an ending node. For example, when a Twitter user tags another Twitter user in a tweet, that relationship is directed. The user who wrote the tweet (starting node) applied that relationship to the user who they tagged (ending node). The tagged user has not necessarily reciprocated that relationship. Another example of a directed edge are payments. If a customer (starting node) pays a coffee shop (ending node) for a coffee, that relationship is not necessarily reciprocated because the coffee shop has not also paid the customer.
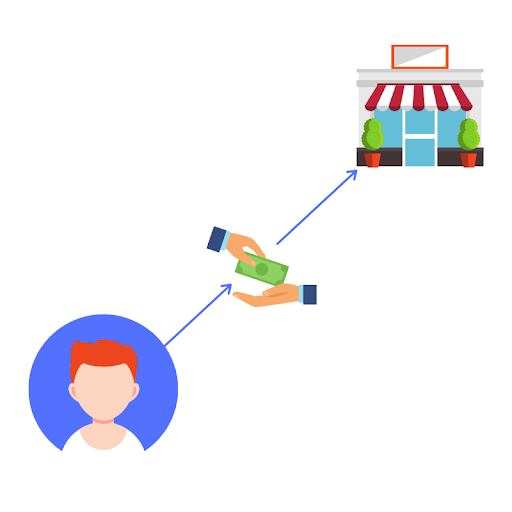
Undirected edges are the opposite of directed edges. These relationships are reciprocated by both parties without a clear starting node or ending node. For example, if two people are friends on Facebook, that relationship is undirected. This is because person A is friends with person B, but we can also say person B is friends with person A.
Edge Weight
An edge’s weight is the number of times that edge appears between two specific nodes. For example, if person A buys a coffee from a coffee shop three times, the edge connecting person A and the coffee shop will have a weight of three. However, if person B only buys coffee from the coffee shop once, the edge connecting person B and the coffee shop will have a weight of one.
Centrality Measures
Centrality is a collection of metrics used to quantify how important and influential a specific node is to the network as a whole. It’s important to remember that centrality measures are used on specific nodes within the network, and don’t provide information on a network level. There are several centrality measures, but this guide will cover degree, closeness and betweenness.
A node’s degree is the number of edges the node has. In an undirected network, there’s only one measure for degree. For example, if node A has edges connecting it to node B and node D, then node A’s degree is two.
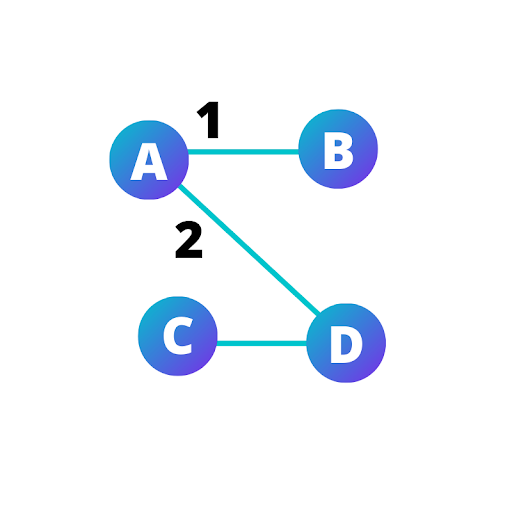
However, in a directed network, there are actually three different degree measures. Because these edges have a starting and end node, the in-degree (number of edges the node is an end node of), out-degree (number of edges a node is a starting node of), and degree (number of edges a node is either a starting node or end node of) can be calculated.
Closeness measures how well connected a node is to every other node in the network. A node’s closeness is the average number of hops required to reach every other node in the network. A hop is the path of an edge from one node to another. For example, node A is connected to node B, and node B is connected to node C. For node A to reach node C it would take two hops.
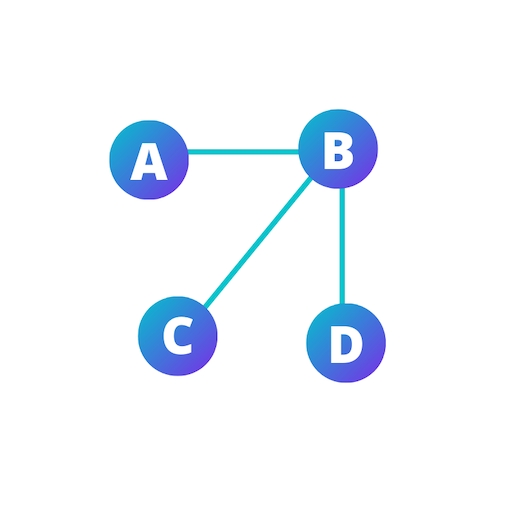
Betweenness
Betweenness measures the importance of a node’s connections in allowing nodes to reach other nodes (in a hop). A node’s betweenness is the number of shortest paths the node is included in divided by the total number of shortest paths. This will provide the percentage of shortest paths in the node’s network.
Network-Level Measures
We can also calculate metrics on the network level to evaluate the entire network instead of merely a single node. Like centrality measures, there are a variety of network-level measures. We’ll look at size and density.
Network Size
Network size is the number of nodes in the network. The size of a network does not take into consideration the number of edges. For example, a network with nodes A, B, and C has a size of three.
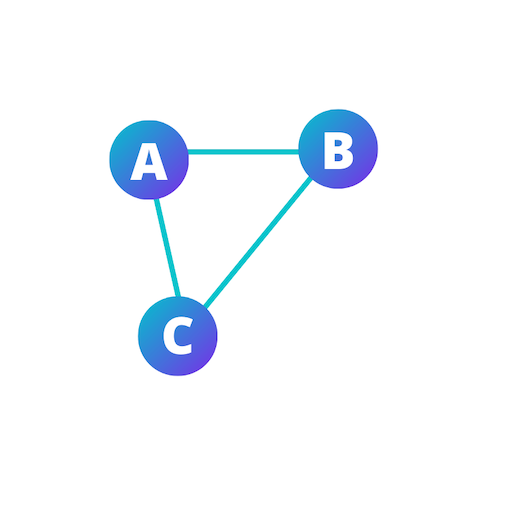
Network Density
Network density is the number of edges divided by the total possible edges. For example, a network with node A connected to node B, and node B connected to node C, the network density is 2/3 because there are two edges out of a possible three.
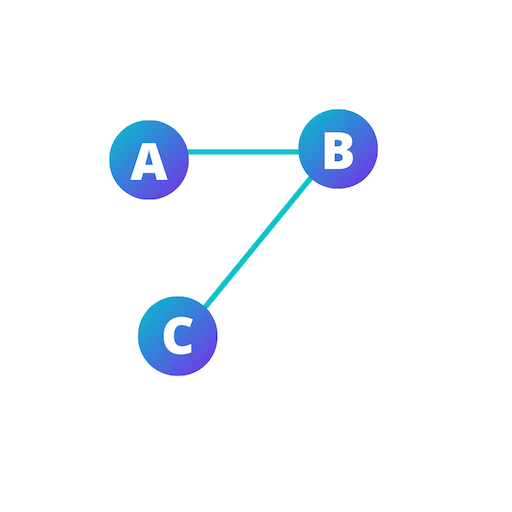
Path-Level Measures
Path-level measures provide information for a path between one node and another node. Paths follow edges between nodes, known as hops. There are also many different path-level measures, but we’ll look at length and distance.
Length is the number of edges between the starting and ending nodes, known as hops. We must predetermine a path in order to calculate the length between two nodes.
Distance is the number of edges or hops between the starting and ending nodes following the shortest path. Unlike length, the distance between two nodes uses only the shortest path — the path that requires the least hops.
Read More From Our Experts What Is Multiple Regression?
Connected Components and Bridges
Not all nodes in a network will necessarily be connected to each other. A connected component is a group of nodes that are connected to each other, but not connected to another group of nodes. Another way of thinking of this is a group of connected nodes that have no path to a node from another group. Depending on the network, there can be many connected components, or even only one. The diagram below shows a network with two connected components.
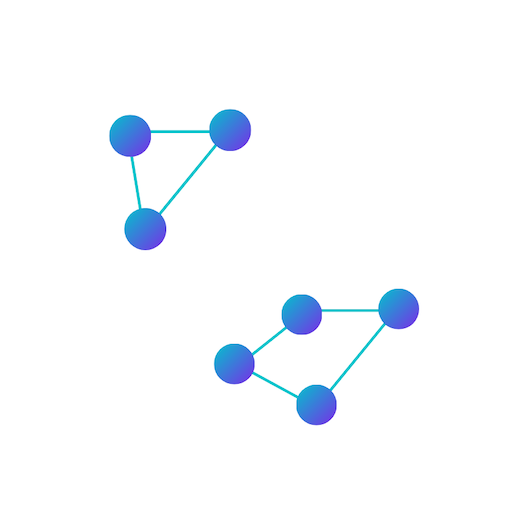
A bridge is a node that when removed, creates a connected component. Another way of thinking about it is that a bridge is a node that is the sole connection of a group of connected nodes to another group of connected nodes.
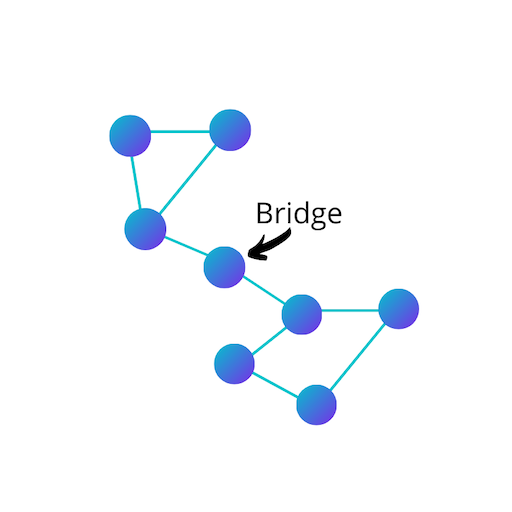
Hubs and Authorities
Hubs and Authorities are node classifications used in directed networks. A hub is a node that has many edges pointing out of it. You can also think of a hub as a node that’s the starting node of many edges. An authority, on the other hand, is a node that has many edges pointing to it. You can also think of authority as a node that is the ending node of many edges. There’s not a pre-determined number of edges that makes a node a hub or an authority; it will depend on the network. In addition, remember that not all nodes in a directed network will be a hub or an authority.
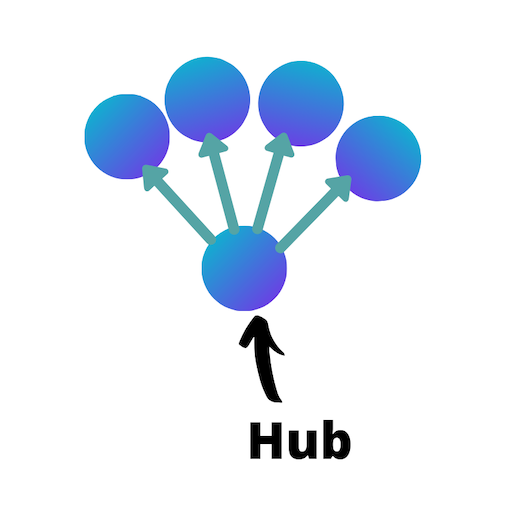
Dyads and Cliques
Dyads and cliques are pairings of nodes connected by edges. A dyad is a pairing of two nodes, while a clique is a pairing of three or more nodes. While a dyad or clique may be a connected component, they can also be part of a larger connected component.
Implementation
Now that you have an understanding of social network analysis terms and concepts, this guide will walk you through applying these techniques to a data set using Gephi
Download and Install Gephi
First, download and install the Gephi software for the operating system your machine is running. Gephi is available for Mac, PC, and Linux .
More Built In Tutorials Create a Linux Virtual Machine on Your Computer
For this guide, we’ll be using the Marvel Universe Social Network data set from Kaggle . While this data set is already laid out with a node and edge list, when working with data sets not structured as a network this will require some data transformation skills. I recommend using Python and Pandas in these situations.
After downloading the data set, there will be three csv files: nodes, edges, and network. Open the file nodes.csv in Excel.
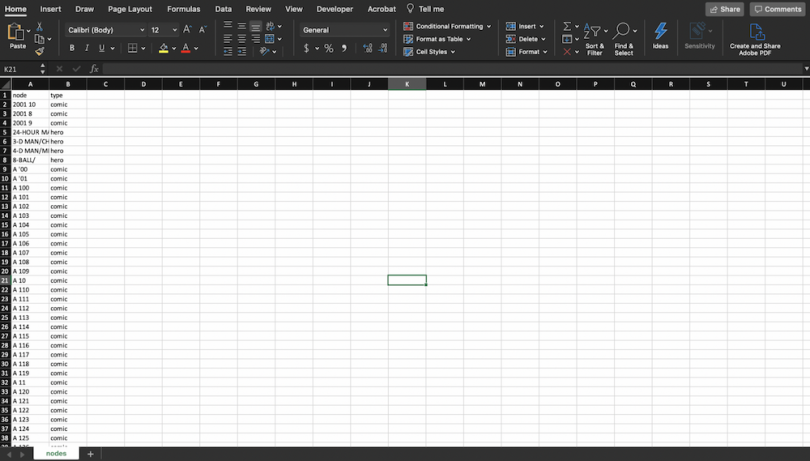
The nodes file contains a list of all the nodes in the network. This file has two columns: node and type. This network contains two different types of nodes that represent different actor types: heroes and comics.
There is no data preparation needed to import this node list into Gephi, so we’ll close the file.
Next, open the file edges.csv in Excel.
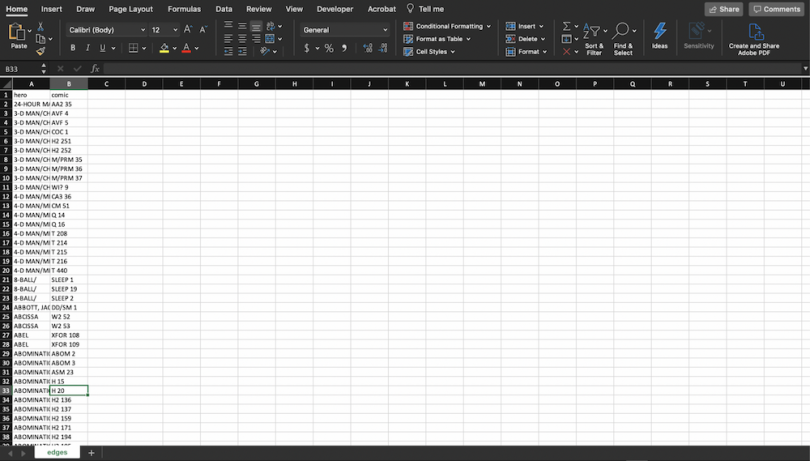
The edges file also contains two columns: hero and comic. Each row in this table represents a single edge. The hero node and comic node are the two nodes connected by the edge.
In Gephi, an edges table requires the column headers of “source” and “target.” In an undirected network it doesn’t matter which node is in which column. However, in a directed network the source column contains the starting node and the target column contains the ending node. Rename column A to “source” and column B to “target.” Then save the file.
Loading Network Data into Gephi
Now that the node and edge lists are properly formatted for Gephi, it is time to load the data.
Open the Gephi software. It should look like this:
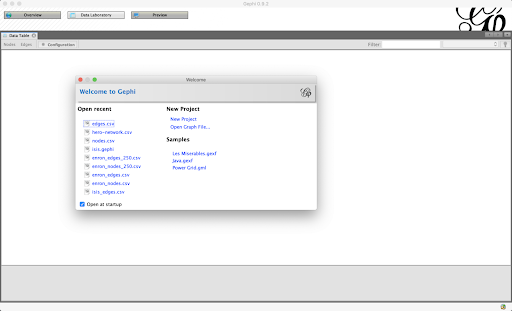
Click on new project. If you don’t see the welcome screen, go to file>new project.
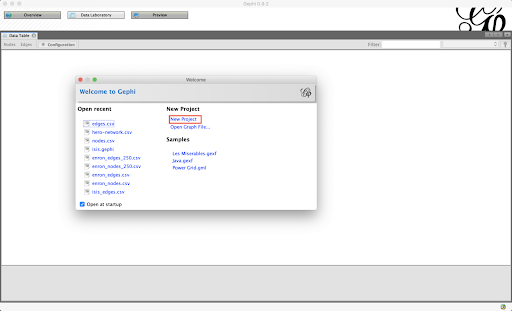
Then, click the data laboratory tab.
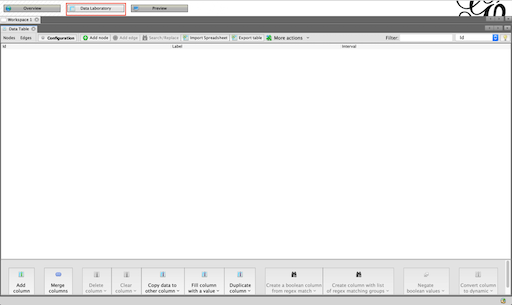
The data laboratory tab is where we’ll load in our edge and node list files. To import a list click the import spreadsheet button.
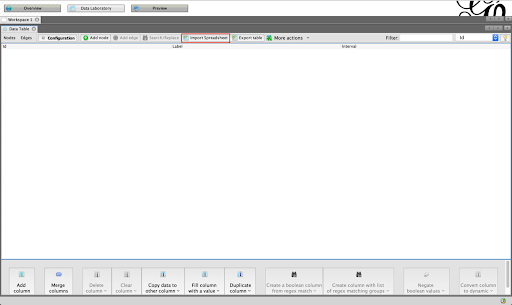
Then navigate to the folder containing the data sets and open the nodes file.
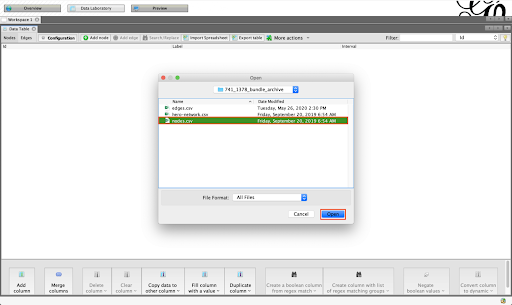
An import wizard will then walk you through correctly importing the node list. Set separator to comma, import as to nodes table, and charset as UTF-8. Then click next.
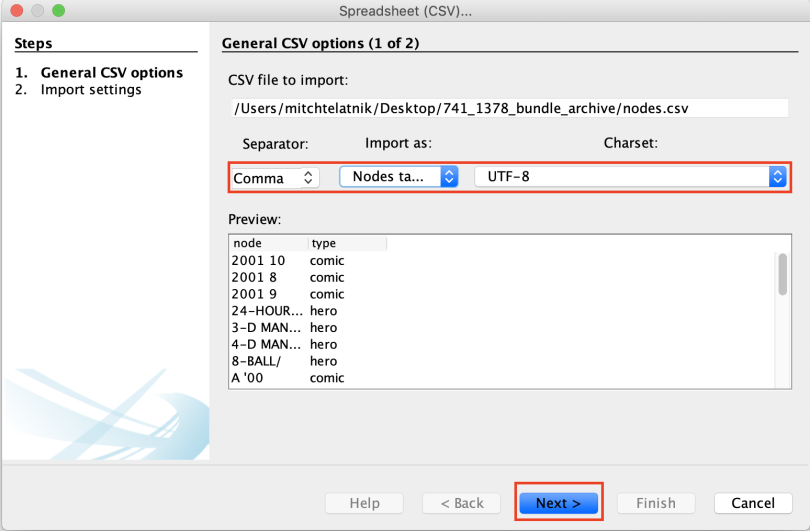
After clicking next, the wizard will provide additional setting configurations. Set time representation to intervals. For imported columns, check the node and type boxes and set their data types to string. Then, click finish.
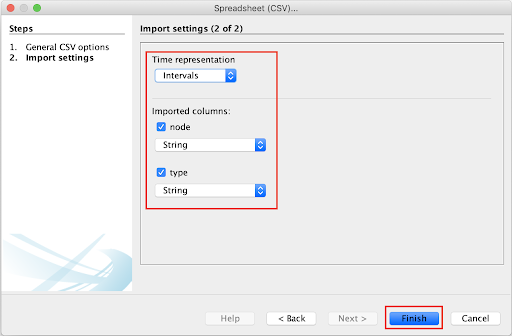
There is one more step in importing the nodes list. Set graph type to undirected and edges merge strategy to sum. Ensure that it’s set up to append to the existing workspace. Then, click OK.
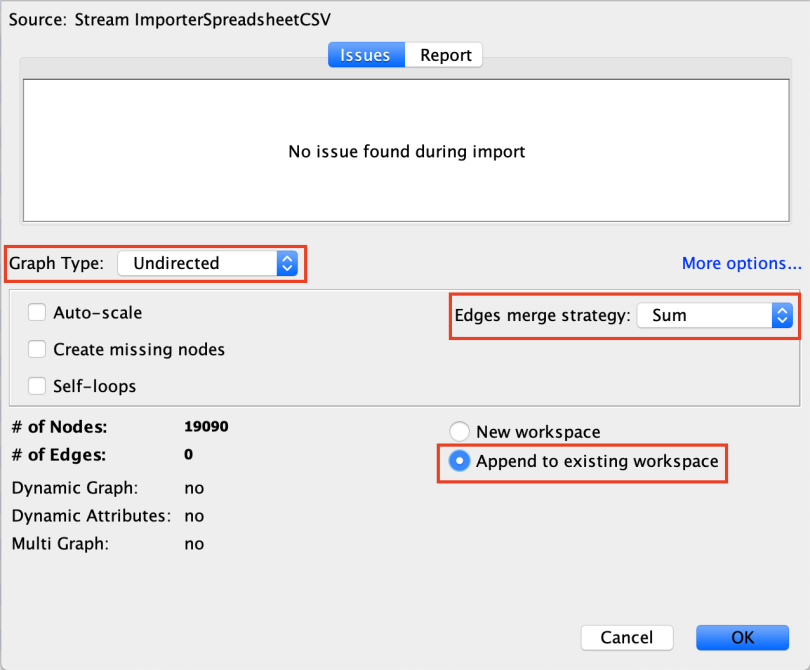
You should now see some data in the data laboratory window! Next we need to import the edges list.
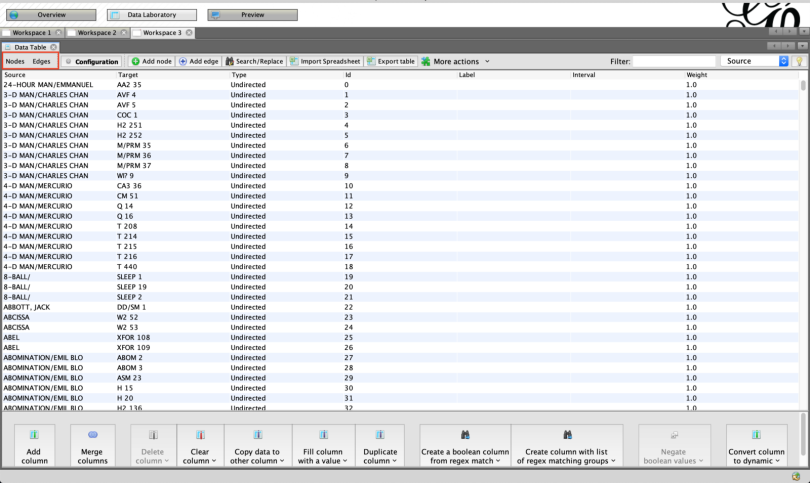
Now that you’ve imported the data it’s s time to view the graph. Click on the overview tab.
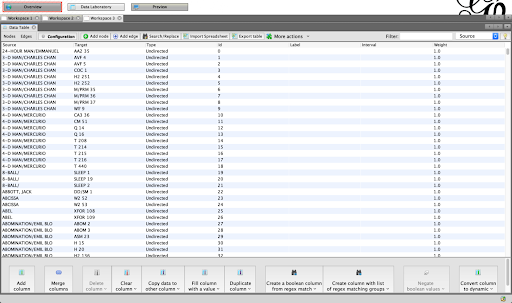
Using Layout Functions
You might be disappointed in the graph that was visualized. It will likely look like the black mess below.
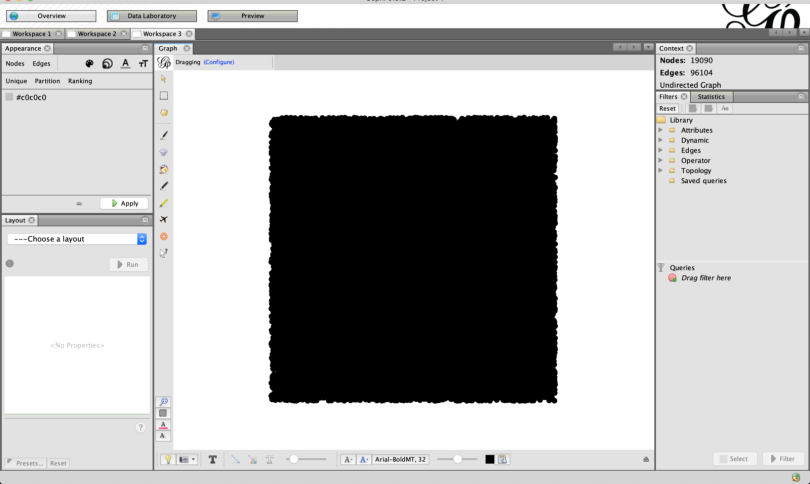
In order to make the graph more readable, we’ll need to use a layout function to change the position of nodes in the graph.
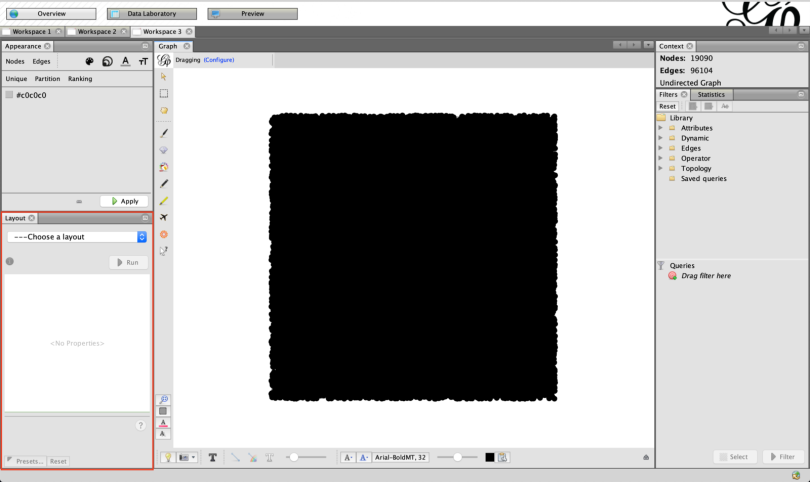
There are a variety of layout functions in Gephi but let’s focus on the ForceAtlas 2 function for now. Select this function and then click run. You’ll see the nodes move in real-time, and you can stop the function when you like the nodes’ position.
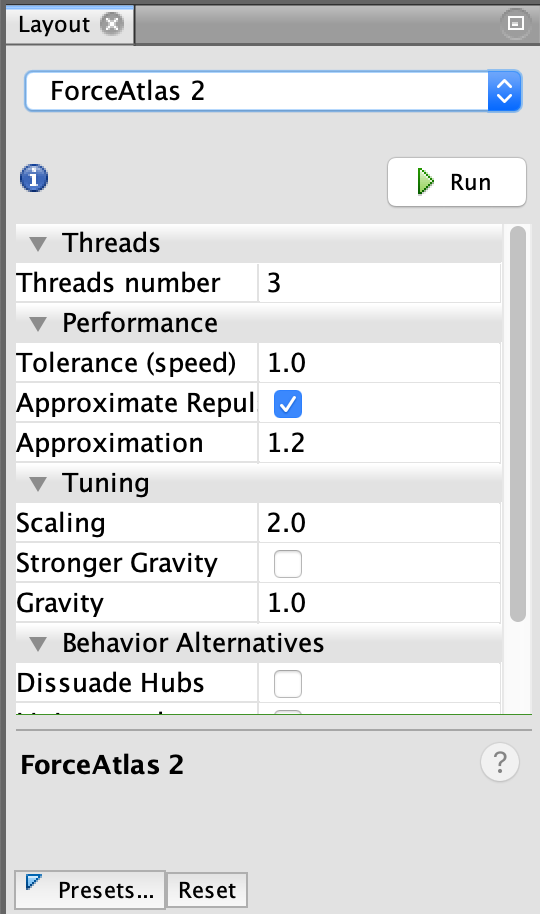
After running the layout function your graph should look something like the one below. You can continue to play with other layout functions if you wish to get a better node position. While we’re using the stock ForceAtlas 2 parameters, changing them can give you better control over the node positions. In addition, you can change the parameters of layout functions.
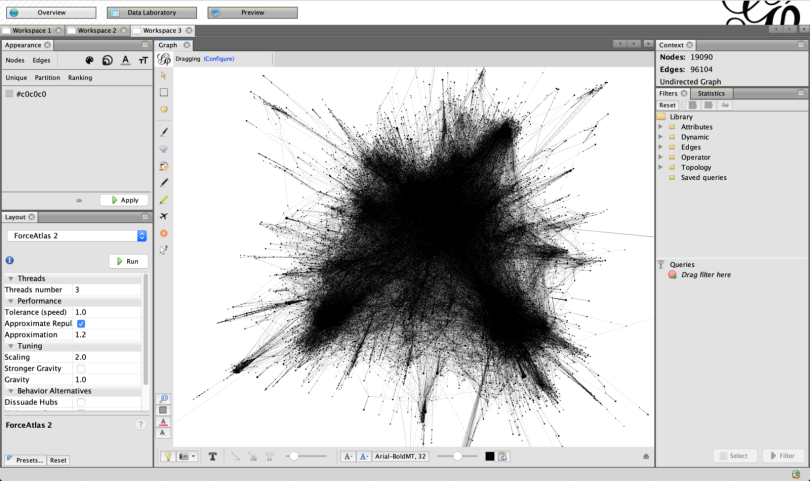
Calculating Network-Level Measures
Now let’s calculate the network size and density of this Marvel network.
The network size is easy to find. In the upper right-hand corner is a pane called context. This window provides the number of nodes and edges in the graph. Because a network’s size is the number of nodes in it, the network size of our Marvel network is 19,090.
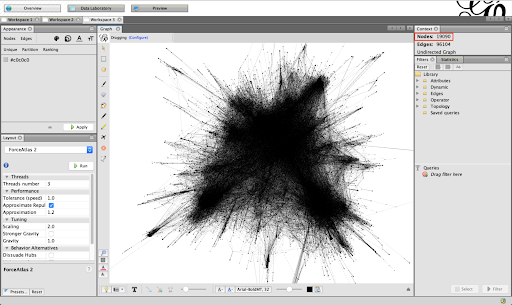
To find the network density, we’ll take our first dive into the statistics window. Click on the statistics tab.
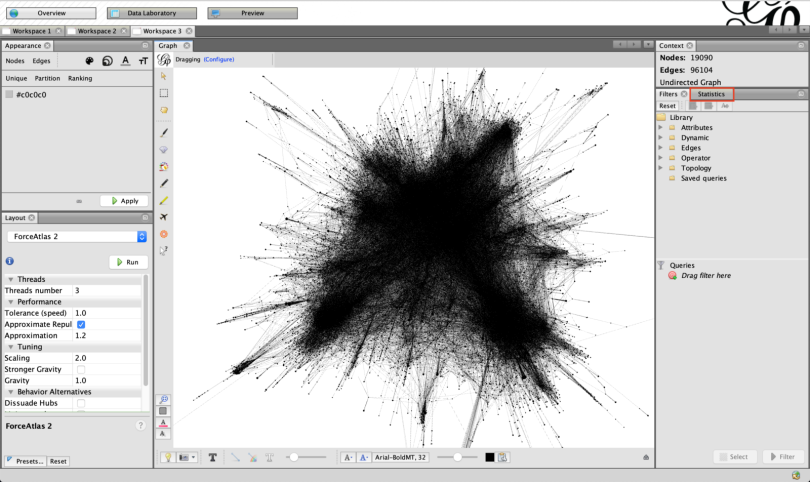
This is what you should see:
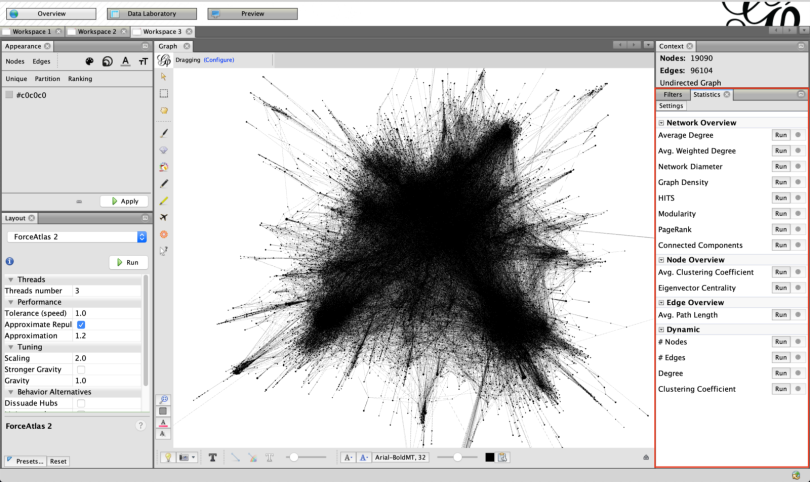
The statistics window contains many measures that can be calculated on the network. To find the network density, click run for graph density.
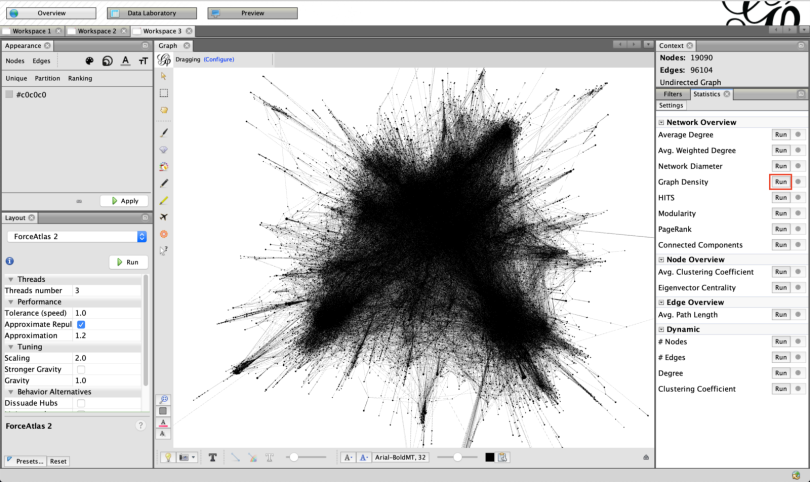
Select undirected, and then click OK.
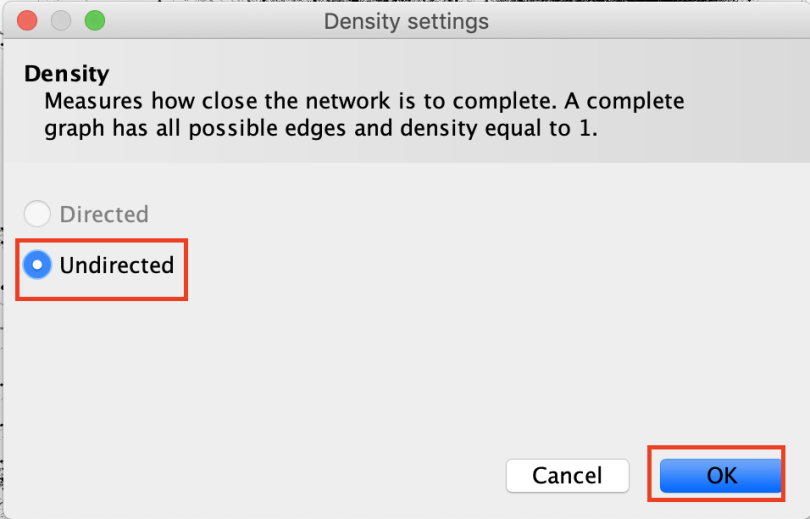
A new window will pop up showing the results. This Marvel network has a density of 0.001.
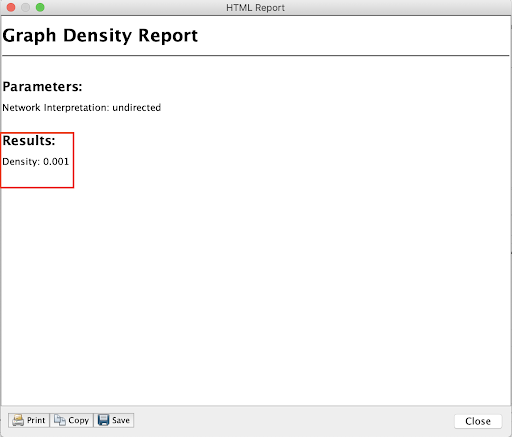
You can save this report by clicking the save button in the bottom left-hand corner, or close it by clicking the close button in the bottom right-hand corner.
Calculating Centrality Measures
Recall that centrality measures are on a node-level, and not a network-level. However, we can also average centrality measures to get a network-level metric. In Gephi, you calculate centrality measures as a network-level average, which then also inputs the centrality measure on a node-level into the data laboratory tab.
Node Degree
To calculate node degree, click run on the average degree algorithm in the statistics window.
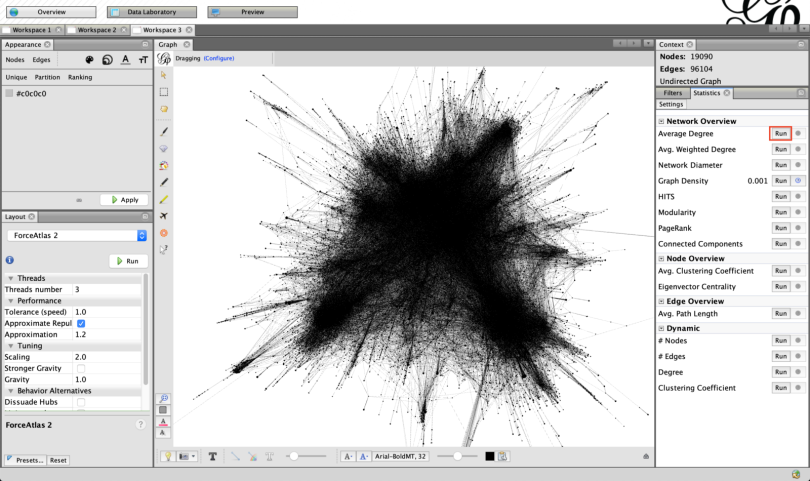
The report will provide you with the average degree for the network, as well as a distribution graph. While these can be useful in some applications, we are more interested in the degree on a node-level. Close the report.
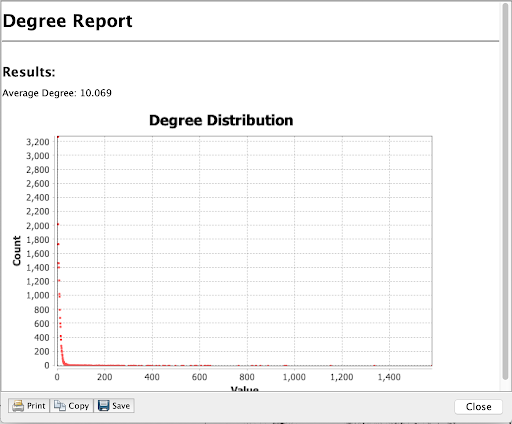
To see the degree for each node in the network, go back to the data laboratory window and click on the node table. You will see a new column in the data titled degree.
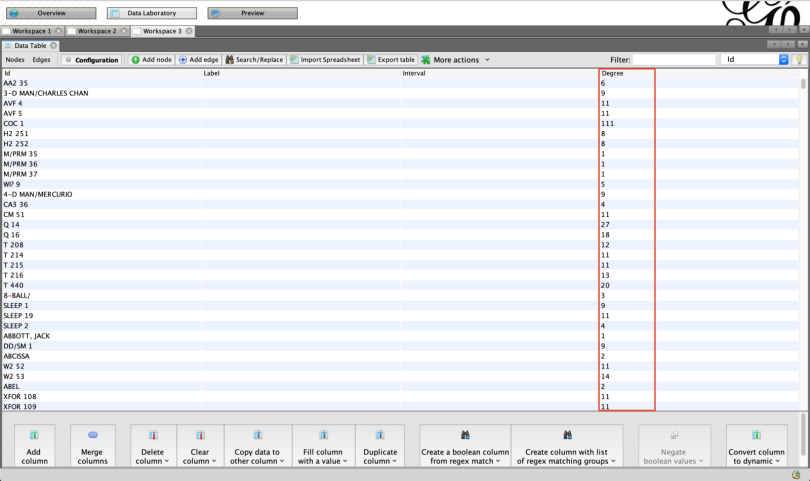
Node Closeness and Betweenness
Calculating node closeness and betweenness is a similar process as calculating node degree. In the statistics window, click run on the network diameter algorithm.
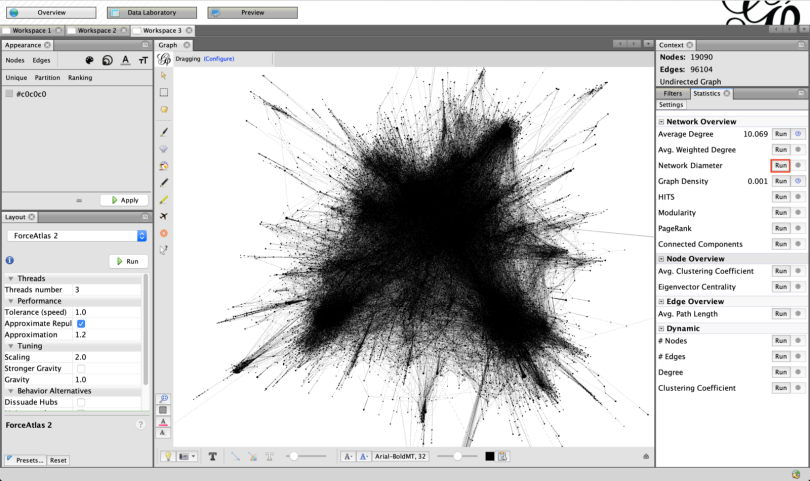
Select undirected and click OK. Depending on the specs of your machine this may take a little while to calculate.
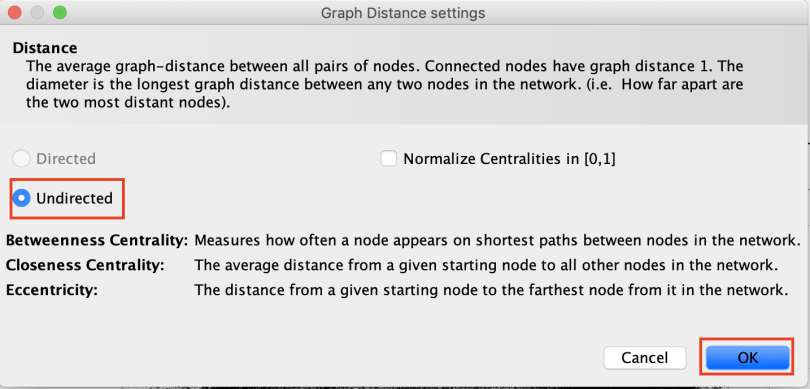
Like with the node measure, Gephi will provide a network-level report. Click close on this report and go to the data laboratory.
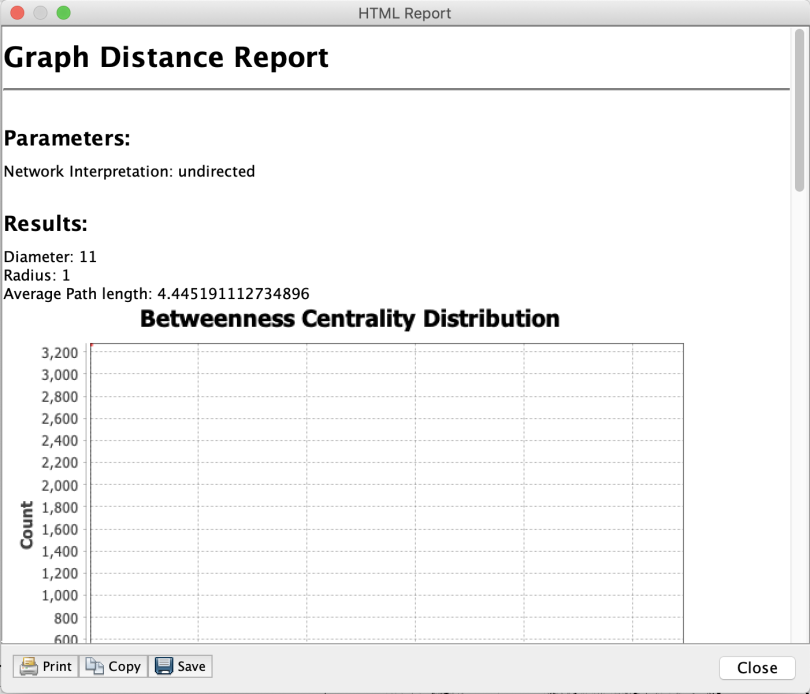
In the data laboratory, you’ll find additional columns in the node table including the node betweenness and closeness.
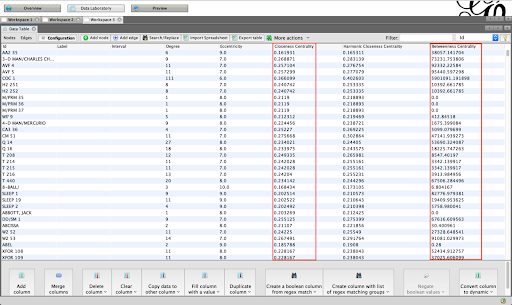
Calculating Edge Weights
Edge weights are auto-calculated in Gephi. You can find them in the edge list within the data laboratory.
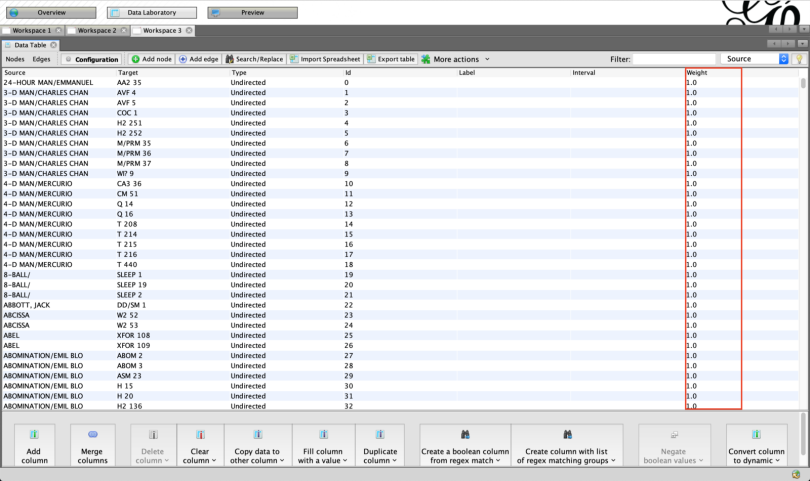
Using Color in Network Graphs
Currently, our graph nodes and edges are black, providing no additional information. You can color-code both nodes and edges in Gephi. The coloring options are in the appearance window.
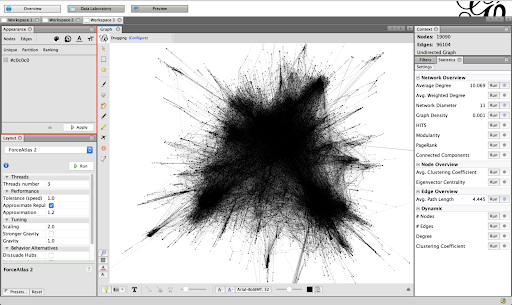
To color-code the nodes of the graph based on the node degree, click on the nodes button and the color palette button in the appearance window.
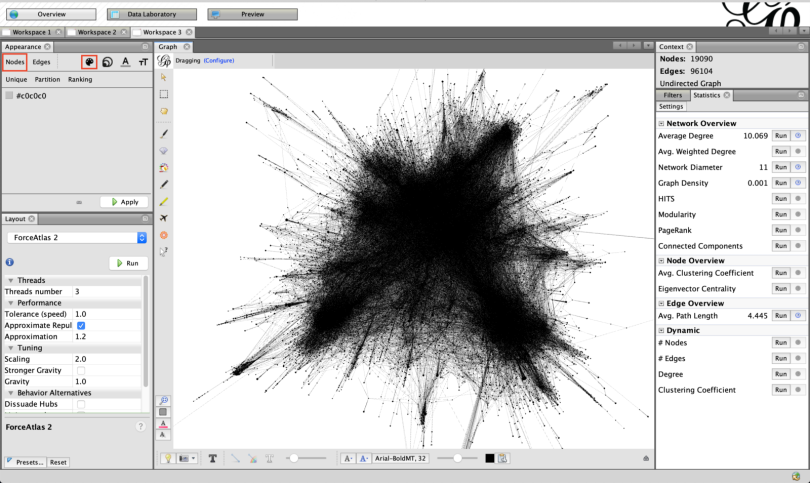
There are three options to encode information in the color of nodes: unique, partition and ranking. If you want to change all the graph’s nodes to the same color, use the unique window. Partition will break the nodes into color-coded groups. Ranking will color-code the nodes on a scale.
Let's color the nodes by their degree. To do this, click on the ranking section and select degree.
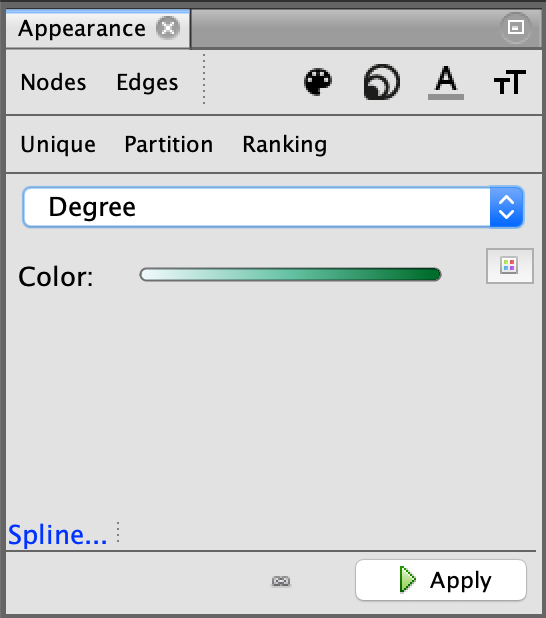
A color scale will be used to color the nodes. To select a new scale, click on the color selector button to the right of the color scale.
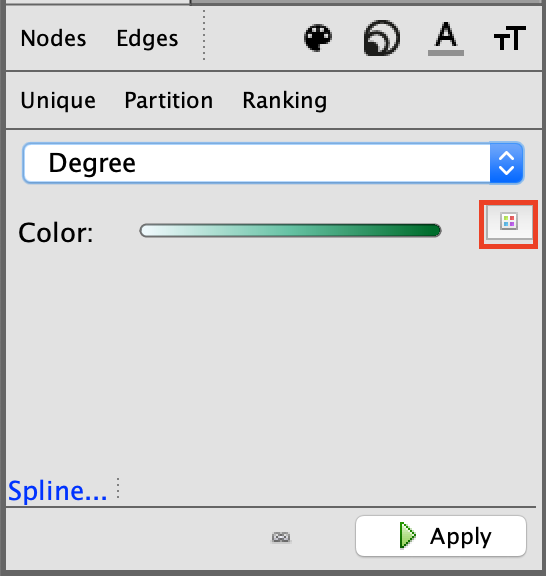
You can select any color scale to use. Then click apply.
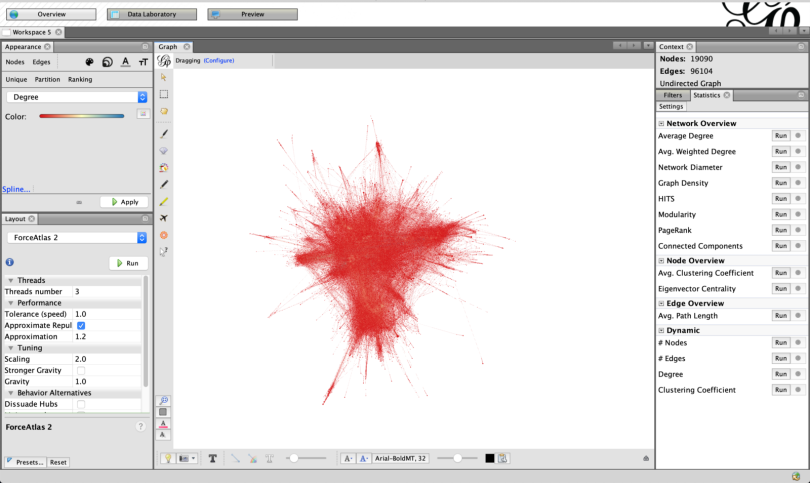
As you can see in the above image, coloring our nodes also colored our edges. You can change the color of edges to a specific color using the unique color tab for edges, or apply a ranking or partitioning color scale to them.
Using Size in Network Graphs
You may also notice that the majority of the graph is colored red. This is because most nodes in the graph have a low degree. Zooming in will show that some nodes are yellow or blue.
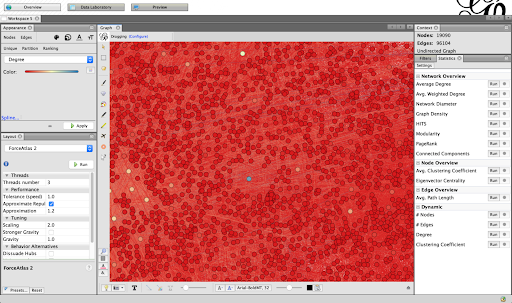
To make these nodes easier to see in the graph let’s scale the size of the nodes to the node degree as well. To do this click on the nodes and size buttons in the appearance window.
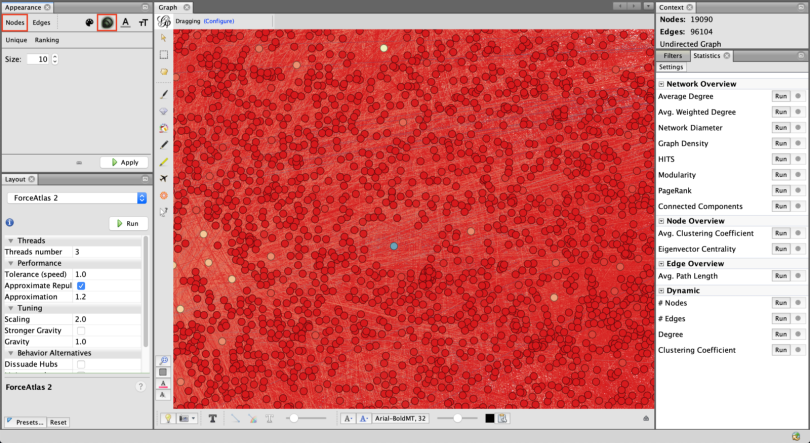
Then, click on ranking and select degree. Change the minimum size to 1 and the maximum size to 100.
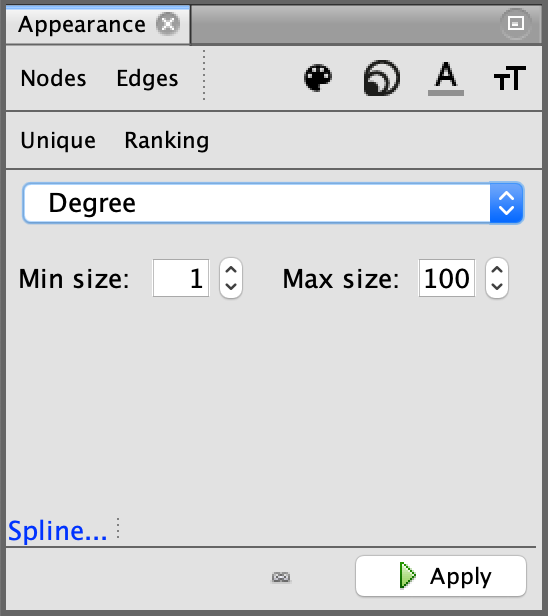
Then click apply. We can now better see what nodes have a high degree.
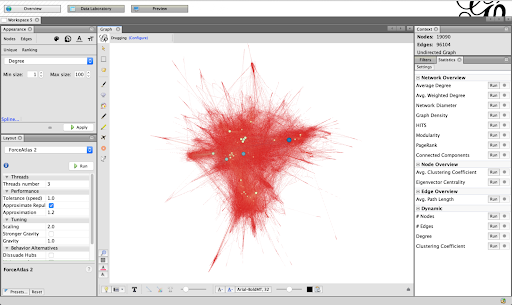
Changing Background Color
Let's also change the background from white to black. Depending on the colors in a graph, either color may look better and it’s often up to personal preference. To change the color to black, press the lightbulb button.
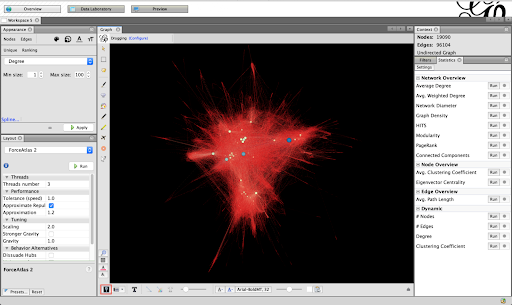
More from Expert Data Scientists How to Write Good Pseudocode
Putting It All Together
This should get you started as you make your first network graph using the Marvel data set and I encourage you to continue playing around with this graph in Gephi. There are many more measures you can calculate and other appearances you can use.
Your next step should be to take another data set and try to reproduce these steps. Eventually, you can try to collect your own data and transform it into a new social network data for analysis.
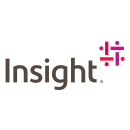
Built In’s expert contributor network publishes thoughtful, solutions-oriented stories written by innovative tech professionals. It is the tech industry’s definitive destination for sharing compelling, first-person accounts of problem-solving on the road to innovation.
Great Companies Need Great People. That's Where We Come In.
- Privacy Policy

Home » Social Network Analysis – Types, Tools and Examples
Social Network Analysis – Types, Tools and Examples
Table of Contents

Social Network Analysis
Social Network Analysis (SNA) is an analytical method used to study social structures through the use of networks and graph theory. It identifies the relationships between individuals, organizations, or other entities and examines the patterns and implications of these relationships.
The nodes in the network represent the actors within the networks and the ties or edges represent relationships between the actors. These might be, for example, friendship ties between people, business relationships between companies, or communication patterns between individuals.
By analyzing the network structure and the characteristics of the actors within the network, SNA can reveal properties such as the distribution of resources, the flow of information, or the overall connectivity of the network.
Here are a few key concepts in SNA:
- Centrality : This measures the importance of a node in the network. Various centrality measures exist, each emphasizing a different aspect of a node’s position within the network, such as degree centrality (the number of direct connections a node has), betweenness centrality (the number of times a node acts as a bridge along the shortest path between two other nodes), and eigenvector centrality (the sum of the centrality scores of all nodes that one node is connected to).
- Density : This is a measure of the proportion of possible connections in a network that are actual connections. A high density suggests that the network participants are highly interconnected.
- Clusters or Communities : These are groups of nodes that are more densely connected with each other than with the rest of the network.
- Structural Holes : These are gaps in the network where a node could potentially act as a bridge between two unconnected parts of the network.
Types of Social Network Analysis
Social Network Analysis can be broadly categorized based on the type of networks being analyzed, the level of analysis, and the methodologies employed. Here are a few ways to categorize SNA:
Whole Network Analysis
This type of analysis focuses on the structure and properties of the network as a whole. This might include measures of network cohesion, centralization, and density. It also looks at the overall distribution of relationships and identifies key groups or clusters within the network.
Ego Network Analysis
In this type of analysis, the focus is on a single actor (the ‘ego’) and their immediate network (the ‘alters’). It’s often used when interest is in the personal networks of individuals. Measures can include the size of the network, the composition of the network in terms of the types of ties and nodes, and measures of network density or diversity.
Two-mode (or Bipartite) Network Analysis
This type of SNA is used when there are two different types of nodes, and connections are only possible between nodes of different types (not within types). For example, authors and the books they write, or actors and the movies they appear in. In such a network, you can study the connections between nodes of one type, mediated by nodes of the other type.
Dynamic Network Analysis (DNA)
This is used to study how social networks evolve over time. This could involve studying how ties between actors develop or disappear, or how actors move around within the network. In addition to traditional network measures, DNA also considers measures that are dynamic in nature, such as change in centrality over time.
Semantic Network Analysis
This type of SNA focuses on the relationships between concepts or ideas, rather than individuals or organizations. For instance, semantic network analysis could map out how different scientific concepts are related to each other in the literature.
Social Media Network Analysis
A specialized form of SNA, this deals with the study of social relationships as expressed through social media platforms. It allows for the mapping and measuring of relationships and flows between people, groups, organizations, computers, URLs, and other connected information/knowledge entities.
Social Network Analysis Techniques
Social Network Analysis involves various techniques to understand the structure and patterns of relationships among actors (people, organizations, etc.) in a network. These techniques may be mathematical, visual, or computational, and often involve the use of specialized software. Here are several common SNA techniques:
Network Visualization
One of the most basic SNA techniques involves creating a visual representation of the network. This can help to reveal patterns and structures within the network that may not be immediately obvious from the raw data. There are various ways to create such visualizations, depending on the specifics of the network and the goals of the analysis. Software such as Gephi or Cytoscape can be used for network visualization.
Centrality Measures
These are techniques used to identify the most important nodes within a network. Various measures of centrality exist, each highlighting different aspects of a node’s position in the network. These include degree centrality (the number of connections a node has), betweenness centrality (how often a node appears on the shortest path between other nodes), closeness centrality (how quickly a node can reach all other nodes in the network), and eigenvector centrality (a measure of the influence of a node in a network).
Community Detection
Also known as clustering, this technique aims to identify groups of nodes that are more closely connected with each other than with the rest of the network. This can help to reveal sub-groups or communities within the network.
Structural Equivalence and Blockmodeling
Structural equivalence is a measure of how similarly two nodes are connected to the rest of the network. Nodes that are structurally equivalent often play similar roles in the network. Blockmodeling is a technique used to simplify a network by grouping together structurally equivalent nodes.
Dynamic Network Analysis
This involves studying how a network changes over time. This can help to reveal patterns of network evolution, including how relationships form and dissolve, how centrality measures change over time, and how communities evolve.
Network Correlation and Regression
These are statistical techniques used to identify and test for patterns within the network. For example, one might use these techniques to test whether nodes with certain characteristics are more likely to form connections with each other.
Social Network Analysis Tools
There are several tools available that can be used to conduct Social Network Analysis (SNA). These range from open-source software to commercial offerings, each with their own strengths and weaknesses. Here are a few examples:
- Gephi : Gephi is an open-source, interactive visualization and exploration platform for all kinds of networks and complex systems. It’s user-friendly and allows users to interactively manipulate the network visualization, perform network analysis, and export results in various formats.
- UCINet : UCINet is a comprehensive package for the analysis of social network data as well as other 1-mode and 2-mode data. It’s widely used in social science research.
- NetDraw : Often used in conjunction with UCINet, NetDraw is a free tool for visualizing networks. It supports the visualization of large networks and allows for various customization options.
- Pajek : Pajek is a program for the analysis and visualization of large networks. It’s an extensive tool, offering a range of complex network metrics, and is free for non-commercial use.
- NodeXL : NodeXL is a free, open-source template for Microsoft Excel that allows users to display and analyze network graphs. Its integration with Excel makes it user-friendly, particularly for those already familiar with Excel.
- Cytoscape : Originally designed for biological research, Cytoscape is now a popular open-source software platform for visualizing complex networks and integrating these with any type of attribute data.
- SocioViz : SocioViz is a social media analytics platform for Twitter data, focused on network analysis and visualization. It’s a powerful tool for researchers interested in online social networks.
- NetworkX : NetworkX is a Python package for the creation, manipulation, and study of the structure, dynamics, and functions of complex networks. It integrates well with other scientific Python tools like SciPy and Matplotlib.
- igraph : igraph is a library available in R, Python, and C for creating, manipulating, and analyzing networks. It’s highly efficient and can handle large networks.
- RSiena : RSiena is an R package dedicated to the statistical analysis of network data, with a particular focus on longitudinal social networks.
Social Network Analysis Examples
Social Network Analysis Examples are as follows:
- Public Health – COVID-19 Pandemic : During the COVID-19 pandemic, SNA was used to model the spread of the virus. The interactions between individuals were mapped as a network, helping identify super-spreader events and informing public health interventions.
- Business – Google’s “PageRank” Algorithm : Google’s PageRank algorithm, which determines the order of search engine results, is a type of SNA. It considers web pages as nodes and hyperlinks as connections, determining a page’s importance by looking at the number and quality of links to it.
- Sociology – Stanley Milgram’s “Small World” Experiment : This is one of the most famous social network experiments, where Milgram demonstrated that any two people in the United States are separated on average by only six acquaintances, leading to the phrase “six degrees of separation.”
- Online Social Networks – Facebook’s “People You May Know” Feature : Facebook uses SNA to suggest new friends. The platform analyzes your current network and suggests people you’re likely to know, typically friends of friends or people who share common networks.
- Criminal Network Analysis – Capture of Osama bin Laden : SNA was reportedly used in the operation to capture Osama bin Laden. By mapping the social connections of known associates, intelligence agencies were able to locate the Al-Qaeda leader.
- Academic Research – Collaboration Networks : SNA is used in scientometrics to analyze collaboration networks among researchers . For example, a study on co-authorship networks in scientific articles can reveal patterns of collaboration and the flow of information in different disciplines.
When to use Social Network Analysis
Social Network Analysis is a powerful tool for studying the relationships between entities (like people, organizations, or even concepts) and the overall structure of these relationships. Here are several situations when SNA might be particularly useful:
- Understanding Complex Systems : SNA is well-suited to studying complex, interconnected systems. If you’re interested in not just individual entities but also the relationships between them, SNA can provide valuable insights.
- Identifying Key Actors : SNA can help identify the most important entities in a network based on their position and connections. These might be influential people within a social network, critical servers in a computer network, or key scholars in a field of study.
- Studying Diffusion Processes : If you’re interested in how something (like information, behaviors, diseases) spreads through a network, SNA can be a valuable tool. It allows for the examination of diffusion pathways and identification of nodes that speed up or hinder diffusion.
- Detecting Communities : SNA can be used to identify clusters or communities within a network. These might be groups of friends within a social network, clusters of companies in a business network, or research clusters in scientific collaboration networks.
- Mapping Out Large Systems : In cases where you have a large system of many interconnected entities, SNA can provide a visual representation of the system, making it easier to understand and analyze.
- Investigating Structural Roles : If you’re interested in the roles individuals or entities play within their network, SNA offers methods to classify these roles based on the pattern of their relationships.
Purpose of Social Network Analysis
Social Network Analysis serves a wide range of purposes across different fields, given its versatile nature. Here are several key purposes:
- Understanding Network Structure : One of the key purposes of SNA is to understand the structure of relationships between actors within a network. This includes understanding how the network is organized, the distribution of connections, and the patterns of interaction.
- Identifying Key Actors or Nodes : SNA can identify crucial nodes within a network. These could be individuals with many connections, or nodes that serve as critical links between different parts of the network. In a business, for instance, such nodes might be key influencers or innovators.
- Detecting Subgroups or Communities : SNA can identify clusters or communities within a network, i.e., groups of nodes that are more connected to each other than to the rest of the network. This can be valuable in numerous contexts, from identifying communities in social media networks to detecting collaboration clusters in scientific networks.
- Analyzing Information or Disease Spread : In public health and communication studies, SNA is used to study the patterns and pathways of information or disease spread. Understanding these patterns can be critical for designing effective interventions or campaigns.
- Analyzing Social Capital : SNA can help understand an individual or group’s social capital – the resources they can access through their network relationships. This analysis can offer insights into power dynamics, access to resources, and inequality within a network.
- Studying Network Dynamics : SNA can examine how networks change over time. This could involve studying how relationships form or dissolve, how centrality measures change over time, or how communities evolve.
- Predicting Future Interactions : SNA can be used to predict future interactions or relationships within a network, which can be useful in a variety of settings such as recommender systems, predicting disease spread, or forecasting emerging trends in social media.
Applications of Social Network Analysis
Social Network Analysis has a wide range of applications across different disciplines due to its capacity to analyze relationships and interactions. Here are some common areas where it is applied:
- Public Health : SNA can be used to understand the spread of infectious diseases within a community or globally. It helps identify “super spreaders” and optimizes strategies for vaccination or containment.
- Business and Organizations : Companies use SNA to analyze communication and workflow patterns, enhance collaboration, boost efficiency, and detect key influencers within their organization. It can also be applied in understanding and leveraging informal networks within a business.
- Social Media Analysis : On platforms like Facebook, Twitter, or Instagram, SNA helps analyze user behavior, track information dissemination, identify influencers, detect communities, and develop recommendation systems.
- Criminal Justice : Law enforcement and intelligence agencies use SNA to understand the structure of criminal or terrorist networks, identify key figures, and predict future activities.
- Internet Infrastructure : SNA helps in mapping the internet, identifying critical nodes, and developing strategies for robustness against cyberattacks or outages.
- Marketing : In marketing, SNA can track the diffusion of advertising messages, identify influential consumers for targeted marketing, and understand consumer behavior and brand communities.
- Scientometrics : SNA is used in academic research to map co-authorship networks or citation networks. It can uncover patterns of collaboration and the flow of knowledge in scientific fields.
- Politics and Policy Making : SNA can help understand political alliances, lobby networks, or policy networks, which can be critical for strategic decision-making in politics.
- Ecology : In ecological studies, SNA can help understand the relationships between different species in an ecosystem, providing valuable insights into ecological dynamics.
Advantages of Social Network Analysis
Social Network Analysis offers several advantages when studying complex systems and relationships. Here are a few key advantages:
- Reveals Complex Relationships : SNA allows for the study of relationships between entities (be they people, organizations, computers, etc.) in a way that many other methodologies do not. It emphasizes the importance of these relationships and helps reveal complex interaction patterns.
- Identifies Key Players : SNA can identify the most influential or important nodes in a network, whether they are individuals within a social network, key servers in an internet network, or central scholars in an academic field.
- Unveils Network Structure and Communities : SNA can help visualize the overall structure of a network and can reveal communities or clusters of nodes within a network. This can provide valuable insights into the organization and division of a network.
- Tracks Changes Over Time : Dynamic SNA allows the study of networks over time. This can help to track changes in the network structure, the role of specific nodes, or the flow of information or resources through the network.
- Helps Predict Future Interactions : Based on the analysis of current and past relationships, SNA can be used to predict future interactions, which can be useful in many fields including public health, marketing, and national security.
- Aids in Designing Effective Strategies : The insights gained from SNA can be used to design targeted strategies, whether that’s intervening in the spread of misinformation online, designing a targeted marketing campaign, disrupting a criminal network, or managing collaboration in an organization.
- Versatility : SNA can be applied to a vast array of fields, from sociology to computer science, biology to business, making it a versatile tool.
Disadvantages of Social Network Analysis
While Social Network Analysis is a powerful tool with wide-ranging applications, it also has certain limitations and disadvantages that are important to consider:
- Data Collection Challenges : Collecting complete and accurate network data can be a major challenge. For larger networks, it may be nearly impossible to collect data on all relevant relationships. There’s also a risk of response bias, as people may forget, overlook, or misinterpret their relationships when providing data.
- Time and Resource Intensive : Collecting network data, especially from primary sources, can be extremely time-consuming and expensive. Additionally, analyzing network data can also require significant computational resources for larger networks.
- Complexity : SNA involves complex concepts and measures, which can be difficult to understand without specialized knowledge. This complexity can make it difficult to communicate findings to a non-technical audience.
- Privacy and Ethical Concerns : SNA often involves sensitive data about individuals’ relationships and interactions, raising important privacy and ethical concerns. It’s important to handle this data carefully to respect individuals’ privacy.
- Static Snapshots : Traditional SNA often provides a static snapshot of a network at a particular point in time, which may not capture the dynamic nature of social relationships. While dynamic SNA does exist, it adds additional complexity and data demands.
- Dependence on Quality of Data : The insights and conclusions drawn from SNA are only as good as the data used. Incomplete, inaccurate, or biased data can lead to misleading results.
- Difficulties in Establishing Causality : While SNA can reveal patterns and associations in network data, it can be difficult to establish causal relationships. For instance, do strong connections between two individuals lead to similar behavior, or does similar behavior lead to strong connections?
- Assumptions about Relationships : SNA often assumes that relationships are equally important, which might not always be the case. Different relationships might have different strengths or meanings, which can be challenging to represent in a network.
About the author
Muhammad Hassan
Researcher, Academic Writer, Web developer
You may also like

Digital Ethnography – Types, Methods and Examples

Predictive Analytics – Techniques, Tools and...

Big Data Analytics -Types, Tools and Methods

Diagnostic Analytics – Methods, Tools and...

Blockchain Research – Methods, Types and Examples

What is Data Science? Components, Process and Tools
- Original Article
- Open access
- Published: 20 October 2013
Social network analysis in innovation research: using a mixed methods approach to analyze social innovations
- Nina Kolleck 1
European Journal of Futures Research volume 1 , Article number: 25 ( 2013 ) Cite this article
18k Accesses
24 Citations
5 Altmetric
Metrics details
The importance of social networks for innovation diffusion and processes of social change is widely recognized in many areas of practice and scientific disciplines. Social networks have the potential to influence learning processes, provide opportunities for problem-solving, and establish new ideas. Thus, they can foster synergy effects, bring together key resources such as know-how of participating actors, and promote innovation diffusion. There is wide agreement regarding the usefulness of empirical methods of Social Network Analysis (SNA) for innovation and futures research. Even so, studies that show the chances of implementing SNA in these fields are still missing. This contribution addresses the research gap by exploring the opportunities of a mixed methods SNA approach for innovation research. It introduces empirical results of the author’s own quantitative and qualitative investigations that concentrate on five different innovation networks in the field of Education for Sustainable Development.
Introduction
Scholars interested in innovation processes and futures research have often stressed the importance of social networks. Social networks are seen as an important factor in how ideas, norms, and innovations are realized. Social network research understands individuals within their social context, acknowledging the influence of relationships with others on one’s behavior. Hence, social networks can promote innovation processes and expand opportunities for learning. Despite the consensus regarding the value of social network approaches, there is a lack of empirical investigations in innovation and futures studies that use Social Network Analysis (SNA). In most cases, the scientific literature uses the concept of social networks metaphorically, ignoring the chances presented by SNA methods. At the same time, conventional empirical research in innovation and futures studies often disregards relational information. Hence, analyses of statistical data on structural and individual levels are treated as separately. Activities that are expected to have impacts on future developments are usually modeled as isolated individual or group behavior, on the one hand, or as the characteristics of structural issues, on the other hand. SNA provides us with empirical tools that capture the social context and help to better understand how innovations are implemented and diffused and why social change takes place. Network approaches explicitly challenge the difference between deduction and induction and highlight the relevance of relationships. Individuals both shape and are shaped by the social context in which they interact. By applying techniques of SNA, actor-centered and structuralist reductions are avoided. Instead, SNA emphasizes the mutual influence of structure and social connections. In order to better understand and model developments in innovation and futures research, relational data inherent to the social network perspective is needed.
This contribution addresses the opportunities of SNA for innovation research. It is divided into six sections. After this introduction , the second section briefly defines crucial concepts of SNA and provides theoretical background. The third section discusses the value of a social network perspective for innovation research. The methodological approach, along with the empirical case studies used, is outlined in the fourth section. The fifth section shows how a combination of both insights from structure based on quantitative SNA and subjective perceptions revealed with qualitative SNA is helpful for understanding innovation processes. Here, the integration of qualitative SNA such as egocentric network maps in quantitative techniques of SNA is illustrated. The contribution concludes with a summary of main arguments.
Theoretical and methodological background
While in the scientific literature there are diverse understandings on what a social network is, this contribution draws on the definition used by Stanley Wassermann and Katherine Faust:
“A social network consists of a finite set or sets of actors and the relation or relations defined on them. The presence of relational information is a critical and defining feature of a social network” [ 1 ].
This conception of social network permits both a governance approach and empirical techniques of SNA. Scholars of governance research understand social networks as a certain type of governance that can be differentiated from other ideal types of governance: markets and hierarchies. Social networks combine market-based and hierarchic dimensions and serve as a form of hybrid governance [ 2 ]. Both weak and strong modes of coordination are integrated into the network concept of governance research, where strong coordination is defined as “the spectrum of activity in which one party alters its own … strategies to accommodate the activity of others in pursuit of a similar goal” [ 3 ]. Weak coordination, on the other hand, takes place when actors observe each other’s behavior, “and then alter their actions to make their … strategies complementary with respect to a common goal” [ 3 ].
Because they promote constant exchange and deliberation, social networks have strong potential to promote ideological or structural changes and to generate new knowledge. Hence, network governance is not reduced to governmental action, but refers to the search for collective and participative problem-solving strategies and the promotion of innovations. Footnote 1 This article uses the concept of network governance to highlight the relevance of relationships for innovation research. Hence, it confronts the assumption that individual behavior is independent of any others, but instead conceives “problem-solving as a collaborative effort in which a network of actors, including both state and non-state organizations, play a part” [ 4 ]. Footnote 2
In order to better understand the opportunities of SNA for innovation research, this contribution introduces innovation networks in five different regions as case studies. Innovation networks are understood as social networks that aim at establishing a social innovation. Here, the social innovation of Education for Sustainable Development (ESD) is used. At the same time, the term social innovation refers to processes of implementing and diffusing new social concepts across different sectors of society. While “innovation” implies a kind of renewal, “social” connotes interaction of actors. Social innovations have a direct connection with the search for solutions to social problems and challenges [ 6 , 7 ]. Likewise, Education for Sustainable Development can be defined as education that empowers people to foresee, try to understand, and solve the problems that threaten life on our planet. With the goal of promoting behavioral changes that will shape a more sustainable future, ESD integrates principles of sustainable development into all aspects of education and learning.
Change and innovation through social relations
How can social networks evoke changes and what are the opportunities for SNA to promote innovation processes? SNA has the potential to overcome uncertainties related to innovation processes. The chance of an innovation gaining acceptance increase significantly if it is supported by interconnected actors rather than singular individuals. Social networks foster change processes and promote innovation diffusion. SNA techniques thus help to understand existing networks and to identify innovation potentials in order to generate new information and reveal options for structural developments. SNA has the capacity to promote innovation processes by dealing with the following issues:
Identification of innovation networks (existing, missing, possible, and realistic cooperation) and investigation of actors, structures, and network boundaries:
By using SNA methods, network structures were determined in previously defined fields. Thus, techniques of egocentric SNA provide us with necessary information with respect to network membership and structural interconnections between actors. Structural properties detected in the context of this project are, for example, centrality, prestige, or weak and strong ties.
Innovation potentials through network development strategies:
Looking at network structures not only fosters the development and diffusion of new ideas. It can also reveal where and how structural conditions enable innovations and development processes. Furthermore, Social Network Analyses disclose where and how cooperation can be optimized and where and how alterations are possible and reasonable. Presenting stakeholders the results of SNA can foster structural changes.
Identification and promotion of coordination, information, and motivation:
Analysis of social networks provides us with useful insights into knowledge transfer processes, showing where they exist and how “well” they function. Also, problems of coordination, information, and motivation become evident, providing us with knowledge related to development potentials.
Development of strategies to reduce uncertainties related to innovation processes:
The costs of information exchange are not only material (money, time), but also social. Uncertainties, lack of confidence, and the fear of a loss of reputation can prevent actors from sharing information and knowledge. Results of SNA help us to identify weaknesses in the knowledge transfer process.
Social network analysis in innovation research
In order to illustrate the key opportunities of SNA in innovation research, this section draws on the author’s own empirical investigations that used a mixed methods approach based on quantitative and qualitative SNA. Data on network members was drawn from five different German municipalities and included initiatives, institutions, thematic groups, and individuals engaged in the field of ESD. The municipalities studied are Alheim, Erfurt, Frankfurt am Main, Gelsenkirchen, and Minden. These municipalities have been awarded by the United Nations Decade of Education for Sustainable Development (UNDESD), 2005–2014, and are characterized by active networks in the field of the social innovation of ESD. Organizations, initiatives, and actors from different sectors of non-formal, informal, and formal education seek to further establish and diffuse the concept of ESD worldwide. Thus, networks within these municipalities can be regarded as best practices concerning their performance in the area of ESD. It should be taken into account, however, that the social networks analyzed here are neither institutionalized nor formally established organizations. Instead, every person engaged in the field of the social innovation is regarded as part of the network to be analyzed. Hence, defining the network boundaries was an important part of the empirical investigation.
The research design included three main steps. First, qualitative data was collected in order to gain a better understanding of the object of research and generate research hypotheses. Second, quantitative SNA was conducted, using both egocentric SNA and complete SNA techniques. Network membership and network boundaries were defined by mixed-mode egocentric SNA. In a first step, a 12-page questionnaire was sent to all persons in each of the five municipalities listed in the data base of the UNDESD. In a second step, all persons from different sectors named more than once were also approached with the questionnaire [ 8 – 10 ]. Referring to Fischer [ 11 ] and Burt [ 12 , 13 ], a name generator was used which allowed to name all relevant persons in the field of ESD. In this way, nodes were only included if they were mentioned more than once by an interviewee in the field of ESD.
The questionnaire first asked respondents to mark people in their ESD network, defined by efforts to contact, cooperation, collaboration, problem-solving, and idea exchange. Respondents were also asked to assess the quality and contact frequency for each relation mentioned and to name those persons with whom the interviewee cooperated especially closely or had established high levels of trust. They were then requested to score their named connections’ impact and the relevance with respect to the diffusion of information and the implementation of ESD. Finally, the questionnaire included questions on future prospects, desires, and developmental possibilities.
Egocentric network data was aggregated in order to enable applications of complete SNA. The (strictly adjusted) dataset of the whole network of all five municipalities consists of 1,306 persons and 2,195 edges. Subsequent to the quantitative studies, qualitative network maps were created in order to gain deeper insights into the qualitative characteristics of the networks’ structural properties. Footnote 3 This article focuses mainly on results from the second and the third part of the data analysis.
Insights from structures and individuals: engaging top-down and bottom-up approaches
Empirical results were visualized drawing back on UCINET, Netdraw, and Pajek in order to provide a comprehensive foundation for stakeholders [ 14 , 15 ]. Top-down visualizations of network data were used to generate courses of action, guidance, and network management strategies with the persons involved in the process. Thus, network visualizations and empirical insights enabled stakeholders to detect weaknesses related to structural issues, information flows, and communication problems.
In order to visualize the networks, directional relations between network members were entered into UCINET and mapped with Netdraw. The iterative method of “spring embedding” was chosen for the graph-theoretic layout, because it supports neat illustrations of data sets. Thus, the lengths of the ties do not have information content. The nodes in network visualizations represent persons engaged in implementing ESD in their municipalities. Against the backdrop of the definition of network boundaries, persons that are represented by nodes with only one ingoing link and no outgoing link were not interviewed.
To give an example, one surprising result was the low level of cooperation beyond municipal borders, as measured by network connections, as seen in Fig. 1 .
Trans-regional ESD network, generated with the graph theoretical layout spring embedding, source: Author’s data
In contrast to Manuel Castells [ 16 ], who observed a diminishing relevance of space due to the information age, the present study finds that space remains a constraint for diffusion of ESD. It seems much easier to establish the social innovation ESD in the local context with dense network structures and to subsequently foster its diffusion through weak ties [ 17 , 18 ].
Furthermore, municipal stakeholders were confronted with the unexpected existence of many structural holes and brokerage positions. The concepts “brokerage” and “structural hole” refer to actors’ structural embeddedness. A person who maintains connections with people, who do themselves not become interconnected, has the ability to mediate between these contacts and to obtain benefits from his brokerage position [ 19 – 21 ]. At the same time, structural holes impede innovation processes and information flows.
Figures 2 and 3 take Erfurt and Gelsenkirchen as examples and show relations regarding to the question of who is contacted to develop new ideas related to ESD. Only those relationships with a contact frequency of at least once a month are represented in this figure. The ESD network of Erfurt is chosen as an extreme example, because the structure of its social network exhibits the highest number of structural holes.
Cooperation in the development of new ideas in Erfurt, source: Author’s data
Cooperation in the development of new ideas in Gelsenkirchen, source: Author’s data
There are only a few network members engaged in developing new ideas with respect to ESD in Erfurt; many structural holes shape the ESD network.
In contrast, cooperation in the development of new ideas related to ESD works very well in Gelsenkirchen, as seen in Fig. 3 .
Figure 3 presents productive relationships in Gelsenkirchen. Gelsenkirchen was chosen as an example here because it demonstrates a nearly perfect cooperation basis, which is very supportive for successful innovation processes. Such results can be used by involved actors in order to disclose strengths and weaknesses and reveal where and how structural conditions enable innovations and development processes.
The network visualizations shown so far are mainly reduced to structural information. Network visualizations can also integrate further actor-related information. Not least, structural characteristics of social networks, processes of innovation, idea exchange, and trust also depend on the areas of activity to which network members belong. Thus, Fig. 4 integrates some actor-specific information. Nodes represent those people who are actively engaged in the field of ESD in Alheim. The color of the nodes indicates the sector in which the relevant person deals with ESD. The size of the nodes correlates with the individual centrality index. Centrality is measured by the frequency of the responses—the indegree [ 1 , 22 ]. The more often a person was identified by others, the more central she appears in the picture. The thickness of the connections varies depending on its individual clustering value. While there are two different measures (global and local) for clustering, the local version was used to give an indication of the embeddedness of single nodes [ 23 ]. Thus, clustering is defined as the number of common acquaintances; the thickness of the arrow connecting two nodes points to the number of triangular connections.
ESD network in Alheim; color of the nodes according to the area of activity ( blue black : non-formal education, red : administration/policy, yellow : NGOs, green : economy, light blue : formal education, orange : church, grey : other areas), numbers indicate the IDs of individuals, illustration in cooperation with fas.research, source: Author’s data
Figure 4 indicates the central role that people in the field of non-formal education play in Alheim, as measured by how often they were named by other people. Another central position is held by someone in government. The big red node has many incoming and outgoing links, but few triangle relations and thus a low clustering value. Further comparative quantitative studies reveal that despite its high density value, there is little clustering in Alheim. Certainly, the clustering value always depends on the data collection process, but as the study for this article has used the same methodological approach for all five municipal networks, it is possible to compare the municipal clustering values. However, the low clustering value in Alheim is because cooperation beyond institutional borders works very well in this municipality and persons are not always connected to the same partners. The fear expressed by other municipalities, that ESD in Alheim would be dominated by powerful politicians, cannot be confirmed from these results.
In general, quantitative SNA is able to highlight network boundaries and structural characteristics of social networks that are important to understand innovation potential and impediments. It is difficult or even impossible, however, to reveal the causes, motivations, ideas, or perceptions that lie behind such network structures by solely drawing back on quantitative SNA. How, for example, can we explain the central role of one politician in Alheim, while there are many other central persons from non-formal education? What role does this central politician play for the clustering value in Alheim? In order to answer these questions, the study had to draw on further qualitative social network research methods. The researchers thus used a combination of egocentric network maps and semi-structured interviews.
Egocentric network maps are more individual-oriented than quantitative SNA methods. One benefit of network map visualizations lies in their potential for mental or cognitive support. Such visualizations are able to promote subjective validations of interview narratives as well as to highlight subjective perceptions, reasons, motivations, and network dynamics. The technique of structured and standardized network maps, which has often been described as the “method of concentric circles” [ 24 ], was chosen for this study [ 25 ]. Here, network maps are not only aids, but a main purpose of the survey. A sheet with four concentric circles is given to the interviewee. The inner circle represents the ego, that is to say the interviewee. Interviewees are then asked to draw the initials of people important to them personally, differentiated by the degree of emotional proximity or contact frequency. The three circles around the ego represent the emotional closeness or formal distance with respect to her or his alters (or connections). The closer to the ego, the tighter a contact person is perceived by the interviewee. In addition, the circles are divided in parts through lines; each part represents a different area of activity. In this way, interviewees can dedicate their contacts to specific areas of activity, such as civil society, formal education, non-formal education, business or government. The space around ego is structured by both concentric circles that illustrate the closeness of the alters to ego and the area of activity in which alters are engaged for ESD. An essential advantage of the structured and standardized instruments in relation to unstructured techniques lies in the comparability between different network cards (both intrapersonal and interpersonal).
At the same time, the high degree of structuring and standardization constrains the significance of the data obtained. Indications beyond the pre-fixed circles are only possible if interviewers get the opportunity to pose further questions or if interviewees are encouraged to further discuss issues that are not explicitly part of the visualization process. In order to combine both standardization and openness, this study enabled the interviewers to pose further important questions and explore relevant information related to the research aims. The application of egocentric network maps also served as a medium through which interviewees talked about their relationships. In this sense, network maps were integrated into semi-structured interviews in order to generate narratives and disclose relevant relationships and action orientation. In addition, interviewees had the opportunity to choose the categories representing different areas of activity as well as the colors for the visualizations. Thus, the technique implemented in the study supported the comparability of the cases, but it was also open for new variables and dimensions related to the specific context.
Altogether 25 network maps and interviews, five in every municipality, were generated. Interviewees were chosen according to their area of activity (to obtain a variance of the cases), their position within the social network, and their centrality indexes. To give an example, Fig. 5 presents the network map of a central politician in Alheim. This network map of Alheim is also chosen to further illustrate the case of Alheim, which was also depicted in Fig. 4 . Furthermore, this ESD actor in Alheim possesses a high centrality value according to quantitative SNA.
Network Map of a central politician in Alheim, anonymized, source: Author’s data
As Fig. 5 shows, the interviewee mainly distinguishes five areas of activity: civil society, educational institutions, government/administration, business, and persons from trans-regional contexts. In some cases, the politician just wrote down an organization. During the interview, he referred to concrete persons from these organizations. Surprisingly, the sector of government/administration, to which the interviewee himself belongs, is empty: no persons or organizations are indicated. This is also reflected in the visualization based on quantitative network data (Fig. 4 ), where only one individual from government plays a central role. In a sense, qualitative studies validate quantitative results by showing that the social innovation ESD in Alheim is mainly implemented by actors from non-formal education. At the same time, qualitative results stress that the topic is supported and disseminated by one central politician who bridges structural holes between different sectors. Furthermore, school actors are not represented in the network map, whereas the closest contact persons are from civil society, educational institutions, and business. The great variety of close contact persons from different sectors can be regarded as one reason for the success of the social innovation in Alheim. The central politician in Alheim himself mentions this as playing a significant role. Further actors within the community stress that the ideological foundation and the adoption of ESD would not be possible without this politician. Hence, the establishment of ESD in Alheim can also be traced back to its structural and discursive power and the general trust of ESD actors in this well-connected politician.
The central role of the interviewee in Alheim can be ascribed to the fact that he bridges institutional clusters, supports cooperation beyond government/administration, and combines close cooperation with weak ties in the field of ESD. Furthermore, centrality is not reduced to one person or one sector. Instead, actors from different sectors play a central role in the field of ESD and cooperation between state and non-state actors is very high. In this way, it was possible to develop and realize aims in the area of political accountability in a short space of time. The dense network structure, supported by strong relations between one central politician and actors from other sectors, resulted in the elaboration of an innovative educational plan, composed according to the principles of ESD. At the same time, future strategies should focus on integrating actors from other important areas such as schools. In addition, strategies that foster trans-regional cooperation would be helpful with the diffusion of ESD.
With respect to some of the municipalities, a future strategy that fosters greater participation of stakeholders from other areas of activity, as required by the UN’s International Implementation Scheme (IIS) and the National Action Plan of the UN Decade may be helpful in promoting the implementation and diffusion of the social innovation ESD. Business actors and teachers, in particular, complain about not being sufficiently integrated into ESD networks and that the same people always take control and create turf wars. Furthermore, a lack of transparency and information exchange on existing ESD projects was seen. Business actors in these municipalities faced biases from other actors concerned that they ignored ecological and social dimensions of sustainable development. In some municipalities, ESD is mainly concentrated on environmental topics and many ESD actors express reservations about business aims. However, if different sectors are not integrated, it’s difficult to achieve a balance between ecological, economic, and social dimensions, as it has been proclaimed by the concept of sustainable development as such.
This article has explored the role of Social Network Analysis in analyzing and supporting innovation processes. In order to better understand the opportunities of SNA in innovation research, the author presented empirical results of her own quantitative and qualitative research on innovation networks in five German municipalities actively engaged in the field of ESD. The article showed the value of using a combination of both quantitative and qualitative SNA in order to better understand how and why social innovations are implemented and the opportunities to further develop the network.
Quantitative SNA was implemented to analyze the impact of structural characteristics of social networks on the implementation and the diffusion of the social innovation of ESD. It was discovered, for example, that cooperation in the field of ESD mainly takes place within municipalities and that cooperation beyond municipal borders is low and marked by structural holes. Furthermore, it was shown that social networks in the area of ESD are mostly composed of small and dense groups each representing different sets of actors (e.g., local administration, educational institutions, and business) and pursuing different interests and ideas under the umbrella of ESD. Weak ties, on the contrary, are very important in the field of ESD as they are responsible for the diffusion of innovations.
However, structural holes also exist within the municipalities with respect to the quality of the relations. The extreme example of Erfurt illustrated how the development of new ideas can be hampered by structural weaknesses. In contrast, cooperation and innovation development in the field of ESD are regarded to work well in Gelsenkirchen. In Alheim, actors from different sectors are integrated. Most central roles are played by non-formal education actors, whereas one central role is wielded by a politician. In terms of innovation diffusion, Alheim can be regarded as a best practice. Not least, cooperation beyond institutional borders works well and individual clustering values are low: persons are not always connected to the same clusters. Finally, the implementation of ESD in Alheim benefits from strong relations between one well-connected political and actors from other sectors. The central politician connects different areas of activity and promotes the integration of ecological, economic, and social dimensions in terms of sustainable development.
Quantitative techniques of SNA enabled to identify innovation networks, to determine network boundaries, to define actors within the innovation network, and to investigate the network position of actors. Problems of coordination, information, and qualitative relations were discussed. At the same time, quantitative SNA was shown unable to analyze reasons, motivations, and perceptions behind network structure. These issues were then analyzed by using qualitative SNA methods, such as network maps. A combination of qualitative and quantitative SNA techniques may thus prove the most fruitful for innovation research. In order to better understand the role of social networks in the diffusion of social innovations and to generate knowledge related to innovation potential and courses of action, qualitative techniques were used to supplement the quantitative analysis. It was assumed that the costs of information exchange are not only material (money, time), but also social. Conflicts and lack of confidence between actors, for example, may prevent successful innovation diffusion. Qualitative egocentric network maps could validate quantitative results as well as disclose subjective perceptions and orientations. The central position of one politician in Alheim could thus be traced back to its discursive and structural power. Actors in Alheim have great trust in the ideological competences of the well-connected person who supports the establishment of ESD in many sectors. Visualizations with qualitative network maps support the completion of the interview situation with visual representations. Visualized networks can also serve as mental or cognitive assistance. In combination with quantitative results, however, qualitative network maps enable us to detect where and how innovations and development processes may be possible due to structural and subjective conditions. Finally, compared to conventional statistical analysis that treat structural and individual levels as separately, analyses and visualizations of network data give us more information about the influence of social relations. SNA enables us to capture the interaction between actors and social context, to better understand how innovations are implemented and diffused, to analyze how and why social or educational change takes place or does not take place, and to disclose opportunities for future strategies.
This contribution has shown that SNA can begin to answer questions related to innovation processes. I hope it will open new avenues for further uses of SNA in innovation and futures research.
At the same time, there is little research on the democratic implications of network governance [ 5 ] as well as on the strengths and limits of the concept related to issues of educational innovations such as Education for Sustainable Development (ESD).
When examined through the framework of Social Network Analysis (SNA), the deficits of the concept of ‘Educational Governance’ become evident. In the scientific literature and in educational and political praxis, the concept of Educational Governance is often exclusively related to institutions of formal learning such as schools or educational training. In this manner, it is not possible to capture the real boundaries of social networks and to conceptualize social networks as can be done with SNA techniques. Furthermore, many actors, initiatives, and activities that play an important role in learning processes are analytically excluded in current applications of Educational Governance. For that reason, this article does not use an Educational Governance approach. Instead, it uses a governance approach that draws on theoretical concepts developed in social science.
Qualitative network maps were gathered in cooperation with a research project coordinated by Inka Bormann.
Wasserman S, Faust K (2009) Social network analysis: Methods and applications. Cambridge University Press, Cambridge
Google Scholar
Wald A, Jansen D (2007) Netzwerke. In: Benz A, Lütz S, Schimank U, Simonis G (eds) Handbuch Governance. Theoretische Grundlagen und empirische Anwendungsbereich. VS Verlag für Sozialwissenschaften, Wiesbaden, pp 93–106
Zafonte M, Sabatier P (1998) Shared beliefs and imposed interdependencies as determinants of ally networks in overlapping subsystems. J Theo Pol 10(4):473–505
Article Google Scholar
Hajer M (2009) Authoritative Governance: Policy-making in the Age of Mediatization. Oxford University Press, Oxford
Book Google Scholar
Sørensen E, Torfing J (2005) Network governance and post-liberal democracy. Admin Theory Praxis J 27(2):197–237
Rogers EM (2003) Diffusion of innovations, 5th edn. Free Press, New York
Zapf W (1989) Über soziale innovationen. Soziale Welt 40(1/2):170–183
Straus F (2002) Netzwerkanalysen: gemeindepsychologische Perspektiven füür Forschung und Praxis. DUV, Wiesbaden
Wolf C (2010) Egozentrierte Netzwerke: Datenerhebung und Datenanalyse. In: Stegbauer C, Häußling R (eds) Handbuch Netzwerkforschung. VS Verlag für Sozialwissenschaften, Wiesbaden, pp 471–483
Chapter Google Scholar
Kolleck N (forthcoming) Qualität, Netzwerke und Vertrauen - Der Einsatz von Netzwerkanalysen in Qualitätsentwicklungsprozessen. Zeitschrift für Erziehungswissenschaft
Fischer C (1982) To Dwell among friends. Personal networks in Town and City. The University Press of Chicago, Chicago
Burt R (1982) Toward a structural theory of action: Network models of a social structure, perceptions and action. Academic, New York
Hennig M (2006) Individuen und ihre sozialen Beziehungen. VS Verlag für Sozialwissenschaften, Wiesbaden
Freeman LC (2000) Visualizing social networks. J Soc Struct 1 1. http://www.cmu.edu/joss/content/articles/volume1/Freeman.html . Accessed 09 July 2013
Krempel L (2005) Visualisierung komplexer Strukturen: Grundlagen der Darstellung mehrdimensionaler Netzwerke. Campus Verlag, Frankfurt
Castells M (2009) The rise of the network society: The information age: Economy, society, and culture, 2nd edn. Oxford, Wiley-Blackwell
Granovetter MS (1973) The strength of weak ties. Am J Soc 78(6):1360–1380
Granovetter MS (1985) Economic action and social structure: the problem of Embeddedness. Am J Soc 91(3):481–510
Burt R (1992) Structural holes. Harvard University Press, Cambridge
Burt R (2004) Structural holes and good ideas. Am J Soc 110(2):349–399
Podolny JM, Baron JN (1997) Resources and relationships: social networks and mobility in the workplace. Am Soc Rev 62(5):673–693
Freeman LC (1979) Centrality in social networks: conceptual clarification. Soc Net 1(3):215–239
Watts DJ, Strogatz SH (1998) Collective dynamics of ‘small-world’ networks. Nature 393(6684):440–442
Hollstein B, Pfeffer J (2010) Netzwerkkarten als instrument zur Erhebung egozentierter Netzwerke. http://www.wiso.uni-hamburg.de/fileadmin/sozialoekonomie/hollstein/Team/Hollstein_Betina/Literatur_Betina/Netzwerkkarten_Hollstein_Pfeffer_2010.pdf . Accessed 09 July 2013
Kahn RL, Antonucci TC (1980) Convoys over the life course. Attachment, roles, and social support. In: Baltes PB, Brim OG (eds) Life pan development and behaviour. Academic, New York, pp 253–286
Download references
Author information
Authors and affiliations.
Universität Heidelberg & Hertie School of Governance, Friedrichstraße 180, 10117, Berlin, Germany
Nina Kolleck
You can also search for this author in PubMed Google Scholar
Corresponding author
Correspondence to Nina Kolleck .
Additional information
I thank the editors and two anonymous reviewers for their constructive comments, which helped me to improve the article. The article is based on results of a study I conducted at the Freie Universität Berlin. I would also like to thank Gerhard de Haan for useful information and for supporting my research.
Rights and permissions
This article is published under license to BioMed Central Ltd. Open Access This article is distributed under the terms of the Creative Commons Attribution License which permits any use, distribution, and reproduction in any medium, provided the original author(s) and the source are credited.
Reprints and permissions
About this article
Cite this article.
Kolleck, N. Social network analysis in innovation research: using a mixed methods approach to analyze social innovations. Eur J Futures Res 1 , 25 (2013). https://doi.org/10.1007/s40309-013-0025-2
Download citation
Received : 29 July 2013
Accepted : 24 September 2013
Published : 20 October 2013
DOI : https://doi.org/10.1007/s40309-013-0025-2
Share this article
Anyone you share the following link with will be able to read this content:
Sorry, a shareable link is not currently available for this article.
Provided by the Springer Nature SharedIt content-sharing initiative
- Social network analysis
- Social innovations
- Education for sustainable development
- Egocentric network maps
Thank you for visiting nature.com. You are using a browser version with limited support for CSS. To obtain the best experience, we recommend you use a more up to date browser (or turn off compatibility mode in Internet Explorer). In the meantime, to ensure continued support, we are displaying the site without styles and JavaScript.
- View all journals
- My Account Login
- Explore content
- About the journal
- Publish with us
- Sign up for alerts
- Open access
- Published: 02 May 2024
Effectiveness of social media-assisted course on learning self-efficacy
- Jiaying Hu 1 ,
- Yicheng Lai 2 &
- Xiuhua Yi 3
Scientific Reports volume 14 , Article number: 10112 ( 2024 ) Cite this article
440 Accesses
1 Altmetric
Metrics details
- Human behaviour
The social media platform and the information dissemination revolution have changed the thinking, needs, and methods of students, bringing development opportunities and challenges to higher education. This paper introduces social media into the classroom and uses quantitative analysis to investigate the relation between design college students’ learning self-efficacy and social media for design students, aiming to determine the effectiveness of social media platforms on self-efficacy. This study is conducted on university students in design media courses and is quasi-experimental, using a randomized pre-test and post-test control group design. The study participants are 73 second-year design undergraduates. Independent samples t-tests showed that the network interaction factors of social media had a significant impact on college students learning self-efficacy. The use of social media has a significant positive predictive effect on all dimensions of learning self-efficacy. Our analysis suggests that using the advantages and value of online social platforms, weakening the disadvantages of the network, scientifically using online learning resources, and combining traditional classrooms with the Internet can improve students' learning self-efficacy.
Similar content being viewed by others
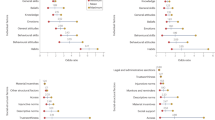
Determinants of behaviour and their efficacy as targets of behavioural change interventions
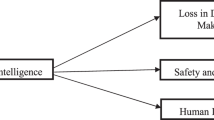
Impact of artificial intelligence on human loss in decision making, laziness and safety in education
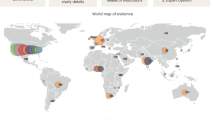
Toolbox of individual-level interventions against online misinformation
Introduction.
Social media is a way of sharing information, ideas, and opinions with others one. It can be used to create relationships between people and businesses. Social media has changed the communication way, it’s no longer just about talking face to face but also using a digital platform such as Facebook or Twitter. Today, social media is becoming increasingly popular in everyone's lives, including students and researchers 1 . Social media provides many opportunities for learners to publish their work globally, bringing many benefits to teaching and learning. The publication of students' work online has led to a more positive attitude towards learning and increased achievement and motivation. Other studies report that student online publications or work promote reflection on personal growth and development and provide opportunities for students to imagine more clearly the purpose of their work 2 . In addition, learning environments that include student publications allow students to examine issues differently, create new connections, and ultimately form new entities that can be shared globally 3 , 4 .
Learning self-efficacy is a belief that you can learn something new. It comes from the Latin word “self” and “efficax” which means efficient or effective. Self-efficacy is based on your beliefs about yourself, how capable you are to learn something new, and your ability to use what you have learned in real-life situations. This concept was first introduced by Bandura (1977), who studied the effects of social reinforcement on children’s learning behavior. He found that when children were rewarded for their efforts they would persist longer at tasks that they did not like or had low interest in doing. Social media, a ubiquitous force in today's digital age, has revolutionized the way people interact and share information. With the rise of social media platforms, individuals now have access to a wealth of online resources that can enhance their learning capabilities. This access to information and communication has also reshaped the way students approach their studies, potentially impacting their learning self-efficacy. Understanding the role of social media in shaping students' learning self-efficacy is crucial in providing effective educational strategies that promote healthy learning and development 5 . Unfortunately, the learning curve for the associated metadata base modeling methodologies and their corresponding computer-aided software engineering (CASE) tools have made it difficult for students to grasp. Addressing this learning issue examined the effect of this MLS on the self-efficacy of learning these topics 6 . Bates et al. 7 hypothesize a mediated model in which a set of antecedent variables influenced students’ online learning self-efficacy which, in turn, affected student outcome expectations, mastery perceptions, and the hours spent per week using online learning technology to complete learning assignments for university courses. Shen et al. 8 through exploratory factor analysis identifies five dimensions of online learning self-efficacy: (a) self-efficacy to complete an online course (b) self-efficacy to interact socially with classmates (c) self-efficacy to handle tools in a Course Management System (CMS) (d) self-efficacy to interact with instructors in an online course, and (e) self-efficacy to interact with classmates for academic purposes. Chiu 9 established a model for analyzing the mediating effect that learning self-efficacy and social self-efficacy have on the relationship between university students’ perceived life stress and smartphone addiction. Kim et al. 10 study was conducted to examine the influence of learning efficacy on nursing students' self-confidence. The objective of Paciello et al. 11 was to identify self-efficacy configurations in different domains (i.e., emotional, social, and self-regulated learning) in a sample of university students using a person-centered approach. The role of university students’ various conceptions of learning in their academic self-efficacy in the domain of physics is initially explored 12 . Kumar et al. 13 investigated factors predicting students’ behavioral intentions towards the continuous use of mobile learning. Other influential work includes 14 .
Many studies have focused on social networking tools such as Facebook and MySpace 15 , 16 . Teachers are concerned that the setup and use of social media apps take up too much of their time, may have plagiarism and privacy issues, and contribute little to actual student learning outcomes; they often consider them redundant or simply not conducive to better learning outcomes 17 . Cao et al. 18 proposed that the central questions in addressing the positive and negative pitfalls of social media on teaching and learning are whether the use of social media in teaching and learning enhances educational effectiveness, and what motivates university teachers to use social media in teaching and learning. Maloney et al. 3 argued that social media can further improve the higher education teaching and learning environment, where students no longer access social media to access course information. Many studies in the past have shown that the use of modern IT in the classroom has increased over the past few years; however, it is still limited mainly to content-driven use, such as accessing course materials, so with the emergence of social media in students’ everyday lives 2 , we need to focus on developing students’ learning self-efficacy so that they can This will enable students to 'turn the tables and learn to learn on their own. Learning self-efficacy is considered an important concept that has a powerful impact on learning outcomes 19 , 20 .
Self-efficacy for learning is vital in teaching students to learn and develop healthily and increasing students' beliefs in the learning process 21 . However, previous studies on social media platforms such as Twitter and Weibo as curriculum support tools have not been further substantiated or analyzed in detail. In addition, the relationship between social media, higher education, and learning self-efficacy has not yet been fully explored by researchers in China. Our research aims to fill this gap in the topic. Our study explored the impact of social media on the learning self-efficacy of Chinese college students. Therefore, it is essential to explore the impact of teachers' use of social media to support teaching and learning on students' learning self-efficacy. Based on educational theory and methodological practice, this study designed a teaching experiment using social media to promote learning self-efficacy by posting an assignment for post-course work on online media to explore the actual impact of social media on university students’ learning self-efficacy. This study examines the impact of a social media-assisted course on university students' learning self-efficacy to explore the positive impact of a social media-assisted course.
Theoretical background
- Social media
Social media has different definitions. Mayfield (2013) first introduced the concept of social media in his book-what is social media? The author summarized the six characteristics of social media: openness, participation, dialogue, communication, interaction, and communication. Mayfield 22 shows that social media is a kind of new media. Its uniqueness is that it can give users great space and freedom to participate in the communication process. Jen (2020) also suggested that the distinguishing feature of social media is that it is “aggregated”. Social media provides users with an interactive service to control their data and information and collaborate and share information 2 . Social media offers opportunities for students to build knowledge and helps them actively create and share information 23 . Millennial students are entering higher education institutions and are accustomed to accessing and using data from the Internet. These individuals go online daily for educational or recreational purposes. Social media is becoming increasingly popular in the lives of everyone, including students and researchers 1 . A previous study has shown that millennials use the Internet as their first source of information and Google as their first choice for finding educational and personal information 24 . Similarly, many institutions encourage teachers to adopt social media applications 25 . Faculty members have also embraced social media applications for personal, professional, and pedagogical purposes 17 .
Social networks allow one to create a personal profile and build various networks that connect him/her to family, friends, and other colleagues. Users use these sites to stay in touch with their friends, make plans, make new friends, or connect with someone online. Therefore, extending this concept, these sites can establish academic connections or promote cooperation and collaboration in higher education classrooms 2 . This study defines social media as an interactive community of users' information sharing and social activities built on the technology of the Internet. Because the concept of social media is broad, its connotations are consistent. Research shows that Meaning and Linking are the two key elements that make up social media existence. Users and individual media outlets generate social media content and use it as a platform to get it out there. Social media distribution is based on social relationships and has a better platform for personal information and relationship management systems. Examples of social media applications include Facebook, Twitter, MySpace, YouTube, Flickr, Skype, Wiki, blogs, Delicious, Second Life, open online course sites, SMS, online games, mobile applications, and more 18 . Ajjan and Hartshorne 2 investigated the intentions of 136 faculty members at a US university to adopt Web 2.0 technologies as tools in their courses. They found that integrating Web 2.0 technologies into the classroom learning environment effectively increased student satisfaction with the course and improved their learning and writing skills. His research focused on improving the perceived usefulness, ease of use, compatibility of Web 2.0 applications, and instructor self-efficacy. The social computing impact of formal education and training and informal learning communities suggested that learning web 2.0 helps users to acquire critical competencies, and promotes technological, pedagogical, and organizational innovation, arguing that social media has a variety of learning content 26 . Users can post digital content online, enabling learners to tap into tacit knowledge while supporting collaboration between learners and teachers. Cao and Hong 27 investigated the antecedents and consequences of social media use in teaching among 249 full-time and part-time faculty members, who reported that the factors for using social media in teaching included personal social media engagement and readiness, external pressures; expected benefits; and perceived risks. The types of Innovators, Early adopters, Early majority, Late majority, Laggards, and objectors. Cao et al. 18 studied the educational effectiveness of 168 teachers' use of social media in university teaching. Their findings suggest that social media use has a positive impact on student learning outcomes and satisfaction. Their research model provides educators with ideas on using social media in the education classroom to improve student performance. Maqableh et al. 28 investigated the use of social networking sites by 366 undergraduate students, and they found that weekly use of social networking sites had a significant impact on student's academic performance and that using social networking sites had a significant impact on improving students' effective time management, and awareness of multitasking. All of the above studies indicate the researcher’s research on social media aids in teaching and learning. All of these studies indicate the positive impact of social media on teaching and learning.
- Learning self-efficacy
For the definition of concepts related to learning self-efficacy, scholars have mainly drawn on the idea proposed by Bandura 29 that defines self-efficacy as “the degree to which people feel confident in their ability to use the skills they possess to perform a task”. Self-efficacy is an assessment of a learner’s confidence in his or her ability to use the skills he or she possesses to complete a learning task and is a subjective judgment and feeling about the individual’s ability to control his or her learning behavior and performance 30 . Liu 31 has defined self-efficacy as the belief’s individuals hold about their motivation to act, cognitive ability, and ability to perform to achieve their goals, showing the individual's evaluation and judgment of their abilities. Zhang (2015) showed that learning efficacy is regarded as the degree of belief and confidence that expresses the success of learning. Yan 32 showed the extent to which learning self-efficacy is viewed as an individual. Pan 33 suggested that learning self-efficacy in an online learning environment is a belief that reflects the learner's ability to succeed in the online learning process. Kang 34 believed that learning self-efficacy is the learner's confidence and belief in his or her ability to complete a learning task. Huang 35 considered self-efficacy as an individual’s self-assessment of his or her ability to complete a particular task or perform a specific behavior and the degree of confidence in one’s ability to achieve a specific goal. Kong 36 defined learning self-efficacy as an individual’s judgment of one’s ability to complete academic tasks.
Based on the above analysis, we found that scholars' focus on learning self-efficacy is on learning behavioral efficacy and learning ability efficacy, so this study divides learning self-efficacy into learning behavioral efficacy and learning ability efficacy for further analysis and research 37 , 38 . Search the CNKI database and ProQuest Dissertations for keywords such as “design students’ learning self-efficacy”, “design classroom self-efficacy”, “design learning self-efficacy”, and other keywords. There are few relevant pieces of literature about design majors. Qiu 39 showed that mobile learning-assisted classroom teaching can control the source of self-efficacy from many aspects, thereby improving students’ sense of learning efficacy and helping middle and lower-level students improve their sense of learning efficacy from all dimensions. Yin and Xu 40 argued that the three elements of the network environment—“learning content”, “learning support”, and “social structure of learning”—all have an impact on university students’ learning self-efficacy. Duo et al. 41 recommend that learning activities based on the mobile network learning community increase the trust between students and the sense of belonging in the learning community, promote mutual communication and collaboration between students, and encourage each other to stimulate their learning motivation. In the context of social media applications, self-efficacy refers to the level of confidence that teachers can successfully use social media applications in the classroom 18 . Researchers have found that self-efficacy is related to social media applications 42 . Students had positive experiences with social media applications through content enhancement, creativity experiences, connectivity enrichment, and collaborative engagement 26 . Students who wish to communicate with their tutors in real-time find social media tools such as web pages, blogs, and virtual interactions very satisfying 27 . Overall, students report their enjoyment of different learning processes through social media applications; simultaneously, they show satisfactory tangible achievement of tangible learning outcomes 18 . According to Bandura's 'triadic interaction theory’, Bian 43 and Shi 44 divided learning self-efficacy into two main elements, basic competence, and control, where basic competence includes the individual's sense of effort, competence, the individual sense of the environment, and the individual's sense of control over behavior. The primary sense of competence includes the individual's Sense of effort, competence, environment, and control over behavior. In this study, learning self-efficacy is divided into Learning behavioral efficacy and Learning ability efficacy. Learning behavioral efficacy includes individuals' sense of effort, environment, and control; learning ability efficacy includes individuals' sense of ability, belief, and interest.
In Fig. 1 , learning self-efficacy includes learning behavior efficacy and learning ability efficacy, in which the learning behavior efficacy is determined by the sense of effort, the sense of environment, the sense of control, and the learning ability efficacy is determined by the sense of ability, sense of belief, sense of interest. “Sense of effort” is the understanding of whether one can study hard. Self-efficacy includes the estimation of self-effort and the ability, adaptability, and creativity shown in a particular situation. One with a strong sense of learning self-efficacy thinks they can study hard and focus on tasks 44 . “Sense of environment” refers to the individual’s feeling of their learning environment and grasp of the environment. The individual is the creator of the environment. A person’s feeling and grasp of the environment reflect the strength of his sense of efficacy to some extent. A person with a shared sense of learning self-efficacy is often dissatisfied with his environment, but he cannot do anything about it. He thinks the environment can only dominate him. A person with a high sense of learning self-efficacy will be more satisfied with his school and think that his teachers like him and are willing to study in school 44 . “Sense of control” is an individual’s sense of control over learning activities and learning behavior. It includes the arrangement of individual learning time, whether they can control themselves from external interference, and so on. A person with a strong sense of self-efficacy will feel that he is the master of action and can control the behavior and results of learning. Such a person actively participates in various learning activities. When he encounters difficulties in learning, he thinks he can find a way to solve them, is not easy to be disturbed by the outside world, and can arrange his own learning time. The opposite is the sense of losing control of learning behavior 44 . “Sense of ability” includes an individual’s perception of their natural abilities, expectations of learning outcomes, and perception of achieving their learning goals. A person with a high sense of learning self-efficacy will believe that he or she is brighter and more capable in all areas of learning; that he or she is more confident in learning in all subjects. In contrast, people with low learning self-efficacy have a sense of powerlessness. They are self-doubters who often feel overwhelmed by their learning and are less confident that they can achieve the appropriate learning goals 44 . “Sense of belief” is when an individual knows why he or she is doing something, knows where he or she is going to learn, and does not think before he or she even does it: What if I fail? These are meaningless, useless questions. A person with a high sense of learning self-efficacy is more robust, less afraid of difficulties, and more likely to reach their learning goals. A person with a shared sense of learning self-efficacy, on the other hand, is always going with the flow and is uncertain about the outcome of their learning, causing them to fall behind. “Sense of interest” is a person's tendency to recognize and study the psychological characteristics of acquiring specific knowledge. It is an internal force that can promote people's knowledge and learning. It refers to a person's positive cognitive tendency and emotional state of learning. A person with a high sense of self-efficacy in learning will continue to concentrate on studying and studying, thereby improving learning. However, one with low learning self-efficacy will have psychology such as not being proactive about learning, lacking passion for learning, and being impatient with learning. The elements of learning self-efficacy can be quantified and detailed in the following Fig. 1 .
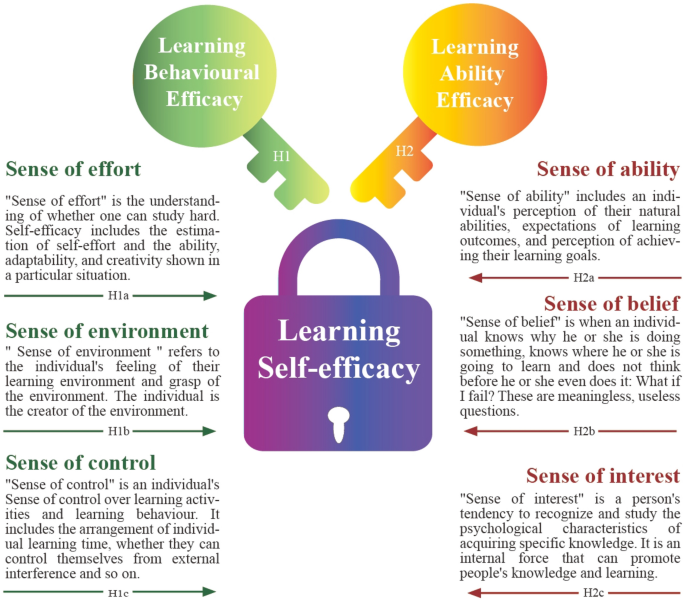
Learning self-efficacy research structure in this paper.
Research participants
All the procedures were conducted in adherence to the guidelines and regulations set by the institution. Prior to initiating the study, informed consent was obtained in writing from the participants, and the Institutional Review Board for Behavioral and Human Movement Sciences at Nanning Normal University granted approval for all protocols.
Two parallel classes are pre-selected as experimental subjects in our study, one as the experimental group and one as the control group. Social media assisted classroom teaching to intervene in the experimental group, while the control group did not intervene. When selecting the sample, it is essential to consider, as far as possible, the shortcomings of not using randomization to select or assign the study participants, resulting in unequal experimental and control groups. When selecting the experimental subjects, classes with no significant differences in initial status and external conditions, i.e. groups with homogeneity, should be selected. Our study finally decided to select a total of 44 students from Class 2021 Design 1 and a total of 29 students from Class 2021 Design 2, a total of 74 students from Nanning Normal University, as the experimental subjects. The former served as the experimental group, and the latter served as the control group. 73 questionnaires are distributed to measure before the experiment, and 68 are returned, with a return rate of 93.15%. According to the statistics, there were 8 male students and 34 female students in the experimental group, making a total of 44 students (mirrors the demographic trends within the humanities and arts disciplines from which our sample was drawn); there are 10 male students and 16 female students in the control group, making a total of 26 students, making a total of 68 students in both groups. The sample of those who took the course were mainly sophomores, with a small number of first-year students and juniors, which may be related to the nature of the subject of this course and the course system offered by the university. From the analysis of students' majors, liberal arts students in the experimental group accounted for the majority, science students and art students accounted for a small part. In contrast, the control group had more art students, and liberal arts students and science students were small. In the daily self-study time, the experimental and control groups are 2–3 h. The demographic information of research participants is shown in Table 1 .
Research procedure
Firstly, the ADDIE model is used for the innovative design of the teaching method of the course. The number of students in the experimental group was 44, 8 male and 35 females; the number of students in the control group was 29, 10 male and 19 females. Secondly, the classes are targeted at students and applied. Thirdly, the course for both the experimental and control classes is a convenient and practice-oriented course, with the course title “Graphic Design and Production”, which focuses on learning the graphic design software Photoshop. The course uses different cases to explain in detail the process and techniques used to produce these cases using Photoshop, and incorporates practical experience as well as relevant knowledge in the process, striving to achieve precise and accurate operational steps; at the end of the class, the teacher assigns online assignments to be completed on social media, allowing students to post their edited software tutorials online so that students can master the software functions. The teacher assigns online assignments to be completed on social media at the end of the lesson, allowing students to post their editing software tutorials online so that they can master the software functions and production skills, inspire design inspiration, develop design ideas and improve their design skills, and improve students' learning self-efficacy through group collaboration and online interaction. Fourthly, pre-tests and post-tests are conducted in the experimental and control classes before the experiment. Fifthly, experimental data are collected, analyzed, and summarized.
We use a questionnaire survey to collect data. Self-efficacy is a person’s subjective judgment on whether one can successfully perform a particular achievement. American psychologist Albert Bandura first proposed it. To understand the improvement effect of students’ self-efficacy after the experimental intervention, this work questionnaire was referenced by the author from “Self-efficacy” “General Perceived Self Efficacy Scale” (General Perceived Self Efficacy Scale) German psychologist Schwarzer and Jerusalem (1995) and “Academic Self-Efficacy Questionnaire”, a well-known Chinese scholar Liang 45 . The questionnaire content is detailed in the supplementary information . A pre-survey of the questionnaire is conducted here. The second-year students of design majors collected 32 questionnaires, eliminated similar questions based on the data, and compiled them into a formal survey scale. The scale consists of 54 items, 4 questions about basic personal information, and 50 questions about learning self-efficacy. The Likert five-point scale is the questionnaire used in this study. The answers are divided into “completely inconsistent", “relatively inconsistent”, “unsure”, and “relatively consistent”. The five options of “Completely Meet” and “Compliant” will count as 1, 2, 3, 4, and 5 points, respectively. Divided into a sense of ability (Q5–Q14), a sense of effort (Q15–Q20), a sense of environment (Q21–Q28), a sense of control (Q29–Q36), a sense of Interest (Q37–Q45), a sense of belief (Q46–Q54). To demonstrate the scientific effectiveness of the experiment, and to further control the influence of confounding factors on the experimental intervention. This article thus sets up a control group as a reference. Through the pre-test and post-test in different periods, comparison of experimental data through pre-and post-tests to illustrate the effects of the intervention.
Reliability indicates the consistency of the results of a measurement scale (See Table 2 ). It consists of intrinsic and extrinsic reliability, of which intrinsic reliability is essential. Using an internal consistency reliability test scale, a Cronbach's alpha coefficient of reliability statistics greater than or equal to 0.9 indicates that the scale has good reliability, 0.8–0.9 indicates good reliability, 7–0.8 items are acceptable. Less than 0.7 means to discard some items in the scale 46 . This study conducted a reliability analysis on the effects of the related 6-dimensional pre-test survey to illustrate the reliability of the questionnaire.
From the Table 2 , the Cronbach alpha coefficients for the pre-test, sense of effort, sense of environment, sense of control, sense of interest, sense of belief, and the total questionnaire, were 0.919, 0.839, 0.848, 0.865, 0.852, 0.889 and 0.958 respectively. The post-test Cronbach alpha coefficients were 0.898, 0.888, 0.886, 0.889, 0.900, 0.893 and 0.970 respectively. The Cronbach alpha coefficients were all greater than 0.8, indicating a high degree of reliability of the measurement data.
The validity, also known as accuracy, reflects how close the measurement result is to the “true value”. Validity includes structure validity, content validity, convergent validity, and discriminative validity. Because the experiment is a small sample study, we cannot do any specific factorization. KMO and Bartlett sphericity test values are an important part of structural validity. Indicator, general validity evaluation (KMO value above 0.9, indicating very good validity; 0.8–0.9, indicating good validity; 0.7–0.8 validity is good; 0.6–0.7 validity is acceptable; 0.5–0.6 means poor validity; below 0.45 means that some items should be abandoned.
Table 3 shows that the KMO values of ability, effort, environment, control, interest, belief, and the total questionnaire are 0.911, 0.812, 0.778, 0.825, 0.779, 0.850, 0.613, and the KMO values of the post-test are respectively. The KMO values are 0.887, 0.775, 0.892, 0.868, 0.862, 0.883, 0.715. KMO values are basically above 0.8, and all are greater than 0.6. This result indicates that the validity is acceptable, the scale has a high degree of reasonableness, and the valid data.
In the graphic design and production (professional design course), we will learn the practical software with cases. After class, we will share knowledge on the self-media platform. We will give face-to-face computer instruction offline from 8:00 to 11:20 every Wednesday morning for 16 weeks. China's top online sharing platform (APP) is Tik Tok, micro-blog (Micro Blog) and Xiao hong shu. The experiment began on September 1, 2022, and conducted the pre-questionnaire survey simultaneously. At the end of the course, on January 6, 2023, the post questionnaire survey was conducted. A total of 74 questionnaires were distributed in this study, recovered 74 questionnaires. After excluding the invalid questionnaires with incomplete filling and wrong answers, 68 valid questionnaires were obtained, with an effective rate of 91%, meeting the test requirements. Then, use the social science analysis software SPSS Statistics 26 to analyze the data: (1) descriptive statistical analysis of the dimensions of learning self-efficacy; (2) Using correlation test to analyze the correlation between learning self-efficacy and the use of social media; (3) This study used a comparative analysis of group differences to detect the influence of learning self-efficacy on various dimensions of social media and design courses. For data processing and analysis, use the spss26 version software and frequency statistics to create statistics on the basic situation of the research object and the basic situation of the use of live broadcast. The reliability scale analysis (internal consistency test) and use Bartlett's sphericity test to illustrate the reliability and validity of the questionnaire and the individual differences between the control group and the experimental group in demographic variables (gender, grade, Major, self-study time per day) are explained by cross-analysis (chi-square test). In the experimental group and the control group, the pre-test, post-test, before-and-after test of the experimental group and the control group adopt independent sample T-test and paired sample T-test to illustrate the effect of the experimental intervention (The significance level of the test is 0.05 two-sided).
Results and discussion
Comparison of pre-test and post-test between groups.
To study whether the data of the experimental group and the control group are significantly different in the pre-test and post-test mean of sense of ability, sense of effort, sense of environment, sense of control, sense of interest, and sense of belief. The research for this situation uses an independent sample T-test and an independent sample. The test needs to meet some false parameters, such as normality requirements. Generally passing the normality test index requirements are relatively strict, so it can be relaxed to obey an approximately normal distribution. If there is serious skewness distribution, replace it with the nonparametric test. Variables are required to be continuous variables. The six variables in this study define continuous variables. The variable value information is independent of each other. Therefore, we use the independent sample T-test.
From the Table 4 , a pre-test found that there was no statistically significant difference between the experimental group and the control group at the 0.05 confidence level ( p > 0.05) for perceptions of sense of ability, sense of effort, sense of environment, sense of control, sense of interest, and sense of belief. Before the experiment, the two groups of test groups have the same quality in measuring self-efficacy. The experimental class and the control class are homogeneous groups. Table 5 shows the independent samples t-test for the post-test, used to compare the experimental and control groups on six items, including the sense of ability, sense of effort, sense of environment, sense of control, sense of interest, and sense of belief.
The experimental and control groups have statistically significant scores ( p < 0.05) for sense of ability, sense of effort, sense of environment, sense of control, sense of interest, and sense of belief, and the experimental and control groups have statistically significant scores (t = 3.177, p = 0.002) for a sense of competence. (t = 3.177, p = 0.002) at the 0.01 level, with the experimental group scoring significantly higher (3.91 ± 0.51) than the control group (3.43 ± 0.73). The experimental group and the control group showed significance for the perception of effort at the 0.01 confidence level (t = 2.911, p = 0.005), with the experimental group scoring significantly higher (3.88 ± 0.66) than the control group scoring significantly higher (3.31 ± 0.94). The experimental and control groups show significance at the 0.05 level (t = 2.451, p = 0.017) for the sense of environment, with the experimental group scoring significantly higher (3.95 ± 0.61) than the control group scoring significantly higher (3.58 ± 0.62). The experimental and control groups showed significance for sense of control at the 0.05 level of significance (t = 2.524, p = 0.014), and the score for the experimental group (3.76 ± 0.67) would be significantly higher than the score for the control group (3.31 ± 0.78). The experimental and control groups showed significance at the 0.01 level for sense of interest (t = 2.842, p = 0.006), and the experimental group's score (3.87 ± 0.61) would be significantly higher than the control group's score (3.39 ± 0.77). The experimental and control groups showed significance at the 0.01 level for the sense of belief (t = 3.377, p = 0.001), and the experimental group would have scored significantly higher (4.04 ± 0.52) than the control group (3.56 ± 0.65). Therefore, we can conclude that the experimental group's post-test significantly affects the mean scores of sense of ability, sense of effort, sense of environment, sense of control, sense of interest, and sense of belief. A social media-assisted course has a positive impact on students' self-efficacy.
Comparison of pre-test and post-test of each group
The paired-sample T-test is an extension of the single-sample T-test. The purpose is to explore whether the means of related (paired) groups are significantly different. There are four standard paired designs: (1) Before and after treatment of the same subject Data, (2) Data from two different parts of the same subject, (3) Test results of the same sample with two methods or instruments, 4. Two matched subjects receive two treatments, respectively. This study belongs to the first type, the 6 learning self-efficacy dimensions of the experimental group and the control group is measured before and after different periods.
Paired t-tests is used to analyze whether there is a significant improvement in the learning self-efficacy dimension in the experimental group after the experimental social media-assisted course intervention. In Table 6 , we can see that the six paired data groups showed significant differences ( p < 0.05) in the pre and post-tests of sense of ability, sense of effort, sense of environment, sense of control, sense of interest, and sense of belief. There is a level of significance of 0.01 (t = − 4.540, p = 0.000 < 0.05) before and after the sense of ability, the score after the sense of ability (3.91 ± 0.51), and the score before the Sense of ability (3.41 ± 0.55). The level of significance between the pre-test and post-test of sense of effort is 0.01 (t = − 4.002, p = 0.000). The score of the sense of effort post-test (3.88 ± 0.66) will be significantly higher than the average score of the sense of effort pre-test (3.31 ± 0.659). The significance level between the pre-test and post-test Sense of environment is 0.01 (t = − 3.897, p = 0.000). The average score for post- Sense of environment (3.95 ± 0.61) will be significantly higher than that of sense of environment—the average score of the previous test (3.47 ± 0.44). The average value of a post- sense of control (3.76 ± 0.67) will be significantly higher than the average of the front side of the Sense of control value (3.27 ± 0.52). The sense of interest pre-test and post-test showed a significance level of 0.01 (− 4.765, p = 0.000), and the average value of Sense of interest post-test was 3.87 ± 0.61. It would be significantly higher than the average value of the Sense of interest (3.25 ± 0.59), the significance between the pre-test and post-test of belief sensing is 0.01 level (t = − 3.939, p = 0.000). Thus, the average value of a post-sense of belief (4.04 ± 0.52) will be significantly higher than that of a pre-sense of belief Average value (3.58 ± 0.58). After the experimental group’s post-test, the scores for the Sense of ability, effort, environment, control, interest, and belief before the comparison experiment increased significantly. This result has a significant improvement effect. Table 7 shows that the control group did not show any differences in the pre and post-tests using paired t-tests on the dimensions of learning self-efficacy such as sense of ability, sense of effort, sense of environment, sense of control, sense of interest, and sense of belief ( p > 0.05). It shows no experimental intervention for the control group, and it does not produce a significant effect.
The purpose of this study aims to explore the impact of social media use on college students' learning self-efficacy, examine the changes in the elements of college students' learning self-efficacy before and after the experiment, and make an empirical study to enrich the theory. This study developed an innovative design for course teaching methods using the ADDIE model. The design process followed a series of model rules of analysis, design, development, implementation, and evaluation, as well as conducted a descriptive statistical analysis of the learning self-efficacy of design undergraduates. Using questionnaires and data analysis, the correlation between the various dimensions of learning self-efficacy is tested. We also examined the correlation between the two factors, and verifies whether there was a causal relationship between the two factors.
Based on prior research and the results of existing practice, a learning self-efficacy is developed for university students and tested its reliability and validity. The scale is used to pre-test the self-efficacy levels of the two subjects before the experiment, and a post-test of the self-efficacy of the two groups is conducted. By measuring and investigating the learning self-efficacy of the study participants before the experiment, this study determined that there was no significant difference between the experimental group and the control group in terms of sense of ability, sense of effort, sense of environment, sense of control, sense of interest, and sense of belief. Before the experiment, the two test groups had homogeneity in measuring the dimensionality of learning self-efficacy. During the experiment, this study intervened in social media assignments for the experimental group. The experiment used learning methods such as network assignments, mutual aid communication, mutual evaluation of assignments, and group discussions. After the experiment, the data analysis showed an increase in learning self-efficacy in the experimental group compared to the pre-test. With the test time increased, the learning self-efficacy level of the control group decreased slightly. It shows that social media can promote learning self-efficacy to a certain extent. This conclusion is similar to Cao et al. 18 , who suggested that social media would improve educational outcomes.
We have examined the differences between the experimental and control group post-tests on six items, including the sense of ability, sense of effort, sense of environment, sense of control, sense of interest, and sense of belief. This result proves that a social media-assisted course has a positive impact on students' learning self-efficacy. Compared with the control group, students in the experimental group had a higher interest in their major. They showed that they liked to share their learning experiences and solve difficulties in their studies after class. They had higher motivation and self-directed learning ability after class than students in the control group. In terms of a sense of environment, students in the experimental group were more willing to share their learning with others, speak boldly, and participate in the environment than students in the control group.
The experimental results of this study showed that the experimental group showed significant improvement in the learning self-efficacy dimensions after the experimental intervention in the social media-assisted classroom, with significant increases in the sense of ability, sense of effort, sense of environment, sense of control, sense of interest and sense of belief compared to the pre-experimental scores. This result had a significant improvement effect. Evidence that a social media-assisted course has a positive impact on students' learning self-efficacy. Most of the students recognized the impact of social media on their learning self-efficacy, such as encouragement from peers, help from teachers, attention from online friends, and recognition of their achievements, so that they can gain a sense of achievement that they do not have in the classroom, which stimulates their positive perception of learning and is more conducive to the awakening of positive effects. This phenomenon is in line with Ajjan and Hartshorne 2 . They argue that social media provides many opportunities for learners to publish their work globally, which brings many benefits to teaching and learning. The publication of students' works online led to similar positive attitudes towards learning and improved grades and motivation. This study also found that students in the experimental group in the post-test controlled their behavior, became more interested in learning, became more purposeful, had more faith in their learning abilities, and believed that their efforts would be rewarded. This result is also in line with Ajjan and Hartshorne's (2008) indication that integrating Web 2.0 technologies into classroom learning environments can effectively increase students' satisfaction with the course and improve their learning and writing skills.
We only selected students from one university to conduct a survey, and the survey subjects were self-selected. Therefore, the external validity and generalizability of our study may be limited. Despite the limitations, we believe this study has important implications for researchers and educators. The use of social media is the focus of many studies that aim to assess the impact and potential of social media in learning and teaching environments. We hope that this study will help lay the groundwork for future research on the outcomes of social media utilization. In addition, future research should further examine university support in encouraging teachers to begin using social media and university classrooms in supporting social media (supplementary file 1 ).
The present study has provided preliminary evidence on the positive association between social media integration in education and increased learning self-efficacy among college students. However, several avenues for future research can be identified to extend our understanding of this relationship.
Firstly, replication studies with larger and more diverse samples are needed to validate our findings across different educational contexts and cultural backgrounds. This would enhance the generalizability of our results and provide a more robust foundation for the use of social media in teaching. Secondly, longitudinal investigations should be conducted to explore the sustained effects of social media use on learning self-efficacy. Such studies would offer insights into how the observed benefits evolve over time and whether they lead to improved academic performance or other relevant outcomes. Furthermore, future research should consider the exploration of potential moderators such as individual differences in students' learning styles, prior social media experience, and psychological factors that may influence the effectiveness of social media in education. Additionally, as social media platforms continue to evolve rapidly, it is crucial to assess the impact of emerging features and trends on learning self-efficacy. This includes an examination of advanced tools like virtual reality, augmented reality, and artificial intelligence that are increasingly being integrated into social media environments. Lastly, there is a need for research exploring the development and evaluation of instructional models that effectively combine traditional teaching methods with innovative uses of social media. This could guide educators in designing courses that maximize the benefits of social media while minimizing potential drawbacks.
In conclusion, the current study marks an important step in recognizing the potential of social media as an educational tool. Through continued research, we can further unpack the mechanisms by which social media can enhance learning self-efficacy and inform the development of effective educational strategies in the digital age.
Data availability
The data that support the findings of this study are available from the corresponding authors upon reasonable request. The data are not publicly available due to privacy or ethical restrictions.
Rasheed, M. I. et al. Usage of social media, student engagement, and creativity: The role of knowledge sharing behavior and cyberbullying. Comput. Educ. 159 , 104002 (2020).
Article Google Scholar
Ajjan, H. & Hartshorne, R. Investigating faculty decisions to adopt Web 2.0 technologies: Theory and empirical tests. Internet High. Educ. 11 , 71–80 (2008).
Maloney, E. J. What web 2.0 can teach us about learning. The Chronicle of Higher Education 53 , B26–B27 (2007).
Ustun, A. B., Karaoglan-Yilmaz, F. G. & Yilmaz, R. Educational UTAUT-based virtual reality acceptance scale: A validity and reliability study. Virtual Real. 27 , 1063–1076 (2023).
Schunk, D. H. Self-efficacy and classroom learning. Psychol. Sch. 22 , 208–223 (1985).
Cheung, W., Li, E. Y. & Yee, L. W. Multimedia learning system and its effect on self-efficacy in database modeling and design: An exploratory study. Comput. Educ. 41 , 249–270 (2003).
Bates, R. & Khasawneh, S. Self-efficacy and college students’ perceptions and use of online learning systems. Comput. Hum. Behav. 23 , 175–191 (2007).
Shen, D., Cho, M.-H., Tsai, C.-L. & Marra, R. Unpacking online learning experiences: Online learning self-efficacy and learning satisfaction. Internet High. Educ. 19 , 10–17 (2013).
Chiu, S.-I. The relationship between life stress and smartphone addiction on taiwanese university student: A mediation model of learning self-efficacy and social self-Efficacy. Comput. Hum. Behav. 34 , 49–57 (2014).
Kim, S.-O. & Kang, B.-H. The influence of nursing students’ learning experience, recognition of importance and learning self-efficacy for core fundamental nursing skills on their self-confidence. J. Korea Acad.-Ind. Coop. Soc. 17 , 172–182 (2016).
Google Scholar
Paciello, M., Ghezzi, V., Tramontano, C., Barbaranelli, C. & Fida, R. Self-efficacy configurations and wellbeing in the academic context: A person-centred approach. Pers. Individ. Differ. 99 , 16–21 (2016).
Suprapto, N., Chang, T.-S. & Ku, C.-H. Conception of learning physics and self-efficacy among Indonesian University students. J. Balt. Sci. Educ. 16 , 7–19 (2017).
Kumar, J. A., Bervell, B., Annamalai, N. & Osman, S. Behavioral intention to use mobile learning: Evaluating the role of self-efficacy, subjective norm, and WhatsApp use habit. IEEE Access 8 , 208058–208074 (2020).
Fisk, J. E. & Warr, P. Age-related impairment in associative learning: The role of anxiety, arousal and learning self-efficacy. Pers. Indiv. Differ. 21 , 675–686 (1996).
Pence, H. E. Preparing for the real web generation. J. Educ. Technol. Syst. 35 , 347–356 (2007).
Hu, J., Lee, J. & Yi, X. Blended knowledge sharing model in design professional. Sci. Rep. 13 , 16326 (2023).
Article ADS CAS PubMed PubMed Central Google Scholar
Moran, M., Seaman, J. & Tintikane, H. Blogs, wikis, podcasts and Facebook: How today’s higher education faculty use social media, vol. 22, 1–28 (Pearson Learning Solutions. Retrieved December, 2012).
Cao, Y., Ajjan, H. & Hong, P. Using social media applications for educational outcomes in college teaching: A structural equation analysis: Social media use in teaching. Br. J. Educ. Technol. 44 , 581–593 (2013).
Artino, A. R. Academic self-efficacy: From educational theory to instructional practice. Perspect. Med. Educ. 1 , 76–85 (2012).
Article PubMed PubMed Central Google Scholar
Pajares, F. Self-efficacy beliefs in academic settings. Rev. Educ. Res. 66 , 543–578 (1996).
Zhao, Z. Classroom Teaching Design of Layout Design Based on Self Efficacy Theory (Tianjin University of Technology and Education, 2021).
Yılmaz, F. G. K. & Yılmaz, R. Exploring the role of sociability, sense of community and course satisfaction on students’ engagement in flipped classroom supported by facebook groups. J. Comput. Educ. 10 , 135–162 (2023).
Nguyen, N. P., Yan, G. & Thai, M. T. Analysis of misinformation containment in online social networks. Comput. Netw. 57 , 2133–2146 (2013).
Connaway, L. S., Radford, M. L., Dickey, T. J., Williams, J. D. A. & Confer, P. Sense-making and synchronicity: Information-seeking behaviors of millennials and baby boomers. Libri 58 , 123–135 (2008).
Wankel, C., Marovich, M. & Stanaityte, J. Cutting-edge social media approaches to business education : teaching with LinkedIn, Facebook, Twitter, Second Life, and blogs . (Global Management Journal, 2010).
Redecker, C., Ala-Mutka, K. & Punie, Y. Learning 2.0: The impact of social media on learning in Europe. Policy brief. JRC Scientific and Technical Report. EUR JRC56958 EN . Available from http://bit.ly/cljlpq [Accessed 6 th February 2011] 6 (2010).
Cao, Y. & Hong, P. Antecedents and consequences of social media utilization in college teaching: A proposed model with mixed-methods investigation. Horizon 19 , 297–306 (2011).
Maqableh, M. et al. The impact of social media networks websites usage on students’ academic performance. Commun. Netw. 7 , 159–171 (2015).
Bandura, A. Self-Efficacy (Worth Publishers, 1997).
Karaoglan-Yilmaz, F. G., Ustun, A. B., Zhang, K. & Yilmaz, R. Metacognitive awareness, reflective thinking, problem solving, and community of inquiry as predictors of academic self-efficacy in blended learning: A correlational study. Turk. Online J. Distance Educ. 24 , 20–36 (2023).
Liu, W. Self-efficacy Level and Analysis of Influencing Factors on Non-English Major Bilingual University Students—An Investigation Based on Three (Xinjiang Normal University, 2015).
Yan, W. Influence of College Students’ Positive Emotions on Learning Engagement and Academic Self-efficacy (Shanghai Normal University, 2016).
Pan, J. Relational Model Construction between College Students’ Learning Self-efficacy and Their Online Autonomous Learning Ability (Northeast Normal University, 2017).
Kang, Y. The Study on the Relationship Between Learning Motivation, Self-efficacy and Burnout in College Students (Shanxi University of Finance and Economics, 2018).
Huang, L. A Study on the Relationship between Chinese Learning Efficacy and Learning Motivation of Foreign Students in China (Huaqiao University, 2018).
Kong, W. Research on the Mediating Role of Undergraduates’ Learning Self-efficacy in the Relationship between Professional Identification and Learning Burnout (Shanghai Normal University, 2019).
Kuo, T. M., Tsai, C. C. & Wang, J. C. Linking web-based learning self-efficacy and learning engagement in MOOCs: The role of online academic hardiness. Internet High. Educ. 51 , 100819 (2021).
Zhan, Y. A Study of the Impact of Social Media Use and Dependence on Real-Life Social Interaction Among University Students (Shanghai International Studies University, 2020).
Qiu, S. A study on mobile learning to assist in developing English learning effectiveness among university students. J. Lanzhou Inst. Educ. 33 , 138–140 (2017).
Yin, R. & Xu, D. A study on the relationship between online learning environment and university students’ learning self-efficacy. E-educ. Res. 9 , 46–52 (2011).
Duo, Z., Zhao, W. & Ren, Y. A New paradigm for building mobile online learning communities: A perspective on the development of self-regulated learning efficacy among university students, in Modern distance education 10–17 (2019).
Park, S. Y., Nam, M.-W. & Cha, S.-B. University students’ behavioral intention to use mobile learning: Evaluating the technology acceptance model: Factors related to use mobile learning. Br. J. Educ. Technol. 43 , 592–605 (2012).
Bian, Y. Development and application of the Learning Self-Efficacy Scale (East China Normal University, 2003).
Shi, X. Between Life Stress and Smartphone Addiction on Taiwanese University Student (Southwest University, 2010).
Liang, Y. Study On Achievement Goals、Attribution Styles and Academic Self-efficacy of Collage Students (Central China Normal University, 2000).
Qiu, H. Quantitative Research and Statistical Analysis (Chongqing University Press, 2013).
Download references
Acknowledgements
This work is supported by the 2023 Guangxi University Young and middle-aged Teachers' Basic Research Ability Enhancement Project—“Research on Innovative Communication Strategies and Effects of Zhuang Traditional Crafts from the Perspective of the Metaverse” (Grant Nos. 2023KY0385), and the special project on innovation and entrepreneurship education in universities under the “14th Five-Year Plan” for Guangxi Education Science in 2023, titled “One Core, Two Directions, Three Integrations - Strategy and Practical Research on Innovation and Entrepreneurship Education in Local Universities” (Grant Nos. 2023ZJY1955), and the 2023 Guangxi Higher Education Undergraduate Teaching Reform General Project (Category B) “Research on the Construction and Development of PBL Teaching Model in Advertising” (Grant Nos.2023JGB294), and the 2022 Guangxi Higher Education Undergraduate Teaching Reform Project (General Category A) “Exploration and Practical Research on Public Art Design Courses in Colleges and Universities under Great Aesthetic Education” (Grant Nos. 2022JGA251), and the 2023 Guangxi Higher Education Undergraduate Teaching Reform Project Key Project “Research and Practice on the Training of Interdisciplinary Composite Talents in Design Majors Based on the Concept of Specialization and Integration—Taking Guangxi Institute of Traditional Crafts as an Example” (Grant Nos. 2023JGZ147), and the2024 Nanning Normal University Undergraduate Teaching Reform Project “Research and Practice on the Application of “Guangxi Intangible Cultural Heritage” in Packaging Design Courses from the Ideological and Political Perspective of the Curriculum” (Grant Nos. 2024JGX048),and the 2023 Hubei Normal University Teacher Teaching Reform Research Project (Key Project) -Curriculum Development for Improving Pre-service Music Teachers' Teaching Design Capabilities from the Perspective of OBE (Grant Nos. 2023014), and the 2023 Guangxi Education Science “14th Five-Year Plan” special project: “Specialized Integration” Model and Practice of Art and Design Majors in Colleges and Universities in Ethnic Areas Based on the OBE Concept (Grant Nos. 2023ZJY1805), and the 2024 Guangxi University Young and Middle-aged Teachers’ Scientific Research Basic Ability Improvement Project “Research on the Integration Path of University Entrepreneurship and Intangible Inheritance - Taking Liu Sanjie IP as an Example” (Grant Nos. 2024KY0374), and the 2022 Research Project on the Theory and Practice of Ideological and Political Education for College Students in Guangxi - “Party Building + Red”: Practice and Research on the Innovation of Education Model in College Student Dormitories (Grant Nos. 2022SZ028), and the 2021 Guangxi University Young and Middle-aged Teachers’ Scientific Research Basic Ability Improvement Project - "Research on the Application of Ethnic Elements in the Visual Design of Live Broadcast Delivery of Guangxi Local Products" (Grant Nos. 2021KY0891).
Author information
Authors and affiliations.
College of Art and Design, Nanning Normal University, Nanning, 530000, Guangxi, China
Graduate School of Techno Design, Kookmin University, Seoul, 02707, Korea
Yicheng Lai
College of Music, Hubei Normal University, Huangshi, 435000, Hubei, China
You can also search for this author in PubMed Google Scholar
Contributions
The contribution of H. to this paper primarily lies in research design and experimental execution. H. was responsible for the overall framework design of the paper, setting research objectives and methods, and actively participating in data collection and analysis during the experimentation process. Furthermore, H. was also responsible for conducting literature reviews and played a crucial role in the writing and editing phases of the paper. L.'s contribution to this paper primarily manifests in theoretical derivation and the discussion section. Additionally, author L. also proposed future research directions and recommendations in the discussion section, aiming to facilitate further research explorations. Y.'s contribution to this paper is mainly reflected in data analysis and result interpretation. Y. was responsible for statistically analyzing the experimental data and employing relevant analytical tools and techniques to interpret and elucidate the data results.
Corresponding author
Correspondence to Jiaying Hu .
Ethics declarations
Competing interests.
The authors declare no competing interests.
Additional information
Publisher's note.
Springer Nature remains neutral with regard to jurisdictional claims in published maps and institutional affiliations.
Supplementary Information
Supplementary information., rights and permissions.
Open Access This article is licensed under a Creative Commons Attribution 4.0 International License, which permits use, sharing, adaptation, distribution and reproduction in any medium or format, as long as you give appropriate credit to the original author(s) and the source, provide a link to the Creative Commons licence, and indicate if changes were made. The images or other third party material in this article are included in the article's Creative Commons licence, unless indicated otherwise in a credit line to the material. If material is not included in the article's Creative Commons licence and your intended use is not permitted by statutory regulation or exceeds the permitted use, you will need to obtain permission directly from the copyright holder. To view a copy of this licence, visit http://creativecommons.org/licenses/by/4.0/ .
Reprints and permissions
About this article
Cite this article.
Hu, J., Lai, Y. & Yi, X. Effectiveness of social media-assisted course on learning self-efficacy. Sci Rep 14 , 10112 (2024). https://doi.org/10.1038/s41598-024-60724-0
Download citation
Received : 02 January 2024
Accepted : 26 April 2024
Published : 02 May 2024
DOI : https://doi.org/10.1038/s41598-024-60724-0
Share this article
Anyone you share the following link with will be able to read this content:
Sorry, a shareable link is not currently available for this article.
Provided by the Springer Nature SharedIt content-sharing initiative
- Design students
- Online learning
- Design professional
By submitting a comment you agree to abide by our Terms and Community Guidelines . If you find something abusive or that does not comply with our terms or guidelines please flag it as inappropriate.
Quick links
- Explore articles by subject
- Guide to authors
- Editorial policies
Sign up for the Nature Briefing newsletter — what matters in science, free to your inbox daily.

Visualizing hidden communities of interest: A case-study analysis of topic-based social networks in astrobiology
- Published: 27 May 2024
Cite this article
- Christophe Malaterre ORCID: orcid.org/0000-0003-1413-6710 1 , 2 &
- Francis Lareau ORCID: orcid.org/0000-0002-0352-5246 3
Author networks in science often rely on citation analyses. In such cases, as in others, network interpretation usually depends on supplementary data, notably about authors’ research domains when disciplinary interpretations are sought. More general social networks also face similar interpretation challenges as to the semantic content specificities of their members. In this research-in-progress, we propose to infer author networks not from citation analyses but from topic similarity analyses based on a topic-model of published documents. Such author networks reveal, as we call them, “hidden communities of interest” (HCoIs) whose semantic content can easily be interpreted by means of their associated topics in the model. We use an astrobiology corpus of full-text articles ( N = 3,698) to illustrate the approach. Having conducted an LDA topic-model on all publications, we identify the underlying communities of authors by measuring author correlations in terms of topic distributions. Adding publication dates makes it possible to examine HCoI evolution over time. This approach to social networks supplements traditional methods in contexts where textual data are available.
This is a preview of subscription content, log in via an institution to check access.
Access this article
Price includes VAT (Russian Federation)
Instant access to the full article PDF.
Rent this article via DeepDyve
Institutional subscriptions
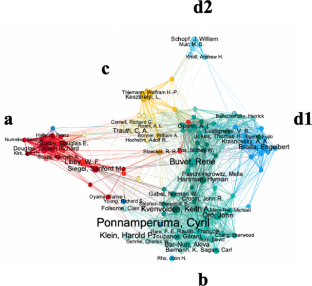
Abbreviations
- Hidden communities of interest
Social network analysis
Latent Dirichlet analysis
Angelov, D. (2020). Top2Vec: Distributed representations of topics ( arXiv:2008.09470 ). http://arxiv.org/abs/2008.09470
Arbelaitz, O., Gurrutxaga, I., Muguerza, J., Pérez, J. M., & Perona, I. (2013). An extensive comparative study of cluster validity indices. Pattern Recognition , 46(1), 243–256. https://doi.org/10.1016/j.patcog.2012.07.021
Bastian, M., Heymann, S., & Jacomy, M. (2009). Gephi: An open source software for exploring and manipulating networks. International Conference on Weblogs and Social Media . https://doi.org/10.1609/icwsm.v3i1.13937
Article Google Scholar
Beyer, K., Goldstein, J., Ramakrishnan, R., & Shaft, U. (1999). When is “nearest neighbor” meaningful? International Conference on Database Theory . https://doi.org/10.1007/3-540-49257-7_15
Blei, D. M., Ng, A. Y., & Jordan, M. I. (2003). Latent dirichlet allocation. Journal of Machine Learning Research, 3 , 993–1022.
Google Scholar
Boyack, K. W., Klavans, R., & Börner, K. (2005). Mapping the backbone of science. Scientometrics, 64 (3), 351–374. https://doi.org/10.1007/s11192-005-0255-6
Boyd-Graber, J. L., Hu, Y., & Mimno, D. (2017). Applications of topic models. Foundations and Trends in Information Retrieval . https://doi.org/10.1561/1500000030
Carley, K. (1993). Coding choices for textual analysis: A comparison of content analysis and map analysis. Sociological Methodology, 23 , 75–126. https://doi.org/10.2307/271007
Castelblanco, G., Guevara, J., Mesa, H., & Sanchez, A. (2021). Semantic network analysis of literature on public-private partnerships. Journal of Construction Engineering and Management, 147 (5), 04021033. https://doi.org/10.1061/(ASCE)CO.1943-7862.0002041
Christensen, A. P., & Kenett, Y. N. (2023). Semantic network analysis (SemNA): A tutorial on preprocessing, estimating, and analyzing semantic networks. Psychological Methods, 28 (4), 860–879. https://doi.org/10.1037/met0000463
Crane, D. (1969). Social structure in a group of scientists: A test of the “invisible college” hypothesis. American Sociological Review, 34 (3), 335. https://doi.org/10.2307/2092499
Danowski, J. A. (1993). Network analysis of message content. In W. D. Richards & G. A. Barnett (Eds.), Progress in communication sciences. Ablex Publishing Corporation.
Danowski, J. A. (2011). Counterterrorism mining for individuals semantically-similar to watchlist members. In U. K. Wiil (Ed.), Counterterrorism and open source intelligence. Springer.
Danowski, J. A., & Cepela, N. (2010). Automatic mapping of social networks of actors from text corpora: Time series analysis. In N. Memon, J. J. Xu, D. L. Hicks, & H. Chen (Eds.), Data mining for social network data. Springer.
Danowski, J. A., Van Klyton, A., Tavera-Mesías, J. F., Duque, K., Radwan, A., & Rutabayiro-Ngoga, S. (2023). Policy semantic networks associated with ICT utilization in Africa. Social Network Analysis and Mining, 13 (1), 73. https://doi.org/10.1007/s13278-023-01068-x
de Vries, E., Schoonvelde, M., & Schumacher, G. (2018). No longer lost in translation: Evidence that Google translate works for comparative bag-of-words text applications. Political Analysis, 26 (4), 417–430. https://doi.org/10.1017/pan.2018.26
Dick, S. J., & Strick, J. E. (2004). The living universe NASA and the development of astrobiology . Rutgers University Press.
Diesner, J., & Carley, K. M. (2004). Using network text analysis to detect the organizational structure of covert networks. In P roceedings of the North American Association for Computational Social and Organizational Science (NAACSOS) Conference (Vol. 3). Pittsburgh: NAACSOS.
DiMaggio, P., Nag, M., & Blei, D. (2013). Exploiting affinities between topic modeling and the sociological perspective on culture: Application to newspaper coverage of U.S. government arts funding. Poetics, 41 (6), 570–606. https://doi.org/10.1016/j.poetic.2013.08.004
Doerfel, M. L., & Barnett, G. A. (1999). A semantic network analysis of the international communication association. Human Communication Research, 25 (4), 589–603. https://doi.org/10.1111/j.1468-2958.1999.tb00463.x
Field, A. P. (2009). Discovering statistics using SPSS: And sex, drugs and rock “n” roll . SAGE Publications.
Firth, J. R. (1957). A synopsis of linguistic theory 1930–1955. In J. R. Firth (Ed.), Studies in linguistic analysis (pp. 1–32). Blackwell.
Fortunato, S., Bergstrom, C. T., Börner, K., Evans, J. A., Helbing, D., Milojević, S., Petersen, A. M., Radicchi, F., Sinatra, R., Uzzi, B., Vespignani, A., Waltman, L., Wang, D., & Barabási, A.-L. (2018). Science of science. Science . https://doi.org/10.1126/science.aao0185
Griffiths, T. L., & Steyvers, M. (2004). Finding scientific topics. Proceedings of the National Academy of Sciences, 101 , 5228–5235. https://doi.org/10.1073/pnas.0307752101
Harris, Z. S. (1954). Distributional structure. Word, 10 (2–3), 146–162. https://doi.org/10.1080/00437956.1954.11659520
Horneck, G., Walter, N., Westall, F., Grenfell, J. L., Martin, W. F., Gomez, F., Leuko, S., Lee, N., Onofri, S., Tsiganis, K., Saladino, R., Pilat-Lohinger, E., Palomba, E., Harrison, J., Rull, F., Muller, C., Strazzulla, G., Brucato, J. R., Rettberg, P., & Capria, M. T. (2016). AstRoMap European astrobiology roadmap. Astrobiology, 16 (3), 201–243. https://doi.org/10.1089/ast.2015.1441
Kherwa, P., & Bansal, P. (2020). Topic modeling: A comprehensive review. EAI Endorsed Transactions on Scalable Information Systems . https://doi.org/10.4108/eai.13-7-2018.159623
Malaterre, C., & Lareau, F. (2022). The early days of contemporary philosophy of science: Novel insights from machine translation and topic-modeling of non-parallel multilingual corpora. Synthese, 200 (3), 242. https://doi.org/10.1007/s11229-022-03722-x
Article MathSciNet Google Scholar
Malaterre, C., & Lareau, F. (2023). The emergence of astrobiology: A topic-modeling perspective. Astrobiology, 23 (5), 496–512. https://doi.org/10.1089/ast.2022.0122
Des Marais, D. J., Allamandola, L. J., Benner, S. A., Boss, A. P., Deamer, D., Falkowski, P. G., Farmer, J. D., Hedges, S. B., Jakosky, B. M., Knoll, A. H., Liskowsky, D. R., Meadows, V. S., Meyer, M. A., Pilcher, C. B., Nealson, K. H., Spormann, A. M., Trent, J. D., Turner, W. W., Woolf, N. J., & Yorke, H. W. (2003). The NASA astrobiology roadmap. Astrobiology, 3 (2), 219–235. https://doi.org/10.1089/153110703769016299
McCallum, A., Wang, X., & Corrada-Emmanuel, A. (2007). Topic and role discovery in social networks with experiments on enron and academic email. Journal of Artificial Intelligence Research, 30 , 249–272. https://doi.org/10.1613/jair.2229
Röder, M., Both, A., & Hinneburg, A. (2015). Exploring the space of topic coherence measures. Proceedings of the Eighth ACM International Conference on Web Search and Data Mining-WSDM ’15 . https://doi.org/10.1145/2684822.2685324
Schmid, H. (1994). Probabilistic part-of-speech tagging using decision trees. Proceedings of International Conference on New Methods in Language Processing , 44–49.
Segev, E. (2021). Semantic network analysis in social sciences . Routledge.
Book Google Scholar
Siew, C. S. Q., Wulff, D. U., Beckage, N. M., & Kenett, Y. N. (2019). Cognitive network science: A review of research on cognition through the lens of network representations, processes, and dynamics. Complexity, 2019 , e2108423. https://doi.org/10.1155/2019/2108423
Steyvers, M., Smyth, P., Rosen-Zvi, M., & Griffiths, T. (2004). Probabilistic author-topic models for information discovery. Proceedings of the of the 2004 ACM SIGKDD International Conference on Knowledge Discovery and Data Mining-KDD ’04 . https://doi.org/10.1145/1014052.1014087
Ye, F., Chen, C., & Zheng, Z. (2018). Deep autoencoder-like nonnegative matrix factorization for community detection. Proceedings of the 27th ACM International Conference on Information and Knowledge Management , 1393–1402.
Zhang, H., Qiu, B., Giles, C. L., Foley, H. C., & Yen, J. (2007). An LDA-based community structure discovery approach for large-scale social networks. 2007 IEEE Intelligence and Security Informatics , 200–207
Zhao, W., Chen, J. J., Perkins, R., Liu, Z., Ge, W., Ding, Y., & Zou, W. (2015). A heuristic approach to determine an appropriate number of topics in topic modeling. BMC Bioinformatics . https://doi.org/10.1186/1471-2105-16-S13-S8
Download references
Acknowledgements
C.M. acknowledges funding from Canada Social Sciences and Humanities Research Council (Grant 430-2018-00899) and Canada Research Chairs (CRC-950-230795). F.L. acknowledges funding from the Canada Social Sciences and Humanities Research Council (756-2024-0557) and the Canada Research Chair in Philosophy of the Life Sciences at UQAM. The authors thank the audience of ISSI 2023 for most helpful comments on an earlier version of this paper published in the conference proceedings as: Malaterre, C., & Lareau, F. (2023). Visualizing hidden communities of interest: A preliminary analysis of topic-based social networks in astrobiology. Proceedings of ISSI 2023 . The 19th Conference of the International Society for Scientometrics and Informetrics, Bloomington, IN.
Author information
Authors and affiliations.
Département de philosophie, Université du Québec à Montréal (UQAM), MontrealMontréal, Canada
Christophe Malaterre
Centre interuniversitaire de recherche sur la science et la technologie, Université du Québec à Montréal (UQAM), Montréal, Canada
Département d’informatique, Université du Québec à Montréal (UQAM), Montréal, Canada
Francis Lareau
You can also search for this author in PubMed Google Scholar
Contributions
Conceptualization: CM, FL; Data curation: FL; Formal analysis and investigation: CM, FL; Funding acquisition: CM; Investigation: CM, FL; Methodology: CM, FL; Project administration: CM; Resources: CM; Software: FL; Supervision: CM; Validation: CM, FL; Visualization: CM; Writing – original draft preparation: CM; Writing—review and editing: CM, FL. Both authors approved the final submitted manuscript.
Corresponding author
Correspondence to Christophe Malaterre .
Ethics declarations
Conflict of interest.
The authors declare no competing interests.
Additional information
Publisher's note.
Springer Nature remains neutral with regard to jurisdictional claims in published maps and institutional affiliations.
Rights and permissions
Springer Nature or its licensor (e.g. a society or other partner) holds exclusive rights to this article under a publishing agreement with the author(s) or other rightsholder(s); author self-archiving of the accepted manuscript version of this article is solely governed by the terms of such publishing agreement and applicable law.
Reprints and permissions
About this article
Malaterre, C., Lareau, F. Visualizing hidden communities of interest: A case-study analysis of topic-based social networks in astrobiology. Scientometrics (2024). https://doi.org/10.1007/s11192-024-05047-7
Download citation
Received : 17 November 2023
Accepted : 29 April 2024
Published : 27 May 2024
DOI : https://doi.org/10.1007/s11192-024-05047-7
Share this article
Anyone you share the following link with will be able to read this content:
Sorry, a shareable link is not currently available for this article.
Provided by the Springer Nature SharedIt content-sharing initiative
- Hidden colleges
- Social networks
- Semantic networks
- Topic-modeling
- Philosophy of science
- Find a journal
- Publish with us
- Track your research

IMAGES
VIDEO
COMMENTS
Social network analysis is a powerful tool for visualizing, understanding, and harnessing the power of networks and relationships. At Visible Network Labs, we use our network science and mapping tools and expertise to track collaborative ecosystems and strengthen systems change initiatives. In this Comprehensive Guide, we'll introduce key ...
Social Network Analysis (SNA) is an important part of a network's overall evaluation strategy, allowing us to measure 1) the depth of relationship between participants, 2) the frequency with ...
Social network analysis as knowledge discovery process: A case study on digital bibliography. ... online social networks provides new opportunities in computer science, sociology, math, information studies, biology, business, and more. Social network analysis (SNA) is a paramount technique supporting understanding ...
Experience Information Technology conferences. Join your peers for the unveiling of the latest insights at Gartner conferences. This report presents five real world examples of social network analysis that will assist in the development of a concrete business case.
Social Network Analysis (SNA), also known as network science, is a general study of the social network utilizing network and graph theory concepts. It explores the behavior of individuals at the micro-level, their relationships (social structure) at the macro level, and the connection between the two. SNA uses several methods and tools to study ...
Prior research has acknowledged the multi-layered nature of a firm's embeddedness in social networks. Firms' or other actors' position in the social network has traditionally been studied by B2B scholars from the ego network or the whole network perspective (e.g., Chakravarty et al., 2020; Swaminathan & Moorman, 2009).The ego network perspective posits that firm behavior and performance are ...
Module 3 • 3 hours to complete. In this module, you will begin with a social network analysis lab activity. You will be able to do data wrangling of databases and visualize a network. You will be able to analyze a social network and also be able to examine other social network analysis through case studies.
A social network is a set of actors—that is, any discrete entity in a network, such as a person, team, organization, place, or collective social unit—and the ties connecting them—that is, some type of relationship, exchange, or interaction between actors that serves as a conduit through which resources such as information, trust, goodwill ...
The search limitations have resulted in 2,881 articles, but many were still not related to network analysis or business and management. Further, 723 articles were excluded, covering articles in neuroscience, bibliometric, circuit network (engineering), earth and planetary science, chemistry, etc., although the articles employed network analysis as a methodology.
The work covers Social Network Analysis theory and methods with a focus on current applications and case studies applied in various domains such as mobile networks, security, machine learning and health. With the increasing popularity of Web 2.0, social media has become a widely used communication platform.
A social network is a social structure made up of actors called nodes, which are connected by various types of relationships. SNA is used to analyze and measure these relationships between people, groups and other information/knowledge processing entities and provides both a structural and a mathematical analysis.
'Social network analysis and theory help us not only delineate the individual actors in the knowledge flow network but also to determine network ties, relations, frequency, and density' (p. 1266). ... Journal of International Business Studies, 38 (2007), pp. 1231-1233, 10.1057/palgrave.jibs.8400321. Google Scholar. Dunning, 1988.
Concluding Insights on Social Network Analysis Social Network Analysis is a comprehensive research methodology that illuminates the intricate dynamics of networks within social and organizational contexts. It is a cornerstone for strategic decision-making, providing a lens to view patterns and trends in relationships and interactions.
1. Introduction. The SNA is a theory investigating the relations and interactions based on anthropology, sociology, and social psychology to assess social structures (Erçetin and Neyişci, 2014).The social structure in a network theory comprises individuals or organisations named nodes linked through one or more types of interdependencies, such as friendship, kinship, financial exchange ...
a Practical Case Study. Abstract. Social networks are considered a new way of. socialization; they can function as a source of interaction. among pe ople enabling contextualization of social ...
Social Network Analysis (SNA) is an established method in sociology since the early 20th century that has gained prominence in recent decades due to technological advances. It is versatile and can be applied in a wide range of fields—including economics, biology, medicine, communications, and more—by identifying key actors within a social ...
The rest of this article is organized as follows: In Sect. 2 we cover the literature related to social network platforms and social media analysis, including Twitter. In Sect. 3 we formally present our analysis methods. Section 4 includes the case study and the analysis of the results.
Social network analysis is the process of investigating social structures through the use of networks and graph theory. This article introduces data scientists to the theory of social networks, with a short introduction to graph theory and information spread. It dives into Python code with NetworkX constructing and implying social networks from ...
The book addresses the issue of interdisciplinary understanding of collaboration on the topic of social network studies. Researchers and practitioners from various disciplines including sociology, computer science, socio-psychology, public health, complex systems, and management science have worked largely independently, each with quite different principles, terminologies, theories. and ...
Social network analysis (SNA), also known as network science, is a field of data analytics that uses networks and graph theory to understand social structures. SNA techniques can also be applied to networks outside of the societal realm. More From Mitch Ditch Your Passwords — They're Only Hurting You. Gephi. Marvel Universe Social Network.
Pajek: Pajek is a program for the analysis and visualization of large networks. It's an extensive tool, offering a range of complex network metrics, and is free for non-commercial use. NodeXL: NodeXL is a free, open-source template for Microsoft Excel that allows users to display and analyze network graphs.
The importance of social networks for innovation diffusion and processes of social change is widely recognized in many areas of practice and scientific disciplines. Social networks have the potential to influence learning processes, provide opportunities for problem-solving, and establish new ideas. Thus, they can foster synergy effects, bring together key resources such as know-how of ...
5.1 Data Analysis for Overall System Network. In Fig. 2, growth coefficient is dependent on number of elements as user. Growth coefficient is increased according to increasing value of number of elements. We have introduced a constant value "Current Element" (C_ELE) for stabilizing value of growth coefficient. Fig. 2.
The social media platform and the information dissemination revolution have changed the thinking, needs, and methods of students, bringing development opportunities and challenges to higher education.
This paper focuses on the problem of information source identification in online social networks (OSNs). By analyzing the research situation of source identification problems and challenges (such as the randomness of the information dissemination process and complexity of the underlying network topology), this paper studies the problem of multiple source diffusion and proposes a source ...
The case study's findings are presented using a novel social network analysis framework in the case discussion section. The paper concludes by summarizing the research insights and implications, stating the limitations and charting the future avenues for prospective researchers in similar domains.
Author networks in science often rely on citation analyses. In such cases, as in others, network interpretation usually depends on supplementary data, notably about authors' research domains when disciplinary interpretations are sought. More general social networks also face similar interpretation challenges as to the semantic content specificities of their members. In this research-in ...