- En español – ExME
- Em português – EME

What are sampling methods and how do you choose the best one?
Posted on 18th November 2020 by Mohamed Khalifa
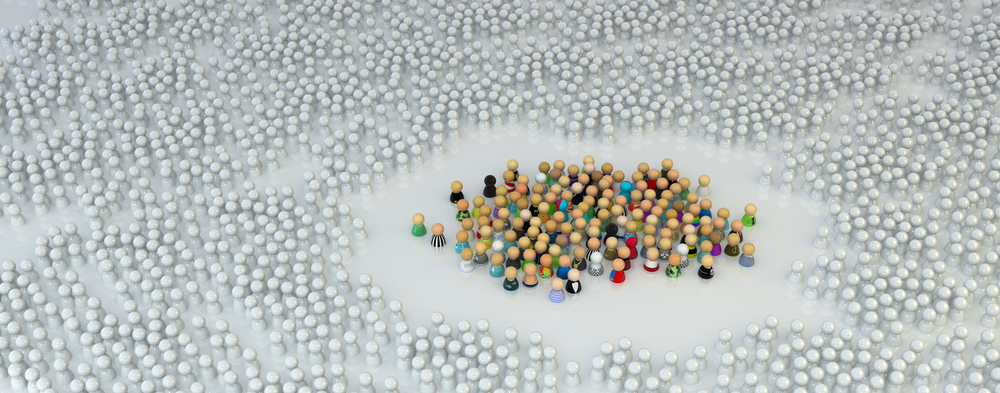
This tutorial will introduce sampling methods and potential sampling errors to avoid when conducting medical research.
Introduction to sampling methods
Examples of different sampling methods, choosing the best sampling method.
It is important to understand why we sample the population; for example, studies are built to investigate the relationships between risk factors and disease. In other words, we want to find out if this is a true association, while still aiming for the minimum risk for errors such as: chance, bias or confounding .
However, it would not be feasible to experiment on the whole population, we would need to take a good sample and aim to reduce the risk of having errors by proper sampling technique.
What is a sampling frame?
A sampling frame is a record of the target population containing all participants of interest. In other words, it is a list from which we can extract a sample.
What makes a good sample?
A good sample should be a representative subset of the population we are interested in studying, therefore, with each participant having equal chance of being randomly selected into the study.
We could choose a sampling method based on whether we want to account for sampling bias; a random sampling method is often preferred over a non-random method for this reason. Random sampling examples include: simple, systematic, stratified, and cluster sampling. Non-random sampling methods are liable to bias, and common examples include: convenience, purposive, snowballing, and quota sampling. For the purposes of this blog we will be focusing on random sampling methods .
Example: We want to conduct an experimental trial in a small population such as: employees in a company, or students in a college. We include everyone in a list and use a random number generator to select the participants
Advantages: Generalisable results possible, random sampling, the sampling frame is the whole population, every participant has an equal probability of being selected
Disadvantages: Less precise than stratified method, less representative than the systematic method
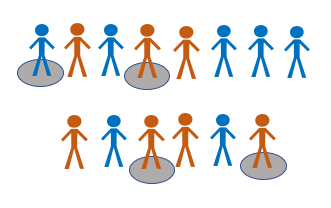
Example: Every nth patient entering the out-patient clinic is selected and included in our sample
Advantages: More feasible than simple or stratified methods, sampling frame is not always required
Disadvantages: Generalisability may decrease if baseline characteristics repeat across every nth participant
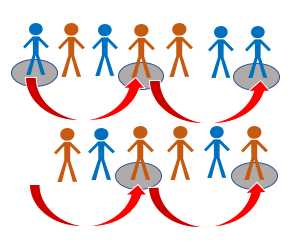
Example: We have a big population (a city) and we want to ensure representativeness of all groups with a pre-determined characteristic such as: age groups, ethnic origin, and gender
Advantages: Inclusive of strata (subgroups), reliable and generalisable results
Disadvantages: Does not work well with multiple variables
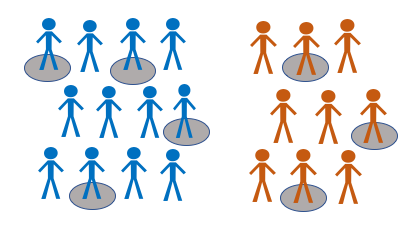
Example: 10 schools have the same number of students across the county. We can randomly select 3 out of 10 schools as our clusters
Advantages: Readily doable with most budgets, does not require a sampling frame
Disadvantages: Results may not be reliable nor generalisable
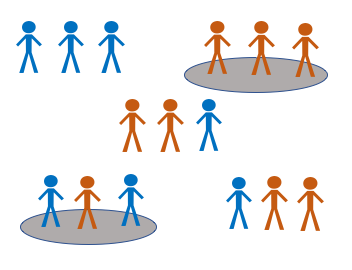
How can you identify sampling errors?
Non-random selection increases the probability of sampling (selection) bias if the sample does not represent the population we want to study. We could avoid this by random sampling and ensuring representativeness of our sample with regards to sample size.
An inadequate sample size decreases the confidence in our results as we may think there is no significant difference when actually there is. This type two error results from having a small sample size, or from participants dropping out of the sample.
In medical research of disease, if we select people with certain diseases while strictly excluding participants with other co-morbidities, we run the risk of diagnostic purity bias where important sub-groups of the population are not represented.
Furthermore, measurement bias may occur during re-collection of risk factors by participants (recall bias) or assessment of outcome where people who live longer are associated with treatment success, when in fact people who died were not included in the sample or data analysis (survivors bias).
By following the steps below we could choose the best sampling method for our study in an orderly fashion.
Research objectiveness
Firstly, a refined research question and goal would help us define our population of interest. If our calculated sample size is small then it would be easier to get a random sample. If, however, the sample size is large, then we should check if our budget and resources can handle a random sampling method.
Sampling frame availability
Secondly, we need to check for availability of a sampling frame (Simple), if not, could we make a list of our own (Stratified). If neither option is possible, we could still use other random sampling methods, for instance, systematic or cluster sampling.
Study design
Moreover, we could consider the prevalence of the topic (exposure or outcome) in the population, and what would be the suitable study design. In addition, checking if our target population is widely varied in its baseline characteristics. For example, a population with large ethnic subgroups could best be studied using a stratified sampling method.
Random sampling
Finally, the best sampling method is always the one that could best answer our research question while also allowing for others to make use of our results (generalisability of results). When we cannot afford a random sampling method, we can always choose from the non-random sampling methods.
To sum up, we now understand that choosing between random or non-random sampling methods is multifactorial. We might often be tempted to choose a convenience sample from the start, but that would not only decrease precision of our results, and would make us miss out on producing research that is more robust and reliable.
References (pdf)
Mohamed Khalifa
Leave a reply cancel reply.
Your email address will not be published. Required fields are marked *
Save my name, email, and website in this browser for the next time I comment.
No Comments on What are sampling methods and how do you choose the best one?
Thank you for this overview. A concise approach for research.
really helps! am an ecology student preparing to write my lab report for sampling.
I learned a lot to the given presentation.. It’s very comprehensive… Thanks for sharing…
Very informative and useful for my study. Thank you
Oversimplified info on sampling methods. Probabilistic of the sampling and sampling of samples by chance does rest solely on the random methods. Factors such as the random visits or presentation of the potential participants at clinics or sites could be sufficiently random in nature and should be used for the sake of efficiency and feasibility. Nevertheless, this approach has to be taken only after careful thoughts. Representativeness of the study samples have to be checked at the end or during reporting by comparing it to the published larger studies or register of some kind in/from the local population.
Thank you so much Mr.mohamed very useful and informative article
Subscribe to our newsletter
You will receive our monthly newsletter and free access to Trip Premium.
Related Articles
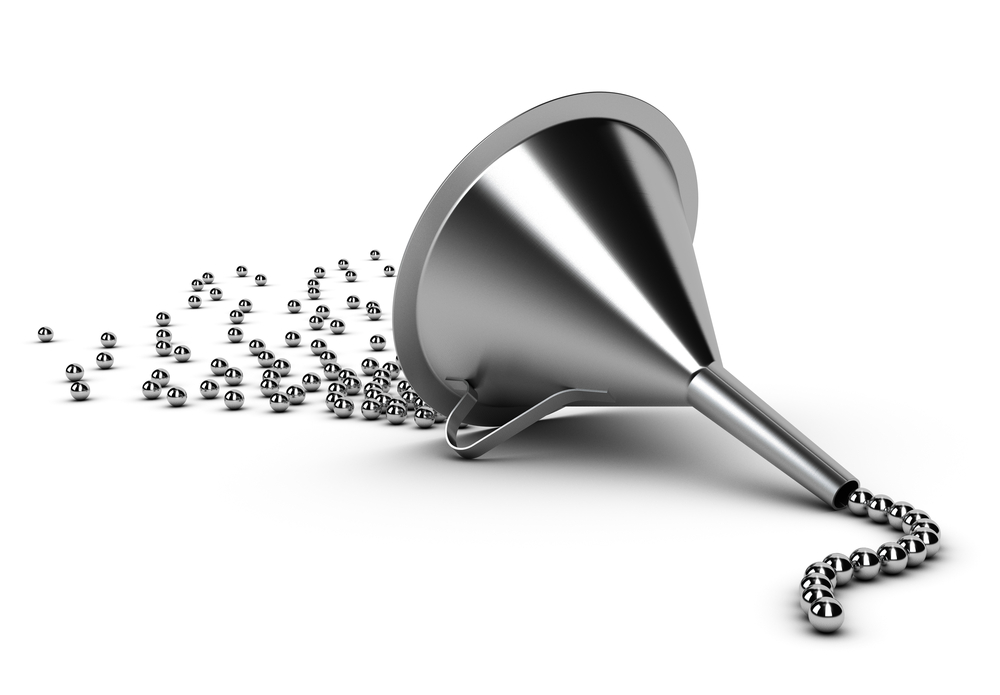
How to read a funnel plot
This blog introduces you to funnel plots, guiding you through how to read them and what may cause them to look asymmetrical.
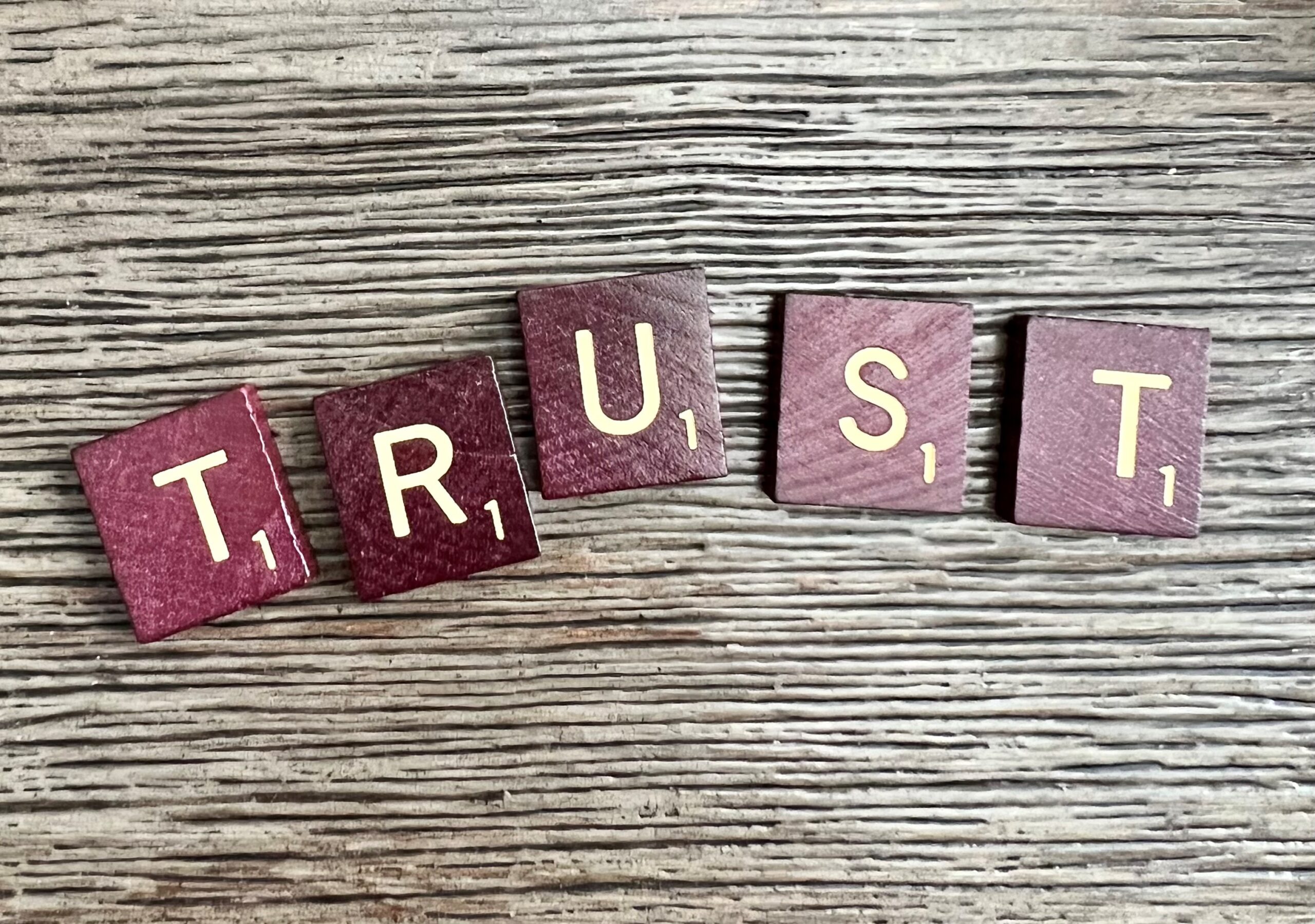
Internal and external validity: what are they and how do they differ?
Is this study valid? Can I trust this study’s methods and design? Can I apply the results of this study to other contexts? Learn more about internal and external validity in research to help you answer these questions when you next look at a paper.
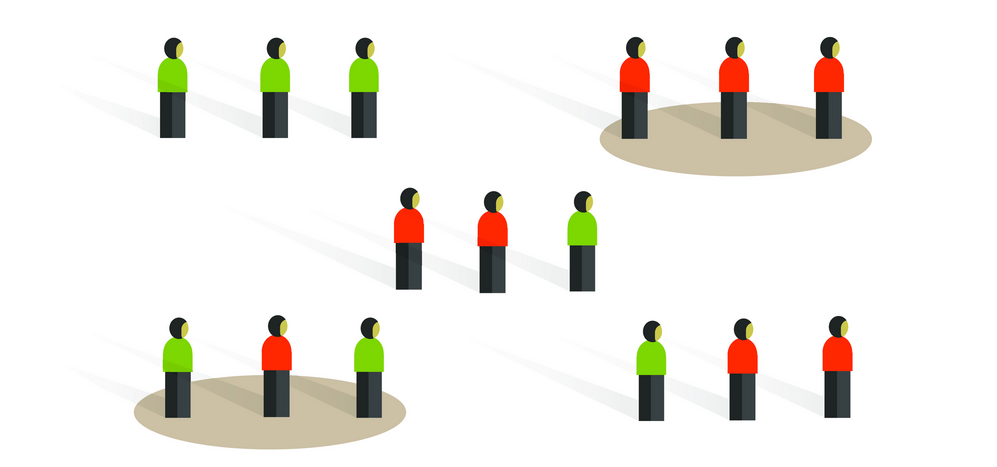
Cluster Randomized Trials: Concepts
This blog summarizes the concepts of cluster randomization, and the logistical and statistical considerations while designing a cluster randomized controlled trial.

Sampling Methods & Strategies 101
Everything you need to know (including examples)
By: Derek Jansen (MBA) | Expert Reviewed By: Kerryn Warren (PhD) | January 2023
If you’re new to research, sooner or later you’re bound to wander into the intimidating world of sampling methods and strategies. If you find yourself on this page, chances are you’re feeling a little overwhelmed or confused. Fear not – in this post we’ll unpack sampling in straightforward language , along with loads of examples .
Overview: Sampling Methods & Strategies
- What is sampling in a research context?
- The two overarching approaches
Simple random sampling
Stratified random sampling, cluster sampling, systematic sampling, purposive sampling, convenience sampling, snowball sampling.
- How to choose the right sampling method
What (exactly) is sampling?
At the simplest level, sampling (within a research context) is the process of selecting a subset of participants from a larger group . For example, if your research involved assessing US consumers’ perceptions about a particular brand of laundry detergent, you wouldn’t be able to collect data from every single person that uses laundry detergent (good luck with that!) – but you could potentially collect data from a smaller subset of this group.
In technical terms, the larger group is referred to as the population , and the subset (the group you’ll actually engage with in your research) is called the sample . Put another way, you can look at the population as a full cake and the sample as a single slice of that cake. In an ideal world, you’d want your sample to be perfectly representative of the population, as that would allow you to generalise your findings to the entire population. In other words, you’d want to cut a perfect cross-sectional slice of cake, such that the slice reflects every layer of the cake in perfect proportion.
Achieving a truly representative sample is, unfortunately, a little trickier than slicing a cake, as there are many practical challenges and obstacles to achieving this in a real-world setting. Thankfully though, you don’t always need to have a perfectly representative sample – it all depends on the specific research aims of each study – so don’t stress yourself out about that just yet!
With the concept of sampling broadly defined, let’s look at the different approaches to sampling to get a better understanding of what it all looks like in practice.
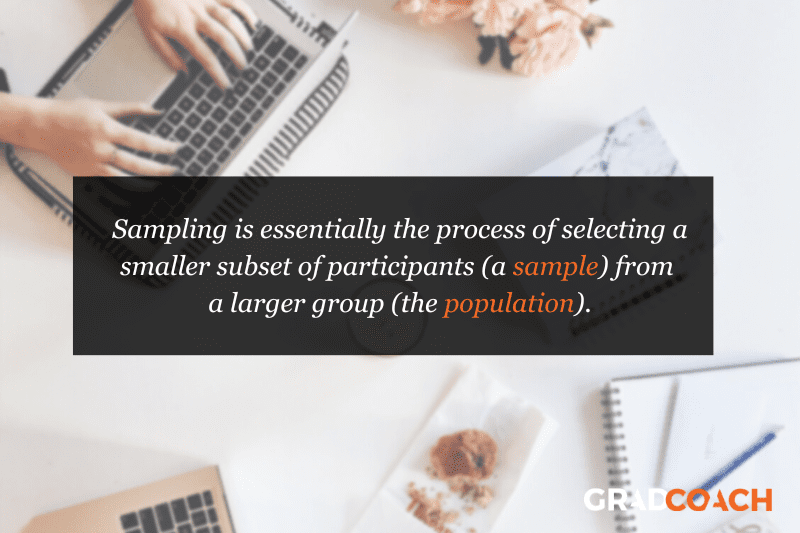
The two overarching sampling approaches
At the highest level, there are two approaches to sampling: probability sampling and non-probability sampling . Within each of these, there are a variety of sampling methods , which we’ll explore a little later.
Probability sampling involves selecting participants (or any unit of interest) on a statistically random basis , which is why it’s also called “random sampling”. In other words, the selection of each individual participant is based on a pre-determined process (not the discretion of the researcher). As a result, this approach achieves a random sample.
Probability-based sampling methods are most commonly used in quantitative research , especially when it’s important to achieve a representative sample that allows the researcher to generalise their findings.
Non-probability sampling , on the other hand, refers to sampling methods in which the selection of participants is not statistically random . In other words, the selection of individual participants is based on the discretion and judgment of the researcher, rather than on a pre-determined process.
Non-probability sampling methods are commonly used in qualitative research , where the richness and depth of the data are more important than the generalisability of the findings.
If that all sounds a little too conceptual and fluffy, don’t worry. Let’s take a look at some actual sampling methods to make it more tangible.
Need a helping hand?
Probability-based sampling methods
First, we’ll look at four common probability-based (random) sampling methods:
Importantly, this is not a comprehensive list of all the probability sampling methods – these are just four of the most common ones. So, if you’re interested in adopting a probability-based sampling approach, be sure to explore all the options.
Simple random sampling involves selecting participants in a completely random fashion , where each participant has an equal chance of being selected. Basically, this sampling method is the equivalent of pulling names out of a hat , except that you can do it digitally. For example, if you had a list of 500 people, you could use a random number generator to draw a list of 50 numbers (each number, reflecting a participant) and then use that dataset as your sample.
Thanks to its simplicity, simple random sampling is easy to implement , and as a consequence, is typically quite cheap and efficient . Given that the selection process is completely random, the results can be generalised fairly reliably. However, this also means it can hide the impact of large subgroups within the data, which can result in minority subgroups having little representation in the results – if any at all. To address this, one needs to take a slightly different approach, which we’ll look at next.
Stratified random sampling is similar to simple random sampling, but it kicks things up a notch. As the name suggests, stratified sampling involves selecting participants randomly , but from within certain pre-defined subgroups (i.e., strata) that share a common trait . For example, you might divide the population into strata based on gender, ethnicity, age range or level of education, and then select randomly from each group.
The benefit of this sampling method is that it gives you more control over the impact of large subgroups (strata) within the population. For example, if a population comprises 80% males and 20% females, you may want to “balance” this skew out by selecting a random sample from an equal number of males and females. This would, of course, reduce the representativeness of the sample, but it would allow you to identify differences between subgroups. So, depending on your research aims, the stratified approach could work well.
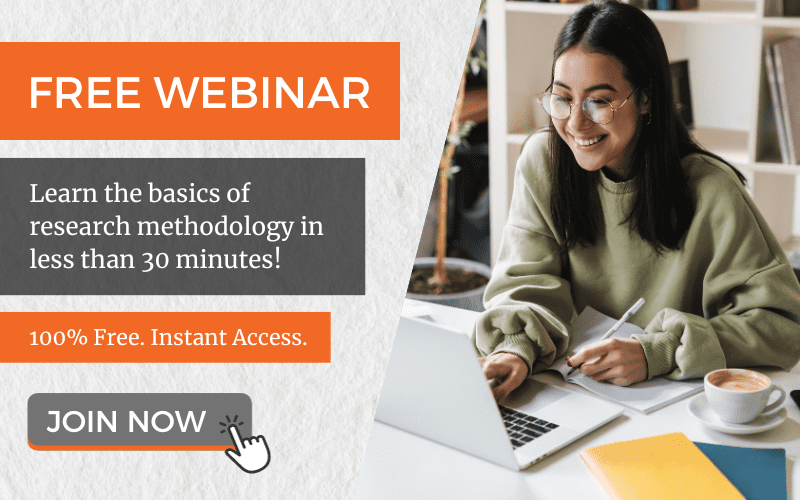
Next on the list is cluster sampling. As the name suggests, this sampling method involves sampling from naturally occurring, mutually exclusive clusters within a population – for example, area codes within a city or cities within a country. Once the clusters are defined, a set of clusters are randomly selected and then a set of participants are randomly selected from each cluster.
Now, you’re probably wondering, “how is cluster sampling different from stratified random sampling?”. Well, let’s look at the previous example where each cluster reflects an area code in a given city.
With cluster sampling, you would collect data from clusters of participants in a handful of area codes (let’s say 5 neighbourhoods). Conversely, with stratified random sampling, you would need to collect data from all over the city (i.e., many more neighbourhoods). You’d still achieve the same sample size either way (let’s say 200 people, for example), but with stratified sampling, you’d need to do a lot more running around, as participants would be scattered across a vast geographic area. As a result, cluster sampling is often the more practical and economical option.
If that all sounds a little mind-bending, you can use the following general rule of thumb. If a population is relatively homogeneous , cluster sampling will often be adequate. Conversely, if a population is quite heterogeneous (i.e., diverse), stratified sampling will generally be more appropriate.
The last probability sampling method we’ll look at is systematic sampling. This method simply involves selecting participants at a set interval , starting from a random point .
For example, if you have a list of students that reflects the population of a university, you could systematically sample that population by selecting participants at an interval of 8 . In other words, you would randomly select a starting point – let’s say student number 40 – followed by student 48, 56, 64, etc.
What’s important with systematic sampling is that the population list you select from needs to be randomly ordered . If there are underlying patterns in the list (for example, if the list is ordered by gender, IQ, age, etc.), this will result in a non-random sample, which would defeat the purpose of adopting this sampling method. Of course, you could safeguard against this by “shuffling” your population list using a random number generator or similar tool.
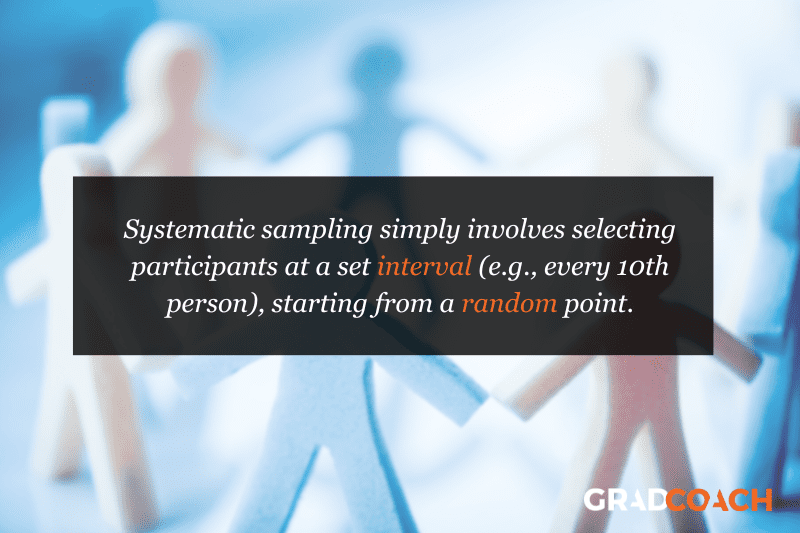
Non-probability-based sampling methods
Right, now that we’ve looked at a few probability-based sampling methods, let’s look at three non-probability methods :
Again, this is not an exhaustive list of all possible sampling methods, so be sure to explore further if you’re interested in adopting a non-probability sampling approach.
First up, we’ve got purposive sampling – also known as judgment , selective or subjective sampling. Again, the name provides some clues, as this method involves the researcher selecting participants using his or her own judgement , based on the purpose of the study (i.e., the research aims).
For example, suppose your research aims were to understand the perceptions of hyper-loyal customers of a particular retail store. In that case, you could use your judgement to engage with frequent shoppers, as well as rare or occasional shoppers, to understand what judgements drive the two behavioural extremes .
Purposive sampling is often used in studies where the aim is to gather information from a small population (especially rare or hard-to-find populations), as it allows the researcher to target specific individuals who have unique knowledge or experience . Naturally, this sampling method is quite prone to researcher bias and judgement error, and it’s unlikely to produce generalisable results, so it’s best suited to studies where the aim is to go deep rather than broad .
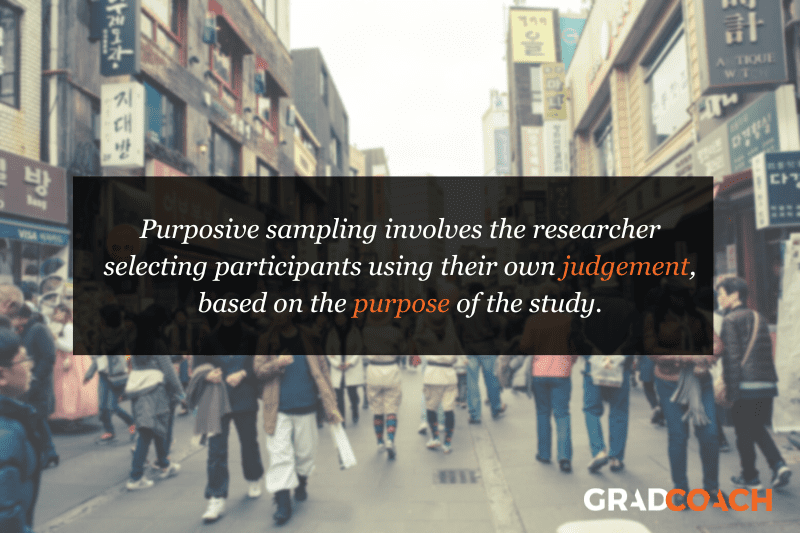
Next up, we have convenience sampling. As the name suggests, with this method, participants are selected based on their availability or accessibility . In other words, the sample is selected based on how convenient it is for the researcher to access it, as opposed to using a defined and objective process.
Naturally, convenience sampling provides a quick and easy way to gather data, as the sample is selected based on the individuals who are readily available or willing to participate. This makes it an attractive option if you’re particularly tight on resources and/or time. However, as you’d expect, this sampling method is unlikely to produce a representative sample and will of course be vulnerable to researcher bias , so it’s important to approach it with caution.
Last but not least, we have the snowball sampling method. This method relies on referrals from initial participants to recruit additional participants. In other words, the initial subjects form the first (small) snowball and each additional subject recruited through referral is added to the snowball, making it larger as it rolls along .
Snowball sampling is often used in research contexts where it’s difficult to identify and access a particular population. For example, people with a rare medical condition or members of an exclusive group. It can also be useful in cases where the research topic is sensitive or taboo and people are unlikely to open up unless they’re referred by someone they trust.
Simply put, snowball sampling is ideal for research that involves reaching hard-to-access populations . But, keep in mind that, once again, it’s a sampling method that’s highly prone to researcher bias and is unlikely to produce a representative sample. So, make sure that it aligns with your research aims and questions before adopting this method.
How to choose a sampling method
Now that we’ve looked at a few popular sampling methods (both probability and non-probability based), the obvious question is, “ how do I choose the right sampling method for my study?”. When selecting a sampling method for your research project, you’ll need to consider two important factors: your research aims and your resources .
As with all research design and methodology choices, your sampling approach needs to be guided by and aligned with your research aims, objectives and research questions – in other words, your golden thread. Specifically, you need to consider whether your research aims are primarily concerned with producing generalisable findings (in which case, you’ll likely opt for a probability-based sampling method) or with achieving rich , deep insights (in which case, a non-probability-based approach could be more practical). Typically, quantitative studies lean toward the former, while qualitative studies aim for the latter, so be sure to consider your broader methodology as well.
The second factor you need to consider is your resources and, more generally, the practical constraints at play. If, for example, you have easy, free access to a large sample at your workplace or university and a healthy budget to help you attract participants, that will open up multiple options in terms of sampling methods. Conversely, if you’re cash-strapped, short on time and don’t have unfettered access to your population of interest, you may be restricted to convenience or referral-based methods.
In short, be ready for trade-offs – you won’t always be able to utilise the “perfect” sampling method for your study, and that’s okay. Much like all the other methodological choices you’ll make as part of your study, you’ll often need to compromise and accept practical trade-offs when it comes to sampling. Don’t let this get you down though – as long as your sampling choice is well explained and justified, and the limitations of your approach are clearly articulated, you’ll be on the right track.
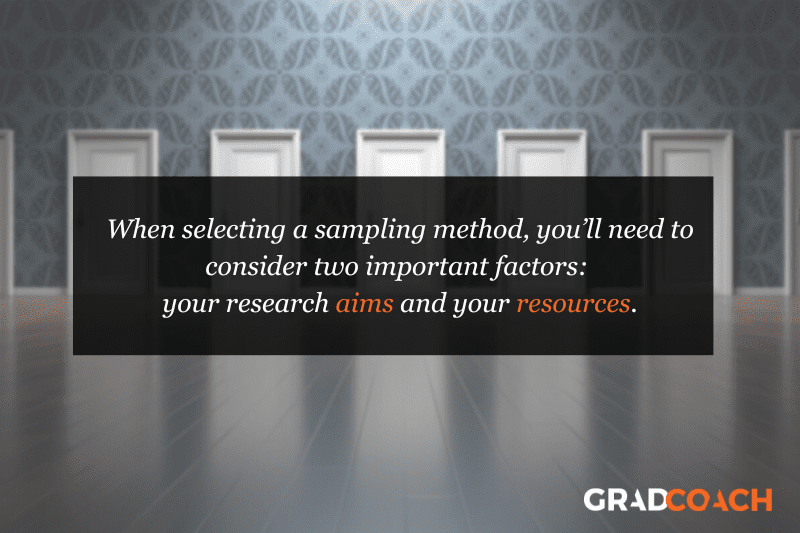
Let’s recap…
In this post, we’ve covered the basics of sampling within the context of a typical research project.
- Sampling refers to the process of defining a subgroup (sample) from the larger group of interest (population).
- The two overarching approaches to sampling are probability sampling (random) and non-probability sampling .
- Common probability-based sampling methods include simple random sampling, stratified random sampling, cluster sampling and systematic sampling.
- Common non-probability-based sampling methods include purposive sampling, convenience sampling and snowball sampling.
- When choosing a sampling method, you need to consider your research aims , objectives and questions, as well as your resources and other practical constraints .
If you’d like to see an example of a sampling strategy in action, be sure to check out our research methodology chapter sample .
Last but not least, if you need hands-on help with your sampling (or any other aspect of your research), take a look at our 1-on-1 coaching service , where we guide you through each step of the research process, at your own pace.
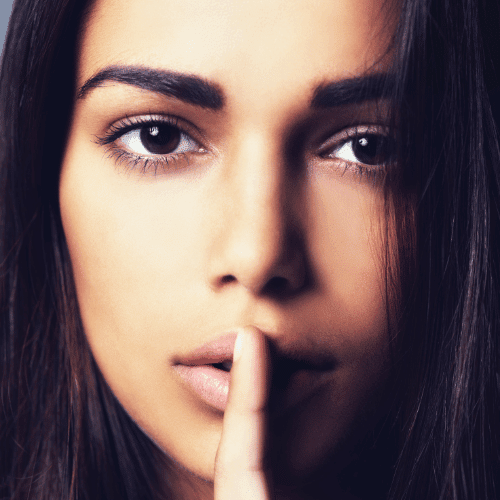
Psst... there’s more!
This post was based on one of our popular Research Bootcamps . If you're working on a research project, you'll definitely want to check this out ...
You Might Also Like:
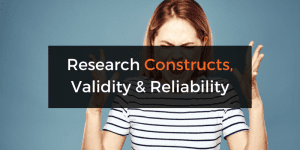
Excellent and helpful. Best site to get a full understanding of Research methodology. I’m nolonger as “clueless “..😉
Excellent and helpful for junior researcher!
Grad Coach tutorials are excellent – I recommend them to everyone doing research. I will be working with a sample of imprisoned women and now have a much clearer idea concerning sampling. Thank you to all at Grad Coach for generously sharing your expertise with students.
Submit a Comment Cancel reply
Your email address will not be published. Required fields are marked *
Save my name, email, and website in this browser for the next time I comment.
- Print Friendly
Have a language expert improve your writing
Run a free plagiarism check in 10 minutes, automatically generate references for free.
- Knowledge Base
- Methodology
- Case Study | Definition, Examples & Methods
Case Study | Definition, Examples & Methods
Published on 5 May 2022 by Shona McCombes . Revised on 30 January 2023.
A case study is a detailed study of a specific subject, such as a person, group, place, event, organisation, or phenomenon. Case studies are commonly used in social, educational, clinical, and business research.
A case study research design usually involves qualitative methods , but quantitative methods are sometimes also used. Case studies are good for describing , comparing, evaluating, and understanding different aspects of a research problem .
Table of contents
When to do a case study, step 1: select a case, step 2: build a theoretical framework, step 3: collect your data, step 4: describe and analyse the case.
A case study is an appropriate research design when you want to gain concrete, contextual, in-depth knowledge about a specific real-world subject. It allows you to explore the key characteristics, meanings, and implications of the case.
Case studies are often a good choice in a thesis or dissertation . They keep your project focused and manageable when you don’t have the time or resources to do large-scale research.
You might use just one complex case study where you explore a single subject in depth, or conduct multiple case studies to compare and illuminate different aspects of your research problem.
Prevent plagiarism, run a free check.
Once you have developed your problem statement and research questions , you should be ready to choose the specific case that you want to focus on. A good case study should have the potential to:
- Provide new or unexpected insights into the subject
- Challenge or complicate existing assumptions and theories
- Propose practical courses of action to resolve a problem
- Open up new directions for future research
Unlike quantitative or experimental research, a strong case study does not require a random or representative sample. In fact, case studies often deliberately focus on unusual, neglected, or outlying cases which may shed new light on the research problem.
If you find yourself aiming to simultaneously investigate and solve an issue, consider conducting action research . As its name suggests, action research conducts research and takes action at the same time, and is highly iterative and flexible.
However, you can also choose a more common or representative case to exemplify a particular category, experience, or phenomenon.
While case studies focus more on concrete details than general theories, they should usually have some connection with theory in the field. This way the case study is not just an isolated description, but is integrated into existing knowledge about the topic. It might aim to:
- Exemplify a theory by showing how it explains the case under investigation
- Expand on a theory by uncovering new concepts and ideas that need to be incorporated
- Challenge a theory by exploring an outlier case that doesn’t fit with established assumptions
To ensure that your analysis of the case has a solid academic grounding, you should conduct a literature review of sources related to the topic and develop a theoretical framework . This means identifying key concepts and theories to guide your analysis and interpretation.
There are many different research methods you can use to collect data on your subject. Case studies tend to focus on qualitative data using methods such as interviews, observations, and analysis of primary and secondary sources (e.g., newspaper articles, photographs, official records). Sometimes a case study will also collect quantitative data .
The aim is to gain as thorough an understanding as possible of the case and its context.
In writing up the case study, you need to bring together all the relevant aspects to give as complete a picture as possible of the subject.
How you report your findings depends on the type of research you are doing. Some case studies are structured like a standard scientific paper or thesis, with separate sections or chapters for the methods , results , and discussion .
Others are written in a more narrative style, aiming to explore the case from various angles and analyse its meanings and implications (for example, by using textual analysis or discourse analysis ).
In all cases, though, make sure to give contextual details about the case, connect it back to the literature and theory, and discuss how it fits into wider patterns or debates.
Cite this Scribbr article
If you want to cite this source, you can copy and paste the citation or click the ‘Cite this Scribbr article’ button to automatically add the citation to our free Reference Generator.
McCombes, S. (2023, January 30). Case Study | Definition, Examples & Methods. Scribbr. Retrieved 14 May 2024, from https://www.scribbr.co.uk/research-methods/case-studies/
Is this article helpful?
Shona McCombes
Other students also liked, correlational research | guide, design & examples, a quick guide to experimental design | 5 steps & examples, descriptive research design | definition, methods & examples.
Participant Selection and Access in Case Study Research
- First Online: 05 January 2019
Cite this chapter
- Zhongyan Wan 4
1550 Accesses
2 Citations
Case study research requires researchers to purposefully select information-rich cases, as they will allow researchers an in-depth understanding of relevant and critical issues under investigation (Patton in Qualitative evaluation and research methods. Sage, Newbury Park, 1990 , Qual Soc Work 1(3):261–283, 2002 ). To gain such insights, purposive sampling is generally believed to contribute to the richness in the range of data collected and help increase the possibilities of uncovering multiple realities (Guba and Lincoln in Handb Qual Res 2(163–194):105, 1994 ). However, dealing with research participants in the case study approach also poses great challenges for novice researchers, as humans can be a difficult factor to control among the variables in research.
This is a preview of subscription content, log in via an institution to check access.
Access this chapter
- Available as EPUB and PDF
- Read on any device
- Instant download
- Own it forever
- Compact, lightweight edition
- Dispatched in 3 to 5 business days
- Free shipping worldwide - see info
Tax calculation will be finalised at checkout
Purchases are for personal use only
Institutional subscriptions
Similar content being viewed by others
Questionable Research Practices in Single-Case Experimental Designs: Examples and Possible Solutions
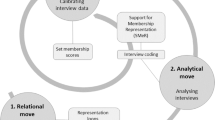
The “qualitative” in qualitative comparative analysis (QCA): research moves, case-intimacy and face-to-face interviews
Alinejad, D. (2011). Mapping homelands through virtual spaces: Transnational embodiment and Iranian diaspora bloggers. Global Networks, 11 (1), 43–62.
Google Scholar
Arcury, T., & Quandt, S. (1999). Participant recruitment for qualitative research: A site-based approach to community research in complex societies. Human Organization, 58 (2), 128–133.
Article Google Scholar
Barbour, R. (2014). Introducing qualitative research: A student’s guide . London: Sage.
Decrop, A. (1999). Qualitative research methods for the study of tourist behaviour. In A. Pizam & Y. Mansfeld. (Eds.), Consumer Behaviour in Travel and Tourism . New York: The Haworth Hospitality Press
Duff, P. (2008). Case study research in applied linguistics . New York: Taylor & Francis.
Feldman, M. S., Bell, J., & Berger, M. T. (2003). Gaining access: A practical and theoretical guide for qualitative researchers . Walnut Creek, CA: Altamira Press.
Gall, M. D., Gall, J. P., & Borg, W. T. (2003). Educational research (7th ed.). White Plains, NY: Pearson Education.
Gerring, J. (2004). What is a case study and what is it good for? American Political Science Review, 98 (02), 341–354.
Guba, E. G., & Lincoln, Y. S. (1994). Competing paradigms in qualitative research. Handbook of Qualitative Research, 2 (163–194), 105.
Gummesson, E. (2000). Qualitative methods in management research . Thousand Oaks, CA: Sage.
Hamilton, R. J., & Bowers, B. J. (2006). Internet recruitment and e-mail interviews in qualitative studies. Qualitative Health Research, 16 (6), 821–835.
Hancock, D. R., & Algozzine, B. (2015). Doing case study research: A practical guide for beginning researchers . New York: Teachers College Press.
Hewson, C. (2014). Qualitative approaches in Internet-mediated research: Opportunities, issues, possibilities. The Oxford Handbook of Qualitative Research , 423–452.
Johl, S. K., & Renganathan, S. (2010). Strategies for gaining access in doing fieldwork: Reflection of two researchers. The Electronic Journal of Business Research Methods, 8 (1), 42–50.
Kothari, C. R. (2004). Research methodology: Methods and techniques . India: New Age International.
Lee, R. (1993). Doing research on sensitive topics . London: Sage.
Merriam, S. (2009). Case study research in education: A qualitative approach . San Francisco: Jossey-Bass.
Mikecz, R. (2012). Interviewing elites addressing methodological issues. Qualitative Inquiry, 18 (6), 482–493.
Miles, M. B., & Huberman, A. M. (1994). Qualitative data analysis: An expanded sourcebook . Thousand Oaks, CA: Sage.
Noy, C. (2008). Sampling knowledge: The hermeneutics of snowball sampling in qualitative research. International Journal of Social Research Methodology, 11 (4), 327–344.
Okumus, F., Altinay, L., & Roper, A. (2007). Gaining access for research: Reflections from experience. Annals of Tourism Research, 34 (1), 7–26.
Patton, M. Q. (1990). Qualitative evaluation and research methods (2nd ed.). Newbury Park, CA: Sage.
Patton, M. Q. (2002). Two decades of developments in qualitative inquiry a personal, experiential perspective. Qualitative Social Work, 1 (3), 261–283.
Punch, K. F. (1998). Introduction to social research . London: Sage.
Seawright, J., & Gerring, J. (2008). Case selection techniques in case study research a menu of qualitative and quantitative options. Political Research Quarterly, 61 (2), 294–308.
Silverman, D. (Ed.). (2016). Qualitative research . London: Sage.
Thomas, G. (2015). How to do your case study . Thousand Oaks, CA: Sage.
Wan, Z. Y. (2016). College teacher beliefs and practices in computer-assisted language learning classrooms in China . Shanghai: Shanghai Jiaotong University Press.
Willis, J. W., Jost, M., & Nilakanta, R. (2007). Foundations of qualitative research: Interpretive and critical approaches . London: Sage.
Book Google Scholar
Wilmot, A. (2005). Designing sampling strategies for qualitative social research: With particular reference to the Office for National Statistics’ Qualitative Respondent Register. Survey Methodology Bulletin-office for National Statistics, 56, 53.
Yin, R. K. (2009). Case study research: Design and methods (4th ed.). Thousand Oaks, CA: Sage.
Yin, R. K. (2013). Case study research: Design and methods (5th ed.). London: Sage publications.
Download references
Author information
Authors and affiliations.
Foreign Language Department, China Pharmaceutical University, Nanjing, China
Zhongyan Wan
You can also search for this author in PubMed Google Scholar
Corresponding author
Correspondence to Zhongyan Wan .
Editor information
Editors and affiliations.
Faculty of Education, Beijing Normal University, Beijing, China
Kwok Kuen Tsang
Faculty of Social Sciences, University of Stavanger, Stavanger, Norway
School of Humanities, Southeast University, Nanjing, Jiangsu, China
Rights and permissions
Reprints and permissions
Copyright information
© 2019 Springer Nature Singapore Pte Ltd.
About this chapter
Wan, Z. (2019). Participant Selection and Access in Case Study Research. In: Tsang, K., Liu, D., Hong, Y. (eds) Challenges and Opportunities in Qualitative Research. Springer, Singapore. https://doi.org/10.1007/978-981-13-5811-1_5
Download citation
DOI : https://doi.org/10.1007/978-981-13-5811-1_5
Published : 05 January 2019
Publisher Name : Springer, Singapore
Print ISBN : 978-981-13-5810-4
Online ISBN : 978-981-13-5811-1
eBook Packages : Social Sciences Social Sciences (R0)
Share this chapter
Anyone you share the following link with will be able to read this content:
Sorry, a shareable link is not currently available for this article.
Provided by the Springer Nature SharedIt content-sharing initiative
- Publish with us
Policies and ethics
- Find a journal
- Track your research
Statistical Sampling Case Study
To learn about sampling techniques in social science research, students practice tackling a real-world research problem through discussing a hypothetical case..
- To enable students to understand the benefits and drawbacks of various sampling techniques.
- To provide students with experience designing sampling methods to address a real-world research problem.
Class: Sociology 128: Models of Social Science Research
Introduction/Background: This course introduces sociology students to concepts and strategies in social science research. In week five, students learn about nonrandom and random sampling techniques (snowball sampling, simple random sampling, etc.). In discussion section later that week, students apply this knowledge to a hypothetical case study where a researcher aims to study the experiences of homeless people in the United States.
Before Class:
Students learned about the pros and cons of various sampling techniques in lecture.
During Class:
- In discussion section, students received a handout about various types of sampling techniques, as well as a hypothetical research scenario about researching a population of homeless people in New York City. The instructor and students reviewed the sampling techniques they had learned, including what types of social science research questions each technique would enable researchers to answer.
- Students were then broken up into groups of 2-3. Guided by four questions on the handout, students analyzed the research problem and discussed within their groups the pros and cons of various sampling techniques. The instructor moved between the groups and provided feedback as students were deciding how to answer each question.
- Once each group determined how they would approach the problem, they shared out their choice with the class. The class discussed the benefits and drawbacks to each group's choices.
Students left class with a deep understanding of a hypothetical research scenario and the various considerations they would have to take into account when deciding how to sample a population. They would later use this knowledge in developing their group projects at the end of the semester.
The research scenario prompt, attached
Submitted by Matthew Clair, Teaching Fellow, Harvard Department of Sociology
- Privacy Policy

Home » Case Study – Methods, Examples and Guide
Case Study – Methods, Examples and Guide
Table of Contents

A case study is a research method that involves an in-depth examination and analysis of a particular phenomenon or case, such as an individual, organization, community, event, or situation.
It is a qualitative research approach that aims to provide a detailed and comprehensive understanding of the case being studied. Case studies typically involve multiple sources of data, including interviews, observations, documents, and artifacts, which are analyzed using various techniques, such as content analysis, thematic analysis, and grounded theory. The findings of a case study are often used to develop theories, inform policy or practice, or generate new research questions.
Types of Case Study
Types and Methods of Case Study are as follows:
Single-Case Study
A single-case study is an in-depth analysis of a single case. This type of case study is useful when the researcher wants to understand a specific phenomenon in detail.
For Example , A researcher might conduct a single-case study on a particular individual to understand their experiences with a particular health condition or a specific organization to explore their management practices. The researcher collects data from multiple sources, such as interviews, observations, and documents, and uses various techniques to analyze the data, such as content analysis or thematic analysis. The findings of a single-case study are often used to generate new research questions, develop theories, or inform policy or practice.
Multiple-Case Study
A multiple-case study involves the analysis of several cases that are similar in nature. This type of case study is useful when the researcher wants to identify similarities and differences between the cases.
For Example, a researcher might conduct a multiple-case study on several companies to explore the factors that contribute to their success or failure. The researcher collects data from each case, compares and contrasts the findings, and uses various techniques to analyze the data, such as comparative analysis or pattern-matching. The findings of a multiple-case study can be used to develop theories, inform policy or practice, or generate new research questions.
Exploratory Case Study
An exploratory case study is used to explore a new or understudied phenomenon. This type of case study is useful when the researcher wants to generate hypotheses or theories about the phenomenon.
For Example, a researcher might conduct an exploratory case study on a new technology to understand its potential impact on society. The researcher collects data from multiple sources, such as interviews, observations, and documents, and uses various techniques to analyze the data, such as grounded theory or content analysis. The findings of an exploratory case study can be used to generate new research questions, develop theories, or inform policy or practice.
Descriptive Case Study
A descriptive case study is used to describe a particular phenomenon in detail. This type of case study is useful when the researcher wants to provide a comprehensive account of the phenomenon.
For Example, a researcher might conduct a descriptive case study on a particular community to understand its social and economic characteristics. The researcher collects data from multiple sources, such as interviews, observations, and documents, and uses various techniques to analyze the data, such as content analysis or thematic analysis. The findings of a descriptive case study can be used to inform policy or practice or generate new research questions.
Instrumental Case Study
An instrumental case study is used to understand a particular phenomenon that is instrumental in achieving a particular goal. This type of case study is useful when the researcher wants to understand the role of the phenomenon in achieving the goal.
For Example, a researcher might conduct an instrumental case study on a particular policy to understand its impact on achieving a particular goal, such as reducing poverty. The researcher collects data from multiple sources, such as interviews, observations, and documents, and uses various techniques to analyze the data, such as content analysis or thematic analysis. The findings of an instrumental case study can be used to inform policy or practice or generate new research questions.
Case Study Data Collection Methods
Here are some common data collection methods for case studies:
Interviews involve asking questions to individuals who have knowledge or experience relevant to the case study. Interviews can be structured (where the same questions are asked to all participants) or unstructured (where the interviewer follows up on the responses with further questions). Interviews can be conducted in person, over the phone, or through video conferencing.
Observations
Observations involve watching and recording the behavior and activities of individuals or groups relevant to the case study. Observations can be participant (where the researcher actively participates in the activities) or non-participant (where the researcher observes from a distance). Observations can be recorded using notes, audio or video recordings, or photographs.
Documents can be used as a source of information for case studies. Documents can include reports, memos, emails, letters, and other written materials related to the case study. Documents can be collected from the case study participants or from public sources.
Surveys involve asking a set of questions to a sample of individuals relevant to the case study. Surveys can be administered in person, over the phone, through mail or email, or online. Surveys can be used to gather information on attitudes, opinions, or behaviors related to the case study.
Artifacts are physical objects relevant to the case study. Artifacts can include tools, equipment, products, or other objects that provide insights into the case study phenomenon.
How to conduct Case Study Research
Conducting a case study research involves several steps that need to be followed to ensure the quality and rigor of the study. Here are the steps to conduct case study research:
- Define the research questions: The first step in conducting a case study research is to define the research questions. The research questions should be specific, measurable, and relevant to the case study phenomenon under investigation.
- Select the case: The next step is to select the case or cases to be studied. The case should be relevant to the research questions and should provide rich and diverse data that can be used to answer the research questions.
- Collect data: Data can be collected using various methods, such as interviews, observations, documents, surveys, and artifacts. The data collection method should be selected based on the research questions and the nature of the case study phenomenon.
- Analyze the data: The data collected from the case study should be analyzed using various techniques, such as content analysis, thematic analysis, or grounded theory. The analysis should be guided by the research questions and should aim to provide insights and conclusions relevant to the research questions.
- Draw conclusions: The conclusions drawn from the case study should be based on the data analysis and should be relevant to the research questions. The conclusions should be supported by evidence and should be clearly stated.
- Validate the findings: The findings of the case study should be validated by reviewing the data and the analysis with participants or other experts in the field. This helps to ensure the validity and reliability of the findings.
- Write the report: The final step is to write the report of the case study research. The report should provide a clear description of the case study phenomenon, the research questions, the data collection methods, the data analysis, the findings, and the conclusions. The report should be written in a clear and concise manner and should follow the guidelines for academic writing.
Examples of Case Study
Here are some examples of case study research:
- The Hawthorne Studies : Conducted between 1924 and 1932, the Hawthorne Studies were a series of case studies conducted by Elton Mayo and his colleagues to examine the impact of work environment on employee productivity. The studies were conducted at the Hawthorne Works plant of the Western Electric Company in Chicago and included interviews, observations, and experiments.
- The Stanford Prison Experiment: Conducted in 1971, the Stanford Prison Experiment was a case study conducted by Philip Zimbardo to examine the psychological effects of power and authority. The study involved simulating a prison environment and assigning participants to the role of guards or prisoners. The study was controversial due to the ethical issues it raised.
- The Challenger Disaster: The Challenger Disaster was a case study conducted to examine the causes of the Space Shuttle Challenger explosion in 1986. The study included interviews, observations, and analysis of data to identify the technical, organizational, and cultural factors that contributed to the disaster.
- The Enron Scandal: The Enron Scandal was a case study conducted to examine the causes of the Enron Corporation’s bankruptcy in 2001. The study included interviews, analysis of financial data, and review of documents to identify the accounting practices, corporate culture, and ethical issues that led to the company’s downfall.
- The Fukushima Nuclear Disaster : The Fukushima Nuclear Disaster was a case study conducted to examine the causes of the nuclear accident that occurred at the Fukushima Daiichi Nuclear Power Plant in Japan in 2011. The study included interviews, analysis of data, and review of documents to identify the technical, organizational, and cultural factors that contributed to the disaster.
Application of Case Study
Case studies have a wide range of applications across various fields and industries. Here are some examples:
Business and Management
Case studies are widely used in business and management to examine real-life situations and develop problem-solving skills. Case studies can help students and professionals to develop a deep understanding of business concepts, theories, and best practices.
Case studies are used in healthcare to examine patient care, treatment options, and outcomes. Case studies can help healthcare professionals to develop critical thinking skills, diagnose complex medical conditions, and develop effective treatment plans.
Case studies are used in education to examine teaching and learning practices. Case studies can help educators to develop effective teaching strategies, evaluate student progress, and identify areas for improvement.
Social Sciences
Case studies are widely used in social sciences to examine human behavior, social phenomena, and cultural practices. Case studies can help researchers to develop theories, test hypotheses, and gain insights into complex social issues.
Law and Ethics
Case studies are used in law and ethics to examine legal and ethical dilemmas. Case studies can help lawyers, policymakers, and ethical professionals to develop critical thinking skills, analyze complex cases, and make informed decisions.
Purpose of Case Study
The purpose of a case study is to provide a detailed analysis of a specific phenomenon, issue, or problem in its real-life context. A case study is a qualitative research method that involves the in-depth exploration and analysis of a particular case, which can be an individual, group, organization, event, or community.
The primary purpose of a case study is to generate a comprehensive and nuanced understanding of the case, including its history, context, and dynamics. Case studies can help researchers to identify and examine the underlying factors, processes, and mechanisms that contribute to the case and its outcomes. This can help to develop a more accurate and detailed understanding of the case, which can inform future research, practice, or policy.
Case studies can also serve other purposes, including:
- Illustrating a theory or concept: Case studies can be used to illustrate and explain theoretical concepts and frameworks, providing concrete examples of how they can be applied in real-life situations.
- Developing hypotheses: Case studies can help to generate hypotheses about the causal relationships between different factors and outcomes, which can be tested through further research.
- Providing insight into complex issues: Case studies can provide insights into complex and multifaceted issues, which may be difficult to understand through other research methods.
- Informing practice or policy: Case studies can be used to inform practice or policy by identifying best practices, lessons learned, or areas for improvement.
Advantages of Case Study Research
There are several advantages of case study research, including:
- In-depth exploration: Case study research allows for a detailed exploration and analysis of a specific phenomenon, issue, or problem in its real-life context. This can provide a comprehensive understanding of the case and its dynamics, which may not be possible through other research methods.
- Rich data: Case study research can generate rich and detailed data, including qualitative data such as interviews, observations, and documents. This can provide a nuanced understanding of the case and its complexity.
- Holistic perspective: Case study research allows for a holistic perspective of the case, taking into account the various factors, processes, and mechanisms that contribute to the case and its outcomes. This can help to develop a more accurate and comprehensive understanding of the case.
- Theory development: Case study research can help to develop and refine theories and concepts by providing empirical evidence and concrete examples of how they can be applied in real-life situations.
- Practical application: Case study research can inform practice or policy by identifying best practices, lessons learned, or areas for improvement.
- Contextualization: Case study research takes into account the specific context in which the case is situated, which can help to understand how the case is influenced by the social, cultural, and historical factors of its environment.
Limitations of Case Study Research
There are several limitations of case study research, including:
- Limited generalizability : Case studies are typically focused on a single case or a small number of cases, which limits the generalizability of the findings. The unique characteristics of the case may not be applicable to other contexts or populations, which may limit the external validity of the research.
- Biased sampling: Case studies may rely on purposive or convenience sampling, which can introduce bias into the sample selection process. This may limit the representativeness of the sample and the generalizability of the findings.
- Subjectivity: Case studies rely on the interpretation of the researcher, which can introduce subjectivity into the analysis. The researcher’s own biases, assumptions, and perspectives may influence the findings, which may limit the objectivity of the research.
- Limited control: Case studies are typically conducted in naturalistic settings, which limits the control that the researcher has over the environment and the variables being studied. This may limit the ability to establish causal relationships between variables.
- Time-consuming: Case studies can be time-consuming to conduct, as they typically involve a detailed exploration and analysis of a specific case. This may limit the feasibility of conducting multiple case studies or conducting case studies in a timely manner.
- Resource-intensive: Case studies may require significant resources, including time, funding, and expertise. This may limit the ability of researchers to conduct case studies in resource-constrained settings.
About the author
Muhammad Hassan
Researcher, Academic Writer, Web developer
You may also like

Questionnaire – Definition, Types, and Examples

Observational Research – Methods and Guide

Quantitative Research – Methods, Types and...

Qualitative Research Methods

Explanatory Research – Types, Methods, Guide

Survey Research – Types, Methods, Examples
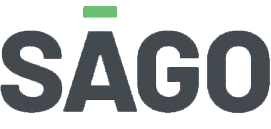
What We Offer
With a comprehensive suite of qualitative and quantitative capabilities and 55 years of experience in the industry, Sago powers insights through adaptive solutions.
- Recruitment
- Communities
- Methodify® Automated research
- QualBoard® Digital Discussions
- QualMeeting® Digital Interviews
- Global Qualitative
- Global Quantitative
- In-Person Facilities
- Research Consulting
- Europe Solutions
- Neuromarketing Tools
- Trial & Jury Consulting
Who We Serve
Form deeper customer connections and make the process of answering your business questions easier. Sago delivers unparalleled access to the audiences you need through adaptive solutions and a consultative approach.
- Consumer Packaged Goods
- Financial Services
- Media Technology
- Medical Device Manufacturing
- Marketing Research
With a 55-year legacy of impact, Sago has proven we have what it takes to be a long-standing industry leader and partner. We continually advance our range of expertise to provide our clients with the highest level of confidence.
- Global Offices
- Partnerships & Certifications
- News & Media
- Researcher Events
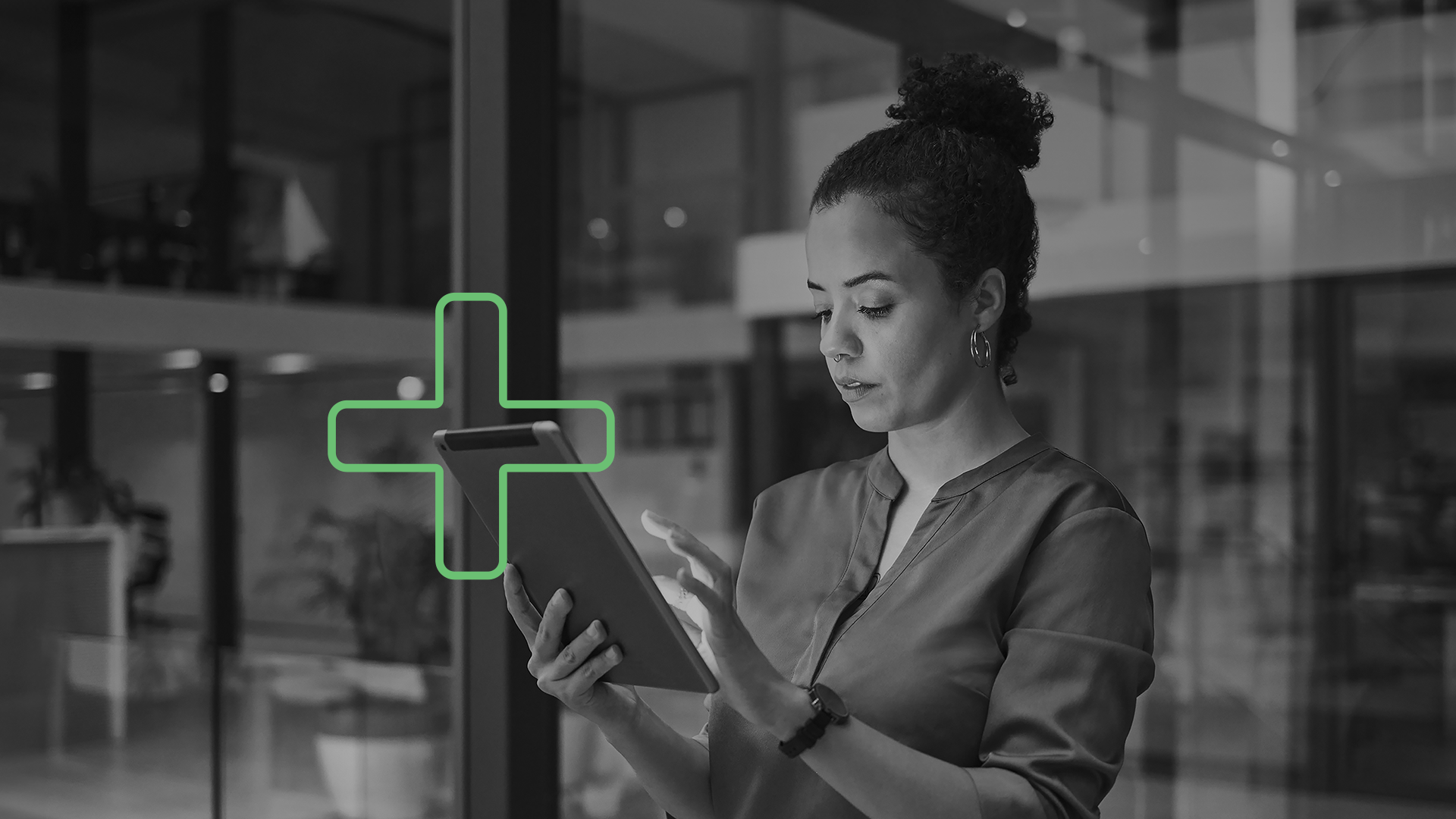
Sago Announces Launch of Sago Health to Elevate Healthcare Research
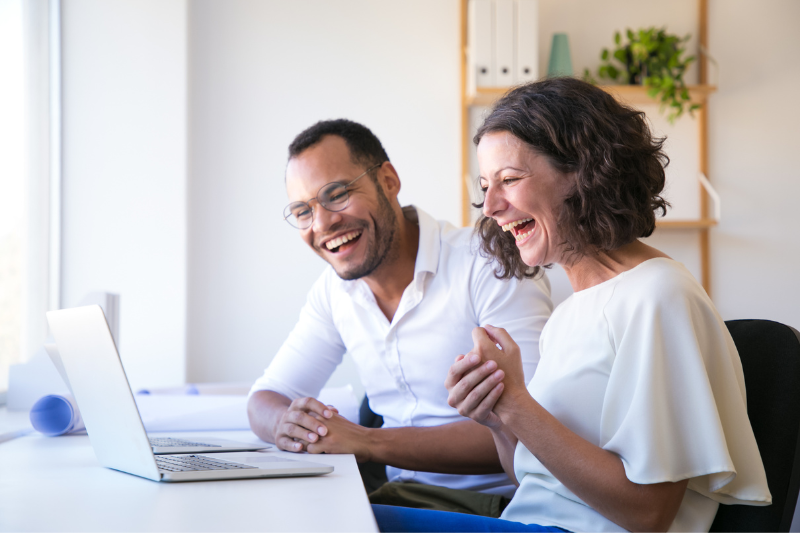
Sago Launches AI Video Summaries on QualBoard to Streamline Data Synthesis
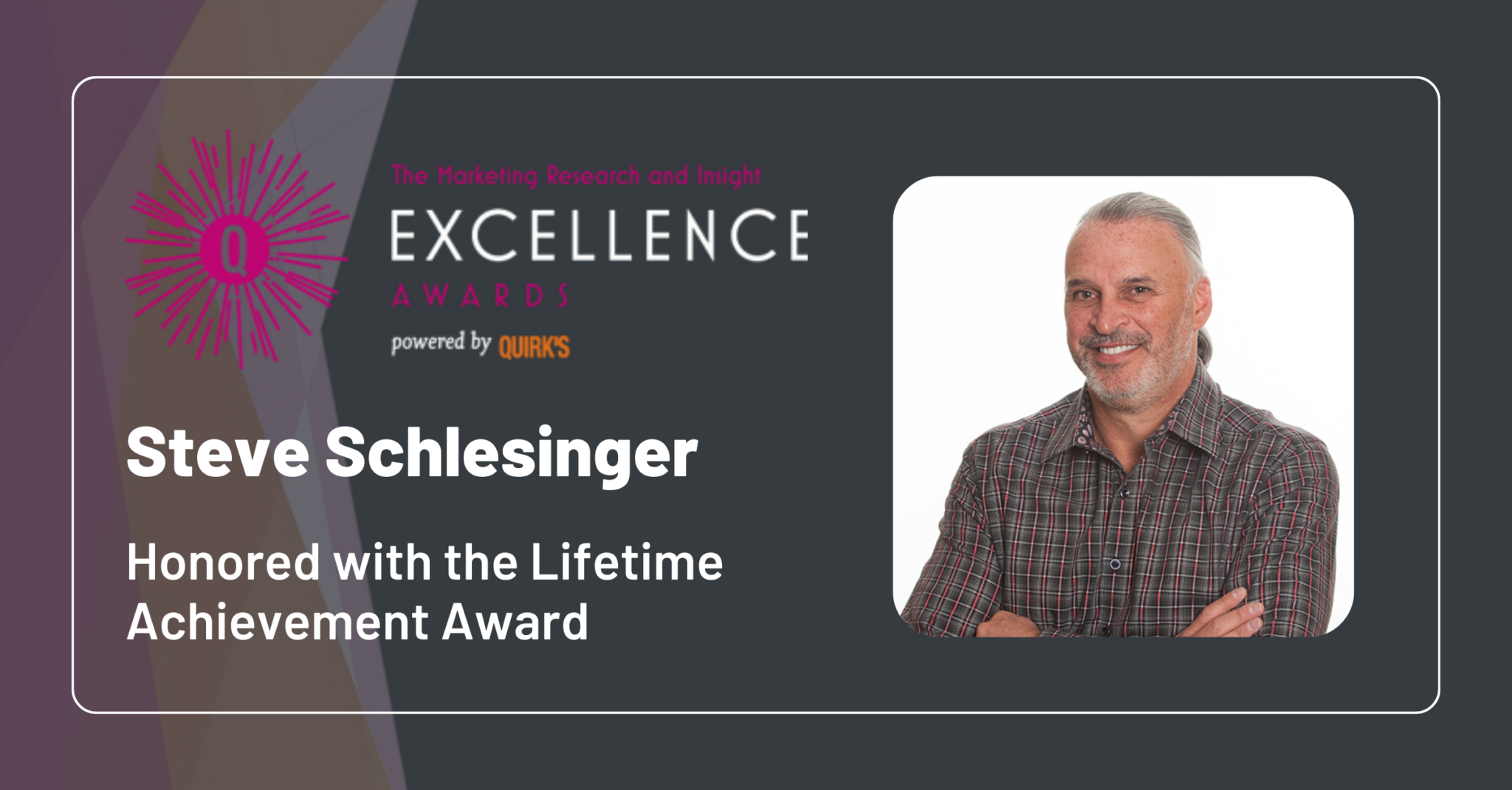
Sago Executive Chairman Steve Schlesinger to Receive Quirk’s Lifetime Achievement Award
Drop into your new favorite insights rabbit hole and explore content created by the leading minds in market research.
- Case Studies
- Knowledge Kit

Women’s Health: Addressing the Elephant in the Room

Guide: Respondent Engagement Playbook
Get in touch

- Account Logins

Different Types of Sampling Techniques in Qualitative Research
- Resources , Blog

Key Takeaways:
- Sampling techniques in qualitative research include purposive, convenience, snowball, and theoretical sampling.
- Choosing the right sampling technique significantly impacts the accuracy and reliability of the research results.
- It’s crucial to consider the potential impact on the bias, sample diversity, and generalizability when choosing a sampling technique for your qualitative research.
Qualitative research seeks to understand social phenomena from the perspective of those experiencing them. It involves collecting non-numerical data such as interviews, observations, and written documents to gain insights into human experiences, attitudes, and behaviors. While qualitative research can provide rich and nuanced insights, the accuracy and generalizability of findings depend on the quality of the sampling process. Sampling is a critical component of qualitative research as it involves selecting a group of participants who can provide valuable insights into the research questions.
This article explores different types of sampling techniques used in qualitative research. First, we’ll provide a comprehensive overview of four standard sampling techniques used in qualitative research. and then compare and contrast these techniques to provide guidance on choosing the most appropriate method for a particular study. Additionally, you’ll find best practices for sampling and learn about ethical considerations researchers need to consider in selecting a sample. Overall, this article aims to help researchers conduct effective and high-quality sampling in qualitative research.
In this Article:
- Purposive Sampling
- Convenience Sampling
- Snowball Sampling
- Theoretical Sampling
Factors to Consider When Choosing a Sampling Technique
Practical approaches to sampling: recommended practices, final thoughts, get expert guidance on your sample needs.
Want expert input on the best sampling technique for your qualitative research project? Book a consultation for trusted advice.
Request a consultation
4 Types of Sampling Techniques and Their Applications
Sampling is a crucial aspect of qualitative research as it determines the representativeness and credibility of the data collected. Several sampling techniques are used in qualitative research, each with strengths and weaknesses. In this section, let’s explore four standard sampling techniques used in qualitative research: purposive sampling, convenience sampling, snowball sampling, and theoretical sampling. We’ll break down the definition of each technique, when to use it, and its advantages and disadvantages.
1. Purposive Sampling
Purposive sampling, or judgmental sampling, is a non-probability sampling technique commonly used in qualitative research. In purposive sampling, researchers intentionally select participants with specific characteristics or unique experiences related to the research question. The goal is to identify and recruit participants who can provide rich and diverse data to enhance the research findings.
Purposive sampling is used when researchers seek to identify individuals or groups with particular knowledge, skills, or experiences relevant to the research question. For instance, in a study examining the experiences of cancer patients undergoing chemotherapy, purposive sampling may be used to recruit participants who have undergone chemotherapy in the past year. Researchers can better understand the phenomenon under investigation by selecting individuals with relevant backgrounds.
Purposive Sampling: Strengths and Weaknesses
Purposive sampling is a powerful tool for researchers seeking to select participants who can provide valuable insight into their research question. This method is advantageous when studying groups with technical characteristics or experiences where a random selection of participants may yield different results.
One of the main advantages of purposive sampling is the ability to improve the quality and accuracy of data collected by selecting participants most relevant to the research question. This approach also enables researchers to collect data from diverse participants with unique perspectives and experiences related to the research question.
However, researchers should also be aware of potential bias when using purposive sampling. The researcher’s judgment may influence the selection of participants, resulting in a biased sample that does not accurately represent the broader population. Another disadvantage is that purposive sampling may not be representative of the more general population, which limits the generalizability of the findings. To guarantee the accuracy and dependability of data obtained through purposive sampling, researchers must provide a clear and transparent justification of their selection criteria and sampling approach. This entails outlining the specific characteristics or experiences required for participants to be included in the study and explaining the rationale behind these criteria. This level of transparency not only helps readers to evaluate the validity of the findings, but also enhances the replicability of the research.
2. Convenience Sampling
When time and resources are limited, researchers may opt for convenience sampling as a quick and cost-effective way to recruit participants. In this non-probability sampling technique, participants are selected based on their accessibility and willingness to participate rather than their suitability for the research question. Qualitative research often uses this approach to generate various perspectives and experiences.
During the COVID-19 pandemic, convenience sampling was a valuable method for researchers to collect data quickly and efficiently from participants who were easily accessible and willing to participate. For example, in a study examining the experiences of university students during the pandemic, convenience sampling allowed researchers to recruit students who were available and willing to share their experiences quickly. While the pandemic may be over, convenience sampling during this time highlights its value in urgent situations where time and resources are limited.
Convenience Sampling: Strengths and Weaknesses
Convenience sampling offers several advantages to researchers, including its ease of implementation and cost-effectiveness. This technique allows researchers to quickly and efficiently recruit participants without spending time and resources identifying and contacting potential participants. Furthermore, convenience sampling can result in a diverse pool of participants, as individuals from various backgrounds and experiences may be more likely to participate.
While convenience sampling has the advantage of being efficient, researchers need to acknowledge its limitations. One of the primary drawbacks of convenience sampling is that it is susceptible to selection bias. Participants who are more easily accessible may not be representative of the broader population, which can limit the generalizability of the findings. Furthermore, convenience sampling may lead to issues with the reliability of the results, as it may not be possible to replicate the study using the same sample or a similar one.
To mitigate these limitations, researchers should carefully define the population of interest and ensure the sample is drawn from that population. For instance, if a study is investigating the experiences of individuals with a particular medical condition, researchers can recruit participants from specialized clinics or support groups for that condition. Researchers can also use statistical techniques such as stratified sampling or weighting to adjust for potential biases in the sample.
3. Snowball Sampling
Snowball sampling, also called referral sampling, is a unique approach researchers use to recruit participants in qualitative research. The technique involves identifying a few initial participants who meet the eligibility criteria and asking them to refer others they know who also fit the requirements. The sample size grows as referrals are added, creating a chain-like structure.
Snowball sampling enables researchers to reach out to individuals who may be hard to locate through traditional sampling methods, such as members of marginalized or hidden communities. For instance, in a study examining the experiences of undocumented immigrants, snowball sampling may be used to identify and recruit participants through referrals from other undocumented immigrants.
Snowball Sampling: Strengths and Weaknesses
Snowball sampling can produce in-depth and detailed data from participants with common characteristics or experiences. Since referrals are made within a network of individuals who share similarities, researchers can gain deep insights into a specific group’s attitudes, behaviors, and perspectives.
4. Theoretical Sampling
Theoretical sampling is a sophisticated and strategic technique that can help researchers develop more in-depth and nuanced theories from their data. Instead of selecting participants based on convenience or accessibility, researchers using theoretical sampling choose participants based on their potential to contribute to the emerging themes and concepts in the data. This approach allows researchers to refine their research question and theory based on the data they collect rather than forcing their data to fit a preconceived idea.
Theoretical sampling is used when researchers conduct grounded theory research and have developed an initial theory or conceptual framework. In a study examining cancer survivors’ experiences, for example, theoretical sampling may be used to identify and recruit participants who can provide new insights into the coping strategies of survivors.
Theoretical Sampling: Strengths and Weaknesses
One of the significant advantages of theoretical sampling is that it allows researchers to refine their research question and theory based on emerging data. This means the research can be highly targeted and focused, leading to a deeper understanding of the phenomenon being studied. Additionally, theoretical sampling can generate rich and in-depth data, as participants are selected based on their potential to provide new insights into the research question.
Participants are selected based on their perceived ability to offer new perspectives on the research question. This means specific perspectives or experiences may be overrepresented in the sample, leading to an incomplete understanding of the phenomenon being studied. Additionally, theoretical sampling can be time-consuming and resource-intensive, as researchers must continuously analyze the data and recruit new participants.
To mitigate the potential for bias, researchers can take several steps. One way to reduce bias is to use a diverse team of researchers to analyze the data and make participant selection decisions. Having multiple perspectives and backgrounds can help prevent researchers from unconsciously selecting participants who fit their preconceived notions or biases.
Another solution would be to use reflexive sampling. Reflexive sampling involves selecting participants aware of the research process and provides insights into how their biases and experiences may influence their perspectives. By including participants who are reflexive about their subjectivity, researchers can generate more nuanced and self-aware findings.
Choosing the proper sampling technique is one of the most critical decisions a researcher makes when conducting a study. The preferred method can significantly impact the accuracy and reliability of the research results.
For instance, purposive sampling provides a more targeted and specific sample, which helps to answer research questions related to that particular population or phenomenon. However, this approach may also introduce bias by limiting the diversity of the sample.
Conversely, convenience sampling may offer a more diverse sample regarding demographics and backgrounds but may also introduce bias by selecting more willing or available participants.
Snowball sampling may help study hard-to-reach populations, but it can also limit the sample’s diversity as participants are selected based on their connections to existing participants.
Theoretical sampling may offer an opportunity to refine the research question and theory based on emerging data, but it can also be time-consuming and resource-intensive.
Additionally, the choice of sampling technique can impact the generalizability of the research findings. Therefore, it’s crucial to consider the potential impact on the bias, sample diversity, and generalizability when choosing a sampling technique. By doing so, researchers can select the most appropriate method for their research question and ensure the validity and reliability of their findings.
Tips for Selecting Participants
When selecting participants for a qualitative research study, it is crucial to consider the research question and the purpose of the study. In addition, researchers should identify the specific characteristics or criteria they seek in their sample and select participants accordingly.
One helpful tip for selecting participants is to use a pre-screening process to ensure potential participants meet the criteria for inclusion in the study. Another technique is using multiple recruitment methods to ensure the sample is diverse and representative of the studied population.
Ensuring Diversity in Samples
Diversity in the sample is important to ensure the study’s findings apply to a wide range of individuals and situations. One way to ensure diversity is to use stratified sampling, which involves dividing the population into subgroups and selecting participants from each subset. This helps establish that the sample is representative of the larger population.
Maintaining Ethical Considerations
When selecting participants for a qualitative research study, it is essential to ensure ethical considerations are taken into account. Researchers must ensure participants are fully informed about the study and provide their voluntary consent to participate. They must also ensure participants understand their rights and that their confidentiality and privacy will be protected.
A qualitative research study’s success hinges on its sampling technique’s effectiveness. The choice of sampling technique must be guided by the research question, the population being studied, and the purpose of the study. Whether purposive, convenience, snowball, or theoretical sampling, the primary goal is to ensure the validity and reliability of the study’s findings.
By thoughtfully weighing the pros and cons of each sampling technique, researchers can make informed decisions that lead to more reliable and accurate results. In conclusion, carefully selecting a sampling technique is integral to the success of a qualitative research study, and a thorough understanding of the available options can make all the difference in achieving high-quality research outcomes.
If you’re interested in improving your research and sampling methods, Sago offers a variety of solutions. Our qualitative research platforms, such as QualBoard and QualMeeting, can assist you in conducting research studies with precision and efficiency. Our robust global panel and recruitment options help you reach the right people. We also offer qualitative and quantitative research services to meet your research needs. Contact us today to learn more about how we can help improve your research outcomes.
Find the Right Sample for Your Qualitative Research
Trust our team to recruit the participants you need using the appropriate techniques. Book a consultation with our team to get started .

Hottest Trends in Market Research Revealed

The Hidden Truths of Women’s Health Revealed

Report: Perceptions of Women’s Health

A New Generation Is Born: Meet Gen C

OnDemand: Breaking the Silence on Women’s Health: From Perception to Truth
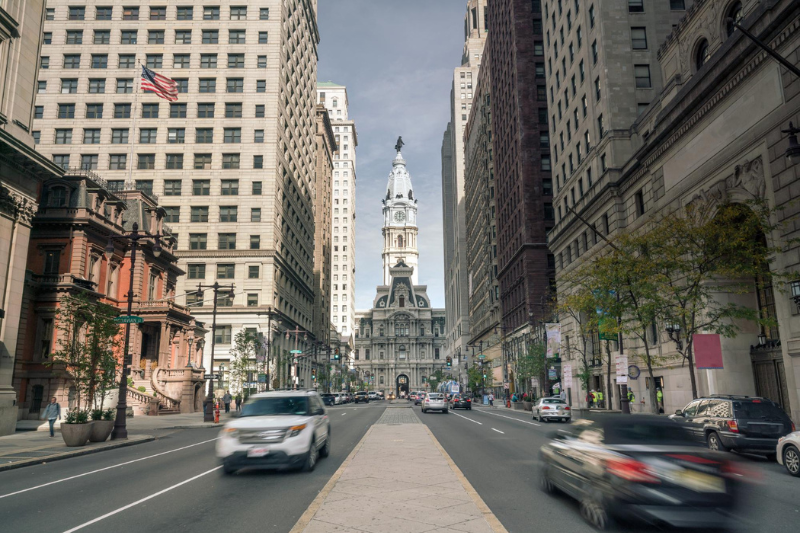
The Swing Voter Project Pennsylvania: April 2024
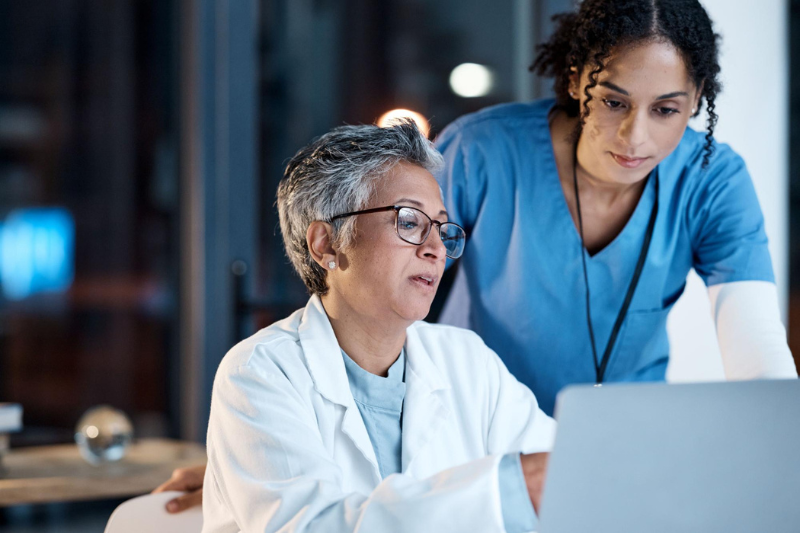
Revolutionizing Healthcare Research: A Q&A With Industry Experts
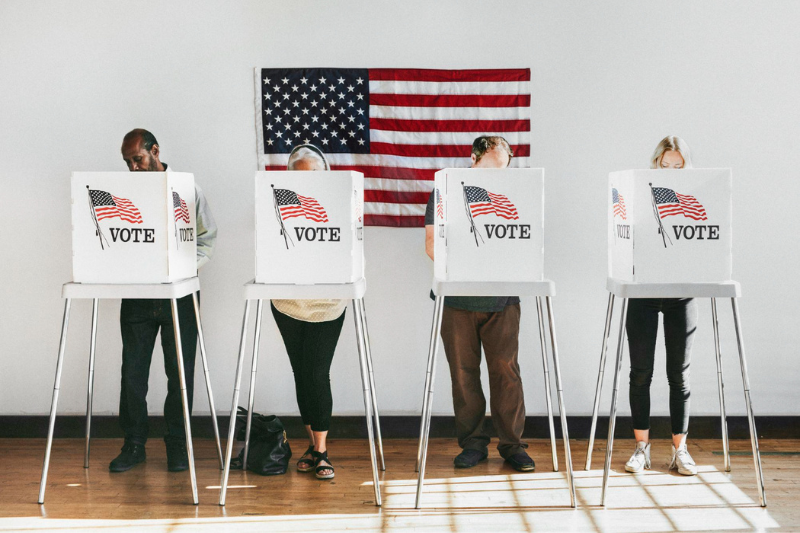
The Deciders April 2024: Michigan Voters in Union Households
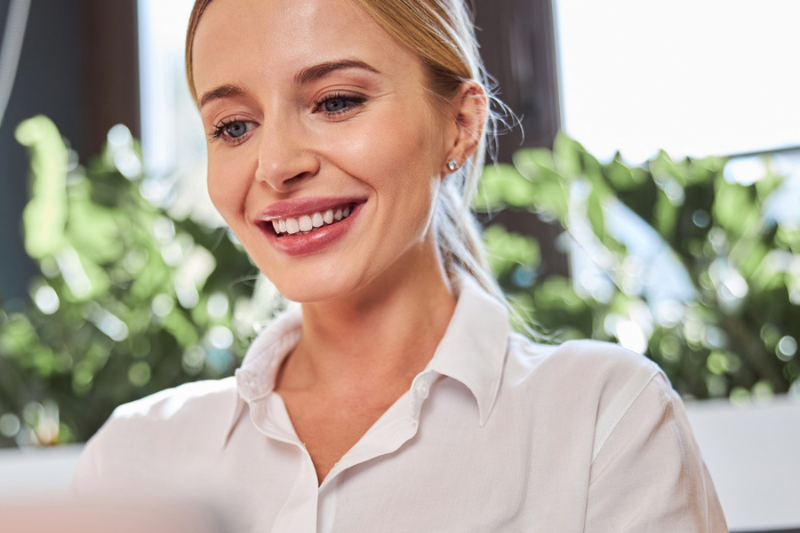
OnDemand: Navigating the Dynamic Future of Qualitative Research
Take a deep dive into your favorite market research topics
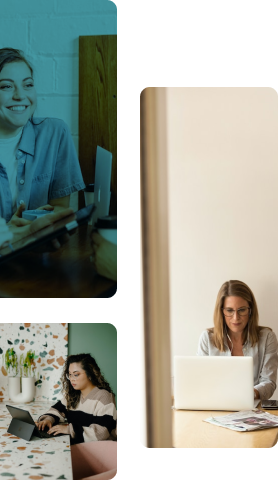
How can we help support you and your research needs?
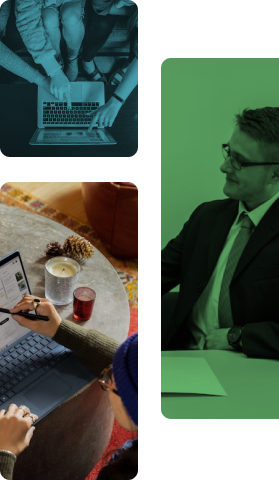
BEFORE YOU GO
Have you considered how to harness AI in your research process? Check out our on-demand webinar for everything you need to know


A .gov website belongs to an official government organization in the United States.
A lock ( ) or https:// means you've safely connected to the .gov website. Share sensitive information only on official, secure websites.
- Training & Resources
- Field Materials
- Interactive Map
Sampling Methodology
At a glance.
CASPER uses a two-stage cluster sampling methodology. In the first stage, clusters (traditionally 30) are selected with a probability proportional to the estimated number of households in the clusters. A cluster is a non-overlapping section in a geographic area with a known number of households. In the second stage, interview teams use systematic random sampling to select seven households from each of the selected clusters.
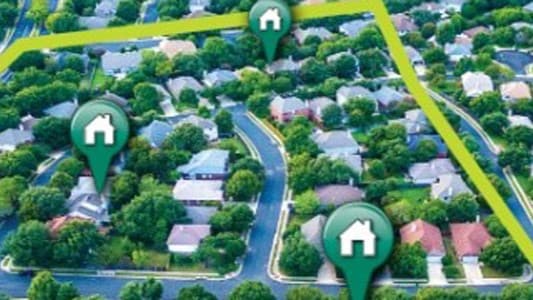
First stage sampling
Selecting a casper sample.
Selecting a CASPER sample requires a list of all clusters within your sampling frame. This includes the number of households within each cluster. U.S. Census blocks are most commonly used.
You can download this information from the Census website or population-based files within Geographic Information System (GIS) software.
Number each household by assigning each cluster with a cumulative sum of the number of households. Finally, select 30 clusters by using a random number generator to select 30 numbers. The numbers should be between one and the total number of households within your sampling frame. Select the entire cluster in which that random number (i.e., household) is located.
Choose all clusters without substitution. This means that clusters originally selected are those that are assessed with no changes or modifications. Any departure from this design (30×7 cluster sampling) is not considered a CASPER. In situations where the 30×7 design may not be feasible or ideal, and a change in methodology is warranted, modified CASPERs may be acceptable but must be described as modified in report(s). See Modified CASPERs for more information.
Develop maps via the Census website or GIS software so teams can easily navigate to the selected clusters. For more information and detail on selecting clusters, please see the CASPER Toolkit, Section 2.4.
Geographic Information System (GIS) CASPER toolbox
Using GIS software rather than the U.S. Census website allows the user to select portions of a:
- Other available geopolitical areas
This provides more flexibility in the selection of the sampling frame.
A CASPER Toolbox is available for GIS sample selection that can automatically select clusters. It is currently available in ArcGIS Desktop for those with GIS expertise. CDC also provides sampling and mapping support to requesting jurisdictions. Email [email protected] for more information.
Second stage sampling
Typically, a single individual will conduct the first stage of sampling (selecting 30 clusters). It is the responsibility of the interview teams to appropriately select the households within each cluster to interview.
Systematic random sampling
To select the seven households to interview, conduct systematic random sampling .
To do this, count (or estimate) the number of households within the selected cluster. Divide that number by 7 (this will be your n ). Starting at a random point, travel through the cluster in a serpentine method to select every nth household for interview. Walk up one side of the street. Then, turn and walk down the other side in such a manner that every house within the selected cluster is passed.
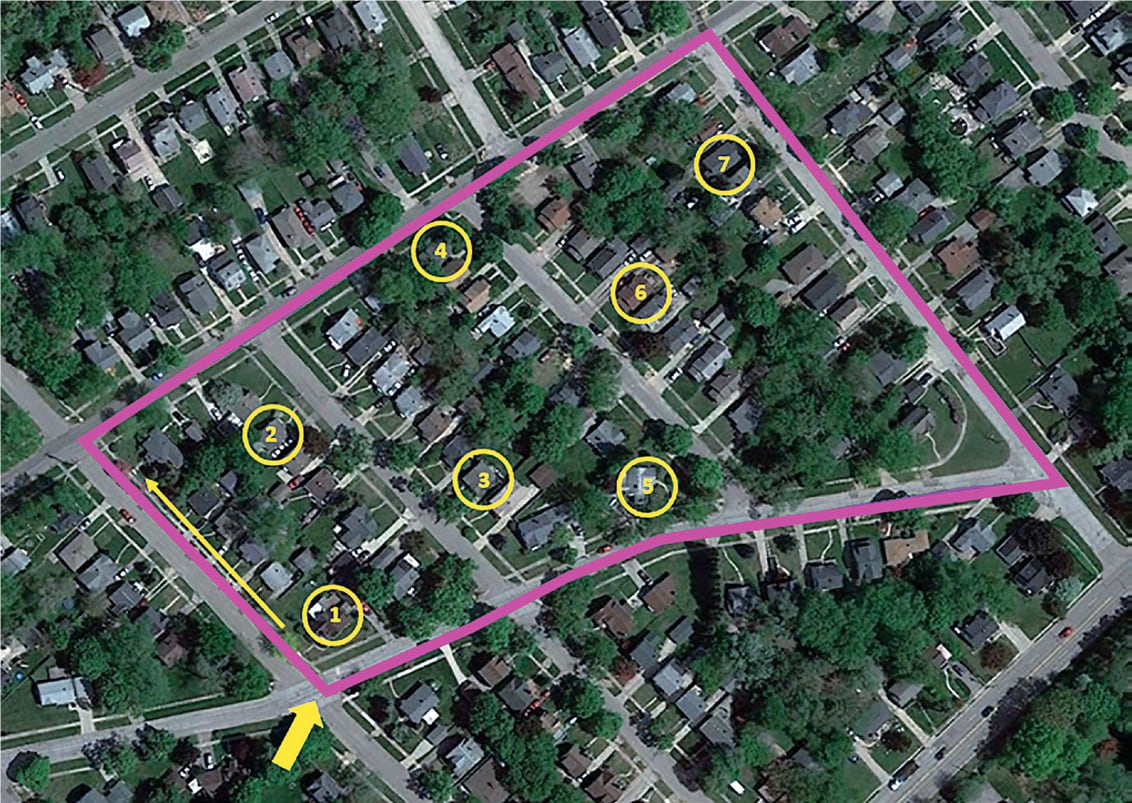
The most scientific and representative way is to select the seven households. Then, continue to return to those households until an interview is completed.
However, it is important to balance the scientifically ideal with the real-world or disaster situation. Therefore, interview teams should attempt to revisit the selected household three times . The interview teams may replace the household if an interview wasn't successful (e.g., household refused, nobody answered after three attempts, house is vacant, or there was language barrier).
Overall, keeping the sample as complete and representative as possible requires sound judgment and quality training of interview teams.
For more information, please see the CASPER Toolkit, Section 3.4 .
Things to avoid
Convenience sampling.
Convenience sampling is a form of non-probability sampling that involves selection based on:
- Availability
- Opportunity
- Convenience
Examples include going to households where there are people outside or where another interviewee told you to go since they know they would answer.

Target sampling
Target sampling is a form of non-probability sampling that involves intentionally sampling a certain population or group. An example is going to the household that looks the most damaged or like it will get "best" results.
Sequential sampling
Sequential sampling is going to one house after another in order. This will likely bias your sample to one section of your cluster.
Note that there are some circumstances which may predetermine sequential sampling as necessary. This includes clusters with less than 10 households. Another circumstance is when your clusters are difficult to navigate. In such a case, sequential sampling is the only way to successfully complete the CASPER in the time allowed. In these circumstances, it is extremely important to select a random starting point prior to going into the field.
Sampling and mapping support
CDC provides sampling and mapping support to aid in the CASPER process. This support is provided to any requesting jurisdiction or agency. Email [email protected] for assistance.
CASPER is a type of rapid needs assessment that provides household-level information to public health leaders and emergency managers.
- Open access
- Published: 14 May 2024
Developing a survey to measure nursing students’ knowledge, attitudes and beliefs, influences, and willingness to be involved in Medical Assistance in Dying (MAiD): a mixed method modified e-Delphi study
- Jocelyn Schroeder 1 ,
- Barbara Pesut 1 , 2 ,
- Lise Olsen 2 ,
- Nelly D. Oelke 2 &
- Helen Sharp 2
BMC Nursing volume 23 , Article number: 326 ( 2024 ) Cite this article
31 Accesses
Metrics details
Medical Assistance in Dying (MAiD) was legalized in Canada in 2016. Canada’s legislation is the first to permit Nurse Practitioners (NP) to serve as independent MAiD assessors and providers. Registered Nurses’ (RN) also have important roles in MAiD that include MAiD care coordination; client and family teaching and support, MAiD procedural quality; healthcare provider and public education; and bereavement care for family. Nurses have a right under the law to conscientious objection to participating in MAiD. Therefore, it is essential to prepare nurses in their entry-level education for the practice implications and moral complexities inherent in this practice. Knowing what nursing students think about MAiD is a critical first step. Therefore, the purpose of this study was to develop a survey to measure nursing students’ knowledge, attitudes and beliefs, influences, and willingness to be involved in MAiD in the Canadian context.
The design was a mixed-method, modified e-Delphi method that entailed item generation from the literature, item refinement through a 2 round survey of an expert faculty panel, and item validation through a cognitive focus group interview with nursing students. The settings were a University located in an urban area and a College located in a rural area in Western Canada.
During phase 1, a 56-item survey was developed from existing literature that included demographic items and items designed to measure experience with death and dying (including MAiD), education and preparation, attitudes and beliefs, influences on those beliefs, and anticipated future involvement. During phase 2, an expert faculty panel reviewed, modified, and prioritized the items yielding 51 items. During phase 3, a sample of nursing students further evaluated and modified the language in the survey to aid readability and comprehension. The final survey consists of 45 items including 4 case studies.
Systematic evaluation of knowledge-to-date coupled with stakeholder perspectives supports robust survey design. This study yielded a survey to assess nursing students’ attitudes toward MAiD in a Canadian context.
The survey is appropriate for use in education and research to measure knowledge and attitudes about MAiD among nurse trainees and can be a helpful step in preparing nursing students for entry-level practice.
Peer Review reports
Medical Assistance in Dying (MAiD) is permitted under an amendment to Canada’s Criminal Code which was passed in 2016 [ 1 ]. MAiD is defined in the legislation as both self-administered and clinician-administered medication for the purpose of causing death. In the 2016 Bill C-14 legislation one of the eligibility criteria was that an applicant for MAiD must have a reasonably foreseeable natural death although this term was not defined. It was left to the clinical judgement of MAiD assessors and providers to determine the time frame that constitutes reasonably foreseeable [ 2 ]. However, in 2021 under Bill C-7, the eligibility criteria for MAiD were changed to allow individuals with irreversible medical conditions, declining health, and suffering, but whose natural death was not reasonably foreseeable, to receive MAiD [ 3 ]. This population of MAiD applicants are referred to as Track 2 MAiD (those whose natural death is foreseeable are referred to as Track 1). Track 2 applicants are subject to additional safeguards under the 2021 C-7 legislation.
Three additional proposed changes to the legislation have been extensively studied by Canadian Expert Panels (Council of Canadian Academics [CCA]) [ 4 , 5 , 6 ] First, under the legislation that defines Track 2, individuals with mental disease as their sole underlying medical condition may apply for MAiD, but implementation of this practice is embargoed until March 2027 [ 4 ]. Second, there is consideration of allowing MAiD to be implemented through advanced consent. This would make it possible for persons living with dementia to receive MAID after they have lost the capacity to consent to the procedure [ 5 ]. Third, there is consideration of extending MAiD to mature minors. A mature minor is defined as “a person under the age of majority…and who has the capacity to understand and appreciate the nature and consequences of a decision” ([ 6 ] p. 5). In summary, since the legalization of MAiD in 2016 the eligibility criteria and safeguards have evolved significantly with consequent implications for nurses and nursing care. Further, the number of Canadians who access MAiD shows steady increases since 2016 [ 7 ] and it is expected that these increases will continue in the foreseeable future.
Nurses have been integral to MAiD care in the Canadian context. While other countries such as Belgium and the Netherlands also permit euthanasia, Canada is the first country to allow Nurse Practitioners (Registered Nurses with additional preparation typically achieved at the graduate level) to act independently as assessors and providers of MAiD [ 1 ]. Although the role of Registered Nurses (RNs) in MAiD is not defined in federal legislation, it has been addressed at the provincial/territorial-level with variability in scope of practice by region [ 8 , 9 ]. For example, there are differences with respect to the obligation of the nurse to provide information to patients about MAiD, and to the degree that nurses are expected to ensure that patient eligibility criteria and safeguards are met prior to their participation [ 10 ]. Studies conducted in the Canadian context indicate that RNs perform essential roles in MAiD care coordination; client and family teaching and support; MAiD procedural quality; healthcare provider and public education; and bereavement care for family [ 9 , 11 ]. Nurse practitioners and RNs are integral to a robust MAiD care system in Canada and hence need to be well-prepared for their role [ 12 ].
Previous studies have found that end of life care, and MAiD specifically, raise complex moral and ethical issues for nurses [ 13 , 14 , 15 , 16 ]. The knowledge, attitudes, and beliefs of nurses are important across practice settings because nurses have consistent, ongoing, and direct contact with patients who experience chronic or life-limiting health conditions. Canadian studies exploring nurses’ moral and ethical decision-making in relation to MAiD reveal that although some nurses are clear in their support for, or opposition to, MAiD, others are unclear on what they believe to be good and right [ 14 ]. Empirical findings suggest that nurses go through a period of moral sense-making that is often informed by their family, peers, and initial experiences with MAID [ 17 , 18 ]. Canadian legislation and policy specifies that nurses are not required to participate in MAiD and may recuse themselves as conscientious objectors with appropriate steps to ensure ongoing and safe care of patients [ 1 , 19 ]. However, with so many nurses having to reflect on and make sense of their moral position, it is essential that they are given adequate time and preparation to make an informed and thoughtful decision before they participate in a MAID death [ 20 , 21 ].
It is well established that nursing students receive inconsistent exposure to end of life care issues [ 22 ] and little or no training related to MAiD [ 23 ]. Without such education and reflection time in pre-entry nursing preparation, nurses are at significant risk for moral harm. An important first step in providing this preparation is to be able to assess the knowledge, values, and beliefs of nursing students regarding MAID and end of life care. As demand for MAiD increases along with the complexities of MAiD, it is critical to understand the knowledge, attitudes, and likelihood of engagement with MAiD among nursing students as a baseline upon which to build curriculum and as a means to track these variables over time.
Aim, design, and setting
The aim of this study was to develop a survey to measure nursing students’ knowledge, attitudes and beliefs, influences, and willingness to be involved in MAiD in the Canadian context. We sought to explore both their willingness to be involved in the registered nursing role and in the nurse practitioner role should they chose to prepare themselves to that level of education. The design was a mixed-method, modified e-Delphi method that entailed item generation, item refinement through an expert faculty panel [ 24 , 25 , 26 ], and initial item validation through a cognitive focus group interview with nursing students [ 27 ]. The settings were a University located in an urban area and a College located in a rural area in Western Canada.
Participants
A panel of 10 faculty from the two nursing education programs were recruited for Phase 2 of the e-Delphi. To be included, faculty were required to have a minimum of three years of experience in nurse education, be employed as nursing faculty, and self-identify as having experience with MAiD. A convenience sample of 5 fourth-year nursing students were recruited to participate in Phase 3. Students had to be in good standing in the nursing program and be willing to share their experiences of the survey in an online group interview format.
The modified e-Delphi was conducted in 3 phases: Phase 1 entailed item generation through literature and existing survey review. Phase 2 entailed item refinement through a faculty expert panel review with focus on content validity, prioritization, and revision of item wording [ 25 ]. Phase 3 entailed an assessment of face validity through focus group-based cognitive interview with nursing students.
Phase I. Item generation through literature review
The goal of phase 1 was to develop a bank of survey items that would represent the variables of interest and which could be provided to expert faculty in Phase 2. Initial survey items were generated through a literature review of similar surveys designed to assess knowledge and attitudes toward MAiD/euthanasia in healthcare providers; Canadian empirical studies on nurses’ roles and/or experiences with MAiD; and legislative and expert panel documents that outlined proposed changes to the legislative eligibility criteria and safeguards. The literature review was conducted in three online databases: CINAHL, PsycINFO, and Medline. Key words for the search included nurses , nursing students , medical students , NPs, MAiD , euthanasia , assisted death , and end-of-life care . Only articles written in English were reviewed. The legalization and legislation of MAiD is new in many countries; therefore, studies that were greater than twenty years old were excluded, no further exclusion criteria set for country.
Items from surveys designed to measure similar variables in other health care providers and geographic contexts were placed in a table and similar items were collated and revised into a single item. Then key variables were identified from the empirical literature on nurses and MAiD in Canada and checked against the items derived from the surveys to ensure that each of the key variables were represented. For example, conscientious objection has figured prominently in the Canadian literature, but there were few items that assessed knowledge of conscientious objection in other surveys and so items were added [ 15 , 21 , 28 , 29 ]. Finally, four case studies were added to the survey to address the anticipated changes to the Canadian legislation. The case studies were based upon the inclusion of mature minors, advanced consent, and mental disorder as the sole underlying medical condition. The intention was to assess nurses’ beliefs and comfort with these potential legislative changes.
Phase 2. Item refinement through expert panel review
The goal of phase 2 was to refine and prioritize the proposed survey items identified in phase 1 using a modified e-Delphi approach to achieve consensus among an expert panel [ 26 ]. Items from phase 1 were presented to an expert faculty panel using a Qualtrics (Provo, UT) online survey. Panel members were asked to review each item to determine if it should be: included, excluded or adapted for the survey. When adapted was selected faculty experts were asked to provide rationale and suggestions for adaptation through the use of an open text box. Items that reached a level of 75% consensus for either inclusion or adaptation were retained [ 25 , 26 ]. New items were categorized and added, and a revised survey was presented to the panel of experts in round 2. Panel members were again asked to review items, including new items, to determine if it should be: included, excluded, or adapted for the survey. Round 2 of the modified e-Delphi approach also included an item prioritization activity, where participants were then asked to rate the importance of each item, based on a 5-point Likert scale (low to high importance), which De Vaus [ 30 ] states is helpful for increasing the reliability of responses. Items that reached a 75% consensus on inclusion were then considered in relation to the importance it was given by the expert panel. Quantitative data were managed using SPSS (IBM Corp).
Phase 3. Face validity through cognitive interviews with nursing students
The goal of phase 3 was to obtain initial face validity of the proposed survey using a sample of nursing student informants. More specifically, student participants were asked to discuss how items were interpreted, to identify confusing wording or other problematic construction of items, and to provide feedback about the survey as a whole including readability and organization [ 31 , 32 , 33 ]. The focus group was held online and audio recorded. A semi-structured interview guide was developed for this study that focused on clarity, meaning, order and wording of questions; emotions evoked by the questions; and overall survey cohesion and length was used to obtain data (see Supplementary Material 2 for the interview guide). A prompt to “think aloud” was used to limit interviewer-imposed bias and encourage participants to describe their thoughts and response to a given item as they reviewed survey items [ 27 ]. Where needed, verbal probes such as “could you expand on that” were used to encourage participants to expand on their responses [ 27 ]. Student participants’ feedback was collated verbatim and presented to the research team where potential survey modifications were negotiated and finalized among team members. Conventional content analysis [ 34 ] of focus group data was conducted to identify key themes that emerged through discussion with students. Themes were derived from the data by grouping common responses and then using those common responses to modify survey items.
Ten nursing faculty participated in the expert panel. Eight of the 10 faculty self-identified as female. No faculty panel members reported conscientious objector status and ninety percent reported general agreement with MAiD with one respondent who indicated their view as “unsure.” Six of the 10 faculty experts had 16 years of experience or more working as a nurse educator.
Five nursing students participated in the cognitive interview focus group. The duration of the focus group was 2.5 h. All participants identified that they were born in Canada, self-identified as female (one preferred not to say) and reported having received some instruction about MAiD as part of their nursing curriculum. See Tables 1 and 2 for the demographic descriptors of the study sample. Study results will be reported in accordance with the study phases. See Fig. 1 for an overview of the results from each phase.

Fig. 1 Overview of survey development findings
Phase 1: survey item generation
Review of the literature identified that no existing survey was available for use with nursing students in the Canadian context. However, an analysis of themes across qualitative and quantitative studies of physicians, medical students, nurses, and nursing students provided sufficient data to develop a preliminary set of items suitable for adaptation to a population of nursing students.
Four major themes and factors that influence knowledge, attitudes, and beliefs about MAiD were evident from the literature: (i) endogenous or individual factors such as age, gender, personally held values, religion, religiosity, and/or spirituality [ 35 , 36 , 37 , 38 , 39 , 40 , 41 , 42 ], (ii) experience with death and dying in personal and/or professional life [ 35 , 40 , 41 , 43 , 44 , 45 ], (iii) training including curricular instruction about clinical role, scope of practice, or the law [ 23 , 36 , 39 ], and (iv) exogenous or social factors such as the influence of key leaders, colleagues, friends and/or family, professional and licensure organizations, support within professional settings, and/or engagement in MAiD in an interdisciplinary team context [ 9 , 35 , 46 ].
Studies of nursing students also suggest overlap across these categories. For example, value for patient autonomy [ 23 ] and the moral complexity of decision-making [ 37 ] are important factors that contribute to attitudes about MAiD and may stem from a blend of personally held values coupled with curricular content, professional training and norms, and clinical exposure. For example, students report that participation in end of life care allows for personal growth, shifts in perception, and opportunities to build therapeutic relationships with their clients [ 44 , 47 , 48 ].
Preliminary items generated from the literature resulted in 56 questions from 11 published sources (See Table 3 ). These items were constructed across four main categories: (i) socio-demographic questions; (ii) end of life care questions; (iii) knowledge about MAiD; or (iv) comfort and willingness to participate in MAiD. Knowledge questions were refined to reflect current MAiD legislation, policies, and regulatory frameworks. Falconer [ 39 ] and Freeman [ 45 ] studies were foundational sources for item selection. Additionally, four case studies were written to reflect the most recent anticipated changes to MAiD legislation and all used the same open-ended core questions to address respondents’ perspectives about the patient’s right to make the decision, comfort in assisting a physician or NP to administer MAiD in that scenario, and hypothesized comfort about serving as a primary provider if qualified as an NP in future. Response options for the survey were also constructed during this stage and included: open text, categorical, yes/no , and Likert scales.
Phase 2: faculty expert panel review
Of the 56 items presented to the faculty panel, 54 questions reached 75% consensus. However, based upon the qualitative responses 9 items were removed largely because they were felt to be repetitive. Items that generated the most controversy were related to measuring religion and spirituality in the Canadian context, defining end of life care when there is no agreed upon time frames (e.g., last days, months, or years), and predicting willingness to be involved in a future events – thus predicting their future selves. Phase 2, round 1 resulted in an initial set of 47 items which were then presented back to the faculty panel in round 2.
Of the 47 initial questions presented to the panel in round 2, 45 reached a level of consensus of 75% or greater, and 34 of these questions reached a level of 100% consensus [ 27 ] of which all participants chose to include without any adaptations) For each question, level of importance was determined based on a 5-point Likert scale (1 = very unimportant, 2 = somewhat unimportant, 3 = neutral, 4 = somewhat important, and 5 = very important). Figure 2 provides an overview of the level of importance assigned to each item.
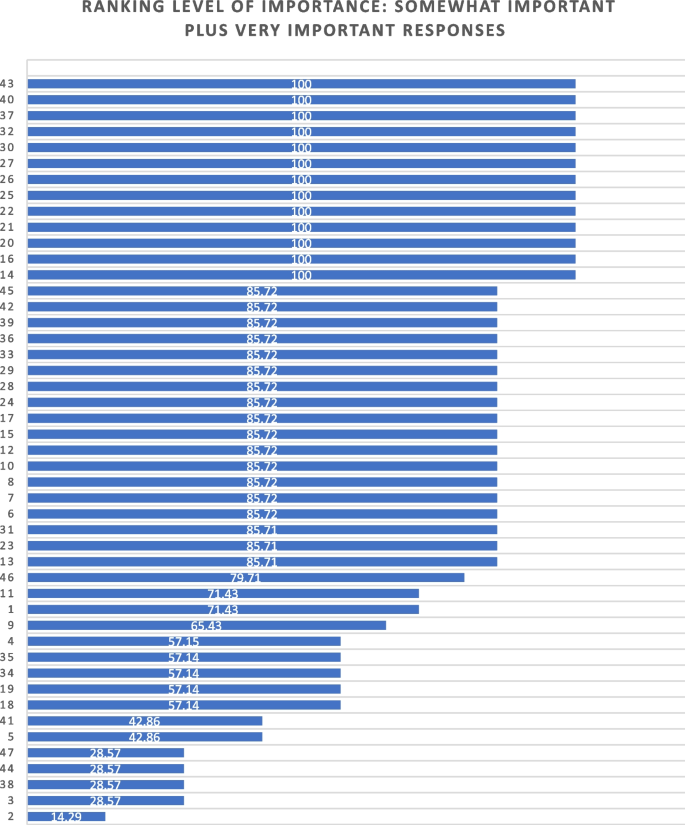
Ranking level of importance for survey items
After round 2, a careful analysis of participant comments and level of importance was completed by the research team. While the main method of survey item development came from participants’ response to the first round of Delphi consensus ratings, level of importance was used to assist in the decision of whether to keep or modify questions that created controversy, or that rated lower in the include/exclude/adapt portion of the Delphi. Survey items that rated low in level of importance included questions about future roles, sex and gender, and religion/spirituality. After deliberation by the research committee, these questions were retained in the survey based upon the importance of these variables in the scientific literature.
Of the 47 questions remaining from Phase 2, round 2, four were revised. In addition, the two questions that did not meet the 75% cut off level for consensus were reviewed by the research team. The first question reviewed was What is your comfort level with providing a MAiD death in the future if you were a qualified NP ? Based on a review of participant comments, it was decided to retain this question for the cognitive interviews with students in the final phase of testing. The second question asked about impacts on respondents’ views of MAiD and was changed from one item with 4 subcategories into 4 separate items, resulting in a final total of 51 items for phase 3. The revised survey was then brought forward to the cognitive interviews with student participants in Phase 3. (see Supplementary Material 1 for a complete description of item modification during round 2).
Phase 3. Outcomes of cognitive interview focus group
Of the 51 items reviewed by student participants, 29 were identified as clear with little or no discussion. Participant comments for the remaining 22 questions were noted and verified against the audio recording. Following content analysis of the comments, four key themes emerged through the student discussion: unclear or ambiguous wording; difficult to answer questions; need for additional response options; and emotional response evoked by questions. An example of unclear or ambiguous wording was a request for clarity in the use of the word “sufficient” in the context of assessing an item that read “My nursing education has provided sufficient content about the nursing role in MAiD.” “Sufficient” was viewed as subjective and “laden with…complexity that distracted me from the question.” The group recommended rewording the item to read “My nursing education has provided enough content for me to care for a patient considering or requesting MAiD.”
An example of having difficulty answering questions related to limited knowledge related to terms used in the legislation such as such as safeguards , mature minor , eligibility criteria , and conscientious objection. Students were unclear about what these words meant relative to the legislation and indicated that this lack of clarity would hamper appropriate responses to the survey. To ensure that respondents are able to answer relevant questions, student participants recommended that the final survey include explanation of key terms such as mature minor and conscientious objection and an overview of current legislation.
Response options were also a point of discussion. Participants noted a lack of distinction between response options of unsure and unable to say . Additionally, scaling of attitudes was noted as important since perspectives about MAiD are dynamic and not dichotomous “agree or disagree” responses. Although the faculty expert panel recommended the integration of the demographic variables of religious and/or spiritual remain as a single item, the student group stated a preference to have religion and spirituality appear as separate items. The student focus group also took issue with separate items for the variables of sex and gender, specifically that non-binary respondents might feel othered or “outed” particularly when asked to identify their sex. These variables had been created based upon best practices in health research but students did not feel they were appropriate in this context [ 49 ]. Finally, students agreed with the faculty expert panel in terms of the complexity of projecting their future involvement as a Nurse Practitioner. One participant stated: “I certainly had to like, whoa, whoa, whoa. Now let me finish this degree first, please.” Another stated, “I'm still imagining myself, my future career as an RN.”
Finally, student participants acknowledged the array of emotions that some of the items produced for them. For example, one student described positive feelings when interacting with the survey. “Brought me a little bit of feeling of joy. Like it reminded me that this is the last piece of independence that people grab on to.” Another participant, described the freedom that the idea of an advance request gave her. “The advance request gives the most comfort for me, just with early onset Alzheimer’s and knowing what it can do.” But other participants described less positive feelings. For example, the mature minor case study yielded a comment: “This whole scenario just made my heart hurt with the idea of a child requesting that.”
Based on the data gathered from the cognitive interview focus group of nursing students, revisions were made to 11 closed-ended questions (see Table 4 ) and 3 items were excluded. In the four case studies, the open-ended question related to a respondents’ hypothesized actions in a future role as NP were removed. The final survey consists of 45 items including 4 case studies (see Supplementary Material 3 ).
The aim of this study was to develop and validate a survey that can be used to track the growth of knowledge about MAiD among nursing students over time, inform training programs about curricular needs, and evaluate attitudes and willingness to participate in MAiD at time-points during training or across nursing programs over time.
The faculty expert panel and student participants in the cognitive interview focus group identified a need to establish core knowledge of the terminology and legislative rules related to MAiD. For example, within the cognitive interview group of student participants, several acknowledged lack of clear understanding of specific terms such as “conscientious objector” and “safeguards.” Participants acknowledged discomfort with the uncertainty of not knowing and their inclination to look up these terms to assist with answering the questions. This survey can be administered to nursing or pre-nursing students at any phase of their training within a program or across training programs. However, in doing so it is important to acknowledge that their baseline knowledge of MAiD will vary. A response option of “not sure” is important and provides a means for respondents to convey uncertainty. If this survey is used to inform curricular needs, respondents should be given explicit instructions not to conduct online searches to inform their responses, but rather to provide an honest appraisal of their current knowledge and these instructions are included in the survey (see Supplementary Material 3 ).
Some provincial regulatory bodies have established core competencies for entry-level nurses that include MAiD. For example, the BC College of Nurses and Midwives (BCCNM) requires “knowledge about ethical, legal, and regulatory implications of medical assistance in dying (MAiD) when providing nursing care.” (10 p. 6) However, across Canada curricular content and coverage related to end of life care and MAiD is variable [ 23 ]. Given the dynamic nature of the legislation that includes portions of the law that are embargoed until 2024, it is important to ensure that respondents are guided by current and accurate information. As the law changes, nursing curricula, and public attitudes continue to evolve, inclusion of core knowledge and content is essential and relevant for investigators to be able to interpret the portions of the survey focused on attitudes and beliefs about MAiD. Content knowledge portions of the survey may need to be modified over time as legislation and training change and to meet the specific purposes of the investigator.
Given the sensitive nature of the topic, it is strongly recommended that surveys be conducted anonymously and that students be provided with an opportunity to discuss their responses to the survey. A majority of feedback from both the expert panel of faculty and from student participants related to the wording and inclusion of demographic variables, in particular religion, religiosity, gender identity, and sex assigned at birth. These and other demographic variables have the potential to be highly identifying in small samples. In any instance in which the survey could be expected to yield demographic group sizes less than 5, users should eliminate the demographic variables from the survey. For example, the profession of nursing is highly dominated by females with over 90% of nurses who identify as female [ 50 ]. Thus, a survey within a single class of students or even across classes in a single institution is likely to yield a small number of male respondents and/or respondents who report a difference between sex assigned at birth and gender identity. When variables that serve to identify respondents are included, respondents are less likely to complete or submit the survey, to obscure their responses so as not to be identifiable, or to be influenced by social desirability bias in their responses rather than to convey their attitudes accurately [ 51 ]. Further, small samples do not allow for conclusive analyses or interpretation of apparent group differences. Although these variables are often included in surveys, such demographics should be included only when anonymity can be sustained. In small and/or known samples, highly identifying variables should be omitted.
There are several limitations associated with the development of this survey. The expert panel was comprised of faculty who teach nursing students and are knowledgeable about MAiD and curricular content, however none identified as a conscientious objector to MAiD. Ideally, our expert panel would have included one or more conscientious objectors to MAiD to provide a broader perspective. Review by practitioners who participate in MAiD, those who are neutral or undecided, and practitioners who are conscientious objectors would ensure broad applicability of the survey. This study included one student cognitive interview focus group with 5 self-selected participants. All student participants had held discussions about end of life care with at least one patient, 4 of 5 participants had worked with a patient who requested MAiD, and one had been present for a MAiD death. It is not clear that these participants are representative of nursing students demographically or by experience with end of life care. It is possible that the students who elected to participate hold perspectives and reflections on patient care and MAiD that differ from students with little or no exposure to end of life care and/or MAiD. However, previous studies find that most nursing students have been involved with end of life care including meaningful discussions about patients’ preferences and care needs during their education [ 40 , 44 , 47 , 48 , 52 ]. Data collection with additional student focus groups with students early in their training and drawn from other training contexts would contribute to further validation of survey items.
Future studies should incorporate pilot testing with small sample of nursing students followed by a larger cross-program sample to allow evaluation of the psychometric properties of specific items and further refinement of the survey tool. Consistent with literature about the importance of leadership in the context of MAiD [ 12 , 53 , 54 ], a study of faculty knowledge, beliefs, and attitudes toward MAiD would provide context for understanding student perspectives within and across programs. Additional research is also needed to understand the timing and content coverage of MAiD across Canadian nurse training programs’ curricula.
The implementation of MAiD is complex and requires understanding of the perspectives of multiple stakeholders. Within the field of nursing this includes clinical providers, educators, and students who will deliver clinical care. A survey to assess nursing students’ attitudes toward and willingness to participate in MAiD in the Canadian context is timely, due to the legislation enacted in 2016 and subsequent modifications to the law in 2021 with portions of the law to be enacted in 2027. Further development of this survey could be undertaken to allow for use in settings with practicing nurses or to allow longitudinal follow up with students as they enter practice. As the Canadian landscape changes, ongoing assessment of the perspectives and needs of health professionals and students in the health professions is needed to inform policy makers, leaders in practice, curricular needs, and to monitor changes in attitudes and practice patterns over time.
Availability of data and materials
The datasets used and/or analysed during the current study are not publicly available due to small sample sizes, but are available from the corresponding author on reasonable request.
Abbreviations
British Columbia College of Nurses and Midwives
Medical assistance in dying
Nurse practitioner
Registered nurse
University of British Columbia Okanagan
Nicol J, Tiedemann M. Legislative Summary: Bill C-14: An Act to amend the Criminal Code and to make related amendments to other Acts (medical assistance in dying). Available from: https://lop.parl.ca/staticfiles/PublicWebsite/Home/ResearchPublications/LegislativeSummaries/PDF/42-1/c14-e.pdf .
Downie J, Scallion K. Foreseeably unclear. The meaning of the “reasonably foreseeable” criterion for access to medical assistance in dying in Canada. Dalhousie Law J. 2018;41(1):23–57.
Nicol J, Tiedeman M. Legislative summary of Bill C-7: an act to amend the criminal code (medical assistance in dying). Ottawa: Government of Canada; 2021.
Google Scholar
Council of Canadian Academies. The state of knowledge on medical assistance in dying where a mental disorder is the sole underlying medical condition. Ottawa; 2018. Available from: https://cca-reports.ca/wp-content/uploads/2018/12/The-State-of-Knowledge-on-Medical-Assistance-in-Dying-Where-a-Mental-Disorder-is-the-Sole-Underlying-Medical-Condition.pdf .
Council of Canadian Academies. The state of knowledge on advance requests for medical assistance in dying. Ottawa; 2018. Available from: https://cca-reports.ca/wp-content/uploads/2019/02/The-State-of-Knowledge-on-Advance-Requests-for-Medical-Assistance-in-Dying.pdf .
Council of Canadian Academies. The state of knowledge on medical assistance in dying for mature minors. Ottawa; 2018. Available from: https://cca-reports.ca/wp-content/uploads/2018/12/The-State-of-Knowledge-on-Medical-Assistance-in-Dying-for-Mature-Minors.pdf .
Health Canada. Third annual report on medical assistance in dying in Canada 2021. Ottawa; 2022. [cited 2023 Oct 23]. Available from: https://www.canada.ca/en/health-canada/services/medical-assistance-dying/annual-report-2021.html .
Banner D, Schiller CJ, Freeman S. Medical assistance in dying: a political issue for nurses and nursing in Canada. Nurs Philos. 2019;20(4): e12281.
Article PubMed Google Scholar
Pesut B, Thorne S, Stager ML, Schiller CJ, Penney C, Hoffman C, et al. Medical assistance in dying: a review of Canadian nursing regulatory documents. Policy Polit Nurs Pract. 2019;20(3):113–30.
Article PubMed PubMed Central Google Scholar
College of Registered Nurses of British Columbia. Scope of practice for registered nurses [Internet]. Vancouver; 2018. Available from: https://www.bccnm.ca/Documents/standards_practice/rn/RN_ScopeofPractice.pdf .
Pesut B, Thorne S, Schiller C, Greig M, Roussel J, Tishelman C. Constructing good nursing practice for medical assistance in dying in Canada: an interpretive descriptive study. Global Qual Nurs Res. 2020;7:2333393620938686. https://doi.org/10.1177/2333393620938686 .
Article Google Scholar
Pesut B, Thorne S, Schiller CJ, Greig M, Roussel J. The rocks and hard places of MAiD: a qualitative study of nursing practice in the context of legislated assisted death. BMC Nurs. 2020;19:12. https://doi.org/10.1186/s12912-020-0404-5 .
Pesut B, Greig M, Thorne S, Burgess M, Storch JL, Tishelman C, et al. Nursing and euthanasia: a narrative review of the nursing ethics literature. Nurs Ethics. 2020;27(1):152–67.
Pesut B, Thorne S, Storch J, Chambaere K, Greig M, Burgess M. Riding an elephant: a qualitative study of nurses’ moral journeys in the context of Medical Assistance in Dying (MAiD). Journal Clin Nurs. 2020;29(19–20):3870–81.
Lamb C, Babenko-Mould Y, Evans M, Wong CA, Kirkwood KW. Conscientious objection and nurses: results of an interpretive phenomenological study. Nurs Ethics. 2018;26(5):1337–49.
Wright DK, Chan LS, Fishman JR, Macdonald ME. “Reflection and soul searching:” Negotiating nursing identity at the fault lines of palliative care and medical assistance in dying. Social Sci & Med. 2021;289: 114366.
Beuthin R, Bruce A, Scaia M. Medical assistance in dying (MAiD): Canadian nurses’ experiences. Nurs Forum. 2018;54(4):511–20.
Bruce A, Beuthin R. Medically assisted dying in Canada: "Beautiful Death" is transforming nurses' experiences of suffering. The Canadian J Nurs Res | Revue Canadienne de Recherche en Sci Infirmieres. 2020;52(4):268–77. https://doi.org/10.1177/0844562119856234 .
Canadian Nurses Association. Code of ethics for registered nurses. Ottawa; 2017. Available from: https://www.cna-aiic.ca/en/nursing/regulated-nursing-in-canada/nursing-ethics .
Canadian Nurses Association. National nursing framework on Medical Assistance in Dying in Canada. Ottawa: 2017. Available from: https://www.virtualhospice.ca/Assets/cna-national-nursing-framework-on-maidEng_20170216155827.pdf .
Pesut B, Thorne S, Greig M. Shades of gray: conscientious objection in medical assistance in dying. Nursing Inq. 2020;27(1): e12308.
Durojaiye A, Ryan R, Doody O. Student nurse education and preparation for palliative care: a scoping review. PLoS ONE. 2023. https://doi.org/10.1371/journal.pone.0286678 .
McMechan C, Bruce A, Beuthin R. Canadian nursing students’ experiences with medical assistance in dying | Les expériences d’étudiantes en sciences infirmières au regard de l’aide médicale à mourir. Qual Adv Nurs Educ - Avancées en Formation Infirmière. 2019;5(1). https://doi.org/10.17483/2368-6669.1179 .
Adler M, Ziglio E. Gazing into the oracle. The Delphi method and its application to social policy and public health. London: Jessica Kingsley Publishers; 1996
Keeney S, Hasson F, McKenna H. Consulting the oracle: ten lessons from using the Delphi technique in nursing research. J Adv Nurs. 2006;53(2):205–12.
Keeney S, Hasson F, McKenna H. The Delphi technique in nursing and health research. 1st ed. City: Wiley; 2011.
Willis GB. Cognitive interviewing: a tool for improving questionnaire design. 1st ed. Thousand Oaks, Calif: Sage; 2005. ISBN: 9780761928041
Lamb C, Evans M, Babenko-Mould Y, Wong CA, Kirkwood EW. Conscience, conscientious objection, and nursing: a concept analysis. Nurs Ethics. 2017;26(1):37–49.
Lamb C, Evans M, Babenko-Mould Y, Wong CA, Kirkwood K. Nurses’ use of conscientious objection and the implications of conscience. J Adv Nurs. 2018;75(3):594–602.
de Vaus D. Surveys in social research. 6th ed. Abingdon, Oxon: Routledge; 2014.
Boateng GO, Neilands TB, Frongillo EA, Melgar-Quiñonez HR, Young SL. Best practices for developing and validating scales for health, social, and behavioral research: A primer. Front Public Health. 2018;6:149. https://doi.org/10.3389/fpubh.2018.00149 .
Puchta C, Potter J. Focus group practice. 1st ed. London: Sage; 2004.
Book Google Scholar
Streiner DL, Norman GR, Cairney J. Health measurement scales: a practical guide to their development and use. 5th ed. Oxford: Oxford University Press; 2015.
Hsieh H-F, Shannon SE. Three approaches to qualitative content analysis. Qual Health Res. 2005;15(9):1277–88.
Adesina O, DeBellis A, Zannettino L. Third-year Australian nursing students’ attitudes, experiences, knowledge, and education concerning end-of-life care. Int J of Palliative Nurs. 2014;20(8):395–401.
Bator EX, Philpott B, Costa AP. This moral coil: a cross-sectional survey of Canadian medical student attitudes toward medical assistance in dying. BMC Med Ethics. 2017;18(1):58.
Beuthin R, Bruce A, Scaia M. Medical assistance in dying (MAiD): Canadian nurses’ experiences. Nurs Forum. 2018;53(4):511–20.
Brown J, Goodridge D, Thorpe L, Crizzle A. What is right for me, is not necessarily right for you: the endogenous factors influencing nonparticipation in medical assistance in dying. Qual Health Res. 2021;31(10):1786–1800.
Falconer J, Couture F, Demir KK, Lang M, Shefman Z, Woo M. Perceptions and intentions toward medical assistance in dying among Canadian medical students. BMC Med Ethics. 2019;20(1):22.
Green G, Reicher S, Herman M, Raspaolo A, Spero T, Blau A. Attitudes toward euthanasia—dual view: Nursing students and nurses. Death Stud. 2022;46(1):124–31.
Hosseinzadeh K, Rafiei H. Nursing student attitudes toward euthanasia: a cross-sectional study. Nurs Ethics. 2019;26(2):496–503.
Ozcelik H, Tekir O, Samancioglu S, Fadiloglu C, Ozkara E. Nursing students’ approaches toward euthanasia. Omega (Westport). 2014;69(1):93–103.
Canning SE, Drew C. Canadian nursing students’ understanding, and comfort levels related to medical assistance in dying. Qual Adv Nurs Educ - Avancées en Formation Infirmière. 2022;8(2). https://doi.org/10.17483/2368-6669.1326 .
Edo-Gual M, Tomás-Sábado J, Bardallo-Porras D, Monforte-Royo C. The impact of death and dying on nursing students: an explanatory model. J Clin Nurs. 2014;23(23–24):3501–12.
Freeman LA, Pfaff KA, Kopchek L, Liebman J. Investigating palliative care nurse attitudes towards medical assistance in dying: an exploratory cross-sectional study. J Adv Nurs. 2020;76(2):535–45.
Brown J, Goodridge D, Thorpe L, Crizzle A. “I am okay with it, but I am not going to do it:” the exogenous factors influencing non-participation in medical assistance in dying. Qual Health Res. 2021;31(12):2274–89.
Dimoula M, Kotronoulas G, Katsaragakis S, Christou M, Sgourou S, Patiraki E. Undergraduate nursing students’ knowledge about palliative care and attitudes towards end-of-life care: A three-cohort, cross-sectional survey. Nurs Educ Today. 2019;74:7–14.
Matchim Y, Raetong P. Thai nursing students’ experiences of caring for patients at the end of life: a phenomenological study. Int J Palliative Nurs. 2018;24(5):220–9.
Canadian Institute for Health Research. Sex and gender in health research [Internet]. Ottawa: CIHR; 2021 [cited 2023 Oct 23]. Available from: https://cihr-irsc.gc.ca/e/50833.html .
Canadian Nurses’ Association. Nursing statistics. Ottawa: CNA; 2023 [cited 2023 Oct 23]. Available from: https://www.cna-aiic.ca/en/nursing/regulated-nursing-in-canada/nursing-statistics .
Krumpal I. Determinants of social desirability bias in sensitive surveys: a literature review. Qual Quant. 2013;47(4):2025–47. https://doi.org/10.1007/s11135-011-9640-9 .
Ferri P, Di Lorenzo R, Stifani S, Morotti E, Vagnini M, Jiménez Herrera MF, et al. Nursing student attitudes toward dying patient care: a European multicenter cross-sectional study. Acta Bio Medica Atenei Parmensis. 2021;92(S2): e2021018.
PubMed PubMed Central Google Scholar
Beuthin R, Bruce A. Medical assistance in dying (MAiD): Ten things leaders need to know. Nurs Leadership. 2018;31(4):74–81.
Thiele T, Dunsford J. Nurse leaders’ role in medical assistance in dying: a relational ethics approach. Nurs Ethics. 2019;26(4):993–9.
Download references
Acknowledgements
We would like to acknowledge the faculty and students who generously contributed their time to this work.
JS received a student traineeship through the Principal Research Chairs program at the University of British Columbia Okanagan.
Author information
Authors and affiliations.
School of Health and Human Services, Selkirk College, Castlegar, BC, Canada
Jocelyn Schroeder & Barbara Pesut
School of Nursing, University of British Columbia Okanagan, Kelowna, BC, Canada
Barbara Pesut, Lise Olsen, Nelly D. Oelke & Helen Sharp
You can also search for this author in PubMed Google Scholar
Contributions
JS made substantial contributions to the conception of the work; data acquisition, analysis, and interpretation; and drafting and substantively revising the work. JS has approved the submitted version and agreed to be personally accountable for the author's own contributions and to ensure that questions related to the accuracy or integrity of any part of the work, even ones in which the author was not personally involved, are appropriately investigated, resolved, and the resolution documented in the literature. BP made substantial contributions to the conception of the work; data acquisition, analysis, and interpretation; and drafting and substantively revising the work. BP has approved the submitted version and agreed to be personally accountable for the author's own contributions and to ensure that questions related to the accuracy or integrity of any part of the work, even ones in which the author was not personally involved, are appropriately investigated, resolved, and the resolution documented in the literature. LO made substantial contributions to the conception of the work; data acquisition, analysis, and interpretation; and substantively revising the work. LO has approved the submitted version and agreed to be personally accountable for the author's own contributions and to ensure that questions related to the accuracy or integrity of any part of the work, even ones in which the author was not personally involved, are appropriately investigated, resolved, and the resolution documented in the literature. NDO made substantial contributions to the conception of the work; data acquisition, analysis, and interpretation; and substantively revising the work. NDO has approved the submitted version and agreed to be personally accountable for the author's own contributions and to ensure that questions related to the accuracy or integrity of any part of the work, even ones in which the author was not personally involved, are appropriately investigated, resolved, and the resolution documented in the literature. HS made substantial contributions to drafting and substantively revising the work. HS has approved the submitted version and agreed to be personally accountable for the author's own contributions and to ensure that questions related to the accuracy or integrity of any part of the work, even ones in which the author was not personally involved, are appropriately investigated, resolved, and the resolution documented in the literature.
Authors’ information
JS conducted this study as part of their graduate requirements in the School of Nursing, University of British Columbia Okanagan.
Corresponding author
Correspondence to Barbara Pesut .
Ethics declarations
Ethics approval and consent to participate.
The research was approved by the Selkirk College Research Ethics Board (REB) ID # 2021–011 and the University of British Columbia Behavioral Research Ethics Board ID # H21-01181.
All participants provided written and informed consent through approved consent processes. Research was conducted in accordance with the Declaration of Helsinki.
Consent for publication
Not applicable.
Competing interests
The authors declare they have no competing interests.
Additional information
Publisher’s note.
Springer Nature remains neutral with regard to jurisdictional claims in published maps and institutional affiliations.
Supplementary Information
Supplementary material 1., supplementary material 2., supplementary material 3., rights and permissions.
Open Access This article is licensed under a Creative Commons Attribution 4.0 International License, which permits use, sharing, adaptation, distribution and reproduction in any medium or format, as long as you give appropriate credit to the original author(s) and the source, provide a link to the Creative Commons licence, and indicate if changes were made. The images or other third party material in this article are included in the article's Creative Commons licence, unless indicated otherwise in a credit line to the material. If material is not included in the article's Creative Commons licence and your intended use is not permitted by statutory regulation or exceeds the permitted use, you will need to obtain permission directly from the copyright holder. To view a copy of this licence, visit http://creativecommons.org/licenses/by/4.0/ . The Creative Commons Public Domain Dedication waiver ( http://creativecommons.org/publicdomain/zero/1.0/ ) applies to the data made available in this article, unless otherwise stated in a credit line to the data.
Reprints and permissions
About this article
Cite this article.
Schroeder, J., Pesut, B., Olsen, L. et al. Developing a survey to measure nursing students’ knowledge, attitudes and beliefs, influences, and willingness to be involved in Medical Assistance in Dying (MAiD): a mixed method modified e-Delphi study. BMC Nurs 23 , 326 (2024). https://doi.org/10.1186/s12912-024-01984-z
Download citation
Received : 24 October 2023
Accepted : 28 April 2024
Published : 14 May 2024
DOI : https://doi.org/10.1186/s12912-024-01984-z
Share this article
Anyone you share the following link with will be able to read this content:
Sorry, a shareable link is not currently available for this article.
Provided by the Springer Nature SharedIt content-sharing initiative
- Medical assistance in dying (MAiD)
- End of life care
- Student nurses
- Nursing education
BMC Nursing
ISSN: 1472-6955
- General enquiries: [email protected]
- - Google Chrome
Intended for healthcare professionals
- Access provided by Google Indexer
- My email alerts
- BMA member login
- Username * Password * Forgot your log in details? Need to activate BMA Member Log In Log in via OpenAthens Log in via your institution

Search form
- Advanced search
- Search responses
- Search blogs
- Clinical and...
Clinical and healthcare use outcomes after cessation of long term opioid treatment due to prescriber workforce exit: quasi-experimental difference-in-differences study
- Related content
- Peer review
- Adrienne H Sabety , assistant professor 1 ,
- Hannah T Neprash , assistant professor 2 ,
- Marema Gaye , doctoral student 3 ,
- Michael L Barnett , associate professor 4
- 1 Department of Health Policy, Stanford University School of Medicine, Stanford, CA, USA
- 2 Division of Health Policy and Management, School of Public Health, University of Minnesota, Minneapolis, MN, USA
- 3 Interfaculty Initiative in Health Policy, Harvard University, Cambridge, MA, USA
- 4 Department of Health Policy and Management, Harvard T H Chan School of Public Health and Division of General Internal Medicine and Primary Care, Department of Medicine, Brigham and Women’s Hospital
- Correspondence to: M L Barnett mbarnett{at}hsph.harvard.edu ( @ml_barnett on X)
- Accepted 13 March 2024
Objective To examine the association between prescriber workforce exit, long term opioid treatment discontinuation, and clinical outcomes.
Design Quasi-experimental difference-in-differences study
Setting 20% sample of US Medicare beneficiaries, 2011-18.
Participants People receiving long term opioid treatment whose prescriber stopped providing office based patient care or exited the workforce, as in the case of retirement or death (n=48 079), and people whose prescriber did not exit the workforce (n=48 079).
Main outcomes Discontinuation from long term opioid treatment, drug overdose, mental health crises, admissions to hospital or emergency department visits, and death. Long term opioid treatment was defined as at least 60 days of opioids per quarter for four consecutive quarters, attributed to the plurality opioid prescriber. A difference-in-differences analysis was used to compare individuals who received long term opioid treatment and who had a prescriber leave the workforce to propensity-matched patients on long term opioid treatment who did not lose a prescriber, before and after prescriber exit.
Results Discontinuation of long term opioid treatment increased from 132 to 229 per 10 000 patients who had prescriber exit from the quarter before to the quarter after exit, compared with 97 to 100 for patients who had a continuation of prescriber (adjusted difference 1.22 percentage points, 95% confidence interval 1.02 to 1.42). In the first quarter after provider exit, when discontinuation rates were highest, a transient but significant elevation was noted between the two groups of patients in suicide attempts (adjusted difference 0.05 percentage points (95% confidence interval 0.01 to 0.09)), opioid or alcohol withdrawal (0.14 (0.01 to 0.27)), and admissions to hospital or emergency department visits (0.04 visits (0.01 to 0.06)). These differences receded after one to two quarters. No significant change in rates of overdose was noted. Across all four quarters after prescriber exit, an increase was reported in the rate of mental health crises (0.39 percentage points (95% confidence interval 0.08 to 0.69)) and opioid or alcohol withdrawal (0.31 (0.014 to 0.58)), but no change was seen for drug overdose (−0.12 (−0.41 to 0.18)).
Conclusions The loss of a prescriber was associated with increased occurrences of discontinuation of long term opioid treatment and transient increases in adverse outcomes, such as suicide attempts, but not other outcomes, such as overdoses. Long term opioid treatment discontinuation may be associated with a temporary period of adverse health impacts after accounting for unobserved confounding.
Introduction
Chronic pain affects more than one in four American adults over 65 years old and is commonly managed using long term opioid treatment (LTOT). 1 2 3 However, the general shift away from prescribing opioids has meant that millions of patients in chronic pain are also being removed from LTOT, often at faster taper speeds than recommended by guidelines. 4 5 6 3 7 8 9 Many have raised concerns that poor adherence to guideline-suggested tapering may lead to undertreated pain, mental health crises, and suicide. 10 11
Work examining the association between tapering or discontinuation of LTOT and health outcomes has important limitations. Systematic reviews on the reduction or discontinuation of LTOT find little high quality evidence, although studies generally indicate improvement in pain and quality of life after discontinuation or tapering. 12 13 By contrast, large scale observational studies find both increased and decreased risk for death or addiction related adverse events. 7 14 15 16 17 18 19 20 21 These studies typically use statistical techniques adjusting for observable, but not unobservable, differences between users of LTOT who taper or discontinue versus those who do not, populations whose clinical profiles can diverge around the time of LTOT changes. 3 22 23 For instance, a patient may be discontinued because prescribers suspect that patients are bordering on behavioral, substance use, or mental health disorders, whereas patients continued on LTOT are maintaining baseline. Without robust evidence that accounts for selection and confounding, clinical knowledge on the association between LTOT discontinuation and patient outcomes is incomplete, impeding both therapeutic management of LTOT and the development of opioid policy.
In this study, we investigated the evidence gap by leveraging prescriber exit from the workforce, a common event, 24 25 26 as an external shock to prescribing patterns. Previous work found that primary care physician exit was associated with substantial shifts in patient prescribing patterns. 27 28 We hypothesized that prescriber market exit would lead to an increase in discontinuation of LTOT unrelated to observed or unobserved patient clinical factors. Patients receiving LTOT who discontinued or tapered from opioids are likely different from those who have not tapered, therefore, we addressed selection bias and confounding by defining the exposure as prescriber workforce exit, an event plausibly not driven by clinical events leading to LTOT discontinuation. 18 This method of experimentation enabled testing for the independent effect of discontinuation of LTOT on patient outcomes.
Data source and study population
The cohort study used a 20% random sample of Medicare fee-for-service and Medicare Advantage beneficiaries from 1 January 2011 to 31 December 2018. Medicare is a public insurance program that enrolls 65 million Americans who are age 65 years or older, receiving social security disability income, or diagnosed with amyotrophic lateral sclerosis or end stage renal disease. Our primary study cohort captured all clinical and healthcare use outcomes for fee-for-service beneficiaries enrolled in fee-for service Medicare parts A, B, and D. For outcomes related to prescriptions, we also included Medicare Advantage beneficiaries with Medicare part D prescription claims. We excluded data with missing racial status accounting for less than 1% of the sample. Additionally, we excluded patients diagnosed with cancer at any point over the sample period because the role of LTOT may differ between cancer and non-cancer indications (see appendix figure 1 for cohort flow diagrams).
Identification of prescribers who exited Medicare
The main study exposure occurred when a prescriber stopped providing office based patient care or exited, as in the case of retirement or death. Patients attributed to such an exiting prescriber were considered exposed to this discontinuation. A prescriber’s exit date was defined as the last date the prescriber billed Medicare for an office based service with no subsequent services observed, as defined in prior research. 29 30 Prescribers were considered exiting if they had at least one office visit 6-12 months before their last observed office visit and had a last office visit between 1 January 2012 and 31 December 2017. These restrictions allowed us to observe patients prescribed an opioid at least four quarters before and after prescriber workforce exit ( fig 1 ). Patients attributed to prescribers with an exit date were considered exposed while those without prescriber exit were considered unexposed.

Matching algorithm. The dashed line indicates the period between the patients’ long term opioid treatment (LTOT) episode and quarter (Q) −5 relative to prescriber exit. The first quarter before exit was excluded when capturing eligible LTOT episodes to avoid bias from anticipation of prescriber exit that could affect patterns of LTOT and subsequent outcomes. Prescriber exit is denoted as quarter 0, with minus numbers indicating quarters before prescriber exit. Patients were matched on calendar year the initial LTOT episode began (2011, 2012, 2013, 2014, 2015, or 2016/2017), patient age, gender (female v male), race (white v not white), state of residence, whether the patient resided in a rural area, Medicare eligible due to disability, Medicare eligible due to end stage renal disease, dual eligibility for Medicare and Medicaid, Medicare Advantage enrollment, diagnosis of chronic non-cancer pain, total number of chronic conditions, average daily morphine milligram equivalents in initial LTOT episode. Information on dual eligibility, Medicare Advantage enrollment, diagnosis of chronic non-cancer pain, and average daily morphine milligram equivalents for the entire duration of the initial LTOT episode were also collected. All other covariate information was obtained from the calendar year in which the initial LTOT episode began
- Download figure
- Open in new tab
- Download powerpoint
Study sample and LTOT definition
The study sample included Medicare beneficiaries receiving LTOT who were exposed and unexposed to prescriber exit. To be included, the beneficiary had to be at least 18 years old and continuously enrolled in Medicare. LTOT was defined as receipt of at least 60 days’ supply of opioids at a dosage of 25 daily morphine milligram equivalents or more on average per quarter for at least four consecutive quarters. The first four or more quarter period meeting this definition was the initial LTOT episode for patients.
Patients in the exposed group were limited to those with an LTOT episode beginning at least five quarters before prescriber exit (meaning the first quarter before exit was excluded when capturing eligible LTOT episodes) to avoid bias from anticipation of prescriber exit that could affect patterns of LTOT and subsequent outcomes ( fig 1 ). 3 Unexposed patients were comprised of those meeting the definition for having an LTOT episode. Patients were attributed to the prescriber providing the plurality of opioid prescriptions over the initial four quarter LTOT episode.
Matching and exit date assignment
To control for observed patient differences, we used propensity score matching to match patients of exiting prescribers (exposed patients) to patients who did not lose their prescriber (unexposed patients). The propensity score, which estimates the likelihood that a given patient would be in the exposed group, was estimated using patient covariates measured the first year they began LTOT. We only matched patients who were unexposed and exposed with an initial LTOT episode in the same calendar year to account for nationwide changes in opioid prescribing over time (appendix methods 1). 31
After propensity score matching, we assigned the patient who was unexposed to the same prescriber exit date of the matched patient who was exposed. For example, consider patient A who was exposed to LTOT in January 2011, subsequently losing their prescriber in August 2013. The closest propensity score match is patient B who was not exposed and began LTOT in January 2011 but did not lose their prescriber. We therefore assigned the exit date of August 2013 from exposed patient A’s prescriber to be the synthetic exit date for unexposed patient B. This assignment of exit dates to unexposed patients enabled us to model changes in exposed patients’ outcomes in response to the loss of a prescriber compared with observably similar unexposed patients before and after prescriber exit in a difference-in-differences design ( fig 1 ).
Defining opioid discontinuation
Discontinuation from opioid treatment occurred when a patient had no resumption of opioid treatment at the end of the prescription’s days supplied for at least one year after discontinuation (see appendix figure 2 for the allocation of prescriptions, as well as by provider type). Discontinuation was defined as equal to one in the quarter of discontinuation and zero in the quarters before and after the discontinuation event.
Outcome measures
Our main outcomes were adverse clinical events that could plausibly be a clinical outcome in response to discontinuation of LTOT: all drug overdoses, mental health crises, opioid or alcohol withdrawal, gastrointestinal bleeding or kidney failure due to substitution to non-steroidal anti-inflammatory drugs, or all cause mortality. We measured all cause hospital use as admission to hospital or emergency department visits identified by claims in the inpatient file or outpatient claims. We categorized emergency department visits for pain by using claims with a primary diagnosis of pain (appendix table 1). Mental health crises included hospital visits with a primary diagnosis of depression, anxiety, or a suicide attempt (appendix table 1). We captured mortality using the Medicare beneficiary summary file. We also quantified beneficiaries’ annual rate of office visits to any type of provider, including specialist and primary care providers. Medicare spending included all charges listed on beneficiaries’ claims for the study period. For prescription outcomes, we used prescription data from Medicare part D (which also captures patients using Medicare Advantage) to estimate all filled prescription quarterly as well as mutually exclusive groups of opioid, buprenorphine, naloxone, and non-opioid prescription drugs.
We collected information on patients’ age, gender, race or ethnicity, state of residence, dual eligibility for Medicaid and Medicare coverage, Medicare Advantage enrollment, disability as the original reason for Medicare enrollment, diagnosis of chronic non-cancer pain (appendix table 1), and morphine milligram equivalent daily dose in the period before prescriber exit, so none of the covariates was causally influenced by future exposure or outcomes. 32 We quantified patients’ opioid total morphine milligram equivalent, average daily dose, and the total number of days supplied during the first four consecutive quarters a patient met the definition of LTOT. We calculated the total morphine milligram equivalent and days supplied from prescriptions’ generic ingredients for opioid compounds. 33 We calculated the average daily dose of opioids as the total morphine milligram equivalent of opioids supplied divided by the total number of days supplied during the initial LTOT episode.
We classified whether the patient’s county of residence was rural or urban, 34 and included 27 chronic conditions classified following prior work: acquired hyperthyroidism, acute myocardial infarction, Alzheimer’s disease, Alzheimer’s disease and related senile dementia disorders, anemia, asthma, atrial fibrillation, benign prostatic hyperplasia, cataract, chronic kidney disease, chronic obstructive pulmonary disease, diabetes, depression, heart failure, glaucoma, hip or pelvic fracture, hyperlipidemia, hypertension, ischemic heart disease, osteoporosis, rheumatoid arthritis or osteoarthritis, stroke or transient ischemic attack, breast cancer, colorectal cancer, prostate cancer, lung cancer, endometrial cancer. 3
Statistical analysis
We compared outcomes for matched exposed and unexposed patients receiving LTOT before and after prescriber exit in a difference-in-differences framework. The framework allowed us to estimate the average treatment effect of physician exit on exposed patients. The difference-in-differences design required two key assumptions in our context. We first assumed that outcomes would trend similarly for unexposed and exposed patients in the absence of treatment. We visually tested this assumption in figure 1 and figure 2 by observing whether outcomes for exposed and unexposed patients moved in parallel before treatment (visual inspection of pre-trends is another advantage of propensity score matching exposed and unexposed groups).We then assumed the exit of a patient’s assigned prescriber was independent of baseline patient outcomes. Table 1 shows that matching unexposed and exposed patients through a propensity score match effectively limited baseline differences between exposed and unexposed patients, supporting this assumption.

Unadjusted change in quarterly rates of long term opioid treatment (LTOT) discontinuation, clinical, and healthcare use outcomes for patients receiving LTOT who had a prescriber exit versus those who did not have a prescriber exit. Prescriber exit is denoted as quarter 0, with minus numbers indicating quarters before prescriber exit. The vertical dashed line delineates the periods before exit (left) and after exit (right). Appendix Table 4 shows adjusted quarterly point estimates. Appendix Figure 1a and 1b diagrams the sample construction. ED=emergency department
Patient characteristics for matched patients whose prescriber exited the workforce versus those whose prescriber remained the same
- View inline
We used linear regression at the level of quarter per patient to estimate a set of interaction terms between indicators for being an exposed patient and indicators for eight quarters relative to prescriber exit (four before exit and four after exit; details in appendix methods 3). The interaction terms describe the mean differential change in the outcome between unexposed and exposed patients by quarter relative to prescriber exit, using quarter −5 as the baseline period. All regression models also included patient and prescriber fixed effects (except for the outcome of mortality, which only contained prescriber fixed effects; appendix methods 3) 29 35 and clustered standard errors at the matched level of the prescriber pair. 30 The use of fixed effects controlled for time invariant differences among patients and prescribers, such as baseline age, race, sex, living in a rural area, reason and type of Medicare enrollment, and baseline chronic conditions.
In a separate set of models, we used the same regression approach, but estimated the differential change between unexposed and exposed patients by year relative to prescriber exit (one year before exit, one year after exit). All regression analyses at the year level defined the period before prescribe exit as quarters −5 to −2 before a prescriber’s exit, excluding quarter −1 to account for potential anticipation (appendix methods 2)
We assessed the robustness of findings with several alternative specifications, including examining patients qualifying for Medicare because of social security disability income as a separate subgroup; repeating adjusted analyses over the entire sample but excluding Medicare Advantage patients; and including patient with cancer. We also examined changes in outcomes attributable to prescriber exit among patients not receiving LTOT with the same prescribers as patients who were receiving LTOT to determine the effect of the exit alone. 30 Additionally, we compared treatment effects by whether the patient lost a primary care physician or specialist. We tested the sensitivity of results by adjusting for multiple comparisons and compared treated patients who discontinued to treated patients who did not discontinue to quantify how the provider’s exit alone affected estimates. The 95% confidence intervals (CI) reflected 0.025 in each tail or P≤0.05. Analyses were performed in Stata, version 16 (StataCorp LLC).
Patient and public involvement
No patients were involved in setting the research question or the outcome measures, nor were they involved in developing plans to design or implement the study. No patients advised the interpretation or writing up of results. We used previously collected, de-identified data purchased from the Centers for Medicare and Medicaid Services that is restricted use. The institutional review board at the Harvard TH Chan School of Public Health approved the study, waived informed consent, and did not require us to involve patients and the public in the research process.
Study sample
Before matching, the full study sample consisted of 80 158 exposed and 322 970 unexposed patients who received LTOT. Propensity score matching led to the exclusion of 32 079 exposed patients and 274 891 unexposed patients, leaving 48 079 patients assigned to 15 713 exiting prescribers (exposed) and 48 079 patients assigned to 28 150 stable prescribers (unexposed (appendix figure 1)). Propensity score matching improved balance on observable characteristics (appendix figures 3 and 4). After matching, patients in both exposed and not exposed groups had similar demographic and clinical characteristics, with almost all standardized mean differences of 0.05 or less ( table 1 ). Comparisons of exiting versus stable prescribers and patients receiving versus not receiving LTOT are in appendix tables 2 and 3.
LTOT discontinuation and prescription outcomes
In the first quarter after prescriber exit, the opioid discontinuation rate for exposed patients receiving LTOT increased from 132 to 229 per 10 000 patients per quarter, compared with 97 to 100 per 10 000 unexposed patients ( fig 2 ; adjusted difference of 1.22 percentage points ((95% CI 1.02 to 1.42), 160% increase from a baseline of 0.77%, appendix table 4). The adjusted yearly rate of discontinuation differentially increased 2.08 percentage points (1.66 to 2.50), or a 56% increase from the baseline 3.70% rate of discontinuation, for exposed patients relative to unexposed patients ( table 2 ). In the overall post-exit period, the yearly number of opioid prescriptions declined by 1.01 prescriptions ((95% CI −1.11 to −0.91) or −6% off the baseline mean of 15.71 prescriptions), total days’ supply of opioids declined by 29 days ((95% CI −31 to −26), or −7% off the baseline mean of 414 days’ supply), and total morphine milligram equivalent of opioids declined by 5311 morphine milligram equivalent ((95% CI −5759 to −4864), or −15% off baseline mean of 35 336 morphine milligram equivalent). Further, non-opioid prescriptions declined by 0.66 ((95% CI −1.03 to −0.3) or −1% off baseline mean of 59.68 prescriptions), and buprenorphine prescriptions increased by 0.03 ((0.01 to 0.05), or 25% off baseline mean of 0.12 prescriptions). Naloxone prescriptions increased by 0.0025 ((0.0002 to 0.0047), or 40% off baseline mean of 0.0047 prescriptions).
Adjusted differential change in annual rates of LTOT discontinuation, clinical, and healthcare use outcomes for LTOT patients receiving LTOT who were exposed versus not exposed to prescriber exit
Clinical and healthcare use outcomes
From quarter −5 to −1 before provider exit, unadjusted trends in outcomes were similar between exposed and unexposed patients receiving LTOT, supporting the parallel trends assumption needed for the differences-in-difference research design ( fig 3 ). Some outcomes showed potential anticipation of an upcoming prescriber exit, most notably mortality, which motivated our exclusion of quarter −1 from year-level regressions.

Adjusted differential change in quarterly rates of long term opioid treatment (LTOT) discontinuation, clinical, and healthcare use outcomes for patients receiving LTOT who had a prescriber exit versus those who did not have a prescriber exit. Prescriber exit is denoted as quarter 0, with minus numbers indicating quarters before prescriber exit. The vertical dashed line delineates the periods before exit (left) and after exit (right). Absolute risk difference estimates are from the matched difference-in-differences model described in the methods, with an indicator for outcomes for each quarter relative to exit. All cause mortality is modeled similarly but without patient fixed effects. Point estimates are relative to quarter −5 (ie, five quarters before prescriber exit). Regressions patient and prescriber fixed effects, and cluster at the prescriber level. Outer lines show the boundaries of the 95% confidence interval for each quarterly estimate. Appendix Figure 1a and 1b diagrams the sample construction. ED=emergency department
In the first quarter after prescriber exit (denoted quarter 0), when discontinuation rates were highest, a significant increase was noted in the rate of suicide attempts (0.05 absolute percentage points (95% CI 0.01 to 0.09); 122% increase off baseline 0.04% suicide attempt), opioid or alcohol withdrawals (0.14 absolute percentage points (0.004 to 0.28); 50% increase off baseline 0.28% withdrawal rate), and emergency department visits or admissions to hospital (0.04 visits (0.01 to 0.06); 9% increase off baseline 0.45 visits), including emergency department visits with a pain diagnosis (0.011 visits (0.002 to 0.02); 10% increase off baseline 0.11 visits with a pain diagnosis), compared with patients who had a prescriber that exited the workforce and those who had a continuous prescriber ( fig 3 and appendix table 4). Mortality declined by −0.15 percentage points ((95% CI −0.29 to −0.02); 52% decline off base of 0.5%). Significant differences receded by quarter two except for emergency department visits and admissions to hospital, which were 0.02 visits ((0.001 to 0.04); increase of 4.4% from baseline mean) higher among patients with a provider exit but then receded by quarter three. No significant change in the rate of overdose was noted across all quarters of the study period.
In adjusted analyses averaging across the whole post prescriber exit period, a significant increase was noted in opioid or alcohol withdrawal (0.31 percentage points (95% CI 0.041 to 0.58), or 31% increase from baseline mean of 0.99%) and mental health crises (0.39 (0.08 to 0.69), or a 24% increase from a 1.6% baseline mean) comparing patients who had prescriber workforce exit versus those who did not. Additionally, a significant decrease in mortality was recorded (−0.50 (−0.77 to −0.23), or −23% from baseline mean of 2.18%) ( table 2 ). No significant difference in percentage points was noted between patients who were exposed and unexposed in annual rates of drug overdose (−0.12 (−0.41 to 0.18), or 9% decrease from 1.37% baseline rate), suicide attempt (0.02 (−0.07 to 0.11), or 13% from 0.15% increase from baseline rate), gastrointestinal bleeding (0.33 (−0.07 to 0.73), or 11% increase from 2.87% baseline rate), or kidney failure (0.16 (−0.40 to 0.71), or 2% increase from 7.61% baseline rate).
To quantify the effect of only losing a prescriber on results, we examined changes in outcomes associated with prescriber exit among all patients who were not receiving LTOT but lost the same prescriber (appendix table 3, appendix table 5). The unadjusted differences reflect differences in the main results in table 2 that may be attributable to prescriber exit instead of to LTOT discontinuation. After the loss of a prescriber, increases among patients not receiving LTOT were noted for overdose (0.12 percentage points, or a 27% increase from baseline mean of 0.45%), anxiety (0.10, or a 59% increase from baseline mean of 0.17%), opioid or alcohol withdrawal (0.21, or a 47% increase from baseline mean of 0.45%), and mortality (0.90, or 16% increase from baseline rate of 5.68%). Additionally, differences in mental health crises (0.08, or 15% increase from the baseline mean of 0.55%) were small among patients not receiving LTOT when compared with estimates among patients receiving LTOT ( table 2 ).
Additional analyses
In sensitivity analyses, we focused on: beneficiaries qualifying for social security disability income (people with disabilities); excluding patients in Medicare Advantage; including patients with cancer; and separately, patients above or below median morphine milligram equivalent (median 54.36 average daily), which were all similar to the main analysis (appendix tables 6 and 7). Appendix table 8 replicates the main results focusing on patients who were alive after a prescriber’s exit. Appendix table 9 compares treatment effects modeled in table 2 by whether the patient’s main prescriber was a primary care physician or specialist, showing that effects are similar across the two groups. Appendix table 10 indicates that effects maintain significance when adjusting for multiple comparisons. Appendix table 11 compares exposed LTOT patients who did versus did not discontinue LTOT in response to prescriber exit, showing standardized mean differences of 0.12 or less.
Principal findings
The loss of a prescriber was associated with increased discontinuation of LTOT and transient, but significant, increases in adverse outcomes among patients, including suicide attempts, withdrawal, and admissions to hospital or emergency department visits. Rates of adverse outcomes among patients reverted to baseline rates within four to seven months after prescriber exit. However, a significant increase in mental health crises and opioid or alcohol withdrawal was noted on average across the full four quarters after the exit period. Despite these outcomes, drug overdose rates did not change. Our findings suggest that discontinuation of LTOT may be associated with a temporary period of negative health effects, not including overdose or mortality, after accounting for unobserved confounding.
While a small proportion of patients discontinued LTOT in both groups, a substantial increase in discontinuation was reported in the quarter after prescriber exit. As seen in figure 2 , the increase in the first quarter after a prescriber’s exit was not associated with any visible or regression estimated change in overdose rates, despite large increases that might be expected based on standard observational models. 7 15 21 The difference in results is likely because discontinuation is a clinical event associated with other health changes, and patients with discontinuation differ from others, preventing the estimation of a causal effect. 3 22 23 The small or null results over the post prescriber exit period for outcomes such as overdose suggest that despite potential harm, discontinuation of LTOT may have counteracting benefits, such as reduced overdose risk, for some patients. The overall reduction in mortality among patients receiving LTOT who had a prescriber exit supports the potential longer term benefit of shorter duration and lower dosage to LTOT, although we interpret our mortality results with caution given pre-exit period trends that diverged prior to prescriber exit ( fig 2 ).
The harm we do observe associated with discontinuation of LTOT could be related to low quality management of transitioning patients across prescribers. Most discontinuations have excessively rapid tapers, 3 and patients receiving LTOT are a population at high risk with many comorbidities who must frequently navigate substantial stigma in the health care system. 36 For instance, discontinuation may accompany distressing clinician abandonment of patients with LTOT and cause opioid withdrawal, emotional harm, and undertreated pain, events that were unobservable in claims data unless they result in diagnoses that we captured.
One question is whether the observed associations were attributable to prescriber exit rather than the accompanying rise in overdose. To address this, we focused on patients receiving LTOT and those who were not on this treatment but who lost the same prescriber. If the prescriber exit explains the effects, patients not receiving LTOT should be impacted similarly to those who were receiving LTOT after prescriber exit. Instead, analyses show that clinical outcomes for patients not on LTOT were either null or opposite to those observed for patients receiving LTOT after the loss of a prescriber. Therefore, the loss of the physician is unlikely to explain the observed results among patients on LTOT and, if anything, may lead to an underestimation of the effects of discontinuation.
Comparison with other studies
One clear conclusion is that the observed clinical effect of LTOT discontinuation is highly dependent on the methods used. Prior research on tapering or discontinuation of LTOT finds a doubling in the rate of overdose and mental health crises comparing populations with discontinuation directly to those without. 7 15 16 17 19 By contrast, the absence of change in overdose rates in our analysis is closer to other observational studies using techniques to control for unobserved confounding, which have found opioid discontinuation to have a small or null impact on rates of addiction related adverse events. 14 18 22 This discrepancy suggests that overdose risk is more likely to be misestimated in conventional approaches.
Policy implications
While the choice of methods is a technical issue, it has great relevance to patients and policymakers struggling with the clinical and public health challenges of LTOT. Some mechanisms are plausible by which LTOT discontinuation could lead to either benefit, harm, or a mixture of both. Both observational research 37 and randomized trials 38 describe that LTOT, especially at a high dose, is associated with a multitude of adverse outcomes. On the one hand, discontinuing LTOT without replacing the clinical role of LTOT could lead to untreated pain, withdrawal, or worsening of mental health issues as described previously. On the other hand, discontinuing LTOT could mitigate these risks and promote patient safety in some circumstances. The clinical and scientific uncertainty around this question suggests clinical equipoise to justify ongoing 39 40 41 and future randomized interventions that promote patient centered, clinically appropriate LTOT discontinuation or tapering to investigate how tapering LTOT can be done safely and respectfully while prioritizing quality of life.
Limitations
This study has several limitations. Firstly, the findings may not generalize to the entire Medicare population or to populations outside of Medicare beneficiaries. 32 Our sample was a younger, Medicare qualifying population: the average patient in our sample was 58.0 years old, and only 33% of the population was over age 65 years ( table 1 ). Additionally, the study may not generalize to discontinuations outside of those caused by losing a prescriber. Secondly, we cannot observe the reason for a prescriber’s exit, which may be associated with patient outcomes in certain circumstances. We attempt to circumvent this issue by controlling for prescriber specific factors by comparing patients receiving LTOT with patients not receiving LTOT with the same assigned, exiting prescriber, showing that effects are directionally opposed between the two groups. Thirdly, our findings may not apply to patients on LTOT not meeting the threshold of the restrictive definition used in this study, such as those receiving lower doses of opioids or those on LTOT for less than a year. Fourthly, our statistical power, as assessed by the size of confidence intervals in the adjusted results, does not enable us to rule out a low magnitude of harm in response to discontinuation. However, across multiple outcomes, point estimates were consistently close to zero, and they did not change in any consistent pattern with the timing of a large change in discontinuation associated with prescriber exit. Fifthly, we chose what we believe to be the best strategy to handle confounding, but other strategies might be equally valid.
An additional limitation is that, while our analysis overcomes the confounding in prior work, our statistical strategy leverages a specific group of patients: those who have their opioid prescriptions discontinued in response to the loss of a prescriber. For instance, the increase in buprenorphine and naloxone prescriptions may suggest that patients receive replacement prescribers who initiated treatment of opioid use disorder in response to the loss of their prescriber. Also, not all patients had their opioid prescriptions discontinued after losing their main prescriber, suggesting that our results do not generalize to all patients who are discontinued or tapered from opioids. Since we followed up patient outcomes for only four quarters, our study does not consider the effects on patients losing a prescriber beyond four quarters after prescriber exit. We also do not observe prescriptions not billed to Medicare. Finally, our definition of LTOT discontinuation follows prior work to support comparability across studies. However, our results might not generalize to all alternative definitions of LTOT and discontinuation.
This study finds a complex association between the discontinuation of LTOT coinciding with prescriber exit and subsequent health effects. The cessation of LTOT was linked to a short term increase in negative health events, such as suicide attempts and admissions to hospital, indicating a potential need for heightened mental health support during the transition. Despite this, we found no effect of discontinuation on overdose rates or mortality. These findings differ from prior evidence that did not control unobserved confounding, implying that the observed consequences of LTOT discontinuation may vary considerably depending on the methods used. This variation underscores the importance of randomized interventions to better understand how LTOT discontinuation can be managed safely and effectively.
What is known on the topic
Much research shows that discontinued versus continued long term opioid treatment (LTOT) is associated with an increased rate of overdoses and mental health crises
Uncertainty remains because studies used observational models comparing individuals that discontinue LTOT to those that do not, populations whose clinical profiles diverge around the time of LTOT changes
What this study adds
Unobserved confounding was accounted for by leveraging prescriber workforce exit as an external shock increasing LTOT discontinuation and quantify outcomes in a difference-in-differences analysis
Prescriber workforce exit significantly changed opioid prescriptions and short term increases in adverse events of opioid or alcohol withdrawal, suicide attempts, and admission to hospital, but overdose rates changed little
LTOT discontinuation may be associated with a temporary period of adverse health impacts after accounting for unobserved confounding
Ethics statements
Ethical approval.
The study was approved by the institutional review board at the Harvard TH Chan School of Public Heath.
Data availability statement
No additional data available.
Contributors: All authors contributed to the design and conduct of the study; management, analysis, and interpretation of the data; and preparation, review, or approval of the manuscript. MLB supervised the study and is the guarantor. The corresponding author attests that all listed authors meet authorship criteria and that no others meeting the criteria have been omitted.
Funding: Supported by grants from the Retirement Research Foundation for Aging and the National Institute on Aging (K23 AG058806, MLB). The National Institute of Aging had no role in the design and conduct of the study; collection, management, analysis, and interpretation of the data; preparation, review, or approval of the manuscript; and decision to submit the manuscript for publication. The funders had no role in considering the study design or in the collection, analysis, interpretation of data, writing of the report, or decision to submit the article for publication.
Competing interests: All authors have completed the ICMJE uniform disclosure form at www.icmje.org/disclosure-of-interest and declare: funding from Supported by grants from the Retirement Research Foundation for Aging and the National Institute on Aging; no support from any organization for the submitted work; no financial relationships with any organizations that might have an interest in the submitted work in the previous three years; no other relationships or activities that could appear to have influenced the submitted work.
Transparency: MLB affirms that the manuscript is an honest, accurate, and transparent account of the study being reported; that no important aspects of the study have been omitted; and that any discrepancies have been disclosed.
Dissemination to participants and related patient and public communities: Results will be shared through the dissemination teams at Harvard University, University of Minnesota, and Stanford University. Typical mediums include press releases, social media posts (Twitter, Instagram, Facebook, and LinkedIn), and emails sent directly to journalists representing outlets such as the New York Times, San Francisco Chronicle, CNN, Bloomberg, and Becker’s Hospital Review, which condense the study into its main findings.
Provenance and peer review: Not commissioned; externally peer reviewed.
This is an Open Access article distributed in accordance with the Creative Commons Attribution Non Commercial (CC BY-NC 4.0) license, which permits others to distribute, remix, adapt, build upon this work non-commercially, and license their derivative works on different terms, provided the original work is properly cited and the use is non-commercial. See: http://creativecommons.org/licenses/by-nc/4.0/ .
- Dahlhamer J ,
- Neprash HT ,
- Jeffery MM ,
- Hooten WM ,
- Tancredi DJ ,
- Fenton JJ ,
- Agnoli AL ,
- Haegerich TM ,
- O’Donnell J ,
- Alltucker K
- ↵ Human Rights Watch. Fears of prescribing hurt chronic pain patients. https://www.hrw.org/news/2018/12/18/us-fears-prescribing-hurt-chronic-pain-patients . Published 18 Dec 2018. Accessed 14 Jan 2022.
- Lovejoy TI ,
- Becker WC ,
- ↵ Mackey K, Anderson J, Bourne D, Chen E, Peterson K. Evidence brief: benefits and harms of long-term opioid dose reduction or discontinuation in patients with chronic pain. Department of Veterans Affairs (US); 2019. Accessed 5 May 2022. https://www.ncbi.nlm.nih.gov/books/NBK549202/
- Manhapra A ,
- Gordon KS ,
- Crystal S ,
- Hallvik SE ,
- El Ibrahimi S ,
- Johnston K ,
- DiPrete BL ,
- Ranapurwala SI ,
- Maierhofer CN ,
- Larochelle MR ,
- Clothier BA ,
- Goldsmith ES ,
- Bohnert ASB
- Tseregounis IE ,
- Tancredi DJ
- Husain JM ,
- LaRochelle M ,
- Keosaian J ,
- Lasser KE ,
- Liebschutz JM
- Morasco BJ ,
- Demidenko MI ,
- Meath THA ,
- Sinsky CA ,
- Shanafelt TD ,
- Dyrbye LN ,
- Sabety AH ,
- Carlasare LE ,
- McWilliams JM ,
- Hatfield LA
- ↵ Leuven E, Sianesi B. PSMATCH2: Stata module to perform full Mahalanobis and propensity score matching, common support graphing, and covariate imbalance testing. Published online 1 Feb 2018. Accessed 1 Dec 2023. https://ideas.repec.org//c/boc/bocode/s432001.html
- ↵ Chronic Conditions Data Warehouse. Center for Medicare and Medicaid Services; 2014. Accessed 25 Mar 2015. Number https://www.ccwdata.org/
- ↵ Center for Medicare and Medicaid Services. Opioid oral morphine milligram equivalent conversion factors. Accessed 13 Aug 2018. https://www.cms.gov/Medicare/Prescription-Drug-Coverage/PrescriptionDrugCovContra/Downloads/Opioid-Morphine-EQ-Conversion-Factors-April-2017.pdf
- ↵ Centers for disease control. NCHS Urban-Rural Classification Scheme for Counties. National Center for Health Statistics; 2019. Accessed 12 Jun 2019. https://www.cdc.gov/nchs/data_access/urban_rural.htm
- Angrist J ,
- Barnett ML ,
- Gravely A ,
- ↵ Gilman J. Evaluation of medical cannabis and prescription opioid taper support for reduction of pain and opioid dose in patients with chronic non-cancer pain. clinicaltrials.gov; 2022. Accessed 1 May 2022. https://clinicaltrials.gov/ct2/show/NCT04827992
- ↵ Lund University Hospital. Tapering of prescribed opioids in patients with long-term non-malignant pain - efficacy and effects on pain, pain cognitions, and quality of life (TOPIO): a study protocol for a randomized controlled clinical trial with a 12 month follow-up. clinicaltrials.gov; 2022. Accessed 1 May 2022. https://clinicaltrials.gov/ct2/show/NCT03485430
- ↵ Turcotte DD. Evaluation of a patient-centred, multidisciplinary opioid tapering program for individuals with chronic non-cancer pain on long term opioid therapy. clinicaltrials.gov; 2021. Accessed 1 May 2022. https://clinicaltrials.gov/ct2/show/NCT04902547
- Open access
- Published: 14 May 2024
The community of inquiry as a tool for measuring student engagement in blended massive open online courses (MOOCs): a case study of university students in a developing country
- John Kwame Eduafo Edumadze ORCID: orcid.org/0000-0003-2422-4909 1 , 2 &
- Desmond Welsey Govender 2
Smart Learning Environments volume 11 , Article number: 19 ( 2024 ) Cite this article
61 Accesses
Metrics details
While massive open online courses (MOOCs) promise to democratise access to education, the literature reveals a nuanced understanding of engagement in these settings, especially in resource-constrained environments. Blended MOOCs combine MOOCs and physical classroom settings of contents and instructions. This study extends this discourse by focusing on blended MOOCs, which remain under-explored in the context of developing countries. The blended MOOC at the University of Cape Coast (UCC), Ghana, deals with third-party MOOCs as open educational resources (OERs) integrated with campus-based courses. UCC students have been using such blended MOOCs since 2016, when all level 100 students were mandated to enrol in a course entitled Information Technology Skills (ITS101). ITS101 is aligned to courses in a MOOC platform called Alison as an OER. Students' engagement is key to their continued use and satisfaction with online learning, such as MOOCs. However, among all the e-learning modes, students' engagement is the lowest in MOOCs, leading to high dropout rates. Blended MOOCs are one of the techniques recommended to reverse the undesirability of MOOCs, including engagement. However, few studies have been conducted on students' engagement in blended MOOCs, especially among university students in sub-Saharan Africa using MOOCs as OERs. Thus, this paper aims to measure student engagement in blended MOOCs using the revised Community of Inquiry for university students in a developing country. The rationale is to determine whether factors affect engagement positively or negatively. A two-stage cluster sampling technique was used to determine the participants for this study. A list of blended MOOC classes offered at UCC was obtained from the staff's mailing list. In the first stage, academic levels (100, 200, 300 and 800) were randomly selected from the strata using a lottery sampling technique. In the second stage, another simple random selection of blended MOOC courses or classes was used within each selected academic level. All students in the selected classes were then included in the study. Partial Least Squares Structural Equation Modelling was used to validate the model on the predictive relationships existing among the four presences (cognitive, learning, social and teaching) and engagement. Results from the structural model analysis proved a statistically significant predictive relationship among the constructs within the model. Learning presence had the most significant effect on student engagement. Thus, it should be included as one of the presences in the community of inquiry.
Introduction
Envision an educational setting wherein students are actively engaged in their learning process, fostering collaboration among peers and engaging with their instructors locally and across various timeframes globally on a massive scale. This scenario is the promise of blending massive open online courses (MOOCs) with campus-based courses, which offer the potential to integrate the advantages of MOOCs, such as affordability, flexibility and accessibility, with the interactive and social components inherent in traditional campus-based face-to-face instruction (F2F) (Almutairi & White, 2018 ; Edumadze et al., 2022 ). With MOOCs, affordability deals with its fee component, which is typically free or very low-cost for certificates or specific course materials; flexibility is concerned with the self-paced, allowing learners to progress at their speed within enrolment windows or deadlines and accessibility looks at accessing MOOCs materials from any location with a stable internet connection, using a wide range of devices–phones, tablets, computers. This blended learning can bring about a transformative impact on the educational landscape of developing nations, especially at the tertiary level (Kruse & Pongratz, 2017 ), though also applicable at the secondary level (Koutsakas et al., 2020 ). Thus, MOOCs' scalability and adaptability across different educational levels are emphasised. The capacity of MOOCs to simultaneously give the same experience to tens of thousands of students breaks the pattern of conventional university education, having the potential to expand access to education and decrease educational costs (Groves, 2012 ). Increasing access has been the goal of all universities (Vieira et al., 2020 ), and any EdTech solution that “rides on the wings” of the internet can attain global reach (UNICEF Office of Innovation, 2022 ). Again, with the increasing cost of “bricks and mortar” education and the 'swallowing effect' of students' debt. As such, any mode of education that combines high-quality instructional delivery at the barest minimum cost has the potential to be embraced by education seekers. That is why MOOCs should occupy the attention of all learners, educators, teachers, administrators, and policy-makers. McNutt ( 2013 ) quotes journalist Fareed Zakaria's conversation with the prime minister of a large developing nation. This prime minister said delivering wireless internet to every region of his country would make higher education accessible. After that, he will tell students to attend free online courses from American colleges, like MOOCs, so that more people can acquire higher education. Though the Prime Minister oversimplified the solution, using MOOCs to increase access to tertiary education is an option that all must explore. Introducing teachers and students to the continuous use of innovative technology such as MOOCs is a way of leveraging technology in education, especially in cash-strapped institutions such as those in the global South, including Ghana. As a platform for online dissemination of academic content, MOOCs afford students unprecedented access to educational materials that are high-quality content and engaging.
MOOC is a method for distributing educational content via the Internet to anyone who desires to participate, with no restriction on the number of participants (Educause Learning Initiative, 2011). MOOCs are classes intended for many students (usually in hundreds of thousands) and made available anytime, anywhere, using any internet-driven device. They provide a free online educational experience, regardless of their prior entry qualification, training or education (OpenupEd, 2015 ). MOOCs open a world of educational possibilities for learners and lifelong learners alike, particularly in developing countries. Because of MOOCs' unlimited open access, a new breed of open educational resources (OERs) has emerged (Qaffas et al., 2020 ). HEIs are embracing this new breed in their on-campus courses (Kloos et al., 2015 ; Zhang, 2013 ). The embrace has resulted in blended or hybrid learning that includes any learning activity that integrates content or learning objects of MOOCs into a traditional campus-based curriculum of institutions. This educational technology integration strategy has opened avenues for HEIs, especially those from developing nations with no online learning components, to have a feel of online learning through the blend mentioned above. Through this means, students can access free but high-quality academic materials in the form of these OERs or open textbooks, which were previously very expensive or difficult to acquire. Through this integration, MOOCs represent an excellent opportunity to revolutionise blended learning across all levels of education, including organisations providing continuous professional development (Ulrich & Nedelcu, 2015 ). MOOCs also help in the classroom when licensed as the next generation of textbooks and become one of the tools a teacher uses to teach the course (TED, 2014 , 13:09). The name for the combination of MOOC and the on-campus course is currently contentious. Terms such as blended MOOC (bMOOC/ B-MOOC), hybrid MOOC (H-MOOC), wrapped MOOC and distributed flip, among others, have popped up (Almutairi & White, 2018 ; Bruff et al., 2013 ; Koller et al., 2013 ; Yousef et al., 2015 ).
Blended MOOCs come in several models, as explained by Edumadze et al. ( 2022 ), which include university-created MOOCs utilised for credit transfer and integrating other MOOCs within the curriculum. The latter can entail establishing official collaborations with MOOC providers or informal adoption where faculty and students engage autonomously. At UCC, the method follows the second informal model where external MOOCs are included in classroom instruction, either partially or wholly, to supplement or improve the standard course content. In this situation, MOOCs function as supplementary open educational resources (OERs), offering versatility in instructional approaches such as flipped classes and reference materials. This integration showcases a dynamic utilisation of MOOCs to enhance the teaching environment.
Walters ( 2014 ) suggested the following possibilities exist because of the blended MOOCs:
Unbundling content by moving away from a single source (Professor) to multiple sources (online, students, professors (when co-lecturing) and experts from the industry),
Making it possible for students to take some courses locally at their enrolled university and others as MOOCs with content from different providers (institutions and universities),
It is causing the changing of classroom spaces from large lecture halls to small learning spaces.
Universities and tutors adopt blended MOOCs for various reasons, among which are:
Making students aware of the MOOC phenomenon and trends (Holotescu et al., 2014 )
Enlarging knowledge/topics of the course, they have enrolled locally (Holotescu et al., 2014 )
Creating awareness of MOOC among lecturers.
Serving to introduce e-learning to stakeholders of HEIs that do not officially use such technology.
Introducing lifelong learning practice among students.
Serving as a way of participating in open education (i.e., open access, OER) among stakeholders.
Exposing students to teaching materials and pedagogies from other HEIs in different countries.
The benefits of blended MOOC are optimising student engagement, satisfaction, and learning (Bruff et al., 2013 ).
Notwithstanding the benefits of MOOCs, studies such as Onah et al. ( 2022 ) and Yusof et al. ( 2017 ) have identified learners' lack of engagement as a significant drawback leading to high dropout rates. Monitoring how much time students spend on learning activities promotes high-quality learning and reduces course dropouts (Hussain et al., 2018 ; Rahimi, 2024 ). Learners' engagement has been seen as the reason for creating blended MOOCs and a key to making MOOCs work. Contact North ( 2016 ) declared that educators, educationists, instructional designers, instructional technologists, and others with a stake in education should be concerned about how engaged students are in online activities. Furthermore, Contact North ( 2016 ) also made the following points:
Not all courses have high levels of student engagement, and some give students few chances to build their learning (Contact North, 2016 , p. 2).
Student engagement is the best way to predict how well students learn (Contact North, 2016 , p. 7).
Institutions should pay more attention to their understanding of instructional design, student engagement, and assessment (Contact North, 2016 , p. 7).
If we want more students to succeed, we should emphasise student engagement and learning design, and faculty should be very involved in this work (Contact North, 2016 , p. 7).
Other researchers have come to a similar conclusion on student engagement, among which are:
Student engagement is one of the best learning and personal growth predictors (Sukor et al., 2021 , p. 640).
Student engagement, learner interaction, and teacher presence explained many differences in student satisfaction and how much they thought they were learning in online learning environments (Gray & DiLoreto, 2016 , p. 1).
Different things inside and outside the classroom can affect students' engagement (Vezne et al., 2023 , p. 1866).
Students actively engaged in learning tend to do better in school (Deci & Ryan, 2000 , as cited in Vezne et al., 2023 , p. 1866). This is because they enjoy and see the value in their actions.
Student engagement strongly predicts educational results(Wang & Degol, 2014 , p. 3).
Student engagement predicts learning and school performance (Haw et al., 2022 , p. 226).
Student engagement represents a significant challenge in online learning, especially in blended MOOC environments. This challenge is not limited to specific geographies. However, it is a global concern impacting students, teachers, and academic institutions. Contact North ( 2016 ) underscores that educational institutions must critically reassess instructional design, student engagement, and assessment strategies to foster meaningful learning experiences. Garrison and Vaughan ( 2008 ) further highlight the importance of reevaluating course design in blended learning settings to optimise student engagement. These set the stage for understanding the universal relevance of student engagement in educational success.
However, the literature reveals a notable gap in the research concerning the impact of blended MOOCs on student engagement, particularly within the context of Ghana in Sub-Saharan Africa. Despite the proliferation of MOOCs globally, there is a marked scarcity of research on their effectiveness in developing countries, with these studies pointing to this gap (Almutairi & White, 2018 ; Maphosa & Maphosa, 2023 ; Mutisya & Thiong’o, 2021; Montgomery et al., 2015 ; Yunusa et al., 2021 ; Zakaria et al., 2019 ). Sub-Saharan Africa's unique challenges, such as limited internet access and diverse educational needs, present a compelling case for a tailored approach to educational technology (Maphosa & Maphosa, 2023 ). This study aims to bridge this gap by exploring how blended MOOCs can enhance student engagement and learning outcomes in such contexts. The specific focus on Ghana allows for a detailed examination of these dynamics, offering insights that are both locally relevant and globally applicable (Yunusa et al., 2021 ).
Building on the theoretical framework of the revised Community of Inquiry (RCoI), this research investigates their collective impact on student engagement in blended MOOCs. Empirically, this study addresses a critical gap by employing a robust methodological approach to explore the relationship between these presences and student engagement. This research validated a standardised scale to measure student engagement in blended MOOCs by combining instruments from Almutairi and White ( 2018 ) and Wertz ( 2022 ) leading to the standarisation of such instrument as identified as a gap for MOOCs by Deng et al. (2020). Study of this nature enhances the reliability and validity of the instrument leading to its standarisation.
Thus, this study aims to explore the impact of the revised Community of Inquiry (RCoI) framework elements—cognitive, learning, social and teaching presence—on student engagement in blended MOOCs at the University of Cape Coast, Ghana. Grounded in the RCoI framework, this research investigates the synergistic effect of its four presences on enhancing student engagement. The RCoI framework provides a robust theoretical lens through which the dynamics of engagement in blended learning environments can be examined (Garrison et al., 2000 ; Shea & Bidjerano, 2010 ). In achieving the stated aim, the following objectives will be pursued:
Analyse the Community of Inquiry (CoI) framework to identify indicators of student engagement in blended MOOCs.
Investigate the impact of the four CoI presences (teaching presence, cognitive presence, social presence, and learning presence) on student engagement in blended MOOCs.
Examine the specific contribution of learning presence within the CoI framework to student engagement in blended MOOCs.
In pursuance of the second question, the following hypotheses will be investigated:
H1: No significant relationship exists between cognitive presence and students' engagement with blended MOOCs.
H2: No significant relationship exists between learning presence and students' engagement with blended MOOCs.
H3: No significant relationship exists between social presence and students' engagement with blended MOOCs.
H4: No significant relationship exists between teaching presence and students' engagement with blended MOOCs.
Figure 1 shows the research framework for the study.
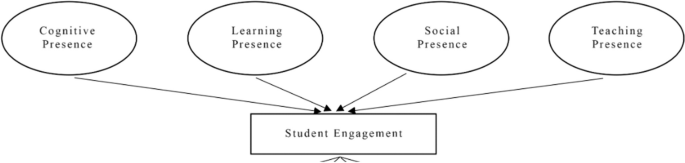
The research framework
This research addresses a vacuum in geographical research on blended MOOCs in Sub-Saharan Africa, notably Ghana, making a significant addition to educational technology’s literature. Examining student participation in this underrepresented region illuminates its unique educational problems and potential (Almutairi and White ( 2018 ). This study's regional is also relevant since Maphosa and Maphosa ( 2023 ) emphasise the necessity for MOOC initiatives in Sub-Saharan Africa. The study explores student engagement in blended MOOCs using the RCoI paradigm, enriching the theoretical landscape. The study confirms the theoretical validity of this approach, enhancing our understanding of how different presences affect student engagement synergistically. This research validated a standardised scale to measure student engagement in blended MOOCs, filling a gap identified by Deng et al. (2020). A rigorous engagement assessment tool improves blended learning empirical research by enabling more precise and replicable results across trials.
This research has significant implications for policymaking and instructional design, providing educators and designers with actionable insights to boost student engagement in blended learning. The study helps create more engaging blended learning experiences by identifying engagement tactics. Additionally, educational policies that promote equity and access are crucial, especially in regions facing infrastructural and resource limitations (King, 2015 ; Yunusa et al., 2021 ). Finally, this study adds to a more inclusive global educational technology debate by focusing on an underrepresented region with distinct educational needs and concerns. It ensures that Sub-Saharan African students' opinions are heard in educational technology transformation talks. This inclusive approach shows the study's dedication to a more equal and accessible learning landscape worldwide, promising to inform future educational policies and build a more inclusive educational environment for all.
The community of inquiry
As a social constructivist-collaborative paradigm, Garrison et al. (1999) initially suggested the Community of Inquiry (CoI) framework. The framework was built around three different ideas called "presences": cognitive presence (CP), social presence (SP) and teaching presence (TP). The way students interact with one another while taking an online course is called their "presence." Each node in the CoI framework is a conversation happening over the internet. The three presences impact learners' inquiry-based learning experiences within a learning community. Vaughan and Garrison ( 2008 ) said that CoI is founded on two fundamental notions for significantly higher education in an era powered by internet technologies–web 2.0 and social media: 'community' and 'inquiry'. The community recognises the social dimension of education, emphasising the need for interaction, collaboration, and conversation in knowledge construction (Arbaugh, 2007 ; Ranjan, 2020 ). The process of generating meaning through personal responsibility and choice is reflected in inquiry (Arbaugh, 2007 ). Again, Ranjan ( 2020 ) defined inquiry as how students develop meaning through their initiative and selection. Thus, CoI is a theoretical description of what makes an online learning environment engaging and cognitively developing. It is a robust and comprehensive approach to teaching, learning, and assessment.
Many authors recommend expanding the CoI framework's three presences to encompass several presences: autonomy, distributed teaching, emotional, instructor, engagement, vicarious and learning (Kozan & Caskurlu, 2018 ). These presences strengthen the CoI framework (Kozan & Caskurlu, 2018 ) and better describe the online educational experience (Anderson, 2017 ; Moore & Miller, 2022 ). The learning presence is the only planned addition that has been extensively embraced. Garrison and Anderson, CoI framework creators, have varied opinions about the learning presence. Anderson supports its inclusion, while Garrison rejects it. The learning presence introduces self-directed learning and transforms COI's "teaching model" into a "teaching and learning paradigm," expanding its use beyond traditional schools and educational settings, according to Anderson ( 2017 ). Though Anderson ( 2017 ) stated that the three presences provide a parsimonious advantage, Anderson ( 2017 ) argues that including learning presence in the CoI framework benefits:
The current CoI is only helpful for building and defining an efficient teaching framework.
It brings CoI closer to the principles of autonomous learning advocated by constructivist learning and heutagogical approaches to education.
It expands the scope of the CoI from purely pedagogical to one that incorporates learning, making it applicable in settings beyond the classroom. It has the learning potential, as demonstrated by the students.
Garrison ( 2017 ) argues against including learning presence in the CoI framework:
It contradicts the concept of a collaborative inquiry community. CoI members are supposed to have varied degrees of presence in the three areas. The teacher and learner are one in this scenario. Individuals have distinct responsibilities as teachers and students (Garrison, 2022 ).
Teaching and social presence were most related to learning presence, and cognitive presence emerged at the junction of these presences.
Adding more presences may increase framework complexity and violate parsimony principles.
The suggested presences can be incorporated into the model by expanding the scope of the three presences' definitions or the interrelationships between and among the three presences (Kozan & Caskurlu, 2018 ).
Shea ( 2010 ) suggested adding a "learning presence" to the CoI architecture. The suggestion came after observing that learner discourse—what students did—did not fit the then CoI model. Thus, Shea could not correctly code the identified discourse as social, cognitive, or teaching presences (Shea, 2010 ). Since online learning is electronic, social, and "self-directed," it was vital to study how students self- and co-regulate environments (Shea & Bidjerano, 2010 ). To do this, Shea and Bidjerano ( 2010 ) investigated various factors, such as the metacognitive abilities, motivational states and behavioural management strategies employed by successful online students. The lack of a learning presence that deals with the online learner role was observed as one of the limits to the CoI model (Shea & Bidjerano, 2010 ). The four presences of the RCoI framework are discussed in the subsequent sections.
Cognitive presence
Cognitive presence refers to the degree to which MOOC participants actively participate in substantive discussions and engage in critical thinking. The defining features of this phenomenon entail learners engaging in the exchange of ideas and perspectives, expanding upon one another's contributions, and critically examining their own and others' thought processes. With cognitive presence blended, MOOC participants are to participate in substantive discussions and engage actively in critical thinking. Cognitive presence significantly promotes learners' active participation and directly impacts their academic performance and overall satisfaction (Kang et al., 2007a , 2007b ).
Social presence
Social presence refers to the degree to which learners experience a sense of connection and belongingness with their peers and the instructor within blended MOOCs. The phenomenon is also marked by perceiving support from their peers and perceiving their ability to contribute to the learning process actively. It is widely recognised as crucial in establishing a conducive learning environment that fosters support and collaboration. A positive correlation exists between social presence and students' level of engagement in their academic pursuits and within the educational institution (Alabbasi, 2022 ). Social interactions among students contribute positively to their social integration and attitude towards the subject and foster a competitive learning environment (Damm, 2016 ). Finally, Miao and Ma ( 2022 ) observed that social presence directly impacts engagement and mediates the association between self-regulation and engagement.
Teaching presence
Teaching presence refers to the degree to which the instructor establishes a nurturing and interactive educational setting within a MOOC. Teaching presence encompasses the deliberate planning, facilitation, and guidance of cognitive and social activities to achieve meaningful and valuable learning outcomes. Empirical research has provided support for the positive impact of teaching presence on various learning outcomes, such as perceived learning, learner satisfaction, and behavioural engagement (Caskurlu et al., 2020 ). The existence of effective teaching plays a crucial role in fostering student engagement and influencing the outcomes of their learning experiences (Zhang et al., 2016 ).
Learning presence
The blended MOOC that this research is based on has the traditional roles of teachers and students assigned. However, it subscribed to the student-centredness of MOOCs, hence the inclusion of the learning presence in this study. Learning presence refers to the degree to which learners actively acquire knowledge and skills and assume accountability for their learning. The phenomenon under consideration is distinguished by the active involvement of learners in establishing objectives, monitoring their advancement, and actively seeking out educational resources to facilitate their learning process. Learners actively acquire knowledge and skills, ultimately attaining the intended educational objectives. Learning presence deals with active participation in the educational process and achieving intended educational objectives.
At first, "learning presence" represented academic self-efficacy and other cognitive, behavioural and motivational factors contributing to online students' capacity to self-regulate their learning (Shea & Bidjerano, 2010 ). Researchers have found that self-efficacy and self-regulated learning are strongly linked to other important factors for learning success. Shea and Bidjerano ( 2010 ) thought that self-efficacy and self-regulation were important parts of the learning presence. Self-efficacy and self-regulatory skills both affect e-learning success. However, they also affect each other in a learning environment. Doo and Bonk ( 2020 ) found that self-efficacy did not affect learning independently but helped self-regulated learning. Later, "learning presence" indicated self-regulated learning within a community of inquiry (Jimoyiannis & Tsiotakis, 2017 ; Shea & Bidjerano, 2012 ). Statistics show high relationships between self-efficacy and self-regulatory scores in online and traditional learning environments, such that high self-efficacy and positive self-regulation are reliable predictors of academic success in online courses(Bradley et al., 2017 ).
Zimmerman ( 2000 ) defines SRL as "self-generated thoughts, feelings, and actions that are planned and cyclically adapted to personal goals" (p. 14). SRL theorists consider meta-cognition, conduct, and motivation (Zimmerman, 1986 ). SRL strategies are "actions and processes directed at acquiring information or skill that involve agency, purpose, and instrumentality perceptions by learners" (Zimmerman, 1989 , p. 329). Examples are goal setting, time management, organisation, self-monitoring, and strategy adjustments. E-learning requires these skills because students’ study independently and at their speed. These variables help learners use e-learning materials. They can affect e-learning learners' motivation, engagement, persistence, and success. Self-regulated learning involves planning, monitoring, controlling, and regulating learning. Studies by Cho and Shen ( 2013 ) have shown that self-regulated learning (SRL) is essential for academic success in online learning environments. It directly contributes to students achieving their learning goals, especially in contexts with high student autonomy and minimal instructor presence.
Beyond self-regulation, co-regulated learning recognises that learning is often social and involves contact with others (Andrade et al., 2021 ). Assessment today involves co-regulation of learning through interactions with students, teachers, peers, and technology. Co-regulation occurs dynamically in online collaborative learning and may be detected and evaluated to improve learning design (Andrade et al., 2021 ). A study conducted by Liao et al. ( 2023 ) found that learning presence, specifically self-regulated learning (SRL) and co-regulated learning (CoRL), is a significant predictor of student engagement in blended learning. The researchers observed that CoRL is more strongly associated with emotional engagement, while SRL is more closely linked to cognitive engagement.
Students' self-efficacy is confidence in their ability to achieve in certain situations or tasks. Self-efficacy is "people's beliefs about their abilities to produce designated levels of performance that influence events that affect their lives" (Bandura, 1994, p. 71). With this, individuals cognitively appraise their capability to effectively perform and achieve desired outcomes in particular circumstances or tasks. Students with positive and comparatively high self-efficacy beliefs are more inclined to exhibit engagement in the classroom, as evidenced by their behaviour, cognition, and motivation (Linnenbrink & Pintrich, 2003a , 2003b ). According to Zhang ( 2022 ), self-efficacy significantly enhances learner engagement. Self-efficacy is a significant cognitive factor influencing motivation and engagement (Schunk & Mullen, 2012 ). Zhang ( 2022 ) revealed that self-efficacy emerged as the sole significant variable influencing learner engagement. Azila-Gbettor et al. ( 2021 ) conducted a study revealing that self-efficacy and autonomous motivation positively impact peer and intellectual engagement. Since self-efficacy, self-regulated learning and co-regulated learning are significant predictors of student engagement. Thus, learning presence predicts student engagement.
- Students’ engagement
One of the most important goals of e-learning environments in higher education is to get students more engaged in learning. This engagement is done through continuous interaction that builds cognitive and non-cognitive skills for success in school (Ituma, 2011 ). However, online learning such as MOOCs has considered problems inherent in retention and engagement (Lambert & Fisher, 2013 ). Thus, student engagement is critical for student retention and satisfaction in online courses. Fredricks et al. ( 2004 ) argue that within the research community, there remains a requirement for consensus about the definitions, frameworks, and constructs of engagement. Researchers employed a variety of indicators to predict learning outcomes and evaluate student engagement in various contexts. The analysis of indicators related to engagement is undertaken from a singular standpoint, operationalised through the participation of students in diverse activities. As an illustration, Wang et al. ( 2015 ) quantitatively analysed engagement levels within online discussion forums. Whitehill et al. ( 2015 ) employed measurements to assess the extent of video lecture viewership.
Furthermore, researchers have made numerous attempts to evaluate student engagement in addition to the abovementioned measures. For example, certain research investigations have employed self-report instruments, such as surveys or questionnaires, to collect data on students' subjective evaluations of their levels of engagement (Joksimović et al., 2018 ). Other studies have employed observational methodologies to directly assess students' behaviour and engagement levels in a classroom setting. However, some researchers have blended these approaches to acquire a more holistic comprehension of student engagement.
Student engagement in online courses refers to the degree to which students actively participate in critical thinking, verbal communication, and interaction with course materials, fellow students, and the instructor. Student participation in the learning process encompasses their engagement and collaboration with the instructor and their peers, indicating their level of involvement (Dixson, 2015a , 2015b ). Hodges ( 2018 ) defined engagement as assessing one's level of involvement, enthusiasm, and commitment to a company. Engagement is described as energy in action, the connection between a person and the exercise they perform for a stated goal in academic settings. So, the student's active participation in a task or activity is vital for engagement. Gallup's 2018 study, 'School Engagement Is More Than Just Talk,' discovered that:
Engaged children are 2.5 times more likely to report receiving outstanding grades and performing well. They are 4.5 times more likely than their actively disengaged peers to be optimistic about the future (Hodges, 2018 ).
Students engagement in the community of inquiry
Students' engagement is key to the success of the CoI model. One of the originators of the CoI framework and a colleague (Garrison & Vaughan, 2008 ) made the following statements attest to that fact. The efficacy of the inquiry technique relies on the presence of engagement; engagement is crucial for a community of inquiry and the overall higher educational experience (p. 16). The educational process within the community of inquiry entails both the public and private domains; engagement within a community of inquiry refers to the convergence of these public and private realms (p. 16). The inquiry approach in education encourages students to engage in responsible learning activities (p. 112) actively.
The CoI framework offers a distinct paradigm for identifying student engagement. It extends beyond mere assessment of engagement and explores the calibre of engagements, the extent of analytical reasoning, and the whole educational experience for students. The CoI framework assesses the instructor's ability to lead the course effectively (teaching presence), the students' interaction and contribution to the community (social presence), the students' active thinking and comprehension of the material (cognitive presence), and the students' proactive approach to managing their learning and supporting their peers (learning presence). The simultaneous collaboration of these four presences signifies a notable degree of student engagement. If any of these elements are lacking, it can identify areas where instructors can enhance their teaching tactics or the course design to cultivate a more captivating learning environment.
CoI for online learning can make students' engagement easier (Oyarzun & Morrison, 2013 ). In online education, a community of inquiry promotes “epistemic engagement “(Shea & Bidjerano, 2010 ). Epistemic engagement involves actively engaging with knowledge to deepen comprehension, thus making online learning environments more successful. The implication is that students are actively engaged in acquiring knowledge, employing critical thinking skills to analyse the subject matter, and actively participating in discussions with their peers and instructors to foster a comprehensive comprehension of the material. Students' engagement is vital for discovering knowledge to make learners active and instil lifelong learning capabilities in the digital era characterised by abundant information and learning initiatives, including MOOCs.
Choo et al. ( 2020 ) support this notion, highlighting the framework's usefulness in measuring engagement. Damm ( 2016 ) also confirms the effectiveness of the CoI survey in measuring engagement within MOOCs; nevertheless, the author acknowledges a limitation: the survey cannot definitively pinpoint the cause of low engagement (e.g., is it due to a lack of strong peer interaction?). Despite such limitations, a study by Das and Madhusudan (2023) assessed the CoI model's ability to promote collaborative learning and enhance engagement. The study further indicated that the CoI model significantly contributes to learner engagement, fosters collaborative learning, and improves learner performance across cognitive, emotional, and behavioural domains. Building on these findings, Ginting ( 2021 ) suggests further optimising the presences to enhance student engagement on online platforms.
Research methodology
The research methodology refers to the systematic approach and techniques employed in conducting a study. It encompasses the overall design and data collection. The present study used a quantitative research methodology to examine the RCol presences that impact student engagement within blended MOOCs serving as an open educational resource (OER).
Participants
Most respondents said they participated in blended MOOCs as the first-year University of UCC students. They enrolled on the Alison MOOC platform for Microsoft Office 2010 -Revised 2018 as OER for a campus-based course entitled ITS 101, which is mandatory for all level 100 (first-year) students. They watched the videos on the platform, participated in both the discussion forums and answered the quizzes to obtain a certificate. The marks on their certificate constituted part of their continual assessment for the campus course in which they enrolled. The continued students at the upper levels (200–900) registered unto other MOOC platforms like Saylor.
The research sample comprised 2875 students at the University of Cape Coast(UCC), Ghana, actively engaged in a blended MOOC centred on multiple courses. The total student population, which is the target population as of 2022–2023, was 60,243 (see Table 1 ), comprising 54,236 undergraduate and 6007 post-graduate students.
Only the regular group is involved with blended MOOCs, making them the target population for the study, which is 26,527 regular students. Table 2 shows the distribution of the students in the regular group.
Furthermore, 25,239 students from Table 2 are the research population. Except for students from levels 50, 250, 850, 900 and 950, most of these students have completed ITS 101: Information Technology Skills course at UCC. This course is a semester course based on blended MOOC instructional delivery. ITS101 is a mandatory course for all level 100 students at UCC. Some 25,239 students have again completed additional blended MOOC courses at levels 200–800. The blended MOOC was an open educational resource (OER), enabling unrestricted access and completion without associated costs. Lecturers selected MOOCs that fit their on-campus courses and instructed students to enrol. Upon completion, the marks students obtained from the MOOCs become part of the continuous assessment for their on-campus-based courses. Lecturers further organised supervised formative assessments on campus.
Sampling method
The sampling method selects a subset of individuals or items from a larger population to conduct research. The researchers employed a two-stage cluster sampling technique to determine the participants for this study. A list of blended MOOC classes offered at UCC was obtained from the staff's mailing list. Thus, the population was stratified by academic or year level (100–800). These levels 50, 250, 850, 900 and 950 were excluded as none of their courses uses blended MOOCs as an instructional format. In the first stage, academic levels were randomly selected from the strata using a lottery sampling technique. Each level of the population was assigned a level number beginning with 100. A single random sampling technique was used to select the levels participating in the research. The levels 100, 200, 300 and 800 were thus picked. In the second stage, another simple random selection of blended MOOC courses or classes was used within each selected academic level. All students in the selected classes were then included in the study.
Data collection
The Google survey questionnaire form was used to design the questionnaire and sent to all students within the stated categories (see Table 3 ) through their institutional email addresses to fill out—an estimated duration of 20 min for participants to complete the questionnaire. Tables 3 and 4 shows the level and class size of courses selected where students completed a blended MOOC course at UCC. Students from this population qualify to participate in the research study. Thus, the sample population shall be drawn from the accessible population. The benefit of using the Google Form approach is that all students have institutional mail based on the Google platform. There was a link to the survey in the email invitation. An introductory letter stating the purpose of the study and guaranteeing the student’s anonymity was added. It further explained to the students how the data would be used for academic purposes only, and they could decline it if they wanted to. They were also informed verbally and encouraged to respond to the questionnaire during classroom sessions. Reminder emails were sent bi-weekly to non-responders after two weeks following the initial survey invitation. The survey was open for the whole semester, and all responses were kept confidential.
Data analysis
After completing the data collection process, the responses to each research question were scrutinised and pruned to ensure that they aligned with the stated instructions. For each research question, the researcher gave the response a numerical code uploaded into SPSS version 28. A missing values analysis was then performed to ensure that missing data did not render the rest invalid. Later, a descriptive analysis was used to assess data distribution normality. The rationale is to know whether a parametric or non-parametric analysis approach is based on the data's skewness. The model is composed of four research questions. The researcher analysed it by converting the data to a comma-separated values file (CSV) and uploading it in SmartPLS 4 software for a partial least square regression (PLS). The approach employed in this study involved two key steps. Firstly, the variables were condensed into a reduced set of components to enhance manageability. Secondly, the analysis was performed on these condensed components instead of conducting a least-squares regression analysis on the original data. The Partial Least Squares (PLS) algorithm employs a methodology akin to principal components analysis to reduce the number of variables. This reduction is achieved by extracting components that capture the strongest correlations among the determinants (Hair et al., 2019 ). The methodology employed in this study involves utilising various components as variables, with the aid of cross-validation, to determine the smaller components that exhibit the highest level of predictive capability (Helland et al., 2018 ). Data were analysed using SPSS version 28 and SmartPLS version 4. Descriptive statistics were employed to comprehensively describe the sample and Community of Inquiry (CoI) dimensions. The researchers employed structural equation modelling (SEM) to examine the postulated associations between Community of Inquiry (CoI) and student engagement dimensions.
Limitations
The present study is subject to several limitations. The study was conducted within a single university (UCC) in Ghana. The generalisability of the study's findings to other settings may be limited. Furthermore, the investigation was carried out utilising a self-report questionnaire. This implies the existence of a potential for social desirability bias. Moreover, it is essential to note that the study employed a cross-sectional design (i.e., collecting data from multiple subjects at a specific moment), limiting its ability to establish causal relationships between the elements of conflict of CoI and student engagement. The study did not investigate additional variables that could impact student engagement in blended MOOCs, such as pre-existing knowledge or motivation.
Ethical considerations
The topic of ethical considerations is of paramount importance in academic discourse. It is crucial to carefully examine and address the ethical implications associated with it. Since this paper is extracted from the PhD dissertation of the lead author, the research was approved by the Institutional Review Board (IRB) of the University of KwaZulu-Natal, South Africa. The participants were provided with information regarding the study and provided their explicit consent to partake in the research. The data was gathered to ensure anonymity and stored in a secure facility. The participants were duly notified that their involvement in the study was entirely voluntary, and they were assured that they had the freedom to withdraw at any time without seeking permission from the researcher.
Response rate
The average response rate for research surveys is very different depending on the type of survey and the audience being surveyed. A meta-analysis of online surveys from various fields showed an average response rate of 39.6% with a standard deviation of 19.6% (Wu et al., 2022 ). The statement means that, on average, 39.6% of the people asked to participate in these online surveys did so. The standard deviation (SD) of 19.6% shows that the response rates to online surveys differed from one study to the other, running from as low as 20% to as high as 60% in some cases. Saunders ( 2014 ) also said that a response rate of around 30% in a random group is considered good. As indicated in Tables 3 and 4 , the researcher sent the questionnaire to all the 3506 students identified in the accessible population. A link to the same questionnaire was added to the Moodle LMS pages for courses that use blended MOOCs to teach. This mailing list comprises 3506 students who have used blended MOOCs at UCC for at least one semester. However, of the 3138 who completed the questionnaire, 2875 students filled it out successfully without missing data. The response rate was 82%, which is adequate for the study.
Demographic characteristics
Table 4 shows the demographic and biographical data of the respondents. EdTech research depends on these bio-demographic data, which is crucial for designing, implementing and evaluating technology interventions. They could help find subpopulations that need more complicated and subtle treatments, eliminate confusing factors and make policy decisions about using technology in the classroom. In a blended MOOC study, including demographic data, like sex, may assist researchers in determining whether gender sex affects learning. This information helps to ensure that instructional technology is sex-neutral and easy to use. Studies suggest sex inequalities in educational technology usage and acceptance. Several studies indicate that males are more tech-savvy than women (Irene, 2019 ).
In educational research, sorting is often used to tell the difference in perception and use of EdTech between students in STEM programmes and those who are not. The grouping is based on the idea that STEM and non-STEM students have different educational needs and experiences. Lin et al. ( 2021 ) reported that the factors affecting students’ intentions to continue using the platforms differed between STEM and non-STEM courses, with perceived usefulness being more important in STEM courses and perceived ease of use being more critical in non-STEM courses. Another study by Alkhalaf and Nguyen ( 2020 ) also showed that EdTech positively affects student learning outcomes in STEM and non-STEM courses. However, the effect was more significant in STEM courses than in non-STEM.
It is essential to consider the academic year of the respondents when analysing the data on satisfaction, academic performance and other variables related to blended MOOCs, as it may impact their experiences and perceptions of the technology. Additionally, understanding how experience with blended MOOCs varies by academic year can inform future design and implementation of such technologies. Based on the data, most respondents (85%) enrolled in blended MOOCs at level 100, indicating they were in their first year of study when they registered. This result suggests that most respondents were relatively new to blended MOOCs and may have needed more experience with them than more advanced students. Additionally, the small number of respondents at higher levels (300, 500, and 800) suggests that blended MOOCs may be less commonly used or required at higher levels of study.
Measurement model analyses
Two stages are involved in the analysis of PLS-SEM. The first is to analyse the validity and reliability of the measurement model. After successfully passing the validity and reliability tests, the subsequent step involves the analysis of the structural model, as Hair Jr et al. ( 2021a ) outlined. The data acquired from the study were subsequently analysed using the Partial Least Squares Structural Equation Modelling (PLS-SEM) technique in SmartPLS version 4.0.8.1. A path model is a graphical representation that illustrates the hypotheses and relationships between variables in a structural equation modelling (SEM) analysis, as Bollen (2002, as cited in Sarstedt et al., 2021 ) described. A path model includes structural and measurement models. In PLS-SEM, the outer models are measurement models, while the inner models are structural. The measurement model deals with the individual survey items and respective latent variables (constructs) measured by the former. The structural model shows the cause-and-effect relationships that deal with the latent variables and their linking relationships. Hair et al. ( 2021a ) suggested evaluating the elements discussed in the section to assess the study's measuring model.
Internal consistency
Internal consistency is usually judged by how items on the same construct relate to each other. It checks whether there is a link between the scores on different items meant to measure the same construct. It is also called internal reliability or internal consistency reliability.
Cronbach's alpha
Researchers can use internal consistency measures such as Cronbach's alpha, split-half, or test–retest reliability. These measures assess the consistency of responses to items or indicators over time or across different forms or versions of the same test. Cronbach’s alpha has been used for ages to check the reliability or consistency of each construct within the model. Cronbach’s alpha (α) is a simple way to determine a score’s reliability. It is used when more than one item measures the same underlying concept. Cronbach's alpha measures internal consistency and shows how closely related questions are as a group for a construct or latent variable (Ravinder & Saraswathi, 2020 ). The alpha value depends on how many indicator items are, how similar they are, and how many dimensions they have. The Cronbach’s alpha results should be between 0 and 1, but they can also get negative numbers. According to Tavakol and Dennick ( 2011 ), Cronbach’s alpha has certain limitations: scores with many items with lower reliability are generally associated with decreased accuracy. The Cronbach's alpha estimates for the constructs are presented in the fourth column of Table 5 . The Cronbach's alpha estimates varied between Student Engagement (0.861) and Learning Presence (0.943), above 0.722, passing the minimum threshold and making the items appropriate for each construct.
Composite reliability
Jöreskog and Sörbom's ( 1995 ) composite reliability(rho_C) is a statistical measure used to assess the internal consistency of scale items. It serves a similar purpose as Cronbach's alpha, as Netemeyer et al. ( 2003 ) discussed. It considers the reliability of a set of items when loaded on a latent construct. Composite reliability thresholds are still a matter of contention, with varying recommendations from researchers. A reasonable threshold can be anywhere from (Nunnally & Bernstein, 1994 ; Shivdas et al., 2020 ). The complexity of a construct is very variable concerning its number of components. Fewer items on a scale imply poorer reliability, with more items producing improved reliability. Within the realm of exploratory research, it is generally accepted that composite reliability ratings within the range of 0.60 to 0.70 are deemed acceptable; furthermore, ratings falling between 0.70 and 0.90 are considered indicative of an adequate level of reliability. Composite reliability scores above 0.90 (significantly above 0.95) show that some indicators are the same, hurting construct validity (Diamantopoulos et al., 2012 ). The composite reliability estimates for the constructs are presented in the sixth column of Table 5 . The composite reliability estimates varied between Student Engagement (0.894) and Learning Presence (0.95). The estimated values for the composite reliability of each construct exceeded the recommended minimum threshold of 0.70(Fornell & Larcker, 1981 ; Hair et al., 2021b ).
Reliability coefficient
Cronbach's alpha underestimates how reliable both latent variable scores are, while composite reliability overestimates their reliability (Dijkstra & Henseler, 2015 ). According to Hair et al. ( 2021b ), it was observed that Cronbach's alpha tends to yield conservative reliability estimates, while composite reliability tends to produce more liberal estimates. However, the actual reliability of a construct typically falls within the range between these two extremes. Hence, the reliability coefficient(rho_A) generally falls within the spectrum encompassing Cronbach's alpha and the composite reliability. The (rho_A) value can be between 0 and 1; the more reliable an item scale is, the higher the rho_A value. Higher rho_A values show more reliable item scales. The rho_A value of 0.7 is the bottom limit of adequacy (Ahmad & Hussain, 2019 ; Prasetyo et al., 2022 ). The results of the reliability coefficients of each construct are shown in the fifth column of Table 5 . By inspection, rho_A values range from Student Engagement (0.862) to Learning Presence (0.943). All the constructs met the recommended threshold, indicating that the values for reliability were significant and acceptable.
Construct validity
Construct validity is how well each item indicator assesses its intended idea or construct. Assessing construct validity is especially important when researching latent constructs, which cannot be directly measured or observed; thus, measurable indicators are needed. Convergent and discriminant validity are used to examine construct validity, and when both prerequisites are met, a test has construct validity. Convergent validity measures how similar indicators relating to the same construct are identical. In such a case, the indicators should have a strong correlation. Discriminant validity tests whether, theoretically, unrelated constructs have unrelated indicators. In such a case, the indicators should have no or weak correlation.
Convergent Validity
Convergent validity refers to the extent to which the indicators of a particular concept exhibit consistent alignment in their measurements. The process involves elucidating the differences among the various items. Examining the outer loadings of the different items and calculating the average variance extracted (AVE) allowed us to check the convergent validity of the constructs.
Outer loadings
When the outer loadings of the indicator items that measure a construct are high, the items that make up that construct have a lot to share in common (higher commonalities). This situation is termed indicator reliability. According to the recommendation by Hair et al. ( 2017 ), loadings of 0.708 or above are considered statistically significant. When the indicator's loading is above 0.708, the construct accounts for more than 50% of the indicator's variance, signalling that the indicator has sufficient item reliability (Sarstedt et al., 2021 ). Though enormous studies have suggested that outer loadings of 0.50 indicate low but significant reliability (Afthanorhan, 2013 ; Hair et al., 2019 ; Hulland, 1999 ), the researcher eliminated all items that showed outer loadings lower than 0.70 during the initial analysis because they were less significant, as per Hair et al. ( 2017 ). The removed items were coded as CEBE 1, 2, 7, 8, 9, 10, 11, 12, 13, 14, 15; CPTE1, 2; CPR3, LPER4, LPMSR10, LPSEL1, ME1, 5, 6, and 7. Thus, the remaining items showed item loadings ranging from 0.701(TPDO1) to 0.798(CPI3), as presented in the third column of Table 5 . These values indicate that the remaining items had significant indicator reliability and were included in the main study.
Average variance extracted
The average variance extracted (AVE) shows the variance of each indicator item explained by its construct. AVE assesses the extent to which the variance observed in a construct can be attributed to the construct rather than measurement error. To calculate AVE, square each indicator's loading and calculate the average. AVE for each construct is calculated by summing the squares of the standard errors of the indicator variances and then dividing by that amount. An acceptable value for AVE is 0.50 (Fornell & Larker, 1981). The seventh column of Table 5 shows that the measurement model's AVE values range from 0.546 to 0.609. The observation is that they were all more than the minimal value, making them acceptable. The indicator's outer loadings and AVE both pointed out that the remaining components of the measurement model possessed substantial convergent validity.
Discriminant validity
Discriminant validity pertains to the degree to which a latent variable exhibits dissimilarity from other latent variables and, thus, represents a phenomenon that other latent variables do not represent (Yeboah, 2020 ). Discriminant validity, sometimes called "divergent validity," looks at whether or not two things that are not supposed to be related are not. The discriminant validity method determines how unique the constructs being looked at are. The correlation between two ideas that do not go together will likely be weaker than the correlation between two things that go together (Nikolopoulou, 2022 ). In Smart PLS, there are three ways to determine if a discriminant is valid. These use a) cross-loadings, b) Fornell and Larcker's criteria, and c) the heterotrait-monotrait (HTMT) correlation ratio.
Cross-loadings
Cross-loadings are when an item significantly affects not just one but other factors. Items that load on two (or more) factors or a different factor than intended are said to have cross-loadings. The cross-loading principle posits that an item's loadings on its parent construct should exhibit greater magnitude than on any other research construct. Assume an item loads more on a distinct construct than its parent construct. It shows that the construct is not discriminatory and must be validated and improved to meet the standard. According to Yeboah ( 2020 ) and Yeboah and Nyagorme ( 2020 ), measurement items have significant convergent validity when their cross-loadings are estimated at least 0.708 and are higher on their respective constructs than their loadings on other constructs. As shown in Table 6 , the items used in the study that remained after removing those with lower outer loadings had the highest cross-loadings on their respective constructs (bolded) than other constructs. They were all greater than the minimum recommended value. Thus, the instrument was found to have significant discriminant validity and was suitable for the study.
Criteria of Fornell and Larcker
Based on this criterion, it is required that the correlation between a construct and other constructs should have values smaller than the square root of the average variance extracted by the construct. The Pearson correlation refers to the correlation coefficient that quantifies the relationship between the relevant item indicators in this measurement. According to Fornell and Larcker's criterion, establishing discriminant validity is contingent upon fulfilling a specified condition. The researcher evaluated the instrument's discriminant validity using the Fornell–Larker criterion presented in Table 7 . According to Yeboah ( 2020 ), the square root of the average variance extracted (AVE) for each construct, as indicated in the main diagonal, is anticipated to exceed the corresponding values in the vertical direction. The data presented in Table 7 suggest that the measurement model successfully meets the Fornell–Larker criterion.
Heterotrait-Monotrait (HTMT) ratio of correlation
The Heterotrait-Monotrait Ratio of Correlations (HTMT) is a metric utilised to evaluate the discriminant validity within the context of structural equation modelling (SEM) employing the partial least squares (PLS) approach (Henseler et al., 2015 ). The HTMT is grounded in the multitrait-multimethod matrix framework, which involves the examination of correlations between indicators of distinct constructs (heterotrait) and correlations between indicators of the same construct (monotrait) (Henseler et al., 2015 ). The HTMT is computed by dividing the mean of the heterotrait correlations and dividing it by the mean of the monotrait correlations, as Henseler et al. ( 2015 ) described.
The HTMT method offers certain benefits compared to alternative ways of evaluating discriminant validity, such as the Fornell–Larcker criterion and cross-loading analysis (Henseler et al., 2015 ). The HTMT method does not assume tau-equivalent measurement models, which are improbable to be applicable in most empirical research endeavours (Henseler et al., 2015 ). The HTMT measure proposed by Henseler et al. ( 2015 ) offers a more straightforward and intuitive approach to assessing discriminant validity. It accomplishes this by comparing the magnitude of relationships between constructs with the magnitude of relationships within constructs. According to Ringle et al. ( 2022 ), when the HTMT value falls below 0.90, it indicates the presence of discriminant validity between two constructs. The inference can be made that an HTMT value exceeding 0.90 suggests a deficiency in discriminant validity. However, it is recommended that researchers employ a threshold of 0.85 for the HTMT when there are substantial differences in the path model structures in terms of conceptualisation (Henseler et al., 2015 ). Per the cut-off value of 0.90 for the HTMT ratio defined by Henseler et al. ( 2015 ), the values shown in Table 8 are statistically significant. The HTMT values found for the constructs show that each construct in the model differed enough from the others and measured different characteristics. So, the measuring model could tell the difference between the two groups. Consequently, the measuring model successfully demonstrated discriminant validity.
Multicollinearity
When two independent predictors are highly correlated, we have a problem known as collinearity. Collinearity means that two predictors are linked linearly. Multicollinearity is a problem in multiple linear regression models when two or more independent variables (predictors) are highly correlated, meaning that the predictors can make accurate linear predictions about one from the other. Condition indices and variance inflation factors (VIFs) help find multicollinearity (Lindner et al., 2020 ). There are several approaches to deal with multicollinearity, such as a) deleting one or more independent variables from the fit, b) performing a main components regression, and c) removing variables having strong partial correlations with other variables (Lindner et al., 2020 ). Feldman ( 2018 ) suggested these general rules of thumb: a) there is no multicollinearity among the factors if VIF = 1, b) there is moderate multicollinearity if 1 < VIF < 5 and c) there is high multicollinearity, indicating much overlap if VIF > = 5.
Thus, a high VIF value means a construct is strongly linked to other constructs, making it hard to estimate and understand the coefficients. The VIF values of the model are shown in Table 9 .
The highest VIF number in the table is 2.252, which is below the standard threshold of 5 for finding high multicollinearity. This shows that there is no big problem with the model's constructs as they are not too similar. The lowest VIF number in the table is 1.000, meaning no multicollinearity exists between E and SP. The implication is that E and SP are two different constructs with no shared variance.In general, whereas social presence seems unrelated to other factors, cognitive presence, learning presence, and teaching presence show a modest level of multicollinearity. Nevertheless, Kock ( 2015 ) establishes that VIF values should not exceed a minimum threshold of 3.3. Thus, all VIF values from Table 9 were below Kock's ( 2015 ) suggested threshold, indicating that the estimated model was free from multicollinearity.
Discussion of the analysis of the structural model
It is necessary to conduct a structural model analysis to evaluate the hypothesised paths inside the calculated model for statistical significance. The methodology employed in this study involved a bootstrapping sequence consisting of 5000 resamples conducted using the Partial Least Squares Structural Equation Modelling (PLS-SEM) software. Figure 2 and Table 10 show what happened when the bootstrapping method was used.
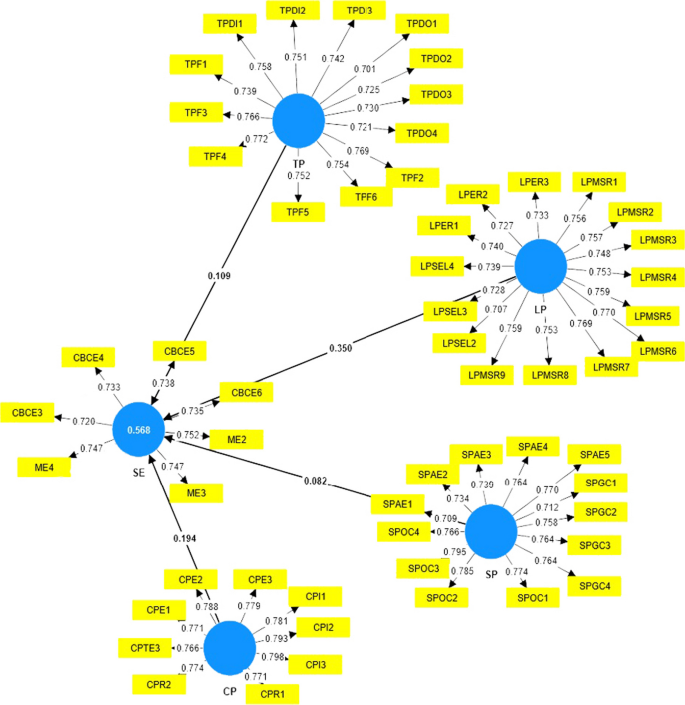
Diagram of the path analysis (path diagram) using SmartPLS
The community of inquiry (CoI) model by Garrison et al. ( 2000 ) and later extension from Shea and Bidjerano ( 2010 ) known as the revised community of inquiry (RCoI) emphasises the importance of teaching, social, cognitive and learning presences to a) promote meaningful learning engagement in online environments and b) understand and design effective online learning environments, including blended MOOCs. Students’ engagement has many dimensions. Since the study deals with blended MOOCs, the item indicators for engagement were adapted from the blended MOOC engagement model (Almutairi & White, 2018 ). In this part, the researchers discuss how four presences of RCoI are linked to student engagement with the help of the path diagram depicted in Fig. 2 .
Teaching presence and students' engagement
H1 : Teaching presence (TP) will positively impact students' engagement (SE) in the blended MOOC system.
The relationship between teaching presence (TP) and student engagement (SE) in blended MOOCs is complicated. Although TP has been demonstrated to improve SE, the magnitude of this effect depends on context and demographics. This idea is significant because it helps us understand how TP affects SE in blended MOOCs.
The statistics for the relationship between TP and SE are β = 0.109, t = 5.574 > 1.96 for α = 0.05, p = 0. 000, CI (0.072, 0.149), f 2 = 0.015 .
The Cohen’s effect size ( f 2 ) is classified as: a) 0.00 ≤ f 2 < 0.20 as Negligible; b) 0.20 ≤ f 2 < 0.50 as Small; c) 0.50 ≤ f 2 < 0.80 as Moderate and d) 0.80 ≤ f 2 is as Large (Cohen, 1988 ; Hair et al., 2017 ). Little is known about the effect of teaching presence on student engagement in blended MOOCs. However, the empirical evidence from this study found a positive relationship between TP and SE, with a path coefficient of β = 0.109 and statistical significance at p = 0.000, supporting the idea that TP is a determinant of SE in blended MOOCs (Littler, 2024 ). Although statistically significant, the impact size ( f 2 = 0.015) is negligible, explaining only 1.5% of SE variation(Hair et al., 2017 ). This result suggests investigating other variables that may moderate or mediate TP and SE.
This result aligns with the community of inquiry framework, which emphasizes the importance of TP, alongside social and cognitive presence, in fostering a rich educational experience (Garrison et al., 2000 ). The implications of these findings are significant for the design and delivery of blended MOOCs. They suggest that TP, which includes the design, facilitation, and direction of other presence or processes to support learning (Anderson et al., 2001 ), is a key factor in promoting student engagement. This is consistent with research suggesting that TP influences learning persistence in MOOCs (Jung & Lee, 2018 ). Based on the blended MOOC setting, teaching presence (TP) can affect student engagement (SE) differently. Course material, teaching tactics, and platform features can affect TP effectiveness. TP may significantly affect SE in courses requiring more cognitive presence (Cui et al., 2024 ; Pakula, 2024 ; Su, 2023). TP may affect SE differently depending on the MOOC's discipline. STEM and non-STEM courses have varied material and student engagement so that the TP may be perceived differently. TP effectiveness can also be affected by instructional design and student variables like age, level, culture, and online learning experience. These differences show that TP may not predict SE in all learning circumstances. TP can alter SE depending on student age, education, and culture. These aspects must be considered when evaluating data and exploring how TP can meet varied learner needs (Agarwal, 2021 ). The clarity of instructional objectives, content arrangement, and interactive components of the MOOC can increase or decrease TP's influence in SE (Agarwal, 2021 ; He et al., 2023 ). Well-designed TP-aligned courses may have a more significant impact on SE (Agarwal, 2021 ). According to the study, various characteristics may mediate or moderate TP-SE. Future research should examine mediators like cognitive and social presence and moderators such as student motivation and self-regulation (He et al., 2023 ; Su et al., 2023). Even though the effect size is minimal, the positive link between TP and SE emphasises its importance in blended MOOCs. For SE, educators and instructional designers could strengthen TP by giving clear guidance, timely feedback, and promoting dialogue (He et al., 2023 ; Pakula, 2024 ; Su et al., 2023).
Cognitive presence and students' engagement
H2 : The students' cognitive presence (CP) will positively impact their engagement (SE) in the blended MOOC system.
H2 suggests that students' cognitive presence (CP) will boost their blended MOOC engagement (SE). Deep online learning relies on cognitive presence, the amount to which learners can generate meaning through persistent conversation (Garrison & Akyol, 2013 ). CP is crucial to meaningful learning in blended MOOCs, as Garrison et al. ( 2003 ) noted. This study confirms this.
The statistics for the relationship between CP and SE are β = 0.194, t = 7.140 > 1.96 for α = 0.05, p = 0.000, f 2 = 0.036, CI (0.141, 0.247 ).
Analysis shows a significant positive relationship between CP and SE, with a path coefficient of β = 0.194. This substantial outcome (p-value < 0.001) supports the idea that CP is critical in fostering student engagement in blended MOOCs. This study’s result demonstrates that CP significantly impacts SE, supporting Lee's ( 2014 ) findings that higher CP correlates with increasing student engagement in online debates. Although significant, the impact size ( f 2 = 0.036) is negligible, explaining only 3.6% of SE variance. This outcome suggests that while CP is undoubtedly important, its overall impact on SE may be influenced by other factors or presences within the blended MOOC context.
In the context of blended MOOC systems, cognitive presence is crucial as it reflects the extent to which learners are able to construct and confirm meaning through sustained reflection and discourse (Garrison et al., 2001 ). A higher cognitive presence is associated with deeper levels of learning and understanding, which can lead to increased student engagement—a key indicator of successful learning outcomes (Chi, 2023 ). This assertion shows that deeper knowledge construction promotes engagement in the learning environment. This relationship may not be isolated. Blended MOOCs with collaborative tools and discussion forums can boost CP's impact on SE (Akyol & Garrison, 2013 ). CP affects Student Engagement (SE) together with teaching, social, and learning presence (Littler, 2024 ); Maranna et al., 2022 ). These interactions can result from a more holistic learning environment that fosters SE (Garrison et al., 2010 ). While our data show that CP directly affects SE, they also suggest that its position in the CoI framework has to be better understood. In future studies, these intricate interrelationships could inform blended learning instructional design and pedagogy. CP may mediate TP and SE, improving student satisfaction and learning (Shea et al., 2003 ). More research on how CP interacts with SP and LP may yield more insights.
Social presence and students' engagement
H3 : The students' social presence (SP) will positively influence their engagement (SE) in the blended MOOC system
The idea that social presence (SP) dramatically affects students' engagement (SE) in blended MOOCs yields intriguing results(Bergdahl et al., 2020 ; Ma et al., 2022 ). Online and blended learning settings depend on social presence—the feeling of belonging and mutual support—to build a sense of community.
The statistics for the relationship between SP and SE are β = 0.082, t = 3.595 > 1.96 for α = 0.05, p = 0.000, CI (0.039, 0.128), f 2 = 0.007) .
This study found a positive correlation between SP and SE, with a path coefficient of β = 0.082, showing that an increase in SP leads to an increase in SE. A significant connection (p-value < 0.001) supports that SP positively impacts student engagement in blended MOOCs. Although statistically significant, the effect size ( f 2 = 0.007) indicates a low practical influence, accounting for only 0.7% of SE variance. This negligible effect size (Hair et al., 2017 ) forces us to examine SP's complex role in student engagement and its connection with the Community of Inquiry (CoI) framework.
The statistical results support the theory that social presence (social interactions and a sense of belonging in online learning environments) increase student engagement (Garrison et al., 2000 ; Littler, 2024 ). The positive β coefficient indicates that social presence promotes student engagement, although the effect size ( f 2 ) is considered negligible by Cohen's ( 1988 ) standards (Hair et al., 2017 ). This negligible effect size raises questions regarding social presence's multifaceted significance in student engagement. Despite its negligible effect size, social presence creates a compelling learning environment (Littler, 2024 ). Furthermore, a strong social presence in online courses can make learning more inclusive and engaging, increasing satisfaction and retention (According to Garrison et al., 2000 ; Littler, 2024 ; Shea & Bidjerano, 2009 ).
SP is crucial to SE, yet it is only one of several factors as engagement is multidimensional, including behavioural, emotional, and cognitive aspects (Fredricks et al., 2004 ). SP may mediate CP and SE by improving cognitive engagement with course content in a supportive social environment (Shea et al., 2003 ). Gupta et al. ( 2024 ) highlight how social media and digital platforms may inform instructors about student participation and build a sense of belonging in virtual learning environments. Blended MOOCs with collaborative tools and discussion forums can boost SP's impact on SE (Akyol & Garrison, 2013 ; Gupta et al., 2024 ). Backgrounds and experiences can affect students' SP perception and participation (Almasi & Zhu, 2023). However, the overarching theme of individual differences in engagement and the role of digital platforms might imply the need to consider these factors. Educational practitioners and instructional designers could promote online discussions and group activities to boost social presence while also addressing other engagement factors.
Learning presence and students' engagement
H4 : The students' learning presence (LP) will positively impact their engagement (SE) in the blended MOOC system.
Learning presence(LP)–a composite of self-efficacy and other cognitive, behavioural and emotional characteristics that help online learners self- and co-regulate–significantly affects their engagement (SE) in blended MOOCs.
The statistics for the relationship between LP and SE are β = 0.350, t = 13.04 > 1.96 for α = 0.05, p = 0.000, f 2 = 0.136, CI(0.299, 0.403).
The association between LP and SE is substantially supported by empirical evidence, with a significant path coefficient of β = 0.350 (p < 0.001). With an f 2 = 0.136, LP accounts for 13.6% of SE variation, making it the strongest predictor of CoI's presence in this study, though negligible. This result indicates that as students' learning presence increases, their engagement in the blended MOOC system also tends to increase (Angelaina & Jimoyiannis, 2012 ; Popescu & Badea, 2020 ; Richardson & Swan, 2003 ; Wicks et al., 2015 ). Learning presence (LP) is a multifaceted construct encompassing self-efficacy and cognitive, behavioural, and emotional characteristics that facilitate online learners' self- and co-regulation. This, in turn, significantly influences their engagement (SE) in blended MOOC environments. The empirical support for the association between LP and SE is substantial, indicating that LP accounts for approximately 13.6% of the variance in SE, thus emerging as the strongest predictor of the revised Community of Inquiry's (RCoI) presence within this study, albeit the effect size being small. This result shows that students' blended MOOC engagement increases with their LP. Studies show that LP is crucial to SE in blended MOOCs (Angelaina & Jimoyiannis, 2012 ; Popescu & Badea, 2020 ; Richardson & Swan, 2003 ; Wicks et al.,2015). The impact of LP on blended learning environments' instructional design and pedagogy goes beyond SE (Shea & Bidjerano, 2010 ). In blended learning, contextual factors like teaching presence and individual factors like self-regulated learning (SRL) and co-regulated learning (CoRL) affect student engagement (Liao et al., 2023 ). Academic self-efficacy (ASE) has also been shown to improve academic success in online learning (Wolverton et al., 2020 ). Self-efficacy affects students' motivation, learning strategies, and online learning engagement and achievement (Bedi, 2023 ; Saefudin & Yusoff, 2021 ; She et al., 2021 ). This study shows that LP is essential in hybrid MOOCs. Educators can boost student engagement and ensure blended learning success by creating an LP-friendly learning environment. By prioritising LP and its components, educators may create successful and engaging blended learning experiences for students. Such an impact shows how important LP is in blended MOOCs for fully engaged learning. The findings have significant implications for blended learning instructional design and instructor practices due to LP's significant impact on SE.
Previous studies have shown that the CoI framework is essential for increasing engagement in online and blended learning environments, which these results support (Garrison & Cleveland-Innes, 2005 ; Garrison et al., 2010 ; Shea et al., 2010 ; Veletsianos & Kimmons, 2012 ). The CoI framework stresses creating a supportive, interactive learning community where students feel involved and driven to participate (Garrison et al., 2010 ). The results of the hypotheses show that the four presences can make students more engaged and interested in blended MOOCs. Therefore, the instructional design of blended learning courses must consider these elements to create an environment conducive to student engagement and learning.
Coefficient of determination
The coefficient of determination (R 2 , R2 or R-square) is a statistical metric that quantifies the extent to which one or more independent variables can account for the variability in a dependent variable in a regression model. The coefficient of determination is bounded within the range of 0 and 1. A value of 0 signifies that the model does not account for any variation observed in the response variable relative to its mean. In contrast, a value of 1 indicates that the model accounts for all the variation observed in the response variable relative to its mean. The coefficient of determination holds significance as it aids in assessing the degree to which data aligns with a statistical model. The purpose of its usage is to determine the degree of fit between a regression model and the observed data. When the value of R 2 approaches 0, it indicates a lack of correlation between the independent and dependent variables. When the value of R 2 comes to 1, it means a significant correlation between the independent and dependent variables.
Falk and Miller ( 1992 ) proposed a minimum threshold of 0.10 for the coefficient of determination (R2). Cohen (1998) proposed R2 values to assess the strength of endogenous latent variables, categorising them as substantial (0.26), moderate (0.13), and weak (0.02). Additionally, Chin ( 1998 ) proposed that the R2 values for endogenous latent variables can be categorised as follows: 0.67 (indicating a substantial relationship), 0.33 (indicating a moderate relationship), and 0.19 (indicating a weak relationship). The R square score for the model is 0.568, which shows a moderately positive link between the four presences of CoI and students' engagement in blended MOOCs (Chin, 1998 ). This result indicates that when the four presences of CoI are present, students are more likely to be interested in the course. The result for R 2 , which is 0.568, indicates that the four presences of CoI explain 56.8% of the difference in how engaged students are in blended MOOCs. This result means that the four presences of CoI are essential factors that affect how engaged students are in blended MOOCs. The path coefficient of SP (0.082) suggests a weaker relationship than the other three presences, while the highest path coefficient is for LP (0.350), which offers a strong relationship. The result indicates that LP is the most influential presence among the four in promoting blended MOOCs. The high path coefficient for LP shows that having a supportive environment that makes it easy for learners to self-regulate their learning is critical if researchers want to get students involved in blended MOOCs. On the other hand, CP and TP can be grown by helping learners with their training and teaching.
Q-squared validation
Predictive relevance, denoted by Q 2 , Q-squared or Q2, is another relevant indicator of the significant paths in the model's validity. Predictive relevance scores of 0.02, 0.15, and 0.35 indicate low, medium, and high relevance, respectively, as stated by Hair et al., ( 2021a , 2021b , 2021c ). Thus, the Q 2 value greater than zero indicates that the model has predictive relevance for the corresponding endogenous construct, students’ engagement for this study. The present study employs the Q-squared (Q2) validation technique to ascertain the significance of paths within the proposed model. Table 11 shows that the Q2 value for social presence is 0.347, indicating that the model has predictive relevance for this construct. The Q 2 values for TP, CP, and LP are all 0, indicating that the model does not have predictive relevance for these constructs. This implies that the model is better at predicting social presence than it is at predicting teaching, cognitive, or social presence.
The interpretation of the Q 2 values I gave earlier suggests that the model is better at predicting learning presence than it is at predicting teaching presence, cognitive presence, or social presence. This means the model has more predictive relevance for Learning Presence than the other three constructs. In practical terms, this could mean that interventions aimed at improving learning presence may be more effective at increasing student engagement than interventions aimed at enhancing teaching presence, cognitive presence, or social presence. However, it is important to note that this is just one way to interpret the results and further analysis may be needed to draw more definitive conclusions.
Importance-performance map analysis
The Importance-Performance Map Analysis (IPMA) is a valuable tool for decision-making because it provides a clear and concise representation of complex data and helps decision-makers identify underperforming and overperforming factors. This result makes it easy to identify trade-offs between competing priorities. The IPMA that SmartPLS makes is a graph with two dimensions. The horizontal axis shows each factor's importance, and the vertical line shows how well each factor performs.
"Importance" is how respondents or stakeholders value a construct or factor. It shows how significant each construct is thought to be. Higher scores from 0 to 1 indicate that respondents value the construct (Ringle & Sarstedt, 2016 ).
Performance is how well each construct performs compared to what respondents expected. It shows how well or effectively each construct works. Lower ratings indicate from 100 to 0 that the construct is not working as well as planned(Ringle & Sarstedt, 2016 ).
On the line, each factor is shown by a dot. The dot size shows how often the respondent talked about that factor. The factor's importance is indicated by where the dot falls. To use the IPMA for decision-making, we can split the constructs into four quadrants based on their importance and performance. From Ringle and Sarstedt ( 2016 ), we interpret the four quadrants as indicated below:
The constructs in the upper-right quadrant are very important and perform highly to the respondents, meaning they work well and should be kept.
The constructs in the lower-right quadrant are important but have low success ratings, so they need improvement.
The constructs in the upper-left quadrant are not very important but have a high-performance rate, which means they are doing too well, and resources could be moved elsewhere. They are an example of possible overdoing.
The lower-left section has unimportant constructs that do not perform well, which means they can be moved around or thrown out.
When assigning priorities, the constructions that fall into the upper-right quadrant are seen as having a high priority, whilst the constructs that fall into the lower-left quadrant are regarded as having a low priority.
As described in Table 12 , the IPMA aims to identify direct and indirect exogenous variables within the model that exhibit strong performance or significant importance about the endogenous variable, which pertains to students' engagement(Hair Jr et al., 2021a; Ringle & Sarstedt, 2016 ). The performance measure is based on how well the direct variables have contributed to engagement using the available resources within a given time frame. The importance index quantifies how important each construct is in forecasting student engagement. The performance index is a metric that quantifies the average score of each construct.
The findings indicate that learning presence holds the highest importance, as evidenced by its index value of 0.225. It is closely followed by cognitive presence, which has an importance index of 0.125. Teaching presence ranks third in importance, with an index value of 0.07. Lastly, social presence demonstrates the lowest level of importance, as indicated by its importance index of 0.053. This finding implies that the primary determinant of student engagement is learning presence, with cognitive, teaching, and social presence as secondary factors. Concerning performance, each of the four constructs demonstrates relatively high-performance indices. Teaching presence exhibits the highest score (81.728), followed by cognitive presence (81.553), learning presence (81.126), and social presence (79.684). This observation implies that all four constructs exhibit satisfactory performance regarding their influence on student engagement.
Implications relating to the effects of students’ engagement within blended MOOCs
The findings suggest that the four presences of the revised Community of Inquiry (RCoI) framework, namely teaching, cognitive, social, and learning presence, significantly influence student engagement in blended MOOCs. Institutions offering blended MOOCs should focus on creating a learning environment that supports all four presence. Again, institutions offering blended MOOCs should focus on designing courses that promote these four presences. Similarly, institutions that provide blended MOOCs should put the most effort into making classes that help people grow the four presences.
The result shows that learning presence significantly affects students' engagement more than cognitive, teaching, and social presence in that order. Institutions that offer blended MOOCs should prioritise creating a good learning environment that encourages peer-to-peer learning, teamwork, idea sharing, and getting feedback from instructors and other students. The results also show that the four presences of RCoI explain 56.8% of the difference in student engagement levels in blended MOOCs, as demonstrated by the R square score of 0.568. This statement suggests that other things, such as students' attentiveness, the amount of academic difficulty, and the intellectual work they do, also affect how engaged they are (Ginting, 2021 ). As student engagement is a complex concept, academic institutions that offer blended MOOCs should broadly promote it.
Although statistical research forms a solid basis for comprehending these patterns, it is crucial to convert these findings into practical methods for educators, instructional designers, and policymakers who seek to optimise blended MOOCs to improve student engagement. Below are some specific ways to use these results in their blended MOOCs.
For educators and professionals in instructional design
Fostering teaching presence : To cultivate teaching presence, educators should prioritise cultivating effective communication channels, providing timely and constructive feedback, and establishing a nurturing online learning environment. Training programmes can be developed to improve instructors' digital pedagogical abilities, ensuring they are well-prepared to engage students in blended MOOCs effectively.
Enhancing cognitive presence: To enhance cognitive presence, instructional designers should integrate activities that foster critical thinking and facilitate knowledge creation. These activities may include case studies, problem-solving tasks, and assignments that undergo peer review. Course structures can promote active discourse and introspection, fostering a more profound comprehension and involvement with the subject matter.
Developing social presence : Facilitating student engagement through platforms such as discussion boards, collaborative projects, and peer feedback sessions can foster a strong feeling of community inside blended MOOCs. By integrating synchronous components, such as real-time webinars or virtual office hours, the immediacy of social interactions can be further intensified.
Supporting learning presence: Embedding strategies to cultivate students' self-regulation and self-efficacy inside the course design is crucial for fostering learning presence. These may encompass activities for setting goals, quizzes for self-assessment, and materials on efficient study techniques. Promoting student autonomy in their learning process is crucial for cultivating a strong learning presence.
For policymakers
Resource allocation: Policymakers should prioritise allocating resources towards developing blended MOOCs that facilitate interactive and captivating learning experiences. This strategy encompasses allocating resources towards digital infrastructure, providing opportunities for professional growth among educators, and researching the most effective online teaching and learning methods.
Accessibility and inclusion: Ensuring the accessibility and inclusion of blended MOOCs for a diverse student body is of utmost importance. Policy measures could be established to tackle obstacles to access. Educational institutions should explore cheaper alternative ways for internet connectivity, e.g. providing zero-rated access to MOOCs and negotiating better student internet deals with internet service providers (ISPs). Nations in the global south should consider adopting United States programmes that provide discounted internet access for educational purposes, such as the Affordable Connectivity Program (ACP)and the Federal Communications Commission's E-Rate programme. Furthermore, consideration should be given to creating culturally and linguistically inclusive material and locally relevant content. Lastly, improving the accessibility of MOOCs is also about making the courses themselves more accessible with the accessibility features required by Web Content Accessibility Guidelines (WCAG), like integrating captions, image descriptions, and various forms of learning into the course curriculum to meet many learners' needs like those with disabilities.
Quality assurance: Establishing standards for online course quality, which includes defining criteria for instructional presence, cognitive engagement, and social interaction, can guide the creation of high-quality blended MOOCs. It is necessary to develop continuous evaluation and improvement processes to sustain the effectiveness of online learning environments.
By taking into account these wider educational consequences, the results of our study can guide the creation of blended MOOCs that are both intellectually demanding and highly captivating while being easily accessible to a diverse group of learners. The objective is to utilise the distinctive capabilities of online learning to offer significant and all-encompassing educational experiences that cater to the requirements of the current varied student population.
The study contributes to the discussion on blended MOOCs by exploring students' engagement. By including the notion of learning presence in the revised Community of Inquiry (RCoI) framework, we have highlighted its crucial significance in enhancing the learning experience, accounting for a 56.8% variation in student engagement. Our analysis highlights the importance of learning presence, demonstrating its more significant influence on student engagement than the conventional triad of cognitive, social, and teaching presences. This discovery supports the idea of a fundamental change in perspective towards recognising learning presence as a central aspect of the CoI framework, strengthening its crucial function in promoting a comprehensive and engaging online learning environment.
The empirical results from this study emphasise the necessity for educators and instructional designers to create learning environments that facilitate individual learning, promote meaningful engagement, and offer well-organised pedagogical support (Garrison et al., 2000 ) Strategies such as collaborative projects, peer reviews, and interactive multimedia are crucial instruments for effectively engaging students. When applied successfully, these strategies can enhance student satisfaction and performance in blended MOOCs, thus enabling more personalised and powerful learning experiences. We support the idea of including learning presence within the CoI framework by other scholars (Anderson, 2017 ; Shea & Bidjerano, 2010 ). By adopting this integrated perspective, educators, instructional designers, and policymakers are equipped to navigate the complexities of online education, creating experiences that align with learners' varied needs and goals (Fink, 2013 ). Exploring the RCoI inside blended MOOCs is an academic pursuit and a collective dedication to revolutionising online education into a realm where any student, irrespective of their background, can flourish and attain their maximum capabilities (Tang, 2018 ).
While each presence affects engagement, their interaction implies a complex ecosystem worth further studying. Future research should examine the synergistic impacts of these presences across educational environments to inform online and blended learning experiences. Furthermore, future studies should investigate each presence's mediating or moderating roles concerning student engagement.
Availability of data and materials
Though the paper is part of an on-going PhD dissertation, the datasets are available from the corresponding author on reasonable request.
Afthanorhan, W. M. A. B. W. (2013). A comparison of partial least square structural equation modeling (PLS-SEM) and covariance based structural equation modeling (CB-SEM) for confirmatory factor analysis. International Journal of Engineering Science and Innovative Technology, 2 (5), 198–205.
Google Scholar
Agarwal, R. K. (2021). MOOCS: Challenges & prospects in Indian higher education. In R. Chheda & S. N. Mehta (Eds.), Management practices in digital world. London: Empyreal Publishing House.
Ahmad, S., & Hussain, A. (2019). Authentication of psychosomatic capability and workplace life of teachers scales by structural equation modeling. Journal of Educational Research, 22 (2), 68–81.
Akyol, Z., & Garrison, D. R. (2013). Educational communities of inquiry: Theoretical framework, research and practice (pp. 1–347). https://doi.org/10.4018/978-1-4666-2110-7 .
Alabbasi, D. (2022, April). Factors influencing student engagement in virtual classrooms and their impact on satisfaction. In Society for information technology & teacher education international conference (pp. 142–151). Association for the Advancement of Computing in Education (AACE).
Alkhalaf, S., & Nguyen, T. (2020). Exploring the factors influencing the adoption of blended learning at higher education institutions: A study of instructors’ perspectives. Education and Information Technologies, 25 (2), 1157–1178. https://doi.org/10.1007/s10639-019-10022-5
Article Google Scholar
Almutairi, F., & White, S. (2018). How to measure student engagement in the context of blended-MOOC. Interactive Technology and Smart Education, 15 (3), 262–278.
Anderson, T. (2017). How communities of inquiry drive teaching and learning in the digital age. North Contact , 1–16.
Anderson, T., Rourke, L., Garrison, D. R., & Archer, W. (2001). Assessing teaching presence in a computer conferencing context. Journal of Asynchronous Learning Networks, 5 (2), 1–17.
Andrade, H. L., Brookhart, S. M., & Yu, E. C. (2021, December). Classroom assessment as co-regulated learning: A systematic review. In Frontiers in education (Vol. 6, p. 751168). Frontiers.
Angelaina, S., & Jimoyiannis, A. (2012). Analysing students’ engagement and learning presence in an educational blog community. Educational Media International, 49 (3), 183–200.
Arbaugh, J. B. (2007). An empirical verification of the community of inquiry framework. Journal of Asynchronous Learning Networks, 11 (1), 73–85.
Azila-Gbettor, E. M., Mensah, C., Abiemo, M. K., & Bokor, M. (2021). Predicting student engagement from self-efficacy and autonomous motivation: A cross-sectional study. Cogent Education, 8 (1), 1942638.
Bedi, A. (2023). Keep learning: Student engagement in an online environment. Online Learning, 27 (2), 119–136.
Bergdahl, N., Nouri, J., & Fors, U. (2020). Disengagement, engagement and digital skills in technology-enhanced learning. Education and Information Technologies, 25 , 957–983.
Bradley, R. L., Browne, B. L., & Kelley, H. M. (2017). Examining the influence of self-efficacy and self-regulation in online learning. College Student Journal, 51 (4), 518–530.
Bruff, D. O., Fisher, D. H., McEwen, K. E., & Smith, B. E. (2013). Wrapping a MOOC: Student perceptions of an experiment in blended learning. Journal of Online Learning and Teaching, 9 (2), 187–199.
Caskurlu, S., Maeda, Y., Richardson, J. C., & Lv, J. (2020). A meta-analysis addressing the relationship between teaching presence and students’ satisfaction and learning. Computers & Education, 157 , 103966.
Chi, X. (2023). The influence of presence types on learning engagement in a MOOC: The role of autonomous motivation and grit. Psychology Research and Behavior Management , 5169–5181.
Chin, W. W. (1998). The partial least squares approach to structural equation modeling. Modern Methods for Business Research, 295 (2), 295–336.
Cho, M. H., & Shen, D. (2013). Self-regulation in online learning. Distance Education, 34 (3), 290–301.
Choo, J., Bakir, N., Scagnoli, N. I., Ju, B., & Tong, X. (2020). Using the Community of Inquiry framework to understand students’ learning experience in online undergraduate business courses. TechTrends, 64 , 172–181.
Cohen, J. (1988). Statistical power analysis for the behavioral sciences (2nd ed.). Lawrence Erlbaum Associates.
Contact North (March 2016). Five ways MOOCs are influencing teaching and learning. Ontarios Distance Education and Training Network . March 2016 contactnorth.ca
Cui, X., Qian, J., Garshasbi, S., Zhang, S., Sun, G., Wang, J., et al. (2024). Enhancing learning effectiveness in livestream teaching: Investigating the impact of teaching, social, and cognitive presences through a community of inquiry lens. STEM Education, 4 (2), 82–105.
Damm, C. A. (2016). Applying a community of inquiry instrument to measure student engagement in large online courses. Current Issues in Emerging eLearning, 3 (1), 9.
Deci, E. L., & Ryan, R. M. (2000). The “what” and “why” of goal pursuits: Human needs and the self-determination of behavior. Psychological Inquiry, 11 (4), 227–268.
Edumadze, J. K. E., Otchere Darko, S., Mensah, S., Bentil, D., & Edumadze, G. E. (2022). SWOT Analysis of blended MOOC from ghanaian university instructors’ perspectives. Shanlax International Journal of Arts, Science and Humanities, 10 (1), 67–79. https://doi.org/10.34293/sijash.v10i1.4793.
De Freitas, S. I., Morgan, J., & Gibson, D. (2015). Will MOOCs transform learning and teaching in higher education? Engagement and course retention in online learning provision. British Journal of Educational Technology, 46 (3), 455–471.
Diamantopoulos, A., Sarstedt, M., Fuchs, C., Wilczynski, P., & Kaiser, S. (2012). Guidelines for choosing between multi-item and single-item scales for construct measurement: A predictive validity perspective. Journal of the Academy of Marketing Science, 40 , 434–449.
Dijkstra, T. K., & Henseler, J. (2015). Consistent partial least squares path modeling. MIS Quarterly, 39 (2), 297–316.
Dixson, M. (2015a). Measuring student engagement in the online course: The online student engagement scale (OSE). Online Learning, 19 (4), 143–158.
Dixson, M. D. (2015b). Measuring student engagement in the online course: The Online Student Engagement scale (OSE). Online Learning, 19 (4), n4.
Doo, M. Y., & Bonk, C. J. (2020). The effects of self-efficacy, self-regulation and social presence on learning engagement in a large university class using flipped Learning. Journal of Computer Assisted Learning, 36 (6), 997–1010.
Falk, R. F., & Miller, N. B. (1992). A primer for soft modeling . University of Akron Press.
Feldman, K. (2018, November 7). Variance Inflation Factor (VIF) . Isixsigma. https://www.isixsigma.com/dictionary/variance-inflation-factor-vif/
Fink, L. D. (2013). Creating significant learning experiences: An integrated approach to designing college courses . Wiley.
Fornell, C., & Larcker, D. F. (1981). Evaluating structural equation models with unobservable variables and measurement error. Journal of Marketing Research, 18 (1), 39–50.
Fredricks, J. A., Blumenfeld, P. C., & Paris, A. H. (2004). School engagement: Potential of the concept, state of the evidence. Review of Educational Research, 74 (1), 59–109.
Garrison, D. R. (2015). Thinking collaboratively: Learning in a community of inquiry . Routledge.
Book Google Scholar
Garrison, D. R. (2017). E-Learning in the 21st century: A community of inquiry framework for research and practice (3rd ed.). Routledge/Taylor and Francis.
Garrison, D. R., & Cleveland-Innes, M. (2005). Facilitating cognitive presence in online learning: Interaction is not enough. The American Journal of Distance Education, 19 (3), 133–148.
Garrison, D. R., & Vaughan, N. D. (2008). Blended learning in higher education: Framework, principles, and guidelines . John Wiley & Sons.
Garrison, D. R., Anderson, T., & Archer, W. (2000). Critical Inquiry in a text-based environment: Computer conferencing in higher education. The Internet and Higher Education, 2 (2), 87–105.
Garrison, D. R., Anderson, T., & Archer, W. (2001). Critical thinking, cognitive presence, and computer conferencing in distance education. American Journal of Distance Education, 15 (1), 7–23.
Garrison, D. R., Anderson, T., & Archer, W. (2003). A theory of critical inquiry in online distance education. Handbook of Distance Education, 1 (4), 113–127.
Garrison, D. R., Anderson, T., & Archer, W. (2010). The first decade of the community of inquiry framework: A retrospective. The Internet and Higher Education, 13 (1–2), 5–9.
Garrison, D. R. (2022, August 8). Shared metacognition and regulation response . The Community of Inquiry: Editorials
Garrison, D.R., & Akyol, Z. (2013). The Community of Inquiry Theoretical Framework. In Handbook of distance education (pp. 122–138). Routledge.
Ginting, D. (2021). Student engagement and factors affecting active learning in English language teaching. VELES (Voices of English Language Education Society), 5 (2), 215–228.
Gray, J., & Diloreto, M. (2016). The effects of student engagement, student satisfaction, and perceived learning in online learning environments. NCPEA International Journal of Educational Leadership Preparation, 11 (1), 98–119.
Groves, R. (2012, September 21). Georgetown University . Our moment in time: https://blog.provost.georgetown.edu/our-moment-in-time/
Gupta, D., Khan, A. A., Kumar, A., Baghel, M. S., & Tiwar, A. (2024). Socially connected learning harnessing digital platforms for educational engagement. In Navigating innovative technologies and intelligent systems in modern education (pp. 210–228). IGI Global.
Hair, J. F., Jr., Hult, G. T. M., Ringle, C. M., & Sarstedt, M. (2021a). A primer on partial least squares structural equation modeling (PLS-SEM) . Sage publications.
Hair, J. F., Jr., Hult, G. T. M., Ringle, C. M., Sarstedt, M., Danks, N. P., & Ray, S. (2021b). Partial Least Squares Structural Equation Modeling (PLS-SEM) using R, classroom companion: Business . Springer Nature. https://doi.org/10.1007/978-3-030-80519-7_1
Hair, J. F., Jr., Matthews, L. M., Matthews, R. L., & Sarstedt, M. (2017). PLS-SEM or CB-SEM: Updated guidelines on which method to use. International Journal of Multivariate Data Analysis, 1 (2), 107–123.
Hair, J., Jr., Hair, J. F., Jr., Hult, G. T. M., Ringle, C. M., & Sarstedt, M. (2021c). A primer on partial least squares structural equation modelling (PLS-SEM) . Sage publications.
Hair, J. F., Risher, J. J., Sarstedt, M., & Ringle, C. M. (2019). When to use and how to report the results of PLS-SEM. European Business Review, 31 (1), 2–24.
Haw, L. H., Sharif, S. B., & Han, C. G. K. (2022). Predictors of student engagement in science learning: The role of science laboratory learning environment and science learning motivation. ASIA Pacific Journal of Educators and Education . https://doi.org/10.21315/apjee2022.37.2.1
He, J., Liu, Z., & Kong, X. (2023, September). A novel link prediction approach for MOOC forum thread recommendation using personalized pagerank and machine learning. In 2023 3rd international conference on educational technology (ICET) (pp. 37–41). IEEE.
Helland, I. S., Sæbø, S., Almøy, T., & Rimal, R. (2018). Model and estimators for partial least squares regression. Journal of Chemometrics , 32 (9), e3044.
Henseler, J., Ringle, C. M., & Sarstedt, M. (2015). A new criterion for assessing discriminant validity in variance-based structural equation modeling. Journal of the Academy of Marketing Science, 43 , 115–135.
Hodges, T. (2018, October 25). School engagement is more than just talk . Gallup https://www.gallup.com/education/244022/school-engagement-talk.aspx?version=print
Holotescu, C., Grosseck, G., Crețu, V., & Naaji, A. (2014). Integrating MOOCs in blended courses. In 10th international scientific conference eLearning and software for education. Bucharest.
Hulland, J. (1999). Use of partial least squares (PLS) in strategic management research: A review of four recent studies. Strategic Management Journal, 20 (2), 195–204.
Hussain, M., Zhu, W., Zhang, W., & Abidi, S. M. R. (2018). Student engagement predictions in an e-learning system and their impact on student course assessment scores. In Computational intelligence and neuroscience , 2018 .
Irene, B. N. O. (2019). Technopreneurship: a discursive analysis of the impact of technology on the success of women entrepreneurs in South Africa. Digital Entrepreneurship in Sub-Saharan Africa: Challenges, Opportunities and Prospects (pp. 147–173).
Ituma, A. (2011). An evaluation of students’ perceptions and engagement with e-learning components in a campus-based university. Active Learning in Higher Education, 12 (1), 57–68.
Jovanović, J., Gašević, D., Dawson, S., Pardo, A., & Mirriahi, N. (2017). Learning analytics to unveil learning strategies in a flipped classroom. The Internet and Higher Education, 33 , 74–85.
Jimoyiannis, A., & Tsiotakis, P. (2017). Beyond students’ perceptions: Investigating learning presence in an educational blogging community. Journal of Applied Research in Higher Education., 9 (1), 129–146.
Joksimović, S., Poquet, O., Kovanović, V., Dowell, N., Mills, C., Gašević, D., Dawson, S., Graesser, A. C., & Brooks, C. (2018). How do we model learning at scale? A systematic review of research on MOOCs. Review of Educational Research, 88 (1), 43–86.
Jöreskog, K. G., & Sörbom, D. (1995). LISREL 8: Structural equation modeling with the SIMPLIS command language . Scientific Software International.
Jung, Y., & Lee, J. (2018). Learning engagement and persistence in massive open online courses (MOOCS). Computers & Education, 122 , 9–22.
Kang, M. H., Park, J., U., & Shin, S. Y. (2007). Developing a cognitive presence scale for measuring students' involvement during e-learning process. In C. Montgomerie & J. Seale (Eds.), Proceedings of world conference on educational multimedia, hypermedia and telecommunications 2007 (pp. 2823–2828). Association for the Advancement of Computing in Education (AACE).
Kang, M., Park, J. U., & Shin, S. (2007, June). Developing a cognitive presence scale for measuring students' involvement during e-learning process. In EdMedia+ innovate learning (pp. 2823–2828). Association for the Advancement of Computing in Education (AACE).
King, R. B. (2015). Sense of relatedness boosts engagement, performance, and well-being: A latent growth model study. Contemporary Educational Psychology, 42 , 26–38.
Kloos, C. D., Muñoz-Merino, P. J., Alario-Hoyos, C., Ayres, I. E., & Fernández-Panadero, C. (2015). Mixing and blending MOOC technologies with face-to-face pedagogies. In Proceedings of the IEEE global engineering education conference (EDUCON) , Tallin, Estonia (pp. 967–971).
Kock, N. (2015). Common method bias in PLS-SEM: A full collinearity assessment approach. International Journal of e-Collaboration (ijec), 11 (4), 1–10.
Koller, D., Ng, A., Do, C., & Chen, Z. (2013). Retention and intention in massive open online courses: In depth. Educause Review, 48 (3), 62–63.
Koutsakas, P., Karagiannidis, C., Politis, P., & Karasavvidis, I. (2020). A computer programming hybrid MOOC for Greek secondary education. Smart Learning Environments, 7 , 7.
Kozan, K., & Caskurlu, S. (2018). On the Nth presence for the Community of Inquiry framework. Computers & Education, 122 , 104–118.
Kruse, A., & Pongratz, H. (2017). Digital change: How MOOCs transform the educational landscape. In H. Ellermann, P. Kreutter, & W. Messner (Eds.), The Palgrave handbook of managing continuous business transformation (pp. 353–373). Springer.
Chapter Google Scholar
Lambert, J. L., & Fisher, J. L. (2013). Community of inquiry framework: Establishing community in an online course. Journal of Interactive Online Learning, 12 (1), 1–16.
Lee, S. M. (2014). The relationships between higher order thinking skills, cognitive density, and social presence in online learning. The Internet and Higher Education, 21 , 41–52.
Liao, H., Zhang, Q., Yang, L., & Fei, Y. (2023). Investigating relationships among regulated learning, teaching presence and student engagement in blended learning: An experience sampling analysis. Education and Information Technologies, 28 (10), 12997–13025.
Lin, K. Y., Wu, Y. T., Hsu, Y. T., & Williams, P. J. (2021). Effects of infusing the engineering design process into STEM project-based learning to develop preservice technology teachers’ engineering design thinking. International Journal of STEM Education, 8 (1), 1–15. https://doi.org/10.1186/s40594-020-00258-9
Lindner, T., Puck, J., & Verbeke, A. (2020). Misconceptions about multicollinearity in international business research: Identification, consequences, and remedies. Journal of International Business Studies, 51 (3), 283–298.
Linnenbrink, E. A., & Pintrich, P. R. (2003a). The role of self-efficacy beliefs instudent engagement and learning intheclassroom. Reading & Writing Quarterly, 19 (2), 119–137.
Linnenbrink, E. A., & Pintrich, P. R. (2003b). The role of self-efficacy beliefs in student engagement and learning in the classroom. Reading & Writing Quarterly, 19 (2), 119–137.
Littler, M. (2024). Social, Cognitive, and Teaching Presence as Predictors of Online Student Engagement Among MSN Students [Ph.D. thesis, Walden University]. Walden Dissertations and Doctoral Studies Collection.
Ma, Y., Zuo, M., Yan, Y., Wang, K., & Luo, H. (2022). How do K-12 students’ perceptions of online learning environments affect their online learning engagement? Evidence from China’s COVID-19 school closure period. Sustainability, 14 (23), 15691.
Maphosa, V., & Maphosa, M. (2023). Opportunities and challenges of adopting MOOCs in Africa: A systematic literature review. In S. Goundar (Ed.). Massive open online courses-current practice and future trends . IntechOpen. https://doi.org/10.5772/intechopen.1001518 .
Maranna, S., Willison, J., Joksimovic, S., Parange, N., & Costabile, M. (2022). Factors that influence cognitive presence: A scoping review. Australasian Journal of Educational Technology, 38 (4), 95–111.
McNutt, M. (2013). Bricks and MOOCs. Science, 342 (6157), 402.
Miao, J., & Ma, L. (2022). Students’ online interaction, self-regulation, and learning engagement in higher education: The importance of social presence to online learning. Frontiers in Psychology, 13 , 815220.
Montgomery, A. P., Hayward, D. V., Dunn, W., Carbonaro, M., & Amrhein, C. G. (2015). Blending for student engagement: Lessons learned for MOOCs and beyond. Australasian Journal of Educational Technology, 31 (6), 657.
Moore, R. L., & Miller, C. N. (2022). Fostering cognitive presence in online courses: A systematic review (2008–2020). Online Learning, 26 (1), 130–149.
Netemeyer, R. G., Bearden, W. O., & Sharma, S. (2003). Scaling procedures . SAGE, London: Issues and applications.
Nikolopoulou, K. (2022, September 2). What is discriminant validity? Definition & example. Scribbr. https://www.scribbr.co.uk/research-methods/discriminant-validity-explained/
Nunnally, J. C., & Bernstein, I. H. (1994). Psychometric theory (3rd ed.). McGraw-Hill.
Onah, D. F., Pang, E. L., & Sinclair, J. E. (2022). An investigation of self-regulated learning in a novel MOOC platform. Journal of Computing in Higher Education , 1–34.
OpenupEd. (2015). Definition massive open online courses. Heerlen: EADTU. http://www.openuped.eu/images/docs/Definition_Massive_Open_Online_Courses.pdf
Oyarzun, B., & Morrison, G. (2013). Cooperative learning effects on achievement and community of inquiry in online education. The Quarterly Review of Distance Education, 14 (4), 181–194.
Pakula, A. (2024). The role of tutor in massive social language learning: A case study of an academic Italian MOOC. In 18th international technology, education and development conference (pp. 2195–2203). Valencia, Spain. https://doi.org/10.21125/inted.2024.0603 .
Popescu, E., & Badea, G. (2020). Exploring a community of inquiry supported by a social media-based learning environment. Educational Technology & Society, 23 (2), 61–76.
Prasetyo, A., Tamrin, A. G., & Estriyanto, Y. (2022). A successful model of microsoft teams online learning platform in vocational high school. FWU Journal of Social Sciences, 16 (2).
Qaffas, A. A., Kaabi, K., Shadiev, R., & Essalmi, F. (2020). (2020) Towards an optimal personalization strategy in MOOCs. Smart Learning Environments, 7 , 14. https://doi.org/10.1186/s40561-020-0117-y
Rahimi, A. R. (2024). A tri-phenomenon perspective to mitigate MOOCs’ high dropout rates: the role of technical, pedagogical, and contextual factors on language learners’ L2 motivational selves, and learning approaches to MOOC. Smart Learning Environments . https://doi.org/10.1186/s40561-024-00297-7
Ranjan, P. (2020). Exploring the Models of Designing Blended & Online Learning Courses for Adoption in Regular Teacher Education Course. In Voices of teachers and teacher educators IX . National Council of Educational Research and Training (NCERT).
Ravinder, E. B., & Saraswathi, A. B. (2020). Literature Review Of Cronbach alpha coefficient (Α) And Mcdonald’s Omega Coefficient (Ω). European Journal of Molecular & Clinical Medicine, 7 (6), 2943–2949.
Richardson, J. C., & Swan, K. (2003). Examining social presence in online courses in relation to students’ perceive learning and satisfaction. Journal of Asynchronous Learning Networks, 7 (1), 68–88.
Ringle, C. M., & Sarstedt, M. (2016). Gain more insight from your PLS-SEM results: The importance-performance map analysis. Industrial Management & Data Systems, 116 (9), 1865–1886.
Ringle, C. M., Wende, S., & Becker, J. M. (2022). SmartPLS 4. Oststeinbek: SmartPLS GmbH. Journal of Applied Structural Equation Modeling.
Saefudin, W., & Yusoff, S. H. M. (2021). Self-efficacy and student engagement in online learning during pandemic. Global Journal of Educational Research and Management, 1 (4), 219–231.
Sarstedt, M., Ringle, C. M., & Hair, J. F. (2021). Partial least squares structural equation modeling. In Handbook of market research (pp. 587–632). Springer International Publishing.
Saunders, M. (2014). Research methods for business students (6th edn, Greek language edition) . Pearson Education.
Schunk, D. H., & Mullen, C. A. (2012). Self-efficacy as an engaged learner. In Handbook of research on student engagement (pp. 219–235). Springer US.
She, L., Ma, L., Jan, A., Sharif Nia, H., & Rahmatpour, P. (2021). Online learning satisfaction during COVID-19 pandemic among Chinese university students: The serial mediation model. Frontiers in Psychology, 12 , 743936.
Shea, P. (2010). Online learning presence. In Proceeding of the European Distance and e-learning network (EDEN) annual conference . Valencia, Spain.
Shea, P., & Bidjerano, T. (2009). Community of inquiry as a theoretical framework to foster “epistemic engagement” and “cognitive presence” in online education. Computers & Education , 52.
Shea, P., & Bidjerano, T. (2010). Learning presence: Towards a theory of self-efficacy, self-regulation, and the development of a community of inquiry in online and blended learning environments. Computers & Education, 55 (4), 1721–1731.
Shea, P., & Bidjerano, T. (2012). Learning presence as a moderator in the community of inquiry model. Computers & Education, 59 (2), 316–326.
Shea, P., Fredericksen, E. E., Pickett, A. M., & Pelz, W. (2003). Student satisfaction and Reported learning in the SUNY Learning Network. In T. Duffy & J. Kirkley (Eds.), Learner-centred theory and practice in distance education. Lawrence Erlbaum.
Shea, P., Hayes, S., & Vickers, J. (2010). Online instructional effort measured through the lens of teaching presence in the community of inquiry framework: A re-examination of measures and approach. International Review of Research in Open and Distributed Learning, 11 (3), 127–154.
Shivdas, A., Menon, D. G., & Nair, C. S. (2020). Antecedents of acceptance and use of a digital library system: Experience from a Tier 3 Indian city. The Electronic Library, 38 (1), 170–185.
Sukor, R., Ayub, A. F. M., Ab, N. K. M. A. R., & Halim, F. A. (2021). Relationship between students’ engagement with academic performance among non-food science students enrolled in food science course. Journal of Turkish Science Education, 18 (4), 638–648.
Tang, H. (2018). Exploring self-regulated learner profiles in MOOCs: A comparative study . The Pennsylvania State University.
Tavakol, M., & Dennick, R. (2011). Making sense of Cronbach’s alpha. International Journal of Medical Education, 2 , 53.
TED. (2014, January 27). Anant Agarwal: Why massively open online courses (still) matter [Video]. YouTube. https://www.youtube.com/watch?v=rYwTA5RA9eU
Ulrich, C., & Nedelcu, A. (2015). Moocs in our university: Hopes and worries. Procedia-Social and Behavioral Sciences, 180 , 1541–1547.
UNICEF Office of Innovation. (2022, May 10). Can tech solve the global education crisis? UNICEF. https://www.unicef.org/innovation/xtc-unicef-edtech-award-finalists
Veletsianos, G., & Kimmons, R. (2012). Networked participatory scholarship: Emergent techno-cultural pressures toward open and digital scholarship in online networks. Computers & Education, 58 (2), 766–774.
Vezne, R., Yildiz Durak, H., & Atman Uslu, N. (2023). Online learning in higher education: Examining the predictors of students’ online engagement. Education and Information Technologies, 28 (2), 1865–1889.
Vieira,D. Mutize, T. & Jaime Roser Chinchilla, J.B.(2020, December 21). Understanding access to higher education in the last two decades . UNESCO. https://www.iesalc.unesco.org/en/2020/12/23/understanding-access-to-higher-education-in-the-last-two-decades/
Walters, H. (2014, January 27). We need to change everything on campus . Ideas. Ted. Com. https://ideas.ted.com/we-need-to-change-everything-on-campus-anant-agarwal-of-edx-on-moocs-mit-and-new-models-of-higher-education/
Wang, M. T., & Degol, J. (2014). Staying engaged: Knowledge and research needs in student engagement. Child Development Perspectives, 8 (3), 137–143.
Wang, X. Yang, D., Wen, M., Koedinger, K., & Rosé, C. P. (2015). Investigating how student’s cognitive behavior in MOOC discussion forums affect learning gains . In Proceedings of the 8th international conference on educational data mining (EDM 2015) , June 26–29, 2015 (pp. 226–233). International Educational Data Mining Society (IEDMS). http://www.educationaldatamining.org/EDM2015/uploads/papers/paper_89.pdf
Wertz, R. E. (2022). Learning presence within the Community of Inquiry framework: An alternative measurement survey for a four-factor model. The Internet and Higher Education, 52 , 100832.
Whitehill J., Williams J. J., Lopez G., Coleman C. A., Reich J. (2015). Beyond prediction: First steps toward automatic intervention in MOOC student stopout. In Proceedings of the 8th international conference on educational data mining (EDM’15) , June 26–29, 2015 (pp. 171–178). International Educational Data Mining Society (IEDMS). http://www.educationaldatamining.org/EDM2015/uploads/papers/paper_112.pdf
Wicks, D., Craft, B. B., Lee, D., Lumpe, A., Henrikson, R., Baliram, N., Bian, X., Mehlberg, S., & Wicks, K. (2015). An evaluation of low versus high collaboration in online learning. Online Learning, 19 (4), n4.
Wolverton, C. C., Hollier, B. N. G., & Lanier, P. A. (2020). The impact of computer self efficacy on student engagement and group satisfaction in online business courses. Electronic Journal of e-Learning, 18 (2), 175–188.
Wu, M. J., Zhao, K., & Fils-Aime, F. (2022). Response rates of online surveys in published research: A meta-analysis. Computers in Human Behavior Reports, 7 , 100206
Yeboah, D. (2020). Predicting acceptance of WhatsApp as learning-support tool by higher distance education students in Ghana . Unpublished Ph.D. Thesis. Texila American University, Guyana.
Yeboah, D., & Nyagorme, P. (2020). Validation of non-linear relationships-based UTAUT model on higher distance education students’ acceptance of Whatsapp for supporting learning. Texila International Journal of Academic Research, 7 (2), 27–39. https://doi.org/10.21522/TIJAR.2014.07.02.Art004
Yousef, A. M., Chatti, M., Schroeder, U., & Wosnitza, M. (2015). A usability evaluation of a blended MOOC environment: An experimental case study. International Review of Research in Open and Distributed Learning, 16 (2), 69–93.
Yunusa, A. A., Umar, I. N., & Bervell, B. (2021). Massive open online courses (MOOCs) in Sub-Saharan African Higher Education Landscape: A Bibliometric Review. MOOC (Massive Open Online Courses) , 1–25.
Yusof, A., Atan, N. A., Harun, J., & Doulatabadi, M. (2017). Understanding learners’ persistence and engagement in Massive Open Online Courses: A critical review for Universiti Teknologi Malaysia. Man in India, 97 (12), 147–157.
Zakaria, M., Awang, S., & Rahman, R. A. (2019). Are MOOCs in blended learning more effective than traditional classrooms for undergraduate learners. Universal Journal of Educational Research, 7 (11), 2417–2424.
Zhang, H., Lin, L., Zhan, Y., & Ren, Y. (2016). The impact of teaching presence on online engagement behaviors. Journal of Educational Computing Research, 54 (7), 887–900.
Zhang, Y. (2013). Benefiting from MOOC. World conference on educational multimedia, Hypermedia and Telecommunications (pp. 1372–1377).
Zhang, Y. (2022). The effect of educational technology on EFL learners’ self-efficacy. Frontiers in Psychology, 13 , 881301.
Zimmerman, B. J. (1986). Becoming a self-regulated learner: Which are the key subprocesses? Contemporary Educational Psychology, 11 (4), 307–313.
Zimmerman, B. J. (1989). A social cognitive view of self-regulated academic learning. Journal of Educational Psychology, 81 (3), 329.
Zimmerman, B. J. (2000). Attaining self-regulation: A social cognitive perspective. In Handbook of self-regulation (pp. 13–39). Academic Press.
Download references
Acknowledgements
I express my appreciation to (1) Dr. Fadiyah Almutairi for the question items on the Blended MOOCs Engagement Model and (2) Prof Ruth E. H. Wertz for the question items on the community of inquiry. These items were used as part of the survey instrument for the study.
Not available.
Author information
Authors and affiliations.
Network and Infrastructure Services, University of Cape Coast, Cape Coast, Ghana
John Kwame Eduafo Edumadze
Department of Mathematics and Computer Science Education, University of KwaZulu-Natal, Pinetown, South Africa
John Kwame Eduafo Edumadze & Desmond Welsey Govender
You can also search for this author in PubMed Google Scholar
Contributions
JKEE wrote the PhD thesis from which is paper was extracted. DWG supervised the thesis and approved the final manuscript.
Corresponding author
Correspondence to John Kwame Eduafo Edumadze .
Ethics declarations
Competing interests.
The authors declare that they have no competing interests.
Additional information
Publisher's note.
Springer Nature remains neutral with regard to jurisdictional claims in published maps and institutional affiliations.
Rights and permissions
Open Access This article is licensed under a Creative Commons Attribution 4.0 International License, which permits use, sharing, adaptation, distribution and reproduction in any medium or format, as long as you give appropriate credit to the original author(s) and the source, provide a link to the Creative Commons licence, and indicate if changes were made. The images or other third party material in this article are included in the article's Creative Commons licence, unless indicated otherwise in a credit line to the material. If material is not included in the article's Creative Commons licence and your intended use is not permitted by statutory regulation or exceeds the permitted use, you will need to obtain permission directly from the copyright holder. To view a copy of this licence, visit http://creativecommons.org/licenses/by/4.0/ .
Reprints and permissions
About this article
Cite this article.
Edumadze, J.K.E., Govender, D.W. The community of inquiry as a tool for measuring student engagement in blended massive open online courses (MOOCs): a case study of university students in a developing country. Smart Learn. Environ. 11 , 19 (2024). https://doi.org/10.1186/s40561-024-00306-9
Download citation
Received : 24 August 2023
Accepted : 28 April 2024
Published : 14 May 2024
DOI : https://doi.org/10.1186/s40561-024-00306-9
Share this article
Anyone you share the following link with will be able to read this content:
Sorry, a shareable link is not currently available for this article.
Provided by the Springer Nature SharedIt content-sharing initiative
- Community of inquiry
- Blended MOOCs
- MOOCs as open educational resources
- Structural equation modelling
- Sub-Saharan Africa

An official website of the United States government
The .gov means it’s official. Federal government websites often end in .gov or .mil. Before sharing sensitive information, make sure you’re on a federal government site.
The site is secure. The https:// ensures that you are connecting to the official website and that any information you provide is encrypted and transmitted securely.
- Publications
- Account settings
Preview improvements coming to the PMC website in October 2024. Learn More or Try it out now .
- Advanced Search
- Journal List
- An Bras Dermatol
- v.91(3); May-Jun 2016
Sampling: how to select participants in my research study? *
Jeovany martínez-mesa.
1 Faculdade Meridional (IMED) - Passo Fundo (RS), Brazil.
David Alejandro González-Chica
2 University of Adelaide - Adelaide, Australia.
Rodrigo Pereira Duquia
3 Universidade Federal de Ciências da Saúde de Porto Alegre (UFCSPA) - Porto Alegre (RS), Brazil.
Renan Rangel Bonamigo
João luiz bastos.
4 Universidade Federal de Santa Catarina (UFSC) - Florianópolis (RS), Brazil.
In this paper, the basic elements related to the selection of participants for a health research are discussed. Sample representativeness, sample frame, types of sampling, as well as the impact that non-respondents may have on results of a study are described. The whole discussion is supported by practical examples to facilitate the reader's understanding.
To introduce readers to issues related to sampling.
INTRODUCTION
The essential topics related to the selection of participants for a health research are: 1) whether to work with samples or include the whole reference population in the study (census); 2) the sample basis; 3) the sampling process and 4) the potential effects nonrespondents might have on study results. We will refer to each of these aspects with theoretical and practical examples for better understanding in the sections that follow.
TO SAMPLE OR NOT TO SAMPLE
In a previous paper, we discussed the necessary parameters on which to estimate the sample size. 1 We define sample as a finite part or subset of participants drawn from the target population. In turn, the target population corresponds to the entire set of subjects whose characteristics are of interest to the research team. Based on results obtained from a sample, researchers may draw their conclusions about the target population with a certain level of confidence, following a process called statistical inference. When the sample contains fewer individuals than the minimum necessary, but the representativeness is preserved, statistical inference may be compromised in terms of precision (prevalence studies) and/or statistical power to detect the associations of interest. 1 On the other hand, samples without representativeness may not be a reliable source to draw conclusions about the reference population (i.e., statistical inference is not deemed possible), even if the sample size reaches the required number of participants. Lack of representativeness can occur as a result of flawed selection procedures (sampling bias) or when the probability of refusal/non-participation in the study is related to the object of research (nonresponse bias). 1 , 2
Although most studies are performed using samples, whether or not they represent any target population, census-based estimates should be preferred whenever possible. 3 , 4 For instance, if all cases of melanoma are available on a national or regional database, and information on the potential risk factors are also available, it would be preferable to conduct a census instead of investigating a sample.
However, there are several theoretical and practical reasons that prevent us from carrying out census-based surveys, including:
- Ethical issues: it is unethical to include a greater number of individuals than that effectively required;
- Budgetary limitations: the high costs of a census survey often limits its use as a strategy to select participants for a study;
- Logistics: censuses often impose great challenges in terms of required staff, equipment, etc. to conduct the study;
- Time restrictions: the amount of time needed to plan and conduct a census-based survey may be excessive; and,
- Unknown target population size: if the study objective is to investigate the presence of premalignant skin lesions in illicit drugs users, lack of information on all existing users makes it impossible to conduct a census-based study.
All these reasons explain why samples are more frequently used. However, researchers must be aware that sample results can be affected by the random error (or sampling error). 3 To exemplify this concept, we will consider a research study aiming to estimate the prevalence of premalignant skin lesions (outcome) among individuals >18 years residing in a specific city (target population). The city has a total population of 4,000 adults, but the investigator decided to collect data on a representative sample of 400 participants, detecting an 8% prevalence of premalignant skin lesions. A week later, the researcher selects another sample of 400 participants from the same target population to confirm the results, but this time observes a 12% prevalence of premalignant skin lesions. Based on these findings, is it possible to assume that the prevalence of lesions increased from the first to the second week? The answer is probably not. Each time we select a new sample, it is very likely to obtain a different result. These fluctuations are attributed to the "random error." They occur because individuals composing different samples are not the same, even though they were selected from the same target population. Therefore, the parameters of interest may vary randomly from one sample to another. Despite this fluctuation, if it were possible to obtain 100 different samples of the same population, approximately 95 of them would provide prevalence estimates very close to the real estimate in the target population - the value that we would observe if we investigated all the 4,000 adults residing in the city. Thus, during the sample size estimation the investigator must specify in advance the highest or maximum acceptable random error value in the study. Most population-based studies use a random error ranging from 2 to 5 percentage points. Nevertheless, the researcher should be aware that the smaller the random error considered in the study, the larger the required sample size. 1
SAMPLE FRAME
The sample frame is the group of individuals that can be selected from the target population given the sampling process used in the study. For example, to identify cases of cutaneous melanoma the researcher may consider to utilize as sample frame the national cancer registry system or the anatomopathological records of skin biopsies. Given that the sample may represent only a portion of the target population, the researcher needs to examine carefully whether the selected sample frame fits the study objectives or hypotheses, and especially if there are strategies to overcome the sample frame limitations (see Chart 1 for examples and possible limitations).
Examples of sample frames and potential limitations as regards representativeness
Sampling can be defined as the process through which individuals or sampling units are selected from the sample frame. The sampling strategy needs to be specified in advance, given that the sampling method may affect the sample size estimation. 1 , 5 Without a rigorous sampling plan the estimates derived from the study may be biased (selection bias). 3
TYPES OF SAMPLING
In figure 1 , we depict a summary of the main sampling types. There are two major sampling types: probabilistic and nonprobabilistic.

Sampling types used in scientific studies
NONPROBABILISTIC SAMPLING
In the context of nonprobabilistic sampling, the likelihood of selecting some individuals from the target population is null. This type of sampling does not render a representative sample; therefore, the observed results are usually not generalizable to the target population. Still, unrepresentative samples may be useful for some specific research objectives, and may help answer particular research questions, as well as contribute to the generation of new hypotheses. 4 The different types of nonprobabilistic sampling are detailed below.
Convenience sampling : the participants are consecutively selected in order of apperance according to their convenient accessibility (also known as consecutive sampling). The sampling process comes to an end when the total amount of participants (sample saturation) and/or the time limit (time saturation) are reached. Randomized clinical trials are usually based on convenience sampling. After sampling, participants are usually randomly allocated to the intervention or control group (randomization). 3 Although randomization is a probabilistic process to obtain two comparable groups (treatment and control), the samples used in these studies are generally not representative of the target population.
Purposive sampling: this is used when a diverse sample is necessary or the opinion of experts in a particular field is the topic of interest. This technique was used in the study by Roubille et al, in which recommendations for the treatment of comorbidities in patients with rheumatoid arthritis, psoriasis, and psoriatic arthritis were made based on the opinion of a group of experts. 6
Quota sampling: according to this sampling technique, the population is first classified by characteristics such as gender, age, etc. Subsequently, sampling units are selected to complete each quota. For example, in the study by Larkin et al., the combination of vemurafenib and cobimetinib versus placebo was tested in patients with locally-advanced melanoma, stage IIIC or IV, with BRAF mutation. 7 The study recruited 495 patients from 135 health centers located in several countries. In this type of study, each center has a "quota" of patients.
"Snowball" sampling : in this case, the researcher selects an initial group of individuals. Then, these participants indicate other potential members with similar characteristics to take part in the study. This is frequently used in studies investigating special populations, for example, those including illicit drugs users, as was the case of the study by Gonçalves et al, which assessed 27 users of cocaine and crack in combination with marijuana. 8
PROBABILISTIC SAMPLING
In the context of probabilistic sampling, all units of the target population have a nonzero probability to take part in the study. If all participants are equally likely to be selected in the study, equiprobabilistic sampling is being used, and the odds of being selected by the research team may be expressed by the formula: P=1/N, where P equals the probability of taking part in the study and N corresponds to the size of the target population. The main types of probabilistic sampling are described below.
Simple random sampling: in this case, we have a full list of sample units or participants (sample basis), and we randomly select individuals using a table of random numbers. An example is the study by Pimenta et al, in which the authors obtained a listing from the Health Department of all elderly enrolled in the Family Health Strategy and, by simple random sampling, selected a sample of 449 participants. 9
Systematic random sampling: in this case, participants are selected from fixed intervals previously defined from a ranked list of participants. For example, in the study of Kelbore et al, children who were assisted at the Pediatric Dermatology Service were selected to evaluate factors associated with atopic dermatitis, selecting always the second child by consulting order. 10
Stratified sampling: in this type of sampling, the target population is first divided into separate strata. Then, samples are selected within each stratum, either through simple or systematic sampling. The total number of individuals to be selected in each stratum can be fixed or proportional to the size of each stratum. Each individual may be equally likely to be selected to participate in the study. However, the fixed method usually involves the use of sampling weights in the statistical analysis (inverse of the probability of selection or 1/P). An example is the study conducted in South Australia to investigate factors associated with vitamin D deficiency in preschool children. Using the national census as the sample frame, households were randomly selected in each stratum and all children in the age group of interest identified in the selected houses were investigated. 11
Cluster sampling: in this type of probabilistic sampling, groups such as health facilities, schools, etc., are sampled. In the above-mentioned study, the selection of households is an example of cluster sampling. 11
Complex or multi-stage sampling: This probabilistic sampling method combines different strategies in the selection of the sample units. An example is the study of Duquia et al. to assess the prevalence and factors associated with the use of sunscreen in adults. The sampling process included two stages. 12 Using the 2000 Brazilian demographic census as sampling frame, all 404 census tracts from Pelotas (Southern Brazil) were listed in ascending order of family income. A sample of 120 tracts were systematically selected (first sampling stage units). In the second stage, 12 households in each of these census tract (second sampling stage units) were systematically drawn. All adult residents in these households were included in the study (third sampling stage units). All these stages have to be considered in the statistical analysis to provide correct estimates.
NONRESPONDENTS
Frequently, sample sizes are increased by 10% to compensate for potential nonresponses (refusals/losses). 1 Let us imagine that in a study to assess the prevalence of premalignant skin lesions there is a higher percentage of nonrespondents among men (10%) than among women (1%). If the highest percentage of nonresponse occurs because these men are not at home during the scheduled visits, and these participants are more likely to be exposed to the sun, the number of skin lesions will be underestimated. For this reason, it is strongly recommended to collect and describe some basic characteristics of nonrespondents (sex, age, etc.) so they can be compared to the respondents to evaluate whether the results may have been affected by this systematic error.
Often, in study protocols, refusal to participate or sign the informed consent is considered an "exclusion criteria". However, this is not correct, as these individuals are eligible for the study and need to be reported as "nonrespondents".
SAMPLING METHOD ACCORDING TO THE TYPE OF STUDY
In general, clinical trials aim to obtain a homogeneous sample which is not necessarily representative of any target population. Clinical trials often recruit those participants who are most likely to benefit from the intervention. 3 Thus, the more strict criteria for inclusion and exclusion of subjects in clinical trials often make it difficult to locate participants: after verification of the eligibility criteria, just one out of ten possible candidates will enter the study. Therefore, clinical trials usually show limitations to generalize the results to the entire population of patients with the disease, but only to those with similar characteristics to the sample included in the study. These peculiarities in clinical trials justify the necessity of conducting a multicenter and/or global studiesto accelerate the recruitment rate and to reach, in a shorter time, the number of patients required for the study. 13
In turn, in observational studies to build a solid sampling plan is important because of the great heterogeneity usually observed in the target population. Therefore, this heterogeneity has to be also reflected in the sample. A cross-sectional population-based study aiming to assess disease estimates or identify risk factors often uses complex probabilistic sampling, because the sample representativeness is crucial. However, in a case-control study, we face the challenge of selecting two different samples for the same study. One sample is formed by the cases, which are identified based on the diagnosis of the disease of interest. The other consists of controls, which need to be representative of the population that originated the cases. Improper selection of control individuals may introduce selection bias in the results. Thus, the concern with representativeness in this type of study is established based on the relationship between cases and controls (comparability).
In cohort studies, individuals are recruited based on the exposure (exposed and unexposed subjects), and they are followed over time to evaluate the occurrence of the outcome of interest. At baseline, the sample can be selected from a representative sample (population-based cohort studies) or a non-representative sample. However, in the successive follow-ups of the cohort member, study participants must be a representative sample of those included in the baseline. 14 , 15 In this type of study, losses over time may cause follow-up bias.
Researchers need to decide during the planning stage of the study if they will work with the entire target population or a sample. Working with a sample involves different steps, including sample size estimation, identification of the sample frame, and selection of the sampling method to be adopted.
Financial Support: None.
* Study performed at Faculdade Meridional - Escola de Medicina (IMED) - Passo Fundo (RS), Brazil.

A Novel Explainable AI Method to Assess Associations between Temporal Patterns in Patient Trajectories and Adverse Outcome Risks: Analyzing Fitness as a Risk Factor of ADRD
- Find this author on Google Scholar
- Find this author on PubMed
- Search for this author on this site
- For correspondence: [email protected]
- Info/History
- Preview PDF
We present a novel explainable artificial intelligence (XAI) method to assess the associations between the temporal patterns in the patient trajectories recorded in longitudinal clinical data and the adverse outcome risks, through explanations for a type of deep neural network model called Hybrid Value-Aware Transformer (HVAT) model. The HVAT models can learn jointly from longitudinal and non-longitudinal clinical data, and in particular can leverage the time-varying numerical values associated with the clinical codes or concepts within the longitudinal data for outcome prediction. The key component of the XAI method is the definitions of two derived variables, the temporal mean and the temporal slope, which are defined for the clinical concepts with associated time-varying numerical values. The two variables represent the overall level and the rate of change over time, respectively, in the trajectory formed by the values associated with the clinical concept. Two operations on the original values are designed for changing the values of the two derived variables separately. The effects of the two variables on the outcome risks learned by the HVAT model are calculated in terms of impact scores and impacts. Interpretations of the impact scores and impacts as being similar to those of odds ratios are also provided. We applied the XAI method to the study of cardiorespiratory fitness (CRF) as a risk factor of Alzheimer's disease and related dementias (ADRD). Using a retrospective case-control study design, we found that each one-unit increase in the overall CRF level is associated with a 5% reduction in ADRD risk, while each one-unit increase in the changing rate of CRF over time is associated with a 1% reduction. A closer investigation revealed that the association between the changing rate of CRF level and the ADRD risk is nonlinear, or more specifically, approximately piecewise linear along the axis of the changing rate on two pieces: the piece of negative changing rates and the piece of positive changing rates.
Competing Interest Statement
The authors have declared no competing interest.
Funding Statement
This study was funded by grants NIH/NIA RF1AG069121 and NIH/NIA 1R01AG073474-01A1
Author Declarations
I confirm all relevant ethical guidelines have been followed, and any necessary IRB and/or ethics committee approvals have been obtained.
The details of the IRB/oversight body that provided approval or exemption for the research described are given below:
IRB of Washington DC VA gave ethical approval for this work.
I confirm that all necessary patient/participant consent has been obtained and the appropriate institutional forms have been archived, and that any patient/participant/sample identifiers included were not known to anyone (e.g., hospital staff, patients or participants themselves) outside the research group so cannot be used to identify individuals.
I understand that all clinical trials and any other prospective interventional studies must be registered with an ICMJE-approved registry, such as ClinicalTrials.gov. I confirm that any such study reported in the manuscript has been registered and the trial registration ID is provided (note: if posting a prospective study registered retrospectively, please provide a statement in the trial ID field explaining why the study was not registered in advance).
I have followed all appropriate research reporting guidelines, such as any relevant EQUATOR Network research reporting checklist(s) and other pertinent material, if applicable.
Data Availability
Population-level aggregated data in this study are available upon reasonable request to the authors
View the discussion thread.
Thank you for your interest in spreading the word about medRxiv.
NOTE: Your email address is requested solely to identify you as the sender of this article.

Citation Manager Formats
- EndNote (tagged)
- EndNote 8 (xml)
- RefWorks Tagged
- Ref Manager
- Tweet Widget
- Facebook Like
- Google Plus One
- Addiction Medicine (324)
- Allergy and Immunology (628)
- Anesthesia (165)
- Cardiovascular Medicine (2377)
- Dentistry and Oral Medicine (289)
- Dermatology (207)
- Emergency Medicine (379)
- Endocrinology (including Diabetes Mellitus and Metabolic Disease) (837)
- Epidemiology (11775)
- Forensic Medicine (10)
- Gastroenterology (703)
- Genetic and Genomic Medicine (3744)
- Geriatric Medicine (350)
- Health Economics (634)
- Health Informatics (2399)
- Health Policy (933)
- Health Systems and Quality Improvement (898)
- Hematology (341)
- HIV/AIDS (782)
- Infectious Diseases (except HIV/AIDS) (13318)
- Intensive Care and Critical Care Medicine (767)
- Medical Education (365)
- Medical Ethics (105)
- Nephrology (398)
- Neurology (3507)
- Nursing (198)
- Nutrition (526)
- Obstetrics and Gynecology (674)
- Occupational and Environmental Health (664)
- Oncology (1824)
- Ophthalmology (538)
- Orthopedics (219)
- Otolaryngology (287)
- Pain Medicine (233)
- Palliative Medicine (66)
- Pathology (446)
- Pediatrics (1035)
- Pharmacology and Therapeutics (426)
- Primary Care Research (420)
- Psychiatry and Clinical Psychology (3178)
- Public and Global Health (6145)
- Radiology and Imaging (1280)
- Rehabilitation Medicine and Physical Therapy (747)
- Respiratory Medicine (828)
- Rheumatology (379)
- Sexual and Reproductive Health (372)
- Sports Medicine (323)
- Surgery (402)
- Toxicology (50)
- Transplantation (172)
- Urology (146)

IMAGES
VIDEO
COMMENTS
Sampling methods are crucial for conducting reliable research. In this article, you will learn about the types, techniques and examples of sampling methods, and how to choose the best one for your study. Scribbr also offers free tools and guides for other aspects of academic writing, such as citation, bibliography, and fallacy.
A sampling plan is a formal plan specifying a sampling method, a sample size, and procedure for recruiting participants (Box 1) ... Extreme case sampling: Purposeful selection of the most unusual cases. ... It is common in qualitative research to combine more than one data collection method in one study. You should always choose your data ...
Presenting individual case studies has highlighted how purposive sampling can be integrated into varying contexts dependent on study design. The sampling strategies clearly situate each study in terms of trustworthiness for data collection and analysis. The selected approach to purposive sampling used in each case aligns to the research ...
We could choose a sampling method based on whether we want to account for sampling bias; a random sampling method is often preferred over a non-random method for this reason. Random sampling examples include: simple, systematic, stratified, and cluster sampling. Non-random sampling methods are liable to bias, and common examples include ...
This paper will examine the available methods in sampling participants for qualitative study. Specifically, the paper will discuss the sampling frame suitable for case study, such as single-case (holistic and embedded), multi-case, and a snowball or network sampling procedure. Discussion will also involve challenges anticipated for each ...
Simple random sampling. Simple random sampling involves selecting participants in a completely random fashion, where each participant has an equal chance of being selected.Basically, this sampling method is the equivalent of pulling names out of a hat, except that you can do it digitally.For example, if you had a list of 500 people, you could use a random number generator to draw a list of 50 ...
The term case study is confusing because the same term is used multiple ways. The term can refer to the methodology, that is, a system of frameworks used to design a study, or the methods used to conduct it. Or, case study can refer to a type of academic writing that typically delves into a problem, process, or situation.
Presenting individual case studies has highlighted how purposive sampling can be integrated into varying contexts dependent on study design. The sampling strategies clearly situate each study in terms of trustworthiness for data collection and analysis. The selected approach to purposive sampling used in each case aligns to the research ...
A case study is a detailed study of a specific subject, such as a person, group, place, event, organisation, or phenomenon. Case studies are commonly used in social, educational, clinical, and business research. A case study research design usually involves qualitative methods, but quantitative methods are sometimes also used.
Snowball sampling (Noy, 2008) is perhaps the most common sampling method used in qualitative studies. To operationalize this strategy, the researcher starts by identifying some individuals relevant to the study, for example, two cases in a study in the research context, and then these two cases help to locate other useful participants, i.e ...
Case study method is the most widely used method in academia for researchers interested in qualitative research (Baskarada, 2014).Research students select the case study as a method without understanding array of factors that can affect the outcome of their research.
Regarding differences by methodology, he found case studies had a mean sample size of 188; ethnographies 128; grounded theory, 59; narrative inquiry, 18; and phenomenology, 21. ... claim, sample size will largely depend on the method. (p. 87), "In truth," they write, "many decisions about sample size are made on the basis of resources ...
Answer 1: In qualitative research, samples are selected subjectively according to. the pur pose of the study, whereas in quantitative researc h probability sampling. technique are used to select ...
Sampling can be divided in a number of different ways. At a basic level, with the exception of total population sampling you will often see the divide between random sampling of a representative population and non-random sampling. Clearly, for many more quantitative-minded researchers, non-random sampling is the second-choice approach as it creates
In week five, students learn about nonrandom and random sampling techniques (snowball sampling, simple random sampling, etc.). In discussion section later that week, students apply this knowledge to a hypothetical case study where a researcher aims to study the experiences of homeless people in the United States. Students learned about the pros ...
Types and Methods of Case Study are as follows: Single-Case Study. A single-case study is an in-depth analysis of a single case. This type of case study is useful when the researcher wants to understand a specific phenomenon in detail. ... Biased sampling: Case studies may rely on purposive or convenience sampling, which can introduce bias into ...
Principles of Purposeful Sampling. Purposeful sampling is a technique widely used in qualitative research for the identification and selection of information-rich cases for the most effective use of limited resources (Patton, 2002).This involves identifying and selecting individuals or groups of individuals that are especially knowledgeable about or experienced with a phenomenon of interest ...
Hi. This is my first attempt at conducting a research. My topic reads: The effects of supporting employee's personal development and growth on the organization's performance. It involves multiple case studies (about four companies). But I'm struggling with the right sampling technique to use. Kindly help.
Several sampling techniques are used in qualitative research, each with strengths and weaknesses. In this section, let's explore four standard sampling techniques used in qualitative research: purposive sampling, convenience sampling, snowball sampling, and theoretical sampling. We'll break down the definition of each technique, when to use ...
Target sampling is a form of non-probability sampling that involves intentionally sampling a certain population or group. An example is going to the household that looks the most damaged or like it will get "best" results. Sequential sampling. Sequential sampling is going to one house after another in order.
Additionally, four case studies were written to reflect the most recent anticipated changes to MAiD legislation and all used the same open-ended core questions to address respondents' perspectives about the patient's right to make the decision, comfort in assisting a physician or NP to administer MAiD in that scenario, and hypothesized ...
A case study is one of the most commonly used methodologies of social research. This article attempts to look into the various dimensions of a case study research strategy, the different epistemological strands which determine the particular case study type and approach adopted in the field, discusses the factors which can enhance the effectiveness of a case study research, and the debate ...
Objective The primary objective is to identify which observational research methods have been used in the last 5 years in rare disease drug evaluation and how they are applied to generate adequate evidence regarding the real-world effectiveness or safety of rare disease drugs. Background Rare disease is an umbrella term for a condition which affects <200,000 people each year and despite the ...
Objective To examine the association between prescriber workforce exit, long term opioid treatment discontinuation, and clinical outcomes. Design Quasi-experimental difference-in-differences study Setting 20% sample of US Medicare beneficiaries, 2011-18. Participants People receiving long term opioid treatment whose prescriber stopped providing office based patient care or exited the workforce ...
Sampling method. The sampling method selects a subset of individuals or items from a larger population to conduct research. The researchers employed a two-stage cluster sampling technique to determine the participants for this study. A list of blended MOOC classes offered at UCC was obtained from the staff's mailing list.
However, the fixed method usually involves the use of sampling weights in the statistical analysis (inverse of the probability of selection or 1/P). ... However, in a case-control study, we face the challenge of selecting two different samples for the same study. One sample is formed by the cases, which are identified based on the diagnosis of ...
Case 1: In a survey regarding job objectives, a business school wishes to make sure that participants of various ages are represented. They create quotas and sample people until all of the quotas are met for every age group. Case 2: The goal of the study is to comprehend how different business industry job responsibilities are perceived. Participants are sampled to meet the quotas that are set ...
Community green spaces (CGSs) constitute a crucial element of urban land use, playing a pivotal role in maintaining the stability of urban ecosystems and enhancing the overall quality of the urban environment. Through the post-occupancy evaluation (POE) of green spaces, we can gain insights into residents' actual needs and usage habits, providing scientific evidence for the planning, design ...
We present a novel explainable artificial intelligence (XAI) method to assess the associations between the temporal patterns in the patient trajectories recorded in longitudinal clinical data and the adverse outcome risks, through explanations for a type of deep neural network model called Hybrid Value-Aware Transformer (HVAT) model. The HVAT models can learn jointly from longitudinal and non ...