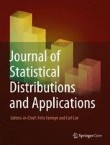
- Search by keyword
- Search by citation
Page 1 of 3

A generalization to the log-inverse Weibull distribution and its applications in cancer research
In this paper we consider a generalization of a log-transformed version of the inverse Weibull distribution. Several theoretical properties of the distribution are studied in detail including expressions for i...
- View Full Text
Approximations of conditional probability density functions in Lebesgue spaces via mixture of experts models
Mixture of experts (MoE) models are widely applied for conditional probability density estimation problems. We demonstrate the richness of the class of MoE models by proving denseness results in Lebesgue space...
Structural properties of generalised Planck distributions
A family of generalised Planck (GP) laws is defined and its structural properties explored. Sometimes subject to parameter restrictions, a GP law is a randomly scaled gamma law; it arises as the equilibrium la...
New class of Lindley distributions: properties and applications
A new generalized class of Lindley distribution is introduced in this paper. This new class is called the T -Lindley{ Y } class of distributions, and it is generated by using the quantile functions of uniform, expon...
Tolerance intervals in statistical software and robustness under model misspecification
A tolerance interval is a statistical interval that covers at least 100 ρ % of the population of interest with a 100(1− α ) % confidence, where ρ and α are pre-specified values in (0, 1). In many scientific fields, su...
Combining assumptions and graphical network into gene expression data analysis
Analyzing gene expression data rigorously requires taking assumptions into consideration but also relies on using information about network relations that exist among genes. Combining these different elements ...
A comparison of zero-inflated and hurdle models for modeling zero-inflated count data
Counts data with excessive zeros are frequently encountered in practice. For example, the number of health services visits often includes many zeros representing the patients with no utilization during a follo...
A general stochastic model for bivariate episodes driven by a gamma sequence
We propose a new stochastic model describing the joint distribution of ( X , N ), where N is a counting variable while X is the sum of N independent gamma random variables. We present the main properties of this gene...
A flexible multivariate model for high-dimensional correlated count data
We propose a flexible multivariate stochastic model for over-dispersed count data. Our methodology is built upon mixed Poisson random vectors ( Y 1 ,…, Y d ), where the { Y i } are conditionally independent Poisson random...
Generalized fiducial inference on the mean of zero-inflated Poisson and Poisson hurdle models
Zero-inflated and hurdle models are widely applied to count data possessing excess zeros, where they can simultaneously model the process from how the zeros were generated and potentially help mitigate the eff...
Multivariate distributions of correlated binary variables generated by pair-copulas
Correlated binary data are prevalent in a wide range of scientific disciplines, including healthcare and medicine. The generalized estimating equations (GEEs) and the multivariate probit (MP) model are two of ...
On two extensions of the canonical Feller–Spitzer distribution
We introduce two extensions of the canonical Feller–Spitzer distribution from the class of Bessel densities, which comprise two distinct stochastically decreasing one-parameter families of positive absolutely ...
A new trivariate model for stochastic episodes
We study the joint distribution of stochastic events described by ( X , Y , N ), where N has a 1-inflated (or deflated) geometric distribution and X , Y are the sum and the maximum of N exponential random variables. Mod...
A flexible univariate moving average time-series model for dispersed count data
Al-Osh and Alzaid ( 1988 ) consider a Poisson moving average (PMA) model to describe the relation among integer-valued time series data; this model, however, is constrained by the underlying equi-dispersion assumpt...
Spatio-temporal analysis of flood data from South Carolina
To investigate the relationship between flood gage height and precipitation in South Carolina from 2012 to 2016, we built a conditional autoregressive (CAR) model using a Bayesian hierarchical framework. This ...
Affine-transformation invariant clustering models
We develop a cluster process which is invariant with respect to unknown affine transformations of the feature space without knowing the number of clusters in advance. Specifically, our proposed method can iden...
Distributions associated with simultaneous multiple hypothesis testing
We develop the distribution for the number of hypotheses found to be statistically significant using the rule from Simes (Biometrika 73: 751–754, 1986) for controlling the family-wise error rate (FWER). We fin...
New families of bivariate copulas via unit weibull distortion
This paper introduces a new family of bivariate copulas constructed using a unit Weibull distortion. Existing copulas play the role of the base or initial copulas that are transformed or distorted into a new f...
Generalized logistic distribution and its regression model
A new generalized asymmetric logistic distribution is defined. In some cases, existing three parameter distributions provide poor fit to heavy tailed data sets. The proposed new distribution consists of only t...
The spherical-Dirichlet distribution
Today, data mining and gene expressions are at the forefront of modern data analysis. Here we introduce a novel probability distribution that is applicable in these fields. This paper develops the proposed sph...
Item fit statistics for Rasch analysis: can we trust them?
To compare fit statistics for the Rasch model based on estimates of unconditional or conditional response probabilities.
Exact distributions of statistics for making inferences on mixed models under the default covariance structure
At this juncture when mixed models are heavily employed in applications ranging from clinical research to business analytics, the purpose of this article is to extend the exact distributional result of Wald (A...
A new discrete pareto type (IV) model: theory, properties and applications
Discrete analogue of a continuous distribution (especially in the univariate domain) is not new in the literature. The work of discretizing continuous distributions begun with the paper by Nakagawa and Osaki (197...
Density deconvolution for generalized skew-symmetric distributions
The density deconvolution problem is considered for random variables assumed to belong to the generalized skew-symmetric (GSS) family of distributions. The approach is semiparametric in that the symmetric comp...
The unifed distribution
We introduce a new distribution with support on (0,1) called unifed. It can be used as the response distribution for a GLM and it is suitable for data aggregation. We make a comparison to the beta regression. ...
On Burr III Marshal Olkin family: development, properties, characterizations and applications
In this paper, a flexible family of distributions with unimodel, bimodal, increasing, increasing and decreasing, inverted bathtub and modified bathtub hazard rate called Burr III-Marshal Olkin-G (BIIIMO-G) fam...
The linearly decreasing stress Weibull (LDSWeibull): a new Weibull-like distribution
Motivated by an engineering pullout test applied to a steel strip embedded in earth, we show how the resulting linearly decreasing force leads naturally to a new distribution, if the force under constant stress i...
Meta analysis of binary data with excessive zeros in two-arm trials
We present a novel Bayesian approach to random effects meta analysis of binary data with excessive zeros in two-arm trials. We discuss the development of likelihood accounting for excessive zeros, the prior, a...
On ( p 1 ,…, p k )-spherical distributions
The class of ( p 1 ,…, p k )-spherical probability laws and a method of simulating random vectors following such distributions are introduced using a new stochastic vector representation. A dynamic geometric disintegra...
A new class of survival distribution for degradation processes subject to shocks
Many systems experience gradual degradation while simultaneously being exposed to a stream of random shocks of varying magnitudes that eventually cause failure when a shock exceeds the residual strength of the...
A new extended normal regression model: simulations and applications
Various applications in natural science require models more accurate than well-known distributions. In this context, several generators of distributions have been recently proposed. We introduce a new four-par...
Multiclass analysis and prediction with network structured covariates
Technological advances associated with data acquisition are leading to the production of complex structured data sets. The recent development on classification with multiclass responses makes it possible to in...
High-dimensional star-shaped distributions
Stochastic representations of star-shaped distributed random vectors having heavy or light tail density generating function g are studied for increasing dimensions along with corresponding geometric measure repre...
A unified complex noncentral Wishart type distribution inspired by massive MIMO systems
The eigenvalue distributions from a complex noncentral Wishart matrix S = X H X has been the subject of interest in various real world applications, where X is assumed to be complex matrix variate normally distribute...
Particle swarm based algorithms for finding locally and Bayesian D -optimal designs
When a model-based approach is appropriate, an optimal design can guide how to collect data judiciously for making reliable inference at minimal cost. However, finding optimal designs for a statistical model w...
Admissible Bernoulli correlations
A multivariate symmetric Bernoulli distribution has marginals that are uniform over the pair {0,1}. Consider the problem of sampling from this distribution given a prescribed correlation between each pair of v...
On p -generalized elliptical random processes
We introduce rank- k -continuous axis-aligned p -generalized elliptically contoured distributions and study their properties such as stochastic representations, moments, and density-like representations. Applying th...
Parameters of stochastic models for electroencephalogram data as biomarkers for child’s neurodevelopment after cerebral malaria
The objective of this study was to test statistical features from the electroencephalogram (EEG) recordings as predictors of neurodevelopment and cognition of Ugandan children after coma due to cerebral malari...
A new generalization of generalized half-normal distribution: properties and regression models
In this paper, a new extension of the generalized half-normal distribution is introduced and studied. We assess the performance of the maximum likelihood estimators of the parameters of the new distribution vi...
Analytical properties of generalized Gaussian distributions
The family of Generalized Gaussian (GG) distributions has received considerable attention from the engineering community, due to the flexible parametric form of its probability density function, in modeling ma...
A new Weibull- X family of distributions: properties, characterizations and applications
We propose a new family of univariate distributions generated from the Weibull random variable, called a new Weibull-X family of distributions. Two special sub-models of the proposed family are presented and t...
The transmuted geometric-quadratic hazard rate distribution: development, properties, characterizations and applications
We propose a five parameter transmuted geometric quadratic hazard rate (TG-QHR) distribution derived from mixture of quadratic hazard rate (QHR), geometric and transmuted distributions via the application of t...
A nonparametric approach for quantile regression
Quantile regression estimates conditional quantiles and has wide applications in the real world. Estimating high conditional quantiles is an important problem. The regular quantile regression (QR) method often...
Mean and variance of ratios of proportions from categories of a multinomial distribution
Ratio distribution is a probability distribution representing the ratio of two random variables, each usually having a known distribution. Currently, there are results when the random variables in the ratio fo...
The power-Cauchy negative-binomial: properties and regression
We propose and study a new compounded model to extend the half-Cauchy and power-Cauchy distributions, which offers more flexibility in modeling lifetime data. The proposed model is analytically tractable and c...
Families of distributions arising from the quantile of generalized lambda distribution
In this paper, the class of T-R { generalized lambda } families of distributions based on the quantile of generalized lambda distribution has been proposed using the T-R { Y } framework. In the development of the T - R {
Risk ratios and Scanlan’s HRX
Risk ratios are distribution function tail ratios and are widely used in health disparities research. Let A and D denote advantaged and disadvantaged populations with cdfs F ...
Joint distribution of k -tuple statistics in zero-one sequences of Markov-dependent trials
We consider a sequence of n , n ≥3, zero (0) - one (1) Markov-dependent trials. We focus on k -tuples of 1s; i.e. runs of 1s of length at least equal to a fixed integer number k , 1≤ k ≤ n . The statistics denoting the n...
Quantile regression for overdispersed count data: a hierarchical method
Generalized Poisson regression is commonly applied to overdispersed count data, and focused on modelling the conditional mean of the response. However, conditional mean regression models may be sensitive to re...
Describing the Flexibility of the Generalized Gamma and Related Distributions
The generalized gamma (GG) distribution is a widely used, flexible tool for parametric survival analysis. Many alternatives and extensions to this family have been proposed. This paper characterizes the flexib...
- ISSN: 2195-5832 (electronic)
Click through the PLOS taxonomy to find articles in your field.
For more information about PLOS Subject Areas, click here .
Loading metrics
Open Access
Peer-reviewed
Research Article
spmodel: Spatial statistical modeling and prediction in R
Roles Conceptualization, Data curation, Formal analysis, Investigation, Methodology, Project administration, Resources, Software, Supervision, Validation, Visualization, Writing – original draft
* E-mail: [email protected]
Affiliation United States Environmental Protection Agency, Corvallis, Oregon, United States of America

Roles Formal analysis, Investigation, Methodology, Project administration, Resources, Software, Supervision, Validation, Visualization, Writing – original draft
Affiliation Department of Math, Computer Science, and Statistics, St. Lawrence University, Canton, New York, United States of America
Roles Data curation, Formal analysis, Investigation, Methodology, Project administration, Resources, Software, Supervision, Validation, Visualization, Writing – original draft
Affiliation Marine Mammal Laboratory, National Oceanic and Atmospheric Administration Alaska Fisheries Science Center, Seattle, Washington, United States of America
- Michael Dumelle,
- Matt Higham,
- Jay M. Ver Hoef
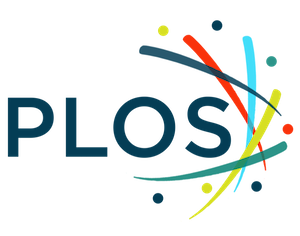
- Published: March 9, 2023
- https://doi.org/10.1371/journal.pone.0282524
- Peer Review
- Reader Comments
Editor: A. K. M. Anisur Rahman, Bangladesh Agricultural University, BANGLADESH
Received: October 11, 2022; Accepted: February 16, 2023; Published: March 9, 2023
This is an open access article, free of all copyright, and may be freely reproduced, distributed, transmitted, modified, built upon, or otherwise used by anyone for any lawful purpose. The work is made available under the Creative Commons CC0 public domain dedication.
Data Availability: The data used in this manuscript are available upon download of the spmodel R package from CRAN. To learn more, visit https://CRAN.R-project.org/package=spmodel . The manuscript also has a supplementary R package that contains all of the text, figures, and code used in the manuscript’s creation. To learn more, visit https://github.com/USEPA/spmodel.manuscript .
Funding: The author(s) received no specific funding for this work.
Competing interests: The authors have declared that no competing interests exist.
Introduction
spmodel implements model-based inference, which relies on fitting a statistical model. Model-based inference is different than design-based inference, which relies on random sampling and estimators that incorporate the properties of the random sample [ 1 ]. [ 2 ] defines two types of spatial data that can be analyzed using model-based inference: point-referenced data and areal data (areal data are sometimes called lattice data). Spatial data are point-referenced when they are observed at point-locations indexed by x-coordinates and y-coordinates on a spatially continuous surface with an infinite number of locations. Spatial models for point-referenced data are sometimes called geostatistical models. Spatial data are areal when they are observed as part of a finite network of polygons whose connections are indexed by a neighborhood structure. For example, the polygons may represent counties in a state who are neighbors if they share at least one boundary. Spatial models for areal data are sometimes called spatial autoregressive models. For thorough overviews of model-based inference in a spatial context, see [ 2 – 4 ].
The rest of this article is organized as follows. We first give a brief theoretical introduction to spatial linear models. We then outline the variety of methods used to estimate the parameters of spatial linear models. Next we explain how to obtain predictions at unobserved locations. Following that, we detail some advanced modeling features, including random effects, partition factors, anisotropy, and big data approaches. Finally we end with a short discussion.
Before proceeding, we install spmodel from CRAN and load it by running
We create visualizations using ggplot2 [ 20 ], which we install from CRAN and load by running
We also show code that can be used to create interactive visualizations of spatial data with mapview [ 21 ]. mapview has many backgrounds available that contextualize spatial data with topographical information. Before running the mapview code provided interactively, make sure that mapview is installed and loaded.
spmodel contains various methods for generic functions defined outside of spmodel . To find relevant documentation for these methods, run help(“generic.spmodel”, “spmodel”) (e.g., help(“fitted.spmodel”, “spmodel”) , help(“summary.spmodel”, “spmodel”) , help(“plot.spmodel”, “spmodel”) , help(“predict.spmodel”, “spmodel”) , help(“tidy.spmodel”, “spmodel”) , etc.). We provide more details and examples regarding these methods and generics throughout this vignette. For a full list of spmodel functions available, see spmodel ’s documentation manual.
The spatial linear model
One way to define W is through queen contiguity [ 23 ]. Two observations are queen contiguous if they share a boundary. The ij th element of W is then one if observation i and observation j are queen contiguous and zero otherwise. Observations are not considered neighbors with themselves, so each diagonal element of W is zero.
Sometimes each element in the weight matrix W is divided by its respective row sum. This is called row-standardization. Row-standardizing W has several benefits, which are discussed in detail by [ 24 ].
Model fitting
In this section, we show how to use the splm() and spautor() functions to estimate parameters of the spatial linear model. We also explore diagnostic tools in spmodel that evaluate model fit. The splm() and spautor() functions share similar syntactic structure with the lm() function used to fit non-spatial linear models from Eq 1 . splm() and spautor() generally require at least three arguments:
- – formula in splm() is the same as formula in lm()
- data : a data.frame or sf object that contains the response variable, explanatory variables, and spatial information
- spcov_type : the spatial covariance type ( “exponential” , “matern” , “car” , etc)
If data is an sf [ 25 ] object, spatial information is stored in the object’s geometry. If data is a data.frame , then the x-coordinates and y-coordinates must be provided via the xcoord and ycoord arguments (for point-referenced data) or the weight matrix must be provided via the W argument (for areal data).
In the following subsections, we use the point-referenced moss data, an sf object that contains data on heavy metals in mosses near a mining road in Alaska. We view the first few rows of moss by running
We can learn more about moss by running help(“moss”, “spmodel”) , and we can visualize the distribution of log zinc concentration in moss ( Fig 1 ) by running
- PPT PowerPoint slide
- PNG larger image
- TIFF original image
https://doi.org/10.1371/journal.pone.0282524.g001
Log zinc concentration can be viewed interactively in mapview by running
Generally the covariance parameters ( θ ) and fixed effects ( β ) of the spatial linear model require estimation. The default estimation method in spmodel is restricted maximum likelihood [ 26 – 28 ]. Maximum likelihood estimation is also available. For point-referenced data, semivariogram weighted least squares [ 29 ] and semivariogram composite likelihood [ 30 ] are additional estimation methods. The estimation method is chosen using the estmethod argument.
We estimate parameters of a spatial linear model regressing log zinc concentration ( log_Zn ) on log distance to a haul road ( log_dist2road ) using an exponential spatial covariance function by running
We summarize the model fit by running
The fixed effects coefficient table contains estimates, standard errors, z-statistics, and asymptotic p-values for each fixed effect. From this table, we notice there is evidence that mean log zinc concentration significantly decreases with distance from the haul road (p-value < 2e-16). We see the fixed effect estimates by running
The model summary also contains the exponential spatial covariance parameter estimates, which we can view by running
https://doi.org/10.1371/journal.pone.0282524.g002
Model-fit statistics
Roughly 68% of the variability in log zinc is explained by log distance from the road. The pseudo R-squared can be adjusted to account for the number of explanatory variables using the adjust argument. Pseudo R-squared (and the adjusted version) is most helpful for comparing models that have the same covariance structure.
The next two model-fit statistics we consider are the AIC and AICc that [ 31 ] derive for spatial data. The AIC and AICc evaluate the fit of a model with a penalty for the number of parameters estimated. This penalty balances model fit and model parsimony. Lower AIC and AICc indicate a better balance of model fit and parsimony. The AICc is a correction to AIC that is better suited for small sample sizes. As the sample size increases, AIC and AICc converge.
Suppose we want to quantify the difference in model quality between the spatial model and a non-spatial model using the AIC and AICc criteria. We fit a non-spatial model ( Eq 1 ) in spmodel by running
This model is equivalent to one fit using lm() . We compute the spatial AIC and AICc of the spatial model and non-spatial model by running
The noticeably lower AIC and AICc of of the spatial model indicate that it is a better fit to the data than the non-spatial model. Recall that these AIC and AICc comparisons are valid because both models are fit using restricted maximum likelihood (the default).
Another approach to comparing the fitted models is to perform leave-one-out cross validation [ 33 ]. In leave-one-out cross validation, a single observation is removed from the data, the model is re-fit, and a prediction is made for the held-out observation. Then, a loss metric like mean-squared-prediction error is computed and used to evaluate model fit. The lower the mean-squared-prediction error, the better the model fit. For computational efficiency, leave-one-out cross validation in spmodel is performed by first estimating θ using all the data and then re-estimating β for each observation. We perform leave-one-out cross validation for the spatial and non-spatial model by running
The noticeably lower mean-squared-prediction error of the spatial model indicates that it is a better fit to the data than the non-spatial model.
Diagnostics
In addition to model fit metrics, spmodel provides functions to compute diagnostic metrics that help assess model assumptions and identify unusual observations.
Larger hat values indicate more leverage, and observations with large hat values may be unusual and warrant further investigation.
Fitted values for the spatially dependent random errors ( τ ), spatially independent random errors ( ϵ ), and random effects can also be obtained via fitted() by changing the type argument.
When the model is correct, the standardized residuals have mean zero, variance one, and are uncorrelated.
It is common to check linear model assumptions through visualizations. We can visualize the standardized residuals vs fitted values by running
When the model is correct, the standardized residuals should be evenly spread around zero with no discernible pattern. We can visualize a normal QQ-plot of the standardized residuals by running
When the standardized residuals are normally distributed, they should closely follow the normal QQ-line.
The Cook’s distance versus leverage (hat values) can be visualized by running
The broom functions: tidy() , glance() , and augment()
This tibble format makes it easy to pull out the coefficient names, estimates, standard errors, z-statistics, and p-values from the summary() output.
The glance() function returns a tidy tibble of model-fit statistics:
The glances() function is an extension of glance() that can be used to look at many models simultaneously:
Finally, the augment() function augments the original data with model diagnostics:
By default, only the columns of data used to fit the model are returned alongside the diagnostics. All original columns of data are returned by setting drop to FALSE . augment() is especially powerful when the data are an sf object because model diagnostics can be easily visualized spatially. For example, we could subset the augmented object so that it only includes observations whose standardized residuals have absolute values greater than some cutoff and then map them.
An areal data example
Next we use the seal data, an sf object that contains the log of the estimated harbor-seal trends from abundance data across polygons in Alaska, to provide an example of fitting a spatial linear model for areal data using spautor() . We view the first few rows of seal by running
We can learn more about the data by running help(“seal”, “spmodel”) .
We can visualize the distribution of log seal trends in the seal data ( Fig 3 ) by running
Polygons are gray if seal trends are missing.
https://doi.org/10.1371/journal.pone.0282524.g003
Log trends can be viewed interactively in mapview by running
The gray polygons denote areas where the log trend is missing. These missing areas need to be kept in the data while fitting the model to preserve the overall neighborhood structure.
We estimate parameters of a spatial autoregressive model for log seal trends ( log_trend ) using an intercept-only model with a conditional autoregressive (CAR) spatial covariance by running
If a weight matrix is not provided to spautor() , it is calculated internally using queen contiguity. Recall that queen contiguity defines two observations as neighbors if they share at least one common boundary. If at least one observation has no neighbors, the extra parameter is estimated, which quantifies variability among observations without neighbors. By default, spautor() uses row standardization [ 24 ] and assumes an independent error variance ( ie ) of zero.
We summarize, tidy, glance at, and augment the fitted model by running
Note that for spautor() models, the ie spatial covariance parameter is assumed zero by default (and omitted from the summary() output). This default behavior can be overridden by specifying ie in the spcov_initial argument to spautor() . Also note that the pseudo R-squared is zero because there are no explanatory variables in the model (i.e., it is an intercept-only model).
In this section, we show how to use predict() to perform spatial prediction (also called Kriging) in spmodel . We will fit a model using the point-referenced sulfate data, an sf object that contains sulfate measurements in the conterminous United States, and make predictions for each location in the point-referenced sulfate_preds data, an sf object that contains locations in the conterminous United States at which to predict sulfate.
We first visualize the distribution of the sulfate data ( Fig 4A ) by running
In A (top), observed sulfate is visualized. In B (bottom), sulfate predictions are visualized.
https://doi.org/10.1371/journal.pone.0282524.g004
We then fit a spatial linear model for sulfate using an intercept-only model with a spherical spatial covariance function by running
Then we obtain best linear unbiased predictions (Kriging predictions) using predict() . The newdata argument contains the locations at which to predict, and we store the predictions as a new variable in sulfate_preds called preds by running
We can visualize the model predictions ( Fig 4B ) by running
It is important to properly specify the newdata object when running predict() . If explanatory variables were used to fit the model, the same explanatory variables must be included in newdata with the same names as they have in data . Additionally, if an explanatory variable is categorical or a factor, the values of this variable in newdata must also be values in data (e.g., if a categorical variable with values “A” , and “B” was used to fit the model, the corresponding variable in newdata cannot have a value “C” ). If data is a data.frame , coordinates must be included in newdata with the same names as they have in data . If data is an sf object, coordinates must be included in newdata with the same geometry name as they have in data . When using projected coordinates, the projection for newdata should be the same as the projection for data .
Prediction standard errors are returned by setting the se.fit argument to TRUE :
The interval argument determines the type of interval returned. If interval is “none” (the default), no intervals are returned. If interval is “prediction” , then 100 * level % prediction intervals are returned (the default is 95% prediction intervals):
If interval is “confidence” , the predictions are instead the estimated mean given each observation’s explanatory variable values (i.e., fitted values) and the corresponding 100 * level % confidence intervals are returned:
The predict() output structure changes based on interval and se.fit . For more details, run help(“predict.spmodel”, “spmodel”) .
Previously we used the augment() function to augment data with model diagnostics. We can also use augment() to augment newdata with predictions, standard errors, and intervals. We remove the model predictions from sulfate_preds before showing how augment() is used to obtain the same predictions by running
We then view the first few rows of sulfate_preds augmented with a 90% prediction interval by running
Here .fitted represents the predictions, .lower represents the lower bound of the 90% prediction intervals, and .upper represents the upper bound of the 90% prediction intervals.
An alternative (but equivalent) approach can be used for model fitting and prediction that circumvents the need to keep data and newdata as separate objects. Suppose that observations requiring prediction are stored in data as missing ( NA ) values. We can add a column of missing values to sulfate_preds and then bind it together with sulfate by running
We can then fit a spatial linear model by running
The missing values are ignored for model-fitting but stored in sulfmod_with_NA as newdata :
We can then predict the missing values by running
The call to predict() finds in sulfmod_with_NA the newdata object and is equivalent to
We can also use augment() to make the predictions for the data set with missing values by running
Unlike predict() , augment() explicitly requires the newdata argument be specified in order to obtain predictions. Omitting newdata (e.g., running augment(sulfmod_with_NA) ) returns model diagnostics, not predictions.
For areal data models fit with spautor() , predictions cannot be computed at locations that were not incorporated in the neighborhood structure used to fit the model. Thus, predictions are only possible for observations in data whose response values are missing ( NA ), as their locations are incorporated into the neighborhood structure. For example, we make predictions of log seal trends at the missing polygons from Fig 3 by running
We can also use augment() to make the predictions:
Advanced features
spmodel offers several advanced features for fitting spatial linear models. We briefly discuss some of these features next using the moss data and some simulated data. Technical details for each advanced feature can be seen by running vignette(“technical”, “spmodel”) .
Fixing spatial covariance parameters
We may desire to fix specific spatial covariance parameters at a particular value. Perhaps some parameter value is known, for example. Or perhaps we want to compare nested models where a reduced model uses a fixed parameter value while the full model estimates the parameter. Fixing spatial covariance parameters while fitting a model is possible using the spcov_initial argument to splm() and spautor() . The spcov_initial argument takes an spcov_initial object (run help(“spcov_initial”, “spmodel”) for more). spcov_initial objects can also be used to specify initial values used during optimization, even if they are not assumed to be fixed. By default, spmodel uses a grid search to find suitable initial values to use during optimization.
As an example, suppose our goal is to compare a model with an exponential covariance and dependent error variance, independent error variance, and range parameter to a similar model that instead assumes the independent random error variance parameter (nugget) is zero. First, the spcov_initial object is specified for the latter model:
The init output shows that the ie parameter has an initial value of zero that is assumed to be known. Next the model is fit:
Notice that because the spcov_initial object contains information about the spatial covariance type, the spcov_type argument is not required when spcov_initial is provided. We can use glances() to glance at both models:
The lower AIC and AICc of the full model compared to the reduced model indicates that the independent random error variance is important to the model. A likelihood ratio test comparing the full and reduced models is also possible using anova() .
Fitting and predicting for multiple models
Fitting multiple models is possible with a single call to splm() or spautor() when spcov_type is a vector with length greater than one or spcov_initial is a list (with length greater than one) of spcov_initial objects. We fit three separate spatial linear models using the exponential spatial covariance, spherical spatial covariance, and no spatial covariance by running
Then glances() is used to glance at each fitted model object:
And predict() is used to predict newdata separately fo each fitted model object:
Currently, glances() and predict() are the only spmodel generic functions that operate on an object that contains multiple model fits. Generic functions that operate on individual models can still be called when the argument is an individual model object. For example, we can compute the AIC of the model fit using the exponential covariance function by running
Random effects
The first example explores random intercepts for the sample variable. The sample variable indexes each unique location, which can have replicate observations due to field duplicates ( field_dup ) and lab replicates ( lab_rep ). There are 365 observations in moss at 318 unique locations, which means that 47 observations in moss are either field duplicates or lab replicates. It is likely that the repeated observations at a location are correlated with one another. We can incorporate this repeated-observation correlation by creating a random intercept for each level of sample . We model the random intercepts for sample by running
Note that ~ sample is shorthand for ~ (1 | sample) , which is more explicit notation that indicates random intercepts for each level of sample .
The second example adds a random intercept for year , which creates extra correlation for observations within a year. It also adds a random slope for log_dist2road within year , which lets the effect of log_dist2road vary between years. We fit this model by running
Note that ~ sample + (log_dist2road | year) is shorthand for ~ (1 | sample) + (log_dist2road | year) . If only random slopes within a year are desired (and no random intercepts), a - 1 is given to the relevant portion of the formula: (log_dist2road—1 | year) . When there is more than one term in random , each term must be surrounded by parentheses (recall that the random intercept shorthand automatically includes relevant parentheses).
We can compare the AIC of all three models by running
The rand2 model has the lowest AIC.
It is possible to fix random effect variances using the randcov_initial argument, and randcov_initial can also be used to set initial values for optimization.
Partition factors
A partition factor is a variable that allows observations to be uncorrelated when they are from different levels of the partition factor. Partition factors are specified in spmodel by providing a formula with a single variable to the partition_factor argument. Suppose that for the moss data, we would like observations in different years ( year ) to be uncorrelated. We fit a model that treats year as a partition factor by running
An isotroptic spatial covariance function (for point-referenced data) behaves similarly in all directions (i.e., is independent of direction) as a function of distance. An anisotropic covariance function does not behave similarly in all directions as a function of distance. Consider the spatial covariance imposed by an eastward-moving wind pattern. A one-unit distance in the x-direction likely means something different than a one-unit distance in the y-direction. Fig 5 shows ellipses for an isotropic and anisotropic covariance function centered at the origin (a distance of zero). The black outline of each ellipse is a level curve of equal correlation. The left ellipse (a circle) represents an isotropic covariance function. The distance at which the correlation between two observations lays on the level curve is the same in all directions. The right ellipse represents an anisotropic covariance function. The distance at which the correlation between two observations lays on the level curve is different in different directions.
In A (left), the isotropic covariance function is visualized. In B (right), the anisotropic covariance function is visualized. The black outline of each ellipse is a level curve of equal correlation.
https://doi.org/10.1371/journal.pone.0282524.g005
Accounting for anisotropy involves a rotation and scaling of the x-coordinates and y-coordinates such that the spatial covariance function that uses these transformed distances is isotropic. We use the anisotropy argument to splm() to fit a model with anisotropy by running
The rotate parameter is between zero and π radians and represents the angle of a clockwise rotation of the ellipse such that the major axis of the ellipse is the new x-axis and the minor axis of the ellipse is the new y-axis. The scale parameter is between zero and one and represents the ratio of the distance between the origin and the edge of the ellipse along the minor axis to the distance between the origin and the edge of the ellipse along the major axis. The transformation that turns an anisotropic ellipse into an isotropic one (i.e., a circle) requires rotating the coordinates clockwise by rotate and then scaling them the reciprocal of scale . The transformed coordinates are then used instead of the original coordinates to compute distances and spatial covariances.
Note that specifying an initial value for rotate that is different from zero, specifying an initial value for scale that is different from one, or assuming either rotate or scale are unknown in spcov_initial will cause splm() to fit a model with anisotropy (and will override anisotropy = FALSE ). Estimating anisotropy parameters is only possible for maximum likelihood and restricted maximum likelihood estimation, but fixed anisotropy parameters can be accommodated for semivariogram weighted least squares or semivariogram composite likelihood estimation. Also note that anisotropy is not relevant for areal data because the spatial covariance function depends on a neighborhood structure instead of distances between locations.
Simulating spatial data
The sprnorm() function is used to simulate normal (Gaussian) spatial data. To use sprnorm() , the spcov_params() function is used to create an spcov_params object. The spcov_params() function requires the spatial covariance type and parameter values. We create an spcov_params object by running
We set a reproducible seed and then simulate data at 3000 random locations in the unit square using the spatial covariance parameters in sim_params by running
We can visualize the simulated data ( Fig 6A ) by running
In A (top), spatial data are simulated in the unit square. A spatial linear model is fit using the default big data approximation for model-fitting. In B (bottom), predictions are made using the fitted model and the default big data approximation for prediction.
https://doi.org/10.1371/journal.pone.0282524.g006
There is noticeable spatial patterning in the response variable ( resp ). The default mean in sprnorm() is zero for all observations, though a mean vector can be provided using the mean argument. The default number of samples generated in sprnorm() is one, though this can be changed using the samples argument. Because sim_data is a tibble ( data.frame ) and not an sf object, the columns in sim_data representing the x-coordinates and y-coordinates must be provided to sprnorm() .
Note that the output from coef(object, type = “spcov”) is a spcov_params object. This is useful we want to simulate data given the estimated spatial covariance parameters from a fitted model. Random effects are incorporated into simulation via the randcov_params argument.
The computational cost associated with model fitting is exponential in the sample size for all estimation methods. For maximum likelihood and restricted maximum likelihood, the computational cost of estimating θ is cubic. For semivariogram weighted least squares and semivariogram composite likelihood, the computational cost of estimating θ is quadratic. The computational cost associated with estimating β and prediction is cubic in the model-fitting sample size, regardless of estimation method. Typically, samples sizes approaching 10,000 make the computational cost of model fitting and prediction infeasible, which necessitates the use of big data methods. spmodel offers big data methods for model fitting of point-referenced data via the local argument to splm() . The method is capable of quickly fitting models with hundreds of thousands to millions of observations. Because of the neighborhood structure of areal data, the big data methods used for point-referenced data do not apply to areal data. Thus, there is no big data method for areal data or local argument to spautor() , so model fitting sample sizes cannot be too large. spmodel offers big data methods for prediction of point-referenced data or areal data via the local argument to predict() , capable of quickly predicting hundreds of thousands to millions of observations rather quickly.
To show how to use spmodel for big data estimation and prediction, we use the sim_data data from the previous subsection. Because sim_data is a tibble ( data.frame ) and not an sf object, the columns in data representing the x-coordinates and y-coordinates must be explicitly provided to splm() .
Model-fitting.
spmodel uses a “local indexing” approximation for big data model fitting of point-referenced data. Observations are first assigned an index. Then for the purposes of model fitting, observations with different indexes are assumed uncorrelated. Assuming observations with different indexes are uncorrelated induces sparsity in the covariance matrix, which greatly reduces the computational time of operations that involve the covariance matrix.
Big data are most simply accommodated by setting local to TRUE . This is shorthand for local = list(method = “random”, size = 50, var_adjust = “theoretical”, parallel = FALSE) , which randomly assigns observations to index groups, ensures each index group has approximately 50 observations, uses the theoretically-correct covariance adjustment, and does not use parallel processing.
Instead of using local = TRUE , we can explicitly set local . For example, we can fit a model using k-means clustering [ 40 ] on the x-coordinates and y-coordinates to create 60 groups (clusters), use the pooled variance adjustment, and use parallel processing with two cores by running
Likelihood-based statistics like AIC() , AICc() , logLik() , and deviance() should not be used to compare a model fit with a big data approximation to a model fit without a big data approximation, as the two approaches maximize different likelihoods.
Prediction.
For point-referenced data, spmodel uses a “local neighborhood” approximation for big data prediction. Each prediction is computed using a subset of the observed data instead of all of the observed data. Before further discussing big data prediction, we simulate 1000 locations in the unit square requiring prediction:
The local argument to predict() controls the big data options. local is a list with several arguments. The arguments to the local list control the method used to subset the observed data, the number of observations in each subset, whether or not to use parallel processing, and if parallel processing is used, the number of cores.
The simplest way to accommodate big data prediction is to set local to TRUE . This is shorthand for local = list(method = “covariance”, size = 50, parallel = FALSE) , which implies that for each location requiring prediction, only the 50 observations in the data most correlated with it are used in the computation, and parallel processing is not used. Using the local1 fitted model, we store these predictions as a variable called preds in the sim_preds data by running
The predictions are visualized ( Fig 6B ) by running
They display a similar pattern as the observed data.
Instead of using local = TRUE , we can explicitly set local :
This code implies that uniquely for each location requiring prediction, only the 30 observations in the data closest to it (in terms of Euclidean distance) are used in the computation and parallel processing is used with two cores.
For areal data, no local neighborhood approximation exists because of the data’s underlying neighborhood structure. Thus, all of the data must be used to compute predictions and by consequence, method and size are not components of the local list. The only components of the local list for areal data are parallel and ncores .
spmodel is a novel, relevant contribution used to fit, summarize, and predict for a variety of spatial statistical models. Spatial linear models for point-referenced data (i.e., geostatistical models) are fit using the splm() function. Spatial linear models for areal data (i.e., autoregressive models) are fit using the spautor() function. Both functions use a common framework and syntax structure. Several model-fit statistics and diagnostics are available. The broom functions tidy() and glance() are used to tidy and glance at a fitted model. The broom function augment() is used to augment data with model diagnostics and augment newdata with predictions. Several advanced features are available to accommodate fixed covariance parameter values, random effects, partition factors, anisotropy, simulating data, and big data approximations for model fitting and prediction.
We appreciate feedback from users regarding spmodel , and we have several plans to add new features to spmodel in the future. To learn more about spmodel or provide feedback, please visit our website at https://usepa.github.io/spmodel/ .
Acknowledgments
We would like to thank the editor and anonymous reviewers for their feedback which greatly improved the manuscript.
The views expressed in this manuscript are those of the authors and do not necessarily represent the views or policies of the U.S. Environmental Protection Agency or the National Oceanic and Atmospheric Administration. Any mention of trade names, products, or services does not imply an endorsement by the U.S. government, the U.S. Environmental Protection Agency, or the National Oceanic and Atmospheric Administration. The U.S. Environmental Protection Agency and the National Oceanic and Atmospheric Administration do not endorse any commercial products, services or enterprises.
- View Article
- Google Scholar
- 2. Cressie N. Statistics for spatial data. John Wiley & Sons; 1993.
- 3. Banerjee S, Carlin BP, Gelfand AE. Hierarchical modeling and analysis for spatial data. Chapman; Hall/CRC; 2003.
- 4. Schabenberger O, Gotway CA. Statistical methods for spatial data analysis: Texts in statistical science. Chapman; Hall/CRC; 2017.
- 5. Nychka D, Furrer R, Paige J, Sain S. Fields: Tools for spatial data. Boulder, CO, USA: University Corporation for Atmospheric Research; 2021. Available: https://github.com/dnychka/fieldsRPackage
- 7. Ribeiro Jr PJ, Diggle P, Christensen O, Schlather M, Bivand R, Ripley B. geoR: Analysis of geostatistical data. 2022. Available: https://CRAN.R-project.org/package=geoR
- 8. Guiness J, Katzfuss M, Fahmy Y. GpGp: Fast Gaussian process computation using Vecchia’s approximation. 2021. Available: https://CRAN.R-project.org/package=GpGp
- 10. Nychka D, Hammerling D, Sain S, Lenssen N. LatticeKrig: Multiresolution Kriging based on Markov random fields. Boulder, CO, USA: University Corporation for Atmospheric Research; 2016.
- 12. Stan Development Team. RStan: The R interface to Stan. 2023. Available: https://mc-stan.org/
- 13. Venables WN, Ripley BD. Modern applied statistics with S. Fourth. New York: Springer; 2002. Available: https://www.stats.ox.ac.uk/pub/MASS4/
- PubMed/NCBI
- 20. Wickham H. Ggplot2: Elegant graphics for data analysis. Springer-Verlag New York; 2016. Available: https://ggplot2.tidyverse.org
- 21. Appelhans T, Detsch F, Reudenbach C, Woellauer S. Mapview: Interactive viewing of spatial data in R. 2022. Available: https://CRAN.R-project.org/package=mapview
- 23. Anselin L, Syabri I, Kho Y. GeoDa: An introduction to spatial data analysis. Handbook of applied spatial analysis. Springer; 2010. pp. 73–89.
- 32. Pinheiro J, Bates D. Mixed-effects models in S and S-PLUS. Springer Science & Business Media; 2006.
- 33. Hastie T, Tibshirani R, Friedman JH, Friedman JH. The elements of statistical learning: Data mining, inference, and prediction. Springer; 2009.
- 34. Montgomery DC, Peck EA, Vining GG. Introduction to linear regression analysis. John Wiley & Sons; 2021.
- 35. Myers RH, Montgomery DC, Vining GG, Robinson TJ. Generalized linear models: With applications in engineering and the sciences. John Wiley & Sons; 2012.
- 36. Cook RD, Weisberg S. Residuals and influence in regression. New York: Chapman; Hall; 1982.
- 37. Robinson D, Hayes A, Couch S. Broom: Convert statistical objects into tidy tibbles. 2021. Available: https://CRAN.R-project.org/package=broom
- 40. MacQueen J. Classification and analysis of multivariate observations. 5th Berkeley Symp Math Statist Probability. University of California Los Angeles LA USA; 1967. pp. 281–297.
Academia.edu no longer supports Internet Explorer.
To browse Academia.edu and the wider internet faster and more securely, please take a few seconds to upgrade your browser .
- We're Hiring!
- Help Center
Statistical Model
- Most Cited Papers
- Most Downloaded Papers
- Newest Papers
- Last »
- Forecasting for Arima Model Follow Following
- Market efficiency Follow Following
- Satellite Data Follow Following
- European Follow Following
- Experimental Evaluation Follow Following
- Evaluation Studies Follow Following
- Randomized Controlled Trial Follow Following
- Construction Industry Follow Following
- Ceramic Technology Follow Following
- Revealed Preference Follow Following
Enter the email address you signed up with and we'll email you a reset link.
- Academia.edu Journals
- We're Hiring!
- Help Center
- Find new research papers in:
- Health Sciences
- Earth Sciences
- Cognitive Science
- Mathematics
- Computer Science
- Academia ©2024
Thank you for visiting nature.com. You are using a browser version with limited support for CSS. To obtain the best experience, we recommend you use a more up to date browser (or turn off compatibility mode in Internet Explorer). In the meantime, to ensure continued support, we are displaying the site without styles and JavaScript.
- View all journals
- Explore content
- About the journal
- Publish with us
- Sign up for alerts
Statistics articles within Scientific Reports
Article 13 September 2024 | Open Access
Effectiveness of non-pharmaceutical interventions for COVID-19 in USA
- , Weihao Wang
- & Wei Zhu
Article 12 September 2024 | Open Access
Analyzing spatio-temporal dynamics of dissolved oxygen for the River Thames using superstatistical methods and machine learning
- , Takuya Boehringer
- & Christian Beck
Passive earth pressure on vertical rigid walls with negative wall friction coupling statically admissible stress field and soft computing
- , Tan Nguyen
- & Tram Bui-Ngoc
Article 06 September 2024 | Open Access
A coordinated adaptive multiscale enhanced spatio-temporal fusion network for multi-lead electrocardiogram arrhythmia detection
- Zicong Yang
- , Aitong Jin
- & Yan Liu
Article 04 September 2024 | Open Access
Modelling the age distribution of longevity leaders
- , László Németh
- & Bálint Vető
Article 29 August 2024 | Open Access
Effective weight optimization strategy for precise deep learning forecasting models using EvoLearn approach
- , Ashima Anand
- & Rajender Parsad
Article 26 August 2024 | Open Access
Quantification of the time-varying epidemic growth rate and of the delays between symptom onset and presenting to healthcare for the mpox epidemic in the UK in 2022
- Robert Hinch
- , Jasmina Panovska-Griffiths
- & Christophe Fraser
Investigating the causal relationship between wealth index and ICT skills: a mediation analysis approach
- Tarikul Islam
- & Nabil Ahmed Uthso
Article 24 August 2024 | Open Access
Statistical analysis of the effect of socio-political factors on individual life satisfaction
- , Isra Hasan
- & Ayman Alzaatreh
Article 23 August 2024 | Open Access
Improving the explainability of autoencoder factors for commodities through forecast-based Shapley values
- Roy Cerqueti
- , Antonio Iovanella
- & Saverio Storani
Article 20 August 2024 | Open Access
Defect detection of printed circuit board assembly based on YOLOv5
- Minghui Shen
- , Yujie Liu
- & Ye Jiang
Breaking the silence: leveraging social interaction data to identify high-risk suicide users online using network analysis and machine learning
- Damien Lekkas
- & Nicholas C. Jacobson
Stochastic image spectroscopy: a discriminative generative approach to hyperspectral image modelling and classification
- Alvaro F. Egaña
- , Alejandro Ehrenfeld
- & Jorge F. Silva
Article 15 August 2024 | Open Access
Data-driven risk analysis of nonlinear factor interactions in road safety using Bayesian networks
- Cinzia Carrodano
Article 13 August 2024 | Open Access
Momentum prediction models of tennis match based on CatBoost regression and random forest algorithms
- Xingchen Lv
- , Dingyu Gu
- & Yanfang li
Article 12 August 2024 | Open Access
Numerical and machine learning modeling of GFRP confined concrete-steel hollow elliptical columns
- Haytham F. Isleem
- , Tang Qiong
- & Ali Jahami
Experimental investigation of the distribution patterns of micro-scratches in abrasive waterjet cutting surface
- & Quan Wen
Article 07 August 2024 | Open Access
PMANet : a time series forecasting model for Chinese stock price prediction
- , Weisi Dai
- & Yunjing Zhao
Article 06 August 2024 | Open Access
Grasshopper platform-assisted design optimization of fujian rural earthen buildings considering low-carbon emissions reduction
- & Yang Ding
Article 03 August 2024 | Open Access
Effects of dietary fish to rapeseed oil ratio on steatosis symptoms in Atlantic salmon ( Salmo salar L) of different sizes
- D. Siciliani
- & Å. Krogdahl
A model-free and distribution-free multi-omics integration approach for detecting novel lung adenocarcinoma genes
- Shaofei Zhao
- & Guifang Fu
Article 01 August 2024 | Open Access
Intrinsic dimension as a multi-scale summary statistics in network modeling
- Iuri Macocco
- , Antonietta Mira
- & Alessandro Laio
A new possibilistic-based clustering method for probability density functions and its application to detecting abnormal elements
- Hung Tran-Nam
- , Thao Nguyen-Trang
- & Ha Che-Ngoc
Article 30 July 2024 | Open Access
A dynamic customer segmentation approach by combining LRFMS and multivariate time series clustering
- Shuhai Wang
- , Linfu Sun
- & Yang Yu
Article 29 July 2024 | Open Access
Field evaluation of a volatile pyrethroid spatial repellent and etofenprox treated clothing for outdoor protection against forest malaria vectors in Cambodia
- Élodie A. Vajda
- , Amanda Ross
- & Neil F. Lobo
Study on crease recovery property of warp-knitted jacquard spacer shoe upper material
- & Shiyu Peng
Article 27 July 2024 | Open Access
Calibration estimation of population total using multi-auxiliary information in the presence of non-response
- , Anant Patel
- & Menakshi Pachori
Simulation-based prior knowledge elicitation for parametric Bayesian models
- Florence Bockting
- , Stefan T. Radev
- & Paul-Christian Bürkner
Article 26 July 2024 | Open Access
Modelling Salmonella Typhi in high-density urban Blantyre neighbourhood, Malawi, using point pattern methods
- Jessie J. Khaki
- , James E. Meiring
- & Emanuele Giorgi
Exogenous variable driven deep learning models for improved price forecasting of TOP crops in India
- G. H. Harish Nayak
- , Md Wasi Alam
- & Chandan Kumar Deb
Generalization of cut-in pre-crash scenarios for autonomous vehicles based on accident data
- , Xinyu Zhu
- & Chang Xu
Article 19 July 2024 | Open Access
Automated PD-L1 status prediction in lung cancer with multi-modal PET/CT fusion
- Ronrick Da-ano
- , Gustavo Andrade-Miranda
- & Catherine Cheze Le Rest
Article 17 July 2024 | Open Access
Optimizing decision-making with aggregation operators for generalized intuitionistic fuzzy sets and their applications in the tech industry
- Muhammad Wasim
- , Awais Yousaf
- & Hamiden Abd El-Wahed Khalifa
Article 15 July 2024 | Open Access
Putting ICAP to the test: how technology-enhanced learning activities are related to cognitive and affective-motivational learning outcomes in higher education
- Christina Wekerle
- , Martin Daumiller
- & Ingo Kollar
The impact of national savings on economic development: a focused study on the ten poorest countries in Sub-Saharan Africa
Article 13 July 2024 | Open Access
Regularized ensemble learning for prediction and risk factors assessment of students at risk in the post-COVID era
- Zardad Khan
- , Amjad Ali
- & Saeed Aldahmani
Article 12 July 2024 | Open Access
Eigen-entropy based time series signatures to support multivariate time series classification
- Abhidnya Patharkar
- , Jiajing Huang
- & Naomi Gades
Article 11 July 2024 | Open Access
Exploring usage pattern variation of free-floating bike-sharing from a night travel perspective
- , Xianke Han
- & Lili Li
Early mutational signatures and transmissibility of SARS-CoV-2 Gamma and Lambda variants in Chile
- Karen Y. Oróstica
- , Sebastian B. Mohr
- & Seba Contreras
Article 10 July 2024 | Open Access
Optimizing the location of vaccination sites to stop a zoonotic epidemic
- Ricardo Castillo-Neyra
- , Sherrie Xie
- & Michael Z. Levy
Article 08 July 2024 | Open Access
Integrating socio-psychological factors in the SEIR model optimized by a genetic algorithm for COVID-19 trend analysis
- Haonan Wang
- , Danhong Wu
- & Junhui Zhang
Article 05 July 2024 | Open Access

Research on bearing fault diagnosis based on improved genetic algorithm and BP neural network
- Zenghua Chen
- , Lingjian Zhu
- & Gang Xiong
Article 04 July 2024 | Open Access
Employees’ pro-environmental behavior in an organization: a case study in the UAE
- Nadin Alherimi
- , Zeki Marva
- & Ayman Alzaaterh
Article 03 July 2024 | Open Access
The predictive capability of several anthropometric indices for identifying the risk of metabolic syndrome and its components among industrial workers
- Ekaterina D. Konstantinova
- , Tatiana A. Maslakova
- & Svetlana Yu. Ogorodnikova
Article 02 July 2024 | Open Access
A bayesian spatio-temporal dynamic analysis of food security in Africa
- Adusei Bofa
- & Temesgen Zewotir
Research on the influencing factors of promoting flipped classroom teaching based on the integrated UTAUT model and learning engagement theory
- & Wang He
Article 28 June 2024 | Open Access
Peak response regularization for localization
- , Jinzhen Yao
- & Qintao Hu
Article 25 June 2024 | Open Access
Prediction and reliability analysis of shear strength of RC deep beams
- Khaled Megahed
Multistage time-to-event models improve survival inference by partitioning mortality processes of tracked organisms
- Suresh A. Sethi
- , Alex L. Koeberle
- & Kenneth Duren
Article 24 June 2024 | Open Access
Summarizing physical performance in professional soccer: development of a new composite index
- José M. Oliva-Lozano
- , Mattia Cefis
- & Ricardo Resta
Browse broader subjects
- Mathematics and computing
Quick links
- Explore articles by subject
- Guide to authors
- Editorial policies

Advertisement
Species distribution modeling: a statistical review with focus in spatio-temporal issues
- Review Paper
- Published: 19 April 2018
- Volume 32 , pages 3227–3244, ( 2018 )
Cite this article
- Joaquín Martínez-Minaya ORCID: orcid.org/0000-0002-1016-8734 1 ,
- Michela Cameletti 2 ,
- David Conesa 1 &
- Maria Grazia Pennino 3
4575 Accesses
87 Citations
Explore all metrics
The use of complex statistical models has recently increased substantially in the context of species distribution behavior. This complexity has made the inferential and predictive processes challenging to perform. The Bayesian approach has become a good option to deal with these models due to the ease with which prior information can be incorporated along with the fact that it provides a more realistic and accurate estimation of uncertainty. In this paper, we first review the sources of information and different approaches (frequentist and Bayesian) to model the distribution of a species. We also discuss the Integrated Nested Laplace approximation as a tool with which to obtain marginal posterior distributions of the parameters involved in these models. We finally discuss some important statistical issues that arise when researchers use species data: the presence of a temporal effect (presenting different spatial and spatio-temporal structures), preferential sampling, spatial misalignment, non-stationarity, imperfect detection, and the excess of zeros.
This is a preview of subscription content, log in via an institution to check access.
Access this article
Subscribe and save.
- Get 10 units per month
- Download Article/Chapter or eBook
- 1 Unit = 1 Article or 1 Chapter
- Cancel anytime
Price includes VAT (Russian Federation)
Instant access to the full article PDF.
Rent this article via DeepDyve
Institutional subscriptions
Similar content being viewed by others
Hierarchical species distribution models.
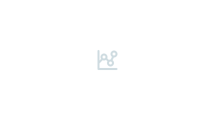
Species-Distribution Modeling: Advantages and Limitations of Its Application. 1. General Approaches
Species-distribution modeling: advantages and limitations of its application. 2. maxent.
Agarwal DK, Gelfand AE, Citron-Pousty S (2002) Zero-inflated models with application to spatial count data. Environ Ecol Stat 9(4):341–355
Google Scholar
Aitchison J (1955) On the distribution of a positive random variable having a discrete probability mass at the origin. J Am Stat Assoc 50(271):901–908
Aizpurua O, Paquet JY, Brotons L, Titeux N (2015) Optimising long-term monitoring projects for species distribution modelling: how atlas data may help. Ecography 38(1):29–40
Anatolyev S, Kosenok G (2005) An alternative to maximum likelihood based on spacings. Econom Theory 21(2):472–476
Andreon S, Weaver B (2015) Bayesian methods for the physical sciences: learning from examples in astronomy and physics. Springer series in astrostatistics, vol 4. Springer, Berlin
Araújo MB, Pearson RG, Thuiller W, Erhard M (2005) Validation of species-climate impact models under climate change. Glob Change Biol 11(9):1504–1513
Baio G (2012) Bayesian methods in health economics. CRC Chapman and Hall, Boca Raton
Bakka H, Vanhatalo J, Illian J, Simpson D, Rue H (2016) Accounting for physical barriers in species distribution modeling with non-stationary spatial random effects. arXiv:1608.03787
Balderama E, Gardner B, Reich BJ (2016) A spatial-temporal double-hurdle model for extremely over-dispersed avian count data. Spat Stat 18:263–275
Banerjee S, Carlin BP, Gelfand AE (2014) Hierarchical modeling and analysis for spatial data. CRC, Boca Raton
Barber X, Conesa D, Lladosa S, López-Quílez A (2016) Modelling the presence of disease under spatial misalignment using Bayesian latent Gaussian models. Geospat Health 11:415
Barber X, Conesa D, López-Quílez A, Mayoral A, Morales J, Barber A (2017) Bayesian hierarchical models for analysing the spatial distribution of bioclimatic indices. SORT-Stat Oper Res Trans 1(2):277–296
Barbet-Massin M, Jiguet F, Albert CH, Thuiller W (2012) Selecting pseudo-absences for species distribution models: how, where and how many? Methods Ecol Evol 3(2):327–338
Bartlett MS (1937) Properties of sufficiency and statistical tests. Proc R Soc Lond A 160(901):268–282
Beale CM, Lennon JJ, Yearsley JM, Brewer MJ, Elston DA (2010) Regression analysis of spatial data. Ecol Lett 13(2):246–264
Berry DA, Stangl D (1999) Bayesian biostatistics. Marcel Dekker, New York City
Blangiardo M, Cameletti M (2015) Spatial and spatio-temporal Bayesian models with R-INLA . Wiley, Hoboken
Blangiardo M, Cameletti M, Baio G, Rue H (2013) Spatial and spatio-temporal models with R-INLA . Spat Spatio-temporal Epidemiol 7:39–55
Bowman K, Shenton L (2006) Estimation: method of moments. In: Kotz S, Read CB, Balakrishnan N, Vidakovic B, Johnson NL (eds) Encyclopedia of statistical sciences
Brezger A, Kneib T, Lang S (2003) BayesX: Analysing Bayesian structured additive regression models. Tech. rep., Discussion paper//Sonderforschungsbereich 386 der Ludwig-Maximilians-Universität München
Brooks S, Gelman A, Jones GL, Meng XL (2011) Handbook of markov chain monte carlo. CRC Press, Boca Raton
Brown P (2015) Model-based geostatistics the easy way. J Stat Softw 63:1–24
Brown CJ, O’connor MI, Poloczanska ES, Schoeman DS, Buckley LB, Burrows MT, Duarte CM, Halpern BS, Pandolfi JM, Parmesan C, Richardson AJ (2016) Ecological and methodological drivers of species distribution and phenology responses to climate change. Glob Change Biol 22:1548–1560
Brunsdon C, Fotheringham S, Charlton M (1998) Geographically weighted regression. J R Stat Soc Ser D (The Statistician) 47(3):431–443
Brynjarsdóttir J, Stefánsson G (2004) Analysis of cod catch data from Icelandic groundfish surveys using generalized linear models. Fish Res 70(2):195–208
Busby JR (1991) BIOCLIM – A bioclimate analysis and prediction system. In: Margules CR, Austin MP (eds) Nature conservation: cost effective biological surveys and data analysis. CSIRO, Melbourne, pp 64–68
Cameletti M, Ignaccolo R, Bande S (2011) Comparing spatio-temporal models for particulate matter in Piemonte. Environmetrics 22(8):985–996
Cameletti M, Lindgren F, Simpson D, Rue H (2013) Spatio-temporal modeling of particulate matter concentration through the SPDE approach. AStA Adv Stat Anal 97(2):109–131
Cameron CA, Trivedi PK (1998) Regression analysis count data. Cambridge University Press, New York
Carpenter G, Gillison A, Winter J (1993) DOMAIN: a flexible modelling procedure for mapping potential distributions of plants and animals. Biodivers Conserv 2(6):667–680
Carroll RJ, Ruppert D, Stefanski LA, Crainiceanu LA (2016) Measurement error in nonlinear models: a modern perspective, 2nd edn. Chapman and Hall/CRC, Boca Raton
Clark J, Gelfand A (2006) Hierarchical modeling for the environmental sciences. Statistical methods and applications. Oxford University Press, New York
Clark JS, Gelfand AE, Woodall CW, Zhu K (2014) More than the sum of the parts: forest climate response from joint species distribution models. Ecol Appl 24(5):990–999
Cosandey-Godin A, Krainski ET, Worm B, Flemming JM (2015) Applying Bayesian spatio-temporal models to fisheries bycatch in the Canadian Arctic. Can J Fish Aquat Sci 72(2):186–197
Cox DR, Reid N (2004) A note on pseudolikelihood constructed from marginal densities. Biometrika 91(3):729–737
Cressie N, Wikle CK (2011) Statistics for spatio-temporal data. Wiley, Hoboken
Daly C (2006) Guidelines for assessing the suitability of spatial climate data sets. Int J Climatol 26(6):707–721
Danks F, Klein D (2002) Using GIS to predict potential wildlife habitat: a case study of muskoxen in northern Alaska. Int J Remote Sens 23(21):4611–4632
Dettmers R, Buehler DA, Bartlett JG, Klaus NA (1999) Influence of point count length and repeated visits on habitat model performance. J Wildl Manag 63:815–823
Diggle PJ (2013) Statistical analysis of spatial and spatio-temporal point patterns. CRC, Boca Raton
Diggle PJ, Ribeiro PJ (2007) Model-based geostatistics. Springer, Berlin
Diggle PJ, Menezes R, Su TL (2010) Geostatistical inference under preferential sampling. J R Stat Soc Ser C (Appl Stat) 59(2):191–232
Dodd CK Jr, Dorazio RM (2004) Using counts to simultaneously estimate abundance and detection probabilities in a salamander community. Herpetologica 60(4):468–478
Dorazio RM, Royle JA, Söderström B, Glimskär A (2006) Estimating species richness and accumulation by modeling species occurrence and detectability. Ecology 87(4):842–854
Elith J, Leathwick JR (2009) Species distribution models: ecological explanation and prediction across space and time. Annu Rev Ecol Evol Syst 40:677–697
Fatima SH, Atif S, Rasheed SB, Zaidi F, Hussain E (2016) Species distribution modelling of Aedes aegypti in two dengue-endemic regions of Pakistan. Trop Med Int Health 21:427–436
Ferrari SLP, Cribari-Neto F (2004) Beta regression for modelling rates and proportions. J Appl Stat 31(7):799–815
Finley AO (2011) Comparing spatially-varying coefficients models for analysis of ecological data with non-stationary and anisotropic residual dependence. Methods Ecol Evol 2(2):143–154
Fitzpatrick MC, Weltzin JF, Sanders NJ, Dunn RR (2007) The biogeography of prediction error: why does the introduced range of the fire ant over-predict its native range? Glob Ecol Biogeogr 16(1):24–33
Fortin MJ, Dale MR (2005) Spatial analysis: a guide for ecologists. Cambridge University Press, Cambridge
Foster SD, Shimadzu H, Darnell R (2012) Uncertainty in spatially predicted covariates: is it ignorable? J Roy Stat Soc Ser C (Appl Stat) 61(4):637–652
Franklin J (2010) Mapping species distributions: spatial inference and prediction. Cambridge University Press, Cambridge
Gaudard M, Karson M, Linder E, Sinha D (1999) Bayesian spatial prediction. Environ Ecol Stat 6(2):147–171
Gelfand AE, Smith AF (1990) Sampling-based approaches to calculating marginal densities. J Am Stat Assoc 85(410):398–409
Gelfand AE, Kim HJ, Sirmans CF, Banerjee S (2003) Spatial modeling with spatially varying coefficient processes. J Am Stat Assoc 98(462):387–396
Gelfand AE, Silander JA, Wu S, Latimer A, Lewis PO, Rebelo AG, Holder M (2006) Explaining species distribution patterns through hierarchical modeling. Bayesian Anal 1(1):41–92
Gelfand AE, Diggle PJ, Fuentes M, Guttorp P (2010) Handbook of spatial statistics. Chapman & Hall, Boca Raton
Gelman A, Carlin JB, Stern HS, Rubin DB (2014) Bayesian data analysis, vol 2. Chapman & Hall/CRC, Boca Raton
Gitzen RA (2012) Design and analysis of long-term ecological monitoring studies. Cambridge University Press, Cambridge
Goetz SJ, Sun M, Zolkos S, Hansen A, Dubayah R (2014) The relative importance of climate and vegetation properties on patterns of North American breeding bird species richness. Environ Res Lett 9(3):034013
Golding N, Purse BV (2016) Fast and flexible Bayesian species distribution modelling using Gaussian processes. Methods Ecol Evol 7:598–608
Gómez-Rubio V, Rue H (2017) Markov chain monte carlo with the integrated nested Laplace approximation. arXiv:1702.07007
Gómez-Rubio V, Bivand RS, Rue H (2014) Spatial models using Laplace approximation methods. In: Fischer MM, Nijkamp P (eds) Handbook of regional science. Springer, Berlin, pp 1401–1417
González-Warleta M, Lladosa S, Castro-Hermida JA, Martínez-Ibeas AM, Conesa D, Muñoz F, López-Quílez A, Manga-González Y, Mezo M (2013) Bovine paramphistomosis in Galicia (Spain): prevalence, intensity, aetiology and geospatial distribution of the infection. Vet Parasitol 191(3):252–263
Gosoniu L, Vounatsou P, Sogoba N, Smith T (2006) Bayesian modelling of geostatistical malaria risk data. Geospat Health 1(1):127–139
CAS Google Scholar
Gotelli NJ, Anderson MJ, Arita HT, Chao A, Colwell RK, Connolly SR, Currie DJ, Dunn RR, Graves GR, Green JL (2009) Patterns and causes of species richness: a general simulation model for macroecology. Ecol Lett 12(9):873–886
Griffith DA (2008) Spatial-filtering-based contributions to a critique of geographically weighted regression (GWR). Environ Plan A 40(11):2751–2769
Gu W, Swihart RK (2004) Absent or undetected? effects of non-detection of species occurrence on wildlife-habitat models. Biol Conserv 116(2):195–203
Guisan A, Thuiller W (2005) Predicting species distribution: offering more than simple habitat models. Ecol Lett 8(9):993–1009
Guisan A, Edwards TC, Hastie T (2002) Generalized linear and generalized additive models in studies of species distributions: setting the scene. Ecol Model 157(2):89–100
Handcock MS, Stein ML (1993) A Bayesian analysis of kriging. Technometrics 35(4):403–410
Hansen LP (1982) Large sample properties of generalized method of moments estimators. Econom J Econom Soc 50(4):1029–1054
He KS, Bradley BA, Cord AF, Rocchini D, Tuanmu MN, Schmidtlein S, Turner W, Wegmann M, Pettorelli N (2015) Will remote sensing shape the next generation of species distribution models? Remote Sens Ecol Conserv 1(1):4–18
Hefley TJ, Hooten MB (2016) Hierarchical species distribution models. Curr Landsc Ecol Rep 1(2):87–97
Hefley TJ, Broms KM, Brost BM, Buderman FE, Kay SL, Scharf HR, Tipton JR, Williams PJ, Hooten MB (2017a) The basis function approach for modeling autocorrelation in ecological data. Ecology 98(3):632–646
Hefley TJ, Hooten MB, Hanks EM, Russell RE, Walsh DP (2017b) Dynamic spatio-temporal models for spatial data. Spat Stat 20:206–220
Hendricks SA, Clee PRS, Harrigan RJ, Pollinger JP, Freedman AH, Callas R, Figura PJ, Wayne RK (2016) Re-defining historical geographic range in species with sparse records: implications for the Mexican wolf reintroduction program. Biol Conserv 194:48–57
Hengl T, Heuvelink GB, Tadić MP, Pebesma EJ (2012) Spatio-temporal prediction of daily temperatures using time-series of MODIS LST images. Theoret Appl Climatol 107(1–2):265–277
Hijmans RJ, Cameron SE, Parra JL, Jones PG, Jarvis A (2005) Very high resolution interpolated climate surfaces for global land areas. Int J Climatol 25(15):1965–1978
Hoeting JA, Leecaster M, Bowden D (2000) An improved model for spatially correlated binary responses. J Agric Biol Environ Stat 5:102–114
Holloway P, Miller JA (2015) Exploring spatial scale, autocorrelation and nonstationarity of bird species richness patterns. ISPRS Int J Geo-Inf 4(2):783–798
Hooten MB, Wikle CK (2008) A hierarchical Bayesian non-linear spatio-temporal model for the spread of invasive species with application to the Eurasian Collared-Dove. Environ Ecol Stat 15(1):59–70
Hooten MB, Wikle CK, Dorazio RM, Royle JA (2007) Hierarchical spatiotemporal matrix models for characterizing invasions. Biometrics 63(2):558–567
Hui FK (2017) Model-based simultaneous clustering and ordination of multivariate abundance data in ecology. Comput Stat Data Anal 105:1–10
Illian JB, Sørbye SH, Rue H (2012) A toolbox for fitting complex spatial point process models using integrated nested Laplace approximation (INLA). Ann Appl Stat 6(4):1499–1530
Ingebrigtsen R, Lindgren F, Steinsland I (2014) Spatial models with explanatory variables in the dependence structure. Spat Stat 8:20–38
Iturbide M, Bedia J, Herrera S, del Hierro O, Pinto M, Gutiérrez JM (2015) A framework for species distribution modelling with improved pseudo-absence generation. Ecol Model 312:166–174
Iverson LR, Schwartz MW, Prasad AM (2004) How fast and far might tree species migrate in the eastern united states due to climate change? Glob Ecol Biogeogr 13(3):209–219
Jackman S (2009) Bayesian analysis for the social sciences. Wiley, Hoboken
Jiménez-Valverde A, Lobo JM (2007) Determinants of local spider ( Araneidae and Thomisidae ) species richness on a regional scale: climate and altitude vs. habitat structure. Ecol Entomol 32(1):113–122
Johnson DS, Conn PB, Hooten MB, Ray JC, Pond BA (2013) Spatial occupancy models for large data sets. Ecology 94(4):801–808
Jona Lasinio G, Mastrantonio G, Pollice A (2013) Discussing the “big n problem”. Stat Methods Appl 22(1):97–112
Joseph LN, Field SA, Wilcox C, Possingham HP (2006) Presence-absence versus abundance data for monitoring threatened species. Conserv Biol 20(6):1679–1687
Juan P, Díaz-Avalos C, Mejía-Domínguez NR, Mateu J (2017) Hierarchical spatial modeling of the presence of Chagas disease insect vectors in Argentina. A comparative approach. Stoch Env Res Risk Assess 31(2):461–479
Karagiannis-Voules DA, Scholte RG, Guimarães LH, Utzinger J, Vounatsou P (2013) Bayesian geostatistical modeling of leishmaniasis incidence in Brazil. PLOS Negl Trop Dis 7(5):e2213
Kneib T, Müller J, Hothorn T (2008) Spatial smoothing techniques for the assessment of habitat suitability. Environ Ecol Stat 15(3):343–364
Kozak KH, Graham CH, Wiens JJ (2008) Integrating GIS-based environmental data into evolutionary biology. Trends Ecol Evol 23(3):141–148
Krainski ET, Lindgren F, Simpson D, Rue H (2017) The R-INLA tutorial: SPDE models. http://www.math.ntnu.no/inla/r-inla.org/tutorials/spde/spde-tutorial.pdf . Accessed 18 Apr 2018
Latimer AM, Wu S, Gelfand AE, Silander JA (2006) Building statistical models to analyze species distributions. Ecol Appl 16(1):33–50
Le Cam L (1990) Maximum likelihood: an introduction. Int Stat Rev/Rev Int Stat 58(2):153–171
Leathwick J, Rowe D, Richardson J, Elith J, Hastie T (2005) Using multivariate adaptive regression splines to predict the distributions of New Zealand’s freshwater diadromous fish. Freshw Biol 50(12):2034–2052
Lee D (2013) CARBayes: an R package for Bayesian spatial modeling with conditional autoregressive priors. J Stat Softw 55(13):1–24
Lindgren F, Rue H (2015a) On the secondorder random walk model for irregular locations. Scand J Stat 35(4):691–700
Lindgren F, Rue H (2015b) Bayesian spatial modelling with R-INLA . J Stat Softw 63(19):1–25
Lindgren F, Rue H, Lindström J (2011) An explicit link between Gaussian fields and Gaussian Markov random fields: the stochastic partial differential equation approach. J R Stat Soc Ser B (Stat Methodol) 73(4):423–498
Liu C, Wan R, Jiao Y, Reid KB (2017) Exploring non-stationary and scale-dependent relationships between walleye ( Sander vitreus ) distribution and habitat variables in lake erie. Mar Freshw Res 68(2):270–281
Luo M, Opaluch JJ (2011) Analyze the risks of biological invasion. Stoch Env Res Risk Assess 25(3):377–388
Lunn DJ, Thomas A, Best N, Spiegelhalter D (2000) WinBUGS-a Bayesian modelling framework: concepts, structure, and extensibility. Stat Comput 10(4):325–337
Lunn D, Spiegelhalter D, Thomas A, Best N (2009) The BUGS project: evolution, critique and future directions. Stat Med 28(25):3049–3067
MacKenzie DI, Nichols JD, Lachman GB, Droege S, Andrew Royle J, Langtimm CA (2002) Estimating site occupancy rates when detection probabilities are less than one. Ecology 83(8):2248–2255
Mallick BK, Gold D, Baladandayuthapani V (2009) Bayesian analysis of gene expression data. Wiley, Hoboken
Martinez-Meyer E, Peterson AT, Servín JI, Kiff LF (2006) Ecological niche modelling and prioritizing areas for species reintroductions. Oryx 40(4):411–418
Martínez-Bello D, López-Quílez A, Prieto AT (2017) Spatiotemporal modeling of relative risk of dengue disease in Colombia. Stoch Environ Res Risk Assess. https://doi.org/10.1007/s00477-017-1461-5
Martínez-Minaya J, Conesa D, López-Quílez A, Vicent A (2018) Spatial and climatic factors associated with the geographical distribution of citrus black spot disease in South Africa. A Bayesian latent Gaussian model approach. Eur J Plant Pathol ( in press )
Martino S, Akerkar R, Rue H (2011) Approximate Bayesian inference for survival models. Scand J Stat 38(3):514–528
Martins TG, Simpson D, Lindgren F, Rue H (2013) Bayesian computing with inla : new features. Comput Stat Data Anal 67:68–83
McCarthy MA (2007) Bayesian methods for ecology. Wiley, Hoboken
McInerny GJ, Purves DW (2011) Fine-scale environmental variation in species distribution modelling: regression dilution, latent variables and neighbourly advice. Methods Ecol Evol 2(3):248–257
Meehan TD, Michel NL, Rue H (2017) Estimating animal abundance with N-mixture models using the R-INLA package for R . arXiv:1705.01581
Meentemeyer RK, Cunniffe NJ, Cook AR, Filipe JA, Hunter RD, Rizzo DM, Gilligan CA (2011) Epidemiological modeling of invasion in heterogeneous landscapes: spread of sudden oak death in California (1990–2030). Ecosphere 2(2):1–24
Miller JA (2012) Species distribution models. Prog Phys Geogr 36(5):681–692
Monnahan CC, Thorson JT, Branch TA (2017) Faster estimation of Bayesian models in ecology using Hamiltonian Monte Carlo. Methods Ecol Evol 8(3):339–348
Muñoz F, Pennino MG, Conesa D, López-Quílez A, Bellido JM (2013) Estimation and prediction of the spatial occurrence of fish species using Bayesian latent Gaussian models. Stoch Environ Res Risk Assess 27(5):1171–1180
Muff S, Riebler A, Held L, Rue H, Saner P (2015) Bayesian analysis of measurement error models using integrated nested Laplace approximations. J Roy Stat Soc Ser C (Appl Stat) 64(2):231–252
Mullahy J (1986) Specification and testing of some modified count data models. J Econom 33(3):341–365
Neelon B, Ghosh P, Loebs PF (2013) A spatial Poisson hurdle model for exploring geographic variation in emergency department visits. J R Stat Soc Ser A 176(2):389–413
Neri FM, Cook AR, Gibson GJ, Gottwald TR, Gilligan CA (2014) Bayesian analysis for inference of an emerging epidemic: citrus canker in urban landscapes. PLOS Comput Biol 10(4):e1003587
New M, Lister D, Hulme M, Makin I (2002) A high-resolution data set of surface climate over global land areas. Clim Res 21(1):1–25
Nichols JD, Hines JE, Sauer JR, Fallon FW, Fallon JE, Heglund PJ (2000) A double-observer approach for estimating detection probability and abundance from point counts. Auk 117(2):393–408
Nielsen SE, Johnson CJ, Heard DC, Boyce MS (2005) Can models of presence-absence be used to scale abundance? Two case studies considering extremes in life history. Ecography 28(2):197–208
Otis DL, Burnham KP, White GC, Anderson DR (1978) Statistical inference from capture data on closed animal populations. Wildl Monogr 62:3–135
Paradinas I, Conesa D, Pennino MG, Muñoz F, Fernández AM, López-Quílez A, Bellido JM (2015) Bayesian spatio-temporal approach to identifying fish nurseries by validating persistence areas. Mar Ecol Prog Ser 528:245–255
Paradinas I, Marín M, Pennino MG, López-Quílez A, Conesa D, Barreda D, Gonzalez M, Bellido JM (2016) Identifying the best fishing-suitable areas under the new European discard ban. ICES J Mar Sci J Cons 73(10):2479–2487
Paradinas I, Conesa D, López-Quílez A, Bellido JM (2017a) Spatio-Temporal model structures with shared components for semi-continuous species distribution modelling. Spat Stat 22:434–450
Paradinas I, Pennino MG, López-Quílez A, Marín M, Bellido JM, Conesa D (2017b) Modelling spatially sampled proportion processes. REVSTATStat J 16(1):71–86
Park YS, Céréghino R, Compin A, Lek S (2003) Applications of artificial neural networks for patterning and predicting aquatic insect species richness in running waters. Ecol Model 160(3):265–280
Parviainen M, Luoto M, Ryttäri T, Heikkinen RK (2008) Modelling the occurrence of threatened plant species in taiga landscapes: methodological and ecological perspectives. J Biogeogr 35(10):1888–1905
Pennino MG, Muñoz F, Conesa D, López-Quílez A, Bellido JM (2013) Modeling sensitive elasmobranch habitats. J Sea Res 83:209–218
Pennino MG, Muñoz F, Conesa D, López-Quílez A, Bellido JM (2014) Bayesian spatio-temporal discard model in a demersal trawl fishery. J Sea Res 90:44–53
Pennino MG, Conesa D, López-Quílez A, Muñoz F, Fernández A, Bellido JM (2016) Fishery-dependent and-independent data lead to consistent estimations of essential habitats. ICES J Mar Sci J Cons 73(9):2302–2310
Pennino MG, Mérigot B, Fonseca VP, Monni V, Rotta A (2017) Habitat modeling for cetacean management: spatial distribution in the southern Pelagos Sanctuary (Mediterranean sea). Deep Sea Res Part II Top Stud Oceanogr 141:203–211
Pennino MG, Paradinas I, Illian JB, Muñoz F, Bellido JM, López-Quílez A, Conesa D (2018) Accounting for preferential sampling in species distribution models ( submitted )
Peterson AT, Sánchez-Cordero V, Beard CB, Ramsey JM (2002) Ecologic niche modeling and potential reservoirs for chagas disease, Mexico. Emerg Infect Dis 8(7):662–667
Phillips SJ, Anderson RP, Schapire RE (2006) Maximum entropy modeling of species geographic distributions. Ecol Model 190(3):231–259
Plummer M (2016) Rjags : Bayesian graphical models using MCMC. R Software Package for Graphical Models. URL https://cran.r-project.org/web/packages/rjags/index.html
Pollock LJ, Tingley R, Morris WK, Golding N, O’Hara RB, Parris KM, Vesk PA, McCarthy MA (2014) Understanding co-occurrence by modelling species simultaneously with a joint species distribution model (JSDM). Methods Ecol Evol 5(5):397–406
Quiroz ZC, Prates MO, Rue H (2015) A Bayesian approach to estimate the biomass of anchovies off the coast of Perú. Biometrics 71(1):208–217
Rachev ST, Hsu JS, Bagasheva BS, Fabozzi FJ (2008) Bayesian methods in finance, vol 153. Wiley, Hoboken
Risser MD (2016) Review: nonstationary spatial modeling, with emphasis on process convolution and covariate-driven approaches. arXiv:1610.02447
Robert C, Casella G (2011) A short history of Markov Chain Monte Carlo: subjective recollections from incomplete data. Stat Sci 26(1):102–115
Rodríguez de Rivera O, López-Quílez A (2017) Development and comparison of species distribution models for forest inventories. ISPRS Int J Geo-Inf 6(6):176
Roos NC, Carvalho AR, Lopes PF, Pennino MG (2015) Modeling sensitive parrotfish (Labridae: Scarini) habitats along the Brazilian coast. Mar Environ Res 110:92–100
Royle JA (2004) N-mixture models for estimating population size from spatially replicated counts. Biometrics 60(1):108–115
Royle JA, Nichols JD (2003) Estimating abundance from repeated presence-absence data or point counts. Ecology 84(3):777–790
Rue H, Held L (2005) Gaussian Markov random fields: theory and applications. Chapman & Hall, Boca Raton
Rue H, Martino S, Chopin N (2009) Approximate Bayesian inference for latent Gaussian models by using integrated nested Laplace approximations. J R Stat Soc Ser B (Stat Methodol) 71(2):319–392
Rue H, Riebler A, Sørbye SH, Illian JB, Simpson DP, Lindgren FK (2017) Bayesian computing with INLA : a review. Annu Rev Stat Appl 4:395–421
Rufener MC, Kinas PG, Nóbrega MF, EL Oliveira J (2017) Bayesian spatial predictive models for data-poor fisheries. Ecol Model 348:125–134
Ruiz-Cárdenas R, Krainski ET, Rue H (2012) Direct fitting of dynamic models using integrated nested Laplace approximations- INLA . Comput Stat Data Anal 56(6):1808–1828
Sbrocco EJ, Barber PH (2013) MARSPEC: ocean climate layers for marine spatial ecology. Ecology 94(4):979–979
Schrödle B, Held L, Riebler A, Danuser J (2011) Using integrated nested Laplace approximations for the evaluation of veterinary surveillance data from Switzerland: a case-study. J Roy Stat Soc Ser C (Appl Stat) 60(2):261–279
Sadykova D, Scott BE, De Dominicis M, Wakelin SL, Sadykov A, Wolf J (2017) Bayesian joint models with INLA exploring marine mobile predatorprey and competitor species habitat overlap. Ecol Evol 7(14):5212–5226
Shapiro A (2000) On the asymptotics of constrained local M-estimators. Ann Stat 28(3):948–960
Stan Development Team (2017) Stan Modeling Language Users Guide and Reference Manual, Version 2.17.0. http://mc-stan.org . Accessed 18 Apr 2018
Stefánsson G (1996) Analysis of groundfish survey abundance data: combining the GLM and delta approaches. ICES J Mar Sci 53(3):577–588
Stein M (1999) Interpolation of spatial data. Some theory for kriging. Springer, Berlin
Stein A, Kocks C, Zadoks J, Frinking H, Ruissen M, Myers D (1994) A geostatistical analysis of the spatio-temporal development of downy mildew epidemics in cabbage. Phytopathology 84(10):1227–1238
Stoklosa J, Daly C, Foster SD, Ashcroft MB, Warton DI (2015) A climate of uncertainty: accounting for error in climate variables for species distribution models. Methods Ecol Evol 6(4):412–423
Taylor-Rodríguez D, Kaufeld K, Schliep EM, Clark JS, Gelfand AE (2017) Joint species distribution modeling: dimension reduction using Dirichlet processes. Bayesian Anal 12(4):939–967
Václavík T, Meentemeyer RK (2009) Invasive species distribution modeling (iSDM): are absence data and dispersal constraints needed to predict actual distributions? Ecol Model 220(23):3248–3258
Ver Hoef JM, Jansen JK (2007) Space-time zero-inflated count models of Harbor seals. Environmetrics 18(7):697–712
Vieilledent G, Latimer A, Gelfand A, Merow C, Wilson A, Mortier F, Silander Jr J (2014) hSDM: hierarchical Bayesian species distribution models. R package version 1
White SM, Bullock JM, Hooftman DA, Chapman DS (2017) Modelling the spread and control of Xylella fastidiosa in the early stages of invasion in Apulia, Italy. Biol Invasions 19:1825–1837
Wikle CK (2003) Hierarchical Bayesian models for predicting the spread of ecological processes. Ecology 84(6):1382–1394
Wikle CK, Hooten MB (2010) A general science-based framework for dynamical spatio-temporal models. Test 19(3):417–451
Williams PJ, Hooten MB, Womble JN, Esslinger GG, Bower MR, Hefley TJ (2017) An integrated data model to estimate spatiotemporal occupancy, abundance, and colonization dynamics. Ecology 98(2):328–336
Windle MJS, Rose GA, Devillers R, Fortin MJ (2010) Exploring spatial non-stationarity of fisheries survey data using geographically weighted regression (GWR): an example from the Northwest Atlantic. ICES J Mar Sci 67(1):145
Yau KK, Wang K, Lee AH (2003) Zero-inflated negative binomial mixed regression modeling of over-dispersed count data with extra zeros. Biom J 45(4):437–452
Yuan Y, Bachl F, Lindgren F, Brochers D, Illian J, Buckland S, Rue H, Gerrodette T (2016) Point process models for spatio-temporal distance sampling data. arXiv:1604.06013
Zhang W (2007) Supervised neural network recognition of habitat zones of rice invertebrates. Stoch Environ Res Risk Assess 21(6):729–735
Zhang W, Zhong X, Liu G (2008) Recognizing spatial distribution patterns of grassland insects: neural network approaches. Stoch Environ Res Risk Assess 22(2):207–216
Download references
Acknowledgements
JM-M would like to thank Generalitat Valenciana for support via VALi+d grant ACIF/2016/455, while DC would like to thank the Ministerio de Educación y Ciencia (Spain) for financial support (jointly financed by the European Regional Development Fund) via Research Grant MTM2016-77501-P. MC has been supported by the PRIN EphaStat Project (Project No. 20154X8K23, https://sites.google.com/site/ephastat/ ) provided by the Italian Ministry for Education, University and Research. We would also like to thank Facundo Muñoz for his detailed and careful reading of our paper. All his comments have helped us in identifying areas where clarification/changes/additional details were needed.
Author information
Authors and affiliations.
Departamento de Estadística e Investigación Operativa, Universidad de Valencia, C/ Dr. Moliner 50, 46100, Burjassot, Valencia, Spain
Joaquín Martínez-Minaya & David Conesa
Department of Management, Economics and Quantitative Methods, University of Bergamo, Bergamo, Italy
Michela Cameletti
Centro Oceanográfico de Murcia, Instituto Español de Oceanografía, C/ Varadero 1, 30740, San Pedro del Pinatar, Murcia, Spain
Maria Grazia Pennino
You can also search for this author in PubMed Google Scholar
Corresponding author
Correspondence to Joaquín Martínez-Minaya .
Rights and permissions
Reprints and permissions
About this article
Martínez-Minaya, J., Cameletti, M., Conesa, D. et al. Species distribution modeling: a statistical review with focus in spatio-temporal issues. Stoch Environ Res Risk Assess 32 , 3227–3244 (2018). https://doi.org/10.1007/s00477-018-1548-7
Download citation
Published : 19 April 2018
Issue Date : November 2018
DOI : https://doi.org/10.1007/s00477-018-1548-7
Share this article
Anyone you share the following link with will be able to read this content:
Sorry, a shareable link is not currently available for this article.
Provided by the Springer Nature SharedIt content-sharing initiative
- Geostatistics
- Hierarchical Bayesian models
- Point processes
- Preferential sampling
- Find a journal
- Publish with us
- Track your research

An official website of the United States government
The .gov means it’s official. Federal government websites often end in .gov or .mil. Before sharing sensitive information, make sure you’re on a federal government site.
The site is secure. The https:// ensures that you are connecting to the official website and that any information you provide is encrypted and transmitted securely.
- Publications
- Account settings
Preview improvements coming to the PMC website in October 2024. Learn More or Try it out now .
- Advanced Search
- Journal List
- Korean J Anesthesiol
- v.75(2); 2022 Apr
The principles of presenting statistical results using figures
Jae hong park.
1 Department of Anesthesiology and Pain Medicine, Haeundae Paik Hospital, Inje University College of Medicine, Busan, Korea
Dong Kyu Lee
2 Department of Anesthesiology and Pain Medicine, Dongguk University Ilsan Hospital, Goyang, Korea
3 Department of Anesthesiology and Pain Medicine, Chung-Ang University College of Medicine, Seoul, Korea
Jong Hae Kim
4 Department of Anesthesiology and Pain Medicine, Daegu Catholic University School of Medicine, Daegu, Korea
Francis Sahngun Nahm
5 Department of Anesthesiology and Pain Medicine, Seoul National University Bundang Hospital, Seongnam, Korea
Sang Gyu Kwak
6 Department of Medical Statistics, Daegu Catholic University School of Medicine, Daegu, Korea
Chi-Yeon Lim
7 Department of Biostatistics, Dongguk University College of Medicine, Goyang, Korea
Associated Data
Tables and figures are commonly adopted methods for presenting specific data or statistical analysis results. Figures can be used to display characteristics and distributions of data, allowing for intuitive understanding through visualization and thus making it easier to interpret the statistical results. To maximize the positive aspects of figure presentation and increase the accuracy of the content, in this article, the authors will describe how to choose an appropriate figure type and the necessary components to include. Additionally, this article includes examples of figures that are commonly used in research and their essential components using virtual data.
Introduction
All studies based on scientific approaches in anesthesia and pain medicine must involve an analysis of data to support a theory. After establishing a hypothesis and determining the research subjects, the researcher organizes the data obtained into specific categories. In most cases, data are composed of numbers or letters, but can also be stored as photos or figures, depending on the type of research. After researchers classify and index the data, they must decide which statistical analysis method to use. In general, data composed of numbers or letters are stored in tables with rows and columns. This can easily be accomplished using spreadsheet-based computer programs. The simple functions provided by spreadsheet programs, such as classification and sorting, facilitate the interpretation of the essential characteristics of the data, such as structure and frequency. In addition, some spreadsheet programs can show the results of these simple functions as graphs (such as dots, straight lines, or bars) such that the structure and characteristics of the data can be grasped quickly through visualization.
Graphs can be used to present the statistical analysis results in such a way as to make them intuitively easy to understand. For many research papers, the statistical results are illustrated using graphs to support their theory and to enable visual comparisons with other study results. Even though presenting data and statistical results using visual graphs have many advantages, representative values of variables are not presented as exact numbers. Therefore, it is essential to follow some basic principles that allow for graphical representations to be both transparent and precise so information is not misinterpreted. A previous Statistical Round article has covered the general principles of presenting statistical results as text, tables, and figures [ 1 ]. The current article provides further examples of how to present basic statistical results as graphs and essential aspects to consider to prevent distorted interpretations.
Common considerations
In this section, general considerations for presenting graphs are described. Although not all aspects are essential, we have summarized the key points to improve accuracy and minimize errors when using graphs for information transfer and interpretation.
When data are expressed using dots, lines, diagrams, etc., the axes of the graph should have ticks on a scale sufficient to identify the value corresponding to the position of each mark. Both major ticks and minor ticks can be used to indicate the scale on an axis; however, a corresponding value should at least be presented as a major tick. The axis title should include the name of the measurement variable or result and the unit of measurement. If the scale of the axis is an arithmetic distribution, the interval between the marks should be displayed uniformly. When the value of a variable is transformed during analysis or if the measured value has already been transformed, the interval between the marks should be adjusted according to the characteristics of the data. In this case, the type of transformation or measurement scale used should be included in the graph legend ( Fig. 1 ).

Histogram and accompanying density plot of baseline BNP. The baseline BNP shows a right-skewed distribution. The X-axis scale is logarithmic, and an explanation regarding the x-axis scale should be included in the footnote. Note the difference between the most frequently observed value and the representative value (dashed line). BNP: B-type natriuretic peptide, hsTnI: high-sensitivity troponin I, POD: postoperative day. From the previously-published article: "Moon YJ, Kwon HM, Jung KW, et al. Preoperative high-sensitivity troponin I and B-type natriuretic peptide, alone and in combination, for risk stratification of mortality after liver transplantation. Korean J Anesthesiol 2021; 74: 242-53."
If a part of the axis is removed, it is recommended that a break be inserted into the axis and the scales before and after the break be the same ( Fig. 2 ). If the numbering of an axis has to start from a non-zero value, or if the scales before and after the break must be different, an explanation should be included.

An example of a line and dot plot. Note that there is a break on the y-axis, which is inserted to reduce the white space. The measured value at each time point is on those at the adjacent time points. The interpolated line between dots (markers) indicates their changing trend. The statistical method used was the two-way mixed ANOVA with one within- and one between-factor, and post-hoc Bonferroni adjusted pairwise comparisons. There was statistical intergroup difference (F[1,112] = 6.542, P = 0.012) and a significant interaction between group and time (F[3, 336.4] = 3.535, P = 0.015). * P < 0.05 between groups, † P < 0.05 between groups at each time point.
Each axis should have an appropriate range to distinguish between the data presented in the graph. In the case that the range is too large or too small for the displayed data values, the visual comparison of the data may appear exaggerated or the difference may not be recognizable.
Two-dimensional graphs with orthogonally oriented horizontal and vertical axes (x-axis and y-axis, respectively) that cross at a reference point of zero are most commonly used. However, an additional vertical axis can be included on the opposite side of the existing vertical axis if necessary to represent two variables with different measurement units in a single diagram. 1)
Representative values
The preferred type of graph should be chosen based on the representative value of the data (absolute value, fraction, average, median, etc.). Choosing the most-commonly used graph type for a specific representative value helps the reader to interpret the data or statistical results accurately. However, in the case that the use of an uncommon type of graph is unavoidable, an explanation of the representative value and error term must be provided to prevent misunderstanding.
Symbols, lines, and diagrams for representative values
When a symbol, line, or diagram is used to indicate the representative value of the data, the size or thickness of the line should be adjusted appropriately. Additionally, the degree of adjustment should be uniform so that different sizes or thicknesses are not misunderstood as large or small values. In addition, the size and thickness should be adjusted to indicate real values. When symbols or lines are expressed in overlapping or very close proximity, they must have an appropriate size and thickness to allow for an accurate comparison of the values ( Fig. 2 ). A statistical program or other types of program that draws a professional graph rather than a picture-editing tool should be used to accurately represent the positions of symbols, lines, and diagrams with the corresponding values. The graph tools provided by most statistical programs offer user-selected symbols and lines that can be accurately marked according to the corresponding values.
It is recommended that the same symbols be used every time a representative value is represented. However, to distinguish between different groups, different symbols can be used to improve discrimination. The use of different symbols to present the representative values of the same group is not recommended.
A line can be used either when every point represents a specific value or when it visually indicates a change between two symbols ( Fig. 3 ). In the latter case, adding lines between symbols can make the interpretation difficult if the change is not meaningful. Different lines should be used for different groups or situations ( Fig. 2 ). Sometimes, it may be difficult to distinguish between different dashes owing to the line thickness, the size of the graph, or overlapping lines. Therefore, different line types should be adjusted to allow for easy discernability. One option may be to use a color graph; however, this is recommended only when it is impossible to express the information accurately in black and white. Because some readers may have difficulty distinguishing colors, care must be taken regarding color selection.

An example of a dot-line graph. Dots and error bars indicate the means and SDs. The interpolated line allows for enhanced estimation of the changing trend. Bar plots could also be used to represent this kind of statistical result.
The representative value can also be presented using a shape. If the area or form of the shape is proportional to the value, an explanation of this fact should be included. For a diagram expressed at regular intervals where the height or length corresponds to the value (such as a histogram), precautions similar to those regarding symbols or lines should be applied.
Various colors or specific patterns can be used inside the diagram to facilitate interpretation. It is good practice to set different colors or patterns for each group or to use them differently to allow for data before and after an event to be distinguishable. However, such a graph may become complicated as a result of too many colors and patterns or a lack of unified notation.
A description of the variable or situation, represented by lines, symbols, or shapes, should be included in the graph legend. The legend can be located inside or outside the graph, as long as it does not interfere with interpretation. Explanations of values that the symbols, lines, and/or diagrams represent should be included. If abbreviations are used, their definitions should be included in the figure legend. Borders of the legend box can be added as needed around the legend to make it easier to read, and it may be helpful to match the order of data as it appears in both the legend and the graph.
Statistically inferred representative values and their corresponding errors can be indicated on the graph in various ways. Most commonly, whisker-shaped symbols are used to express errors. Depending on the type of graph, it is typically expressed by the length of a line or an area. When there are many representative values or considerable overlap, the symbols used to express the error will also overlap, making it difficult to distinguish between them. If the spread of data is equal on both sides, such as with a normal distribution, it can be presented in only one direction; however, both errors should be presented when the data are skewed to one side. Alternatively, to avoid overlap, the positions of the corresponding values may be moved forward or backward slightly; however, an explanation of this should be included in the figure legend. For example, if it is difficult to distinguish between the means and standard deviations of blood pressure measured at 5 sec after medication in two groups, the representative values of each group can be displayed at 4.9, and 5.1 sec. It is recommended to describe an explanation that the blood pressure values of the two groups measured at specific time point are displayed separately in the figure legend ( Fig. 2 ). For representative examples, refer to the previous Statistical Round article [ 1 ].
Annotations can be added to the graph to explain specific values or statistically significant differences. Annotations are also used to highlight visible differences in the graphs (in which case, instead of an annotation, an explanation should be included in the figure legend). Symbols can be used for annotations that explain statistical differences and should be consistent in type and order throughout the paper. As specified in the instructions to the authors for the Korean Journal of Anesthesiology, it usually follows the order: * (asterisk), † (dagger), ‡ (double dagger, diesis), § (silcrow), and ¶ (pilcrow) [ 2 , 3 ].
Figure legend
In order for readers to know what is contained in a figure and the results of any statistical analysis conducted, a figure legend should be included. A figure legend usually consists of a graph title, a brief description of the graph content, statistical methods, and results. Definitions of any abbreviations and/or symbols used should also be included to facilitate interpretation.
Commonly used graphs
Scatter plots.
A scatter plot shows the associations between two numerical variables measured from one subject ( Fig. 4 ). By adding another variable, three-dimensional expression is also possible. Scatter plots can also be used for ordered categorical variables, at the expense of reduced readability. A scatter plot displays the coordinates of the measured values on an orthogonal plane with two variables as axes using specific symbols, such as dots. The two variables may be independent of each other or may have a cause-effect relationship. Scatter plots are primarily used in the data exploration stage to examine the relationship between two variables, and a trend line 2) can be added to indicate a statistically significant relationship between the two variables. Scatter plots help the reader to understand the relationship between two variables and contribute considerably to the visual expression and understanding of correlation or regression analyses.

An example of a scatter plot. This plot presents the cardiac output value for the same patients using two different measurement methods: EDCO (esophageal doppler cardiac output) and TDCO (continuous thermodilution method). From the previously-published article: “Shim YH, Oh YJ, Nam SB, et. al. Cardiac output estimations by esophageal Doppler cannot replace estimations by the thermodilution method in off-pump coronary artery bypass surgery patients. Korean J Anesthesiol 2003; 45: 456–61.”
As described above, a scatter plot usually demonstrates the relationship between the actual values between two variables. In addition, however, a scatter plot is used for interpretation in some statistical methods. One example is the Bland-Altman scatter plot, which is a method used to analyze the agreement between two measurements ( Fig. 5 ). In addition, scatter plots are often used to evaluate residuals in regression analyses or visually check the fit of a statistically estimated model.

Bland-Altman scatter plot comparing the standard frontal position with an alternative mandibular position. The dotted horizontal line represents the mean difference between the two measures. The dashed horizontal lines represent the 95% limit of agreement between the two measures. The 95% limit of agreement is drawn at the mean difference +/- 1.96 times the standard deviation of the difference. The solid line is the line of equality which indicates the exact same value between two measures.
A line plot is a graph that connects a series of repeatedly measured data points using a straight or curved line, based on a scatter plot. This type of graph is used in several fields to represent various statistical results. A commonly used example is any case in which the data are measured at a set time interval. A run chart (run-sequential plot) is a line plot that displays the data in chronological order. When applying a continuous variable on one axis, such as time, caution must be taken regarding the scale interval. Ordered categorical variables are also candidates for line plots. With scatter plots, measured values are mainly used to examine the data distribution; however, line plots are used primarily for averages, which are representative values of the measured data under specific conditions in the relevant group. As previously mentioned, the errors (such as the standard deviation) must be displayed on a line plot with the representative values.
For bar charts, the height or length of each bar represents the value of the variables, and the ratio between them makes it easy to visualize the differences between categorical variables. On either the horizontal or vertical axis, the values are presented as scale values, whereas on the other axis, the values are presented by other measurement parameters. This type of graph can also be used to express continuous variables, and it is possible to express multiple measured values as cumulative or grouped values using different bar appearances.
A histogram is a graph used to represent the frequency distribution of the data ( Fig. 1 ). Each column’s height indicates the number of samples corresponding to each bin, divided by a fixed interval. Because the variable corresponding to the bin has the characteristics of a continuous variable, the bins are adjacent to each other but do not overlap. Bar plots differ from histograms. In a bar plot, the bars are separated from each other because they represent the values of categorical variables. Each column’s height in a histogram can also be normalized in the form of the frequency of the samples for the total sample size. In this case, mathematical methods, such as kernel density estimation, can be used to smooth the overall shape (smoothing) and estimate a density plot that can be used to represent the distribution of the data.
Boxplots and box-and-whisker plots
A boxplot is a graph that is used to express the median and quartiles of data using a box shape. It is often used to represent nonparametric statistics ( Fig. 6 , Supplementary R code ). A whisker, which is represented by a line extending from each box, can be used to indicate the range of the data (box-and-whisker plot). The range of data defined using whiskers can be set according to the researchers’ needs. For example, the ends of both whiskers can be the maximum and minimum values or values corresponding to 10% and 90% of the entire data range. If both ends of the whiskers are set to values that correspond to the first quartile minus 1.5 times the interquartile range (IQR) and the third quartile plus 1.5 times the IQR, data outside this range can be defined as outliers. The box-and-whisker plot enables recognition of the distribution of data without a specific distribution assumption and displays data dispersion and kurtosis. Depending on the data spread, one of the quartiles and the median may overlap. In this case, the location of the median should be clearly expressed. Violin and bee-swarm plots are improved versions of the box-and-whisker plot and can be used to represent the frequency of data at specific values along with the spread of data.

An example of a box-whisker plot. Estimated median (Q1, Q3) [min:max] from the sample data is 1.1 (0.8, 1.3) [0.1:2.1]. This graph includes explanations of the components of the box-whisker plot. These are not necessary for the general purpose of publication. A significance marker can be added, though it was not used in this graph. If a significance maker is added, it should be located on the shoulder or alongside the whisker. If markers are located over the mid-top of the whiskers, these could be interpreted as outliers if no detailed explanation is provided. The limits of the whiskers can be varied depending on the purpose.
Other commonly used graphs
In addition to the basic graphs previously introduced, various graphs have also been used to present the results or evaluate the analysis process for a specific statistical method. Some examples include receiver operating characteristic (ROC) curves [ 4 ], survival curves, regression curves by linear regression analysis, and dose-response curves. These graphs deliver information on a specific relationship between interpreted statistical results or indicate the trend of independent and dependent variables expressed as functions. These graphs have predetermined components that reflect the characteristics of the data and analysis, and these components must be included in the graph. Additional information must also be included with these graphs to facilitate interpretation, such as corresponding statistics, tables, trend lines, and guidelines. The graph output from a statistics program includes most of the basic requirements, but some parts may need to be added or removed in some cases. In addition, the graph should be composed according to the guidelines of the target journal because the requirements may vary.
Graphs for specific statistical analysis methods
In general, statistical analyses begin with the selection of a specific statistical method according to the characteristics of the collected variables and the expected relationship between them. Most statistical methods require particular features and relationships between variables, and the estimated results are formalized. The following sections include graphs that express specific statistical results. The following graphs are only examples, and other graph types may be appropriate, depending on the characteristics of the data collected.
All of the example graphs were created using R software 4.1.0 for Windows (R Development Core Team, Austria, 2021). The ggplot2 package used in the R software provides various options for creating graphs in the medical field and a user-centered graph editing function. All examples are fictitious data assuming clinical or experimental conditions and should not be interpreted as actual data. All virtual data and R codes are provided in the Supplementary Materials ( Supplementary material 1; R code ).
Independent t-tests
For the first example, data on the time from administration of a neuromuscular blocking agent antagonist to the patients’ first movement after general anesthesia between two different agents are compared ( Supplementary material 2; reverse.csv ). In total, 218 patients were included in this study. Both groups satisfied the assumption of normal distribution but violated the equality of variance; therefore, an unequal variance t -test was performed ( Table 1 ). Fig. 7 shows a graph of the results in the form of a vertical bar graph ( Supplementary material 1; R code ). 3)

An example of a horizontal bar plot with an error bar. Positive-sided error bars are marked because the SDs are located at the same distance from the mean. The recommended legend for this figure is: “The elapsed time from administration to first movement for two different reversal agents: an anticholinergic (n = 109) and a new drug (n = 109); *two-sided P value < 0.05 with the unequal variances t -test”.
Time to Movement After Two Neuromuscular Reversal Agents
Reversal agent | Time (s) | P value |
---|---|---|
Anticholinergic (n =109) | 70 ± 11 | < 0.001 |
New drug (n =109) | 58 ± 8 |
Data are presented as mean ± SD.
Paired t-tests
The next example includes virtual data on the required air volume to ensure endotracheal cuff sealing during general anesthesia ( Supplementary material 3; cuff_pressure.csv ). After tracheal intubation with an adequately sized tube, cuff sealing was achieved through either an arbitrary volume that prevented end-inspiratory leak or by a volume resulting in a cuff pressure of 25 mmHg. The two alternative volumes necessary for the two cuff sealing methods were measured for each patient, and a total of 100 patients were included. A paired t -test was performed because the two methods were conducted on each patient. The results are presented in Table 2 . Fig. 3 shows a graphical representation of the results ( Supplementary material 1; R code ).
Cuff Inflation Volume to Prevent End-inspiratory Gas Leakage
Cuff inflation methods | Required volume (ml) | P value |
---|---|---|
Manual | 55.1 ± 20.4 | < 0.001 |
Pressure at 25 mmHg | 25.3 ± 7.8 |
Values are presented as mean ± SD.
Comparisons between more than three independent groups
For the following example, information on the amount of opioids administered for pain control after three types of surgery were obtained ( Supplementary material 4; opioid_surgery.csv ). The total number of patients was 171 (57 in each group).
One-way analysis of variance (ANOVA) was performed, and there was a statistically significant difference in the opioid dose administered according to the surgery type. Tukey’s test was performed for post-hoc testing. The results showed that the opioid dose administered after operation C was significantly higher than that administered after operations A or B ( Table 3 ).
Postoperative Opioid Requirements according to Three Different Types of Surgery
Surgical type | Opioid dose (μg) | P value |
---|---|---|
A | 541 ± 158 | < 0.001 |
B | 561 ± 102 | |
C | 724 ± 121 |
A graph of the statistical results is shown in Fig. 8 . As the three groups were not related to each other, they are expressed as bar graphs. The results of the statistical tests are presented in the Supplementary material 1; R code .

An example of a vertical bar plot. The asterisk (*) is used to represent a comparative statistically significant result.
Comparisons for repeatedly measured data
In the following example, virtual data on the effect of an antihypertensive drug on diastolic blood pressure were used ( Supplementary material 5; dbpmedication.csv ). A total of 114 patients were included, and the control and treatment groups were equally allocated. Data were measured six times at 5-second intervals, including the time of drug administration. For statistical analysis, two-way mixed ANOVA with one within-factor and one between-factor was used. There was a statistically significant difference between the treatment and control groups (F[1,112] = 6.542, P = 0.012), and there was a statistically significant interaction between the treatment and the time (F[3, 336.4] = 3.535, P = 0.015). The treatment group showed significant differences at 15, 20, and 25 s after administration (adjusted P = 0.004, P = 0.003, and P = 0.006, respectively; Table 4 ). The detailed statistical analysis process was omitted, but a graph of the results is shown in Fig. 2 . The graphs are slightly shifted to the left and right so that they can be distinguished from each other, and a gap is set on the y-axis. These methods make the results easier to visualize by preventing the graphs from overlapping and reducing the whitespace ( Supplementary material 1; R code ).
Changes in Diastolic Blood Pressure after Antihypertensive Treatment
Time point | Control (n = 57, mmHg) | Treatment (n = 57, mmHg) |
---|---|---|
Initial | 71.1 ± 11.6 | 73.0 ± 12.2 |
5 s | 70.8 ± 11.9 | 73.5 ± 12.1 |
10 s | 71.4 ± 13.7 | 76.2 ± 13.4 |
15 s | 70.2 ± 14.0 | 78.1 ± 14.2 |
20 s | 68.5 ± 13.8 | 76.6 ± 14.8 |
25 s | 69.2 ± 12.2 | 76.2 ± 14.5 |
Values are presented as mean ± SD. Two-way mixed analysis of variance with one within factor and one between factor. A statistically significant intergroup difference (F[1,112] = 6.542, P = 0.012) and a significant interaction between group and time (F[3, 336.4] = 3.535, P = 0.015) are seen.
Categorical data comparisons
For the following example, two categorical variables (endotracheal intubation success and sore throat occurrence) were assessed in relation to two different intubation techniques ( Supplementary material 6; sorethr.csv ). The data included two observations from 106 patients (53 patients in each group). The chi-square test with Yate’s correction showed that the success rate of the new tracheal intubation technique was significantly higher than that of the conventional technique (P = 0.018), whereas there was no statistical difference in sore throat occurrence ( Table 5 ). The results are represented using a bar graph classified by observation ( Fig. 9 ). Because the 95% CIs are not symmetrically distributed with respect to the representative values, both error bars are presented and statistical significance is indicated using symbols. To better represent the data, the sample size may also be displayed ( Supplementary material 1; R code ).

An example of a grouped bar plot. The height of each bar indicates the observed rate. If the CIs of the rate are not distributed symmetrically from the observed rate, both sides of the error bar should be presented. The asterisk indicates statistical significance.
Observed Intubation Success and Presence of Sore Throat after the Conventional and New Intubation Technique
Event | Control (n = 53) | New (n = 53) | P value |
---|---|---|---|
Successful intubation | 32 (60.4) | 44 (83) | 0.018 |
Sore throat | 20 (37.7) | 11 (20.8) | 0.088 |
Values are presented as numbers (percentiles).
Other commonly used statistical graphs
Correlation analyses, linear regression.
As an example of correlation analysis, the blood concentrations of three intravenous anesthetic adjuvants were measured during propofol general anesthesia ( Supplementary material 7; pretxlevel.csv ). All three adjuvants (A, B, and C) showed a positive correlation with exposure time (correlation coefficient r = 0.71, r = 0.65, and r = 0.42, respectively), but only the coefficient of adjuvant A was statistically significant (P = 0.014, P = 0.117, and P = 0.132, respectively; Fig. 10 ). Various diagrams can be used to show these correlations. However, in this article, a scatter plot with a trend line for the group, and the statistical analysis results are presented ( Supplementary material 1; R code ).

An example of a scatter plot with a linear trend line for the correlation analysis. The asterisk indicates statistical significance.
A scatter plot with a trend line clearly represents the data and is used more often in linear regression analyses than in correlation analyses. For the linear regression example graph, blood glucose concentrations and the degree of glucose deposition in the mitral valve node were used in patients with type 2 diabetes with rheumatic mitral valve insufficiency ( Supplementary material 8; dmmvi.csv ). Linear regression analysis was performed with blood glucose concentration as the independent variable and the degree of glucose deposition in the mitral valve as the dependent variable. The regression equation was estimated to be “Glucose in nodule = 0.048 × Blood glucose concentration + 32.98 (P < 0.001)”. The graph in Fig. 11 shows the observed values with a regression line and other necessary information ( Supplementary R code ).

An example of a scatter plot with a trend line for the linear regression. Around the regression line, the shadowed area indicates the range of the 95% CI of the estimated coefficient. The estimated regression line formula is also presented in the graph with statistics.
Logistic regression
For the following example, virtual data showed the influence of five factors on specific test results ( Supplementary material 9; five_factors.csv ). The test result is a yes/no dichotomous variable, whereas all five factors (F1 to F5) are continuous variables. Although logistic regression analyses involve various assumptions that must be verified before statistical analysis to obtain accurate results, the contents of such verification processes have been omitted. The model estimated by logistic regression provides the odds ratio (OR) for each independent variable ( Table 6 ). A graphic representation of ORs allows for a clearer interpretation than a table in the case of multiple independent variables or ORs with many numbers ( Fig. 12 , Supplementary material 1; R code ).

An example of a dot plot with an error bar. For each level of factors (y-axis), corresponding odds ratio (OR) and 95% CIs are presented using dots and accompanying horizontal error bar. The dotted line indicates the reference value of 1. The estimated OR would not be different from 1.0 statistically if its error bar crossed this reference line.
Estimated OR and 95% CI of Logistic Regression Model
Factor | OR (95% CI) | P value |
---|---|---|
F1 | 1.24 (1.12, 1.38) | < 0.001 |
F2 | 1.76 (1.26, 2.51) | 0.001 |
F3 | 1.10 (0.80, 1.50) | 0.557 |
F4 | 1.00 (0.98, 1.02) | 0.810 |
F5 | 1.09 (0.99, 1.20) | 0.083 |
OR: odds ratio.
Survival analysis
Survival analysis is a statistical method that can be applied to mortality data and various types of longitudinal data. There are various methods, from the nonparametric Kaplan-Meier method to more complex methods involving different parametric models. Kaplan-Meier survival analysis and Cox regression models are widely used in the medical field. Survival analysis results usually accompany the survival curve, which can increase the reader’s understanding of the results through visualization. For details on the survival curve, refer to the previous Statistical Round article [ 5 , 6 ]. An example of a survival curve is shown in Fig. 13 . In addition to several important pieces of information that should be included, the survival table must be attached to the survival curve because the number at risk is reduced at the end of the observation. This can minimize the likelihood of misinterpretation.

An example of a survival curve. Two survival curves with 95% CIs are presented. The median survival time is also indicated for each curve. Because the number at risk decreases at the end of observation, the survival table should be incorporated with curves to clarify the statistical inference process. From the previously-published article: "In J, Lee DK. Survival analysis: part II - applied clinical data analysis. Korean J Anesthesiol 2019; 72: 441-57."
Dose-response curve
For this example, various concentrations of two antibiotics were assessed by measuring the absorbance of a specific light known to be proportional to the normal bacterial flora amount in a culture medium ( Supplementary material 10; antiobsorp.csv ). The data were fitted using a 4-parameter log-logistic model; the estimated parameters are summarized in Table 7 . A graph of the fitted model is presented in Fig. 14 ( Supplementary material 1; R code ). The absorbance values for the doses of the two antibiotics are expressed using symbols, and a dose-response curve was drawn. Compared to a table that includes only numbers, using a graph is more intuitive and easier to interpret.

An example of multiple dose-response curves. Observed values are plotted using dot symbols: filled circles and triangles. The straight solid and dashed lines indicate the ED50 value of each curve. Be aware that the x-axis is log scaled.
Dose-response Curve Model Fit Result
Parameters | A | B | P value |
---|---|---|---|
Slope | 2.57 (1.79, 3.36) | 5.41 (3.74, 7.07) | < 0.001 |
Lower limit | 0.11 (0.09, 0.13) | 0.11 (0.09, 0.13) | < 0.001 |
Upper limit | 0.56 (0.54, 0.59) | 0.56 (0.54, 0.59) | < 0.001 |
ED50 | 17.20 (15.06, 19.33) | 7.32 (6.55, 8.09) | < 0.001 |
Estimated ED ratio (A/B) | |||
ED10 | 1.50 (1.06, 1.95) | - | |
ED50 | 2.35 (2.01, 2.68) | - | |
ED90 | 3.67 (2.55, 4.80) | - |
Dose-response curve fit using a 4-parameter log-logistic model. Values are presented as estimates (95% CI). ED: effective dose at a certain response level indicated by the following number as the percentile.
Conclusions
There are many types of graphs for various statistical methods that can be used to represent data and results, depending on their characteristics. Trying out a few types of graphs that show the characteristics well and then choosing the best one among them is recommended. Presenting results with a table and a figure simultaneously takes up space and can distract readers. Therefore, it is recommended to use graphs and discuss significant results in the body of the manuscript, and tables of granular information can be moved to the supplementary material or vice versa.
1) In addition to a two-dimensional graph consisting of a horizontal (x-axis) and a vertical axis (y-axis), a three-dimensional graph using a third axis (z-axis) perpendicular to both axes is also widely used in specific fields. In this article, we will focus on two-dimensional graphs.
2) The trend line is a type of regression graph that provides useful information regarding the relationship between two variables and can be fitted as linear, quadratic, or cubic formulas.
3) When the range of error has both positive and negative values, like a continuous variable, the histogram contains the possibility of error in a strict sense. This is because, when expressed as a bar graph, the error range on one side does not appear on the graph (as shown in Fig. 7). While there is a way to express both sides when the range of error is different, it is not commonly used. In most medical papers, they are used without distinction given the general perception that the error range expressed in the bar graph is naturally distributed equally on both sides.
Conflicts of Interest
No potential conflict of interest relevant to this article was reported.
Author Contributions
Jae Hong Park (Conceptualization; Methodology; Validation; Writing – review & editing)
Dong Kyu Lee (Data curation; Formal analysis; Methodology; Supervision; Validation; Writing – original draft; Writing – review & editing)
Hyun Kang (Conceptualization; Data curation; Writing – review & editing)
Jong Hae Kim (Conceptualization; Data curation; Writing – review & editing)
Francis Sahngun Nahm (Conceptualization; Data curation; Writing – review & editing)
EunJin Ahn (Conceptualization; Data curation; Writing – review & editing)
Junyong In (Conceptualization; Data curation; Validation; Writing – review & editing)
Sang Gyu Kwak (Conceptualization; Data curation; Writing – review & editing)
Chi-Yeon Lim (Conceptualization; Data curation; Writing – review & editing)
Supplementary Materials
Supplementary material 1., supplementary material 2., supplementary material 3..
cuff pressure
Supplementary Material 4.
opioid_surgery
Supplementary Material 5.
dbpmedication
Supplementary Material 6.
Supplementary material 7., supplementary material 8., supplementary material 9..
five factors
Supplementary Material 10.
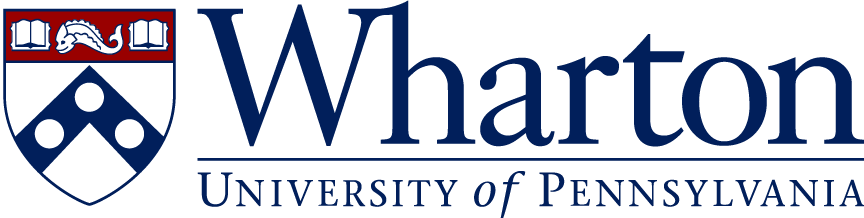
- Youth Program
- Wharton Online
Research Papers / Publications
Have a language expert improve your writing
Run a free plagiarism check in 10 minutes, generate accurate citations for free.
- Knowledge Base
The Beginner's Guide to Statistical Analysis | 5 Steps & Examples
Statistical analysis means investigating trends, patterns, and relationships using quantitative data . It is an important research tool used by scientists, governments, businesses, and other organizations.
To draw valid conclusions, statistical analysis requires careful planning from the very start of the research process . You need to specify your hypotheses and make decisions about your research design, sample size, and sampling procedure.
After collecting data from your sample, you can organize and summarize the data using descriptive statistics . Then, you can use inferential statistics to formally test hypotheses and make estimates about the population. Finally, you can interpret and generalize your findings.
This article is a practical introduction to statistical analysis for students and researchers. We’ll walk you through the steps using two research examples. The first investigates a potential cause-and-effect relationship, while the second investigates a potential correlation between variables.
Table of contents
Step 1: write your hypotheses and plan your research design, step 2: collect data from a sample, step 3: summarize your data with descriptive statistics, step 4: test hypotheses or make estimates with inferential statistics, step 5: interpret your results, other interesting articles.
To collect valid data for statistical analysis, you first need to specify your hypotheses and plan out your research design.
Writing statistical hypotheses
The goal of research is often to investigate a relationship between variables within a population . You start with a prediction, and use statistical analysis to test that prediction.
A statistical hypothesis is a formal way of writing a prediction about a population. Every research prediction is rephrased into null and alternative hypotheses that can be tested using sample data.
While the null hypothesis always predicts no effect or no relationship between variables, the alternative hypothesis states your research prediction of an effect or relationship.
- Null hypothesis: A 5-minute meditation exercise will have no effect on math test scores in teenagers.
- Alternative hypothesis: A 5-minute meditation exercise will improve math test scores in teenagers.
- Null hypothesis: Parental income and GPA have no relationship with each other in college students.
- Alternative hypothesis: Parental income and GPA are positively correlated in college students.
Planning your research design
A research design is your overall strategy for data collection and analysis. It determines the statistical tests you can use to test your hypothesis later on.
First, decide whether your research will use a descriptive, correlational, or experimental design. Experiments directly influence variables, whereas descriptive and correlational studies only measure variables.
- In an experimental design , you can assess a cause-and-effect relationship (e.g., the effect of meditation on test scores) using statistical tests of comparison or regression.
- In a correlational design , you can explore relationships between variables (e.g., parental income and GPA) without any assumption of causality using correlation coefficients and significance tests.
- In a descriptive design , you can study the characteristics of a population or phenomenon (e.g., the prevalence of anxiety in U.S. college students) using statistical tests to draw inferences from sample data.
Your research design also concerns whether you’ll compare participants at the group level or individual level, or both.
- In a between-subjects design , you compare the group-level outcomes of participants who have been exposed to different treatments (e.g., those who performed a meditation exercise vs those who didn’t).
- In a within-subjects design , you compare repeated measures from participants who have participated in all treatments of a study (e.g., scores from before and after performing a meditation exercise).
- In a mixed (factorial) design , one variable is altered between subjects and another is altered within subjects (e.g., pretest and posttest scores from participants who either did or didn’t do a meditation exercise).
- Experimental
- Correlational
First, you’ll take baseline test scores from participants. Then, your participants will undergo a 5-minute meditation exercise. Finally, you’ll record participants’ scores from a second math test.
In this experiment, the independent variable is the 5-minute meditation exercise, and the dependent variable is the math test score from before and after the intervention. Example: Correlational research design In a correlational study, you test whether there is a relationship between parental income and GPA in graduating college students. To collect your data, you will ask participants to fill in a survey and self-report their parents’ incomes and their own GPA.
Measuring variables
When planning a research design, you should operationalize your variables and decide exactly how you will measure them.
For statistical analysis, it’s important to consider the level of measurement of your variables, which tells you what kind of data they contain:
- Categorical data represents groupings. These may be nominal (e.g., gender) or ordinal (e.g. level of language ability).
- Quantitative data represents amounts. These may be on an interval scale (e.g. test score) or a ratio scale (e.g. age).
Many variables can be measured at different levels of precision. For example, age data can be quantitative (8 years old) or categorical (young). If a variable is coded numerically (e.g., level of agreement from 1–5), it doesn’t automatically mean that it’s quantitative instead of categorical.
Identifying the measurement level is important for choosing appropriate statistics and hypothesis tests. For example, you can calculate a mean score with quantitative data, but not with categorical data.
In a research study, along with measures of your variables of interest, you’ll often collect data on relevant participant characteristics.
Variable | Type of data |
---|---|
Age | Quantitative (ratio) |
Gender | Categorical (nominal) |
Race or ethnicity | Categorical (nominal) |
Baseline test scores | Quantitative (interval) |
Final test scores | Quantitative (interval) |
Parental income | Quantitative (ratio) |
---|---|
GPA | Quantitative (interval) |
Here's why students love Scribbr's proofreading services
Discover proofreading & editing
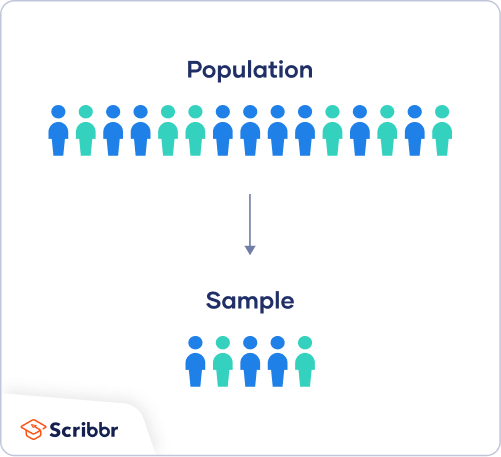
In most cases, it’s too difficult or expensive to collect data from every member of the population you’re interested in studying. Instead, you’ll collect data from a sample.
Statistical analysis allows you to apply your findings beyond your own sample as long as you use appropriate sampling procedures . You should aim for a sample that is representative of the population.
Sampling for statistical analysis
There are two main approaches to selecting a sample.
- Probability sampling: every member of the population has a chance of being selected for the study through random selection.
- Non-probability sampling: some members of the population are more likely than others to be selected for the study because of criteria such as convenience or voluntary self-selection.
In theory, for highly generalizable findings, you should use a probability sampling method. Random selection reduces several types of research bias , like sampling bias , and ensures that data from your sample is actually typical of the population. Parametric tests can be used to make strong statistical inferences when data are collected using probability sampling.
But in practice, it’s rarely possible to gather the ideal sample. While non-probability samples are more likely to at risk for biases like self-selection bias , they are much easier to recruit and collect data from. Non-parametric tests are more appropriate for non-probability samples, but they result in weaker inferences about the population.
If you want to use parametric tests for non-probability samples, you have to make the case that:
- your sample is representative of the population you’re generalizing your findings to.
- your sample lacks systematic bias.
Keep in mind that external validity means that you can only generalize your conclusions to others who share the characteristics of your sample. For instance, results from Western, Educated, Industrialized, Rich and Democratic samples (e.g., college students in the US) aren’t automatically applicable to all non-WEIRD populations.
If you apply parametric tests to data from non-probability samples, be sure to elaborate on the limitations of how far your results can be generalized in your discussion section .
Create an appropriate sampling procedure
Based on the resources available for your research, decide on how you’ll recruit participants.
- Will you have resources to advertise your study widely, including outside of your university setting?
- Will you have the means to recruit a diverse sample that represents a broad population?
- Do you have time to contact and follow up with members of hard-to-reach groups?
Your participants are self-selected by their schools. Although you’re using a non-probability sample, you aim for a diverse and representative sample. Example: Sampling (correlational study) Your main population of interest is male college students in the US. Using social media advertising, you recruit senior-year male college students from a smaller subpopulation: seven universities in the Boston area.
Calculate sufficient sample size
Before recruiting participants, decide on your sample size either by looking at other studies in your field or using statistics. A sample that’s too small may be unrepresentative of the sample, while a sample that’s too large will be more costly than necessary.
There are many sample size calculators online. Different formulas are used depending on whether you have subgroups or how rigorous your study should be (e.g., in clinical research). As a rule of thumb, a minimum of 30 units or more per subgroup is necessary.
To use these calculators, you have to understand and input these key components:
- Significance level (alpha): the risk of rejecting a true null hypothesis that you are willing to take, usually set at 5%.
- Statistical power : the probability of your study detecting an effect of a certain size if there is one, usually 80% or higher.
- Expected effect size : a standardized indication of how large the expected result of your study will be, usually based on other similar studies.
- Population standard deviation: an estimate of the population parameter based on a previous study or a pilot study of your own.
Once you’ve collected all of your data, you can inspect them and calculate descriptive statistics that summarize them.
Inspect your data
There are various ways to inspect your data, including the following:
- Organizing data from each variable in frequency distribution tables .
- Displaying data from a key variable in a bar chart to view the distribution of responses.
- Visualizing the relationship between two variables using a scatter plot .
By visualizing your data in tables and graphs, you can assess whether your data follow a skewed or normal distribution and whether there are any outliers or missing data.
A normal distribution means that your data are symmetrically distributed around a center where most values lie, with the values tapering off at the tail ends.
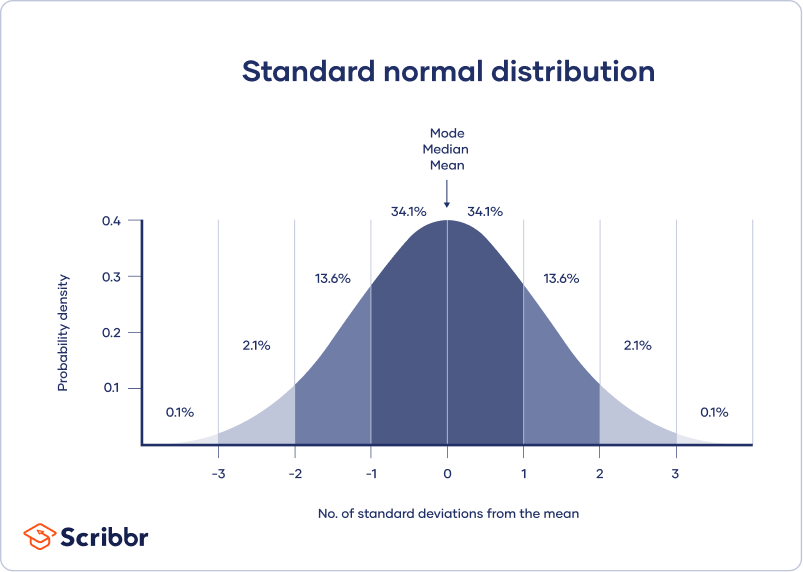
In contrast, a skewed distribution is asymmetric and has more values on one end than the other. The shape of the distribution is important to keep in mind because only some descriptive statistics should be used with skewed distributions.
Extreme outliers can also produce misleading statistics, so you may need a systematic approach to dealing with these values.
Calculate measures of central tendency
Measures of central tendency describe where most of the values in a data set lie. Three main measures of central tendency are often reported:
- Mode : the most popular response or value in the data set.
- Median : the value in the exact middle of the data set when ordered from low to high.
- Mean : the sum of all values divided by the number of values.
However, depending on the shape of the distribution and level of measurement, only one or two of these measures may be appropriate. For example, many demographic characteristics can only be described using the mode or proportions, while a variable like reaction time may not have a mode at all.
Calculate measures of variability
Measures of variability tell you how spread out the values in a data set are. Four main measures of variability are often reported:
- Range : the highest value minus the lowest value of the data set.
- Interquartile range : the range of the middle half of the data set.
- Standard deviation : the average distance between each value in your data set and the mean.
- Variance : the square of the standard deviation.
Once again, the shape of the distribution and level of measurement should guide your choice of variability statistics. The interquartile range is the best measure for skewed distributions, while standard deviation and variance provide the best information for normal distributions.
Using your table, you should check whether the units of the descriptive statistics are comparable for pretest and posttest scores. For example, are the variance levels similar across the groups? Are there any extreme values? If there are, you may need to identify and remove extreme outliers in your data set or transform your data before performing a statistical test.
Pretest scores | Posttest scores | |
---|---|---|
Mean | 68.44 | 75.25 |
Standard deviation | 9.43 | 9.88 |
Variance | 88.96 | 97.96 |
Range | 36.25 | 45.12 |
30 |
From this table, we can see that the mean score increased after the meditation exercise, and the variances of the two scores are comparable. Next, we can perform a statistical test to find out if this improvement in test scores is statistically significant in the population. Example: Descriptive statistics (correlational study) After collecting data from 653 students, you tabulate descriptive statistics for annual parental income and GPA.
It’s important to check whether you have a broad range of data points. If you don’t, your data may be skewed towards some groups more than others (e.g., high academic achievers), and only limited inferences can be made about a relationship.
Parental income (USD) | GPA | |
---|---|---|
Mean | 62,100 | 3.12 |
Standard deviation | 15,000 | 0.45 |
Variance | 225,000,000 | 0.16 |
Range | 8,000–378,000 | 2.64–4.00 |
653 |
A number that describes a sample is called a statistic , while a number describing a population is called a parameter . Using inferential statistics , you can make conclusions about population parameters based on sample statistics.
Researchers often use two main methods (simultaneously) to make inferences in statistics.
- Estimation: calculating population parameters based on sample statistics.
- Hypothesis testing: a formal process for testing research predictions about the population using samples.
You can make two types of estimates of population parameters from sample statistics:
- A point estimate : a value that represents your best guess of the exact parameter.
- An interval estimate : a range of values that represent your best guess of where the parameter lies.
If your aim is to infer and report population characteristics from sample data, it’s best to use both point and interval estimates in your paper.
You can consider a sample statistic a point estimate for the population parameter when you have a representative sample (e.g., in a wide public opinion poll, the proportion of a sample that supports the current government is taken as the population proportion of government supporters).
There’s always error involved in estimation, so you should also provide a confidence interval as an interval estimate to show the variability around a point estimate.
A confidence interval uses the standard error and the z score from the standard normal distribution to convey where you’d generally expect to find the population parameter most of the time.
Hypothesis testing
Using data from a sample, you can test hypotheses about relationships between variables in the population. Hypothesis testing starts with the assumption that the null hypothesis is true in the population, and you use statistical tests to assess whether the null hypothesis can be rejected or not.
Statistical tests determine where your sample data would lie on an expected distribution of sample data if the null hypothesis were true. These tests give two main outputs:
- A test statistic tells you how much your data differs from the null hypothesis of the test.
- A p value tells you the likelihood of obtaining your results if the null hypothesis is actually true in the population.
Statistical tests come in three main varieties:
- Comparison tests assess group differences in outcomes.
- Regression tests assess cause-and-effect relationships between variables.
- Correlation tests assess relationships between variables without assuming causation.
Your choice of statistical test depends on your research questions, research design, sampling method, and data characteristics.
Parametric tests
Parametric tests make powerful inferences about the population based on sample data. But to use them, some assumptions must be met, and only some types of variables can be used. If your data violate these assumptions, you can perform appropriate data transformations or use alternative non-parametric tests instead.
A regression models the extent to which changes in a predictor variable results in changes in outcome variable(s).
- A simple linear regression includes one predictor variable and one outcome variable.
- A multiple linear regression includes two or more predictor variables and one outcome variable.
Comparison tests usually compare the means of groups. These may be the means of different groups within a sample (e.g., a treatment and control group), the means of one sample group taken at different times (e.g., pretest and posttest scores), or a sample mean and a population mean.
- A t test is for exactly 1 or 2 groups when the sample is small (30 or less).
- A z test is for exactly 1 or 2 groups when the sample is large.
- An ANOVA is for 3 or more groups.
The z and t tests have subtypes based on the number and types of samples and the hypotheses:
- If you have only one sample that you want to compare to a population mean, use a one-sample test .
- If you have paired measurements (within-subjects design), use a dependent (paired) samples test .
- If you have completely separate measurements from two unmatched groups (between-subjects design), use an independent (unpaired) samples test .
- If you expect a difference between groups in a specific direction, use a one-tailed test .
- If you don’t have any expectations for the direction of a difference between groups, use a two-tailed test .
The only parametric correlation test is Pearson’s r . The correlation coefficient ( r ) tells you the strength of a linear relationship between two quantitative variables.
However, to test whether the correlation in the sample is strong enough to be important in the population, you also need to perform a significance test of the correlation coefficient, usually a t test, to obtain a p value. This test uses your sample size to calculate how much the correlation coefficient differs from zero in the population.
You use a dependent-samples, one-tailed t test to assess whether the meditation exercise significantly improved math test scores. The test gives you:
- a t value (test statistic) of 3.00
- a p value of 0.0028
Although Pearson’s r is a test statistic, it doesn’t tell you anything about how significant the correlation is in the population. You also need to test whether this sample correlation coefficient is large enough to demonstrate a correlation in the population.
A t test can also determine how significantly a correlation coefficient differs from zero based on sample size. Since you expect a positive correlation between parental income and GPA, you use a one-sample, one-tailed t test. The t test gives you:
- a t value of 3.08
- a p value of 0.001
Prevent plagiarism. Run a free check.
The final step of statistical analysis is interpreting your results.
Statistical significance
In hypothesis testing, statistical significance is the main criterion for forming conclusions. You compare your p value to a set significance level (usually 0.05) to decide whether your results are statistically significant or non-significant.
Statistically significant results are considered unlikely to have arisen solely due to chance. There is only a very low chance of such a result occurring if the null hypothesis is true in the population.
This means that you believe the meditation intervention, rather than random factors, directly caused the increase in test scores. Example: Interpret your results (correlational study) You compare your p value of 0.001 to your significance threshold of 0.05. With a p value under this threshold, you can reject the null hypothesis. This indicates a statistically significant correlation between parental income and GPA in male college students.
Note that correlation doesn’t always mean causation, because there are often many underlying factors contributing to a complex variable like GPA. Even if one variable is related to another, this may be because of a third variable influencing both of them, or indirect links between the two variables.
Effect size
A statistically significant result doesn’t necessarily mean that there are important real life applications or clinical outcomes for a finding.
In contrast, the effect size indicates the practical significance of your results. It’s important to report effect sizes along with your inferential statistics for a complete picture of your results. You should also report interval estimates of effect sizes if you’re writing an APA style paper .
With a Cohen’s d of 0.72, there’s medium to high practical significance to your finding that the meditation exercise improved test scores. Example: Effect size (correlational study) To determine the effect size of the correlation coefficient, you compare your Pearson’s r value to Cohen’s effect size criteria.
Decision errors
Type I and Type II errors are mistakes made in research conclusions. A Type I error means rejecting the null hypothesis when it’s actually true, while a Type II error means failing to reject the null hypothesis when it’s false.
You can aim to minimize the risk of these errors by selecting an optimal significance level and ensuring high power . However, there’s a trade-off between the two errors, so a fine balance is necessary.
Frequentist versus Bayesian statistics
Traditionally, frequentist statistics emphasizes null hypothesis significance testing and always starts with the assumption of a true null hypothesis.
However, Bayesian statistics has grown in popularity as an alternative approach in the last few decades. In this approach, you use previous research to continually update your hypotheses based on your expectations and observations.
Bayes factor compares the relative strength of evidence for the null versus the alternative hypothesis rather than making a conclusion about rejecting the null hypothesis or not.
If you want to know more about statistics , methodology , or research bias , make sure to check out some of our other articles with explanations and examples.
- Student’s t -distribution
- Normal distribution
- Null and Alternative Hypotheses
- Chi square tests
- Confidence interval
Methodology
- Cluster sampling
- Stratified sampling
- Data cleansing
- Reproducibility vs Replicability
- Peer review
- Likert scale
Research bias
- Implicit bias
- Framing effect
- Cognitive bias
- Placebo effect
- Hawthorne effect
- Hostile attribution bias
- Affect heuristic
Is this article helpful?
Other students also liked.
- Descriptive Statistics | Definitions, Types, Examples
- Inferential Statistics | An Easy Introduction & Examples
- Choosing the Right Statistical Test | Types & Examples
More interesting articles
- Akaike Information Criterion | When & How to Use It (Example)
- An Easy Introduction to Statistical Significance (With Examples)
- An Introduction to t Tests | Definitions, Formula and Examples
- ANOVA in R | A Complete Step-by-Step Guide with Examples
- Central Limit Theorem | Formula, Definition & Examples
- Central Tendency | Understanding the Mean, Median & Mode
- Chi-Square (Χ²) Distributions | Definition & Examples
- Chi-Square (Χ²) Table | Examples & Downloadable Table
- Chi-Square (Χ²) Tests | Types, Formula & Examples
- Chi-Square Goodness of Fit Test | Formula, Guide & Examples
- Chi-Square Test of Independence | Formula, Guide & Examples
- Coefficient of Determination (R²) | Calculation & Interpretation
- Correlation Coefficient | Types, Formulas & Examples
- Frequency Distribution | Tables, Types & Examples
- How to Calculate Standard Deviation (Guide) | Calculator & Examples
- How to Calculate Variance | Calculator, Analysis & Examples
- How to Find Degrees of Freedom | Definition & Formula
- How to Find Interquartile Range (IQR) | Calculator & Examples
- How to Find Outliers | 4 Ways with Examples & Explanation
- How to Find the Geometric Mean | Calculator & Formula
- How to Find the Mean | Definition, Examples & Calculator
- How to Find the Median | Definition, Examples & Calculator
- How to Find the Mode | Definition, Examples & Calculator
- How to Find the Range of a Data Set | Calculator & Formula
- Hypothesis Testing | A Step-by-Step Guide with Easy Examples
- Interval Data and How to Analyze It | Definitions & Examples
- Levels of Measurement | Nominal, Ordinal, Interval and Ratio
- Linear Regression in R | A Step-by-Step Guide & Examples
- Missing Data | Types, Explanation, & Imputation
- Multiple Linear Regression | A Quick Guide (Examples)
- Nominal Data | Definition, Examples, Data Collection & Analysis
- Normal Distribution | Examples, Formulas, & Uses
- Null and Alternative Hypotheses | Definitions & Examples
- One-way ANOVA | When and How to Use It (With Examples)
- Ordinal Data | Definition, Examples, Data Collection & Analysis
- Parameter vs Statistic | Definitions, Differences & Examples
- Pearson Correlation Coefficient (r) | Guide & Examples
- Poisson Distributions | Definition, Formula & Examples
- Probability Distribution | Formula, Types, & Examples
- Quartiles & Quantiles | Calculation, Definition & Interpretation
- Ratio Scales | Definition, Examples, & Data Analysis
- Simple Linear Regression | An Easy Introduction & Examples
- Skewness | Definition, Examples & Formula
- Statistical Power and Why It Matters | A Simple Introduction
- Student's t Table (Free Download) | Guide & Examples
- T-distribution: What it is and how to use it
- Test statistics | Definition, Interpretation, and Examples
- The Standard Normal Distribution | Calculator, Examples & Uses
- Two-Way ANOVA | Examples & When To Use It
- Type I & Type II Errors | Differences, Examples, Visualizations
- Understanding Confidence Intervals | Easy Examples & Formulas
- Understanding P values | Definition and Examples
- Variability | Calculating Range, IQR, Variance, Standard Deviation
- What is Effect Size and Why Does It Matter? (Examples)
- What Is Kurtosis? | Definition, Examples & Formula
- What Is Standard Error? | How to Calculate (Guide with Examples)
What is your plagiarism score?
Become a Member
The isi family, call for papers: 2026 special issue of the statistics education research journal.
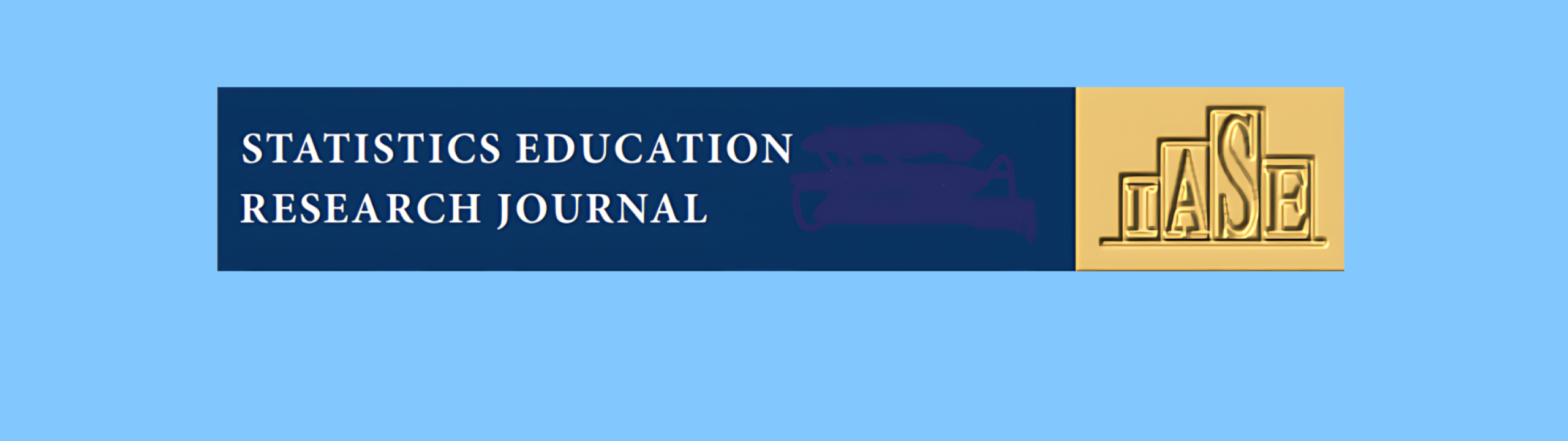
Areas of control in Syria as of April 2023. Source: Liveuamap, 2023
Prior to the conflict, Syria had advanced vaccination governance and high immunization coverage, with World Health Organization (WHO) and United Nations International Children’s Emergency Fund (UNICEF) estimating DTP vaccine coverage at over 89% [ 17 ]. During the conflict, vaccination activities faced significant challenges following the withdrawal of the Syrian Government from opposition-controlled territories in 2012. This led to disruptions in the supply chain, human resource shortages, and governance collapse, resulting in reduced vaccination coverage and outbreaks of diseases such as Polio and Measles [ 18 ]. Emergency vaccination campaigns were initiated by local and international actors to address these outbreaks, with the establishment of entities such as the Polio Task Force and Measles Task Force. Since 2016, vaccination efforts have been led by the Syria Immunisation Group (SIG), formed by local humanitarian actors and co-chaired by WHO and UNICEF. Please see Table 1 for the vaccination schedule in Syria before and after the conflict.
Despite Syria’s eligibility for Global Alliance for Vaccines and Immunization (GAVI) support in 2019, actual funding received remains lower than pledged, making it challenging to assess the total cost of vaccine activities [ 19 , 20 ]. The literature on vaccination governance in northwest Syria is scant, with limited distinction between northwest Syria and government-controlled areas. Comprehensive accounts of SIG’s work are rare, with the WHO 2020 report on Syria providing one notable exception [ 21 ]. This lack of literature may reflect the complex political economy context, as government withholding of vaccinations prompted alternative actors to facilitate vaccination and governance [ 22 ].
This study aims to explore the effectiveness and efficiency of vaccination governance in northwest Syria (NWS), its responsiveness, inclusivity, and informed decision-making processes, as well as its vision, strategy, transparency, and accountability. By examining these aspects, the research seeks to provide a comprehensive understanding of how vaccination programs operate in conflict-affected areas and the unique challenges they face.
Methodology
This study employed a mixed-methods approach consisting of semi-structured qualitative interviews, a validation workshop, and ethnographic observations to comprehensively investigate vaccination governance in northwest Syria.
Firstly, we adapted the Siddiqi framework for health governance [ 23 ] with modifications to accommodate the unique challenges and dynamics present in northwest Syria. Its six key principles offer a structured approach to assess governance effectiveness, inclusivity, transparency, and accountability, which were central to the study’s objectives. This adapted framework guided the data collection, analysis, and interpretation processes, providing a structured approach to examining vaccination governance from a health system perspective.
Secondly, we conducted 14 semi-structured qualitative Key Informant Interviews (KIIs) with key informants involved in vaccination governance in northwest Syria. Purposive sampling was used to select participants representing various stakeholders, including representatives from local health directorates, international organizations, and community leaders - please see Table 2 . Participants were identified based on their expertise and roles in vaccination delivery. We approached potential participants through email and phone calls, explaining the purpose of the study and inviting them to participate. Those who agreed to participate were scheduled for interviews at their convenience. The semi-structured interview guide (see Supplementary Material) aimed to explore participants’ experiences, perspectives, and challenges related to vaccination governance. The interviews were audio-recorded with participants’ consent and transcribed verbatim for analysis. Thematic analysis was conducted using both deductive and inductive approaches, with the Siddiqi framework guiding the thematic grouping and coding process. Notably, only two of the interviewees identified as female. This gender disparity reflects broader gender imbalances in leadership positions within the context of conflict-affected areas and may influence the perspectives and priorities discussed during the workshop.
Thirdly, a validation workshop was conducted in Gaziantep in November 2023 to validate the findings from the interviews and gather additional insights from stakeholders. The 15 participants in the workshop included key informants who had been interviewed, as well as other relevant stakeholders – please see Table 2 . An overview of the key findings per theme identified in the interviews was presented, followed by a discussion to validate and elaborate on these findings. The workshop facilitated a collaborative process to prioritize the main achievements and challenges identified in the interviews.
In addition, ethnographic observations were conducted alongside the field data collection to provide contextual insights into vaccination delivery and governance practices in northwest Syria. These observations involved daily immersion in the field, engaging in informal conversations with stakeholders, and documenting observations through field notes. This approach was used to build trust with key stakeholders, helping them understand the importance of our research and encouraging them to openly share their views and participate in research activities. The informal conversations and daily immersion provided rich qualitative data on the local context, practices, and challenges, which were crucial for interpreting the collected data. Additionally, relevant documents, such as reports and policy documents, were collected and analysed to complement the ethnographic data.
The three sets of data—interviews, workshop discussions, and ethnographic observations—were triangulated to enhance the validity and reliability of the findings. Triangulation was conducted through comparing and cross-referencing information from each data source. Initially, key themes and findings from the interviews were identified and categorised. These themes were then cross-checked against insights gathered from workshop discussions and ethnographic observations to identify common patterns, discrepancies, and unique contributions. Any discrepancies were further investigated through follow-up discussions or additional document analysis to resolve inconsistencies and confirm findings.
Ethical approval was obtained from the Institutional Review Board of King’s College London (MRA-22/23-34048) and, due to the sensitive nature of the subject, anonymity of participants was deemed critical. Informed consent was signed by all interviewees and interview records were deleted within two days after the interview, with notes being de-identified. All records and code-keys were stored on a password-protected secure drive.
This section presents five key themes that emerged from the data: effectiveness and efficiency, inclusiveness and data availability, clear vision with limited participatory strategy development, limited transparency, and accountability and sustainability. For each theme, findings are triangulated from interviews, workshop discussions, and ethnographic observations to provide a comprehensive understanding of vaccination governance in northwest Syria.
Effectiveness and efficiency
Field observations highlighted the operational success of the vaccination strategy, particularly in maintaining cold-chain reliability and conducting extensive outreach activities. Researchers noted that cold-chain facilities appeared well-maintained and outreach teams were active in various communities.
Document analysis corroborated these observations, although it revealed a lack of detailed analysis in formal reports regarding vaccine losses and linkage between disease outbreak data and coverage statistics. The annual report for 2021 noted the distribution of over 1.5 million routine vaccines and approximately 350,000 COVID-19 vaccines (SIG, 2021).
KIIs provided subjective assessments of effectiveness, with most participants rating the vaccination strategy very positively. For example, one key informant stated, “Cold-chain is very complicated, and (…) we have never faced gaps in the cold-chain. The outreach activities too, they are amazing in screening the whole community” (K-07). Another participant commented, “I think there are three successful entities in Syria. White Helmets, Early Warning and Response Network (EWARN) and SIG. Basically, they are performing governmental performance, without being a government” (K-10).
The workshop echoed these sentiments, emphasising the reliability of cold-chain logistics and the effectiveness of outreach programs. Participants highlighted the comprehensive knowledge outreach teams had about the communities, such as culture and health seeking behaviour, which facilitated high vaccine coverage.
Analysis suggests that while the subjective assessments are positive, the lack of detailed data in formal documents indicates a need for more robust quantitative evaluation mechanisms to fully substantiate these claims.
Efficiency was qualitatively explored through factors such as human resources, bureaucracy, corruption, and the non-governmental nature of the program. Field observations noted strong capacity among staff and stable governance structures.
Documents reviewed pointed to significant bureaucracy but suggested it was a necessary component to prevent corruption. KIIs reinforced this, with one participant noting, “You can’t do any humanitarian process without this paperwork, to be honest. It is the right way, because otherwise you are corrupted” (K-01). Another added that corruption was low due to the nature of the resources involved, stating, “There are few reasons for people to steal from this programme. It isn’t food baskets or money, it’s vaccines” (K-01).
Workshops confirmed these findings but also highlighted inefficiencies due to the lack of government services and irregular funding, which led to service discontinuations. One workshop participant explained, “The Expanded Programme for Immunisation (EPI) is continuous, it should be a 2 or 3 year project. For example, the first project ends by the end of May and the next project starts mid-June. So, there is a gap for staff, so they don’t receive their salaries” (W-02).
In conclusion, while the vaccination governance seems to be efficient with limited observed effectiveness, challenges remain in documentation and the impacts of funding irregularities, short termism and uncertainty.
Inclusiveness, responsiveness, and data availability
Field observations indicated that accessibility and inclusiveness are prioritized in vaccination efforts, with outreach activities playing a crucial role in reaching vulnerable groups. Researchers observed that outreach sessions outnumbered fixed sessions, reflecting the emphasis on inclusivity.
Document analysis revealed systematic data collection efforts to identify reasons for missed vaccinations to target vulnerable groups, including zero-dose children, people with disabilities, female-headed households, and those living in remote areas. However, significant gaps in demographic data and reliance on paper-based systems were noted, hindering comprehensive coverage analysis.
KIIs highlighted the challenges in data availability. One participant mentioned, “The most reliable approximations of vaccine coverage come from last year’s vaccination data and the door-to-door polio campaign” (K-05). Another added, “Alternative population data is available from OCHA, but it is considered inferior to the more comprehensive and up-to-date polio data” (K-06). This reliance on figures from previous Polio vaccination campaigns is confirmed by our document analysis. In 2021 the SIG vaccinated 134,083 children with Bacillus Calmette–Guérin (BCG). The Polio campaign in the previous year vaccinated a total of 155.378 children under 1. According to third party monitoring, the coverage rate of this polio campaign was 93%. Assuming that the age-distribution of the coverage is equal, this would make the total number of children under 1 in northwest Syria 167.073. Accordingly, the coverage rate for BCG would then be 80.3%. Similar statistics currently being used as coverage data, but these are suboptimal.
Workshop participants echoed these concerns, emphasizing the need for digitalization of medical and vaccination records. A participant remarked, “Paper vaccination cards are often lost, and manual data collection is prone to error. Digital systems are urgently needed” (W-03).
Our analysis indicates that while inclusivity is a stated priority and efforts are made to collect relevant data, the effectiveness of these efforts is limited by significant data availability challenges. Digitalization initiatives are a positive step but require more support and implementation.
Clear vision with limited participatory strategy development
Field observations showed the SIG’s active involvement in strategic planning, supported by WHO and GAVI. Researchers noted clear mission statements and detailed strategies in the SIG’s multi-year plan, though awareness among partners was limited.
Document analysis confirmed the existence of structured strategic plans but indicated fragmented decision-making processes involving multiple stakeholders, including donors, partners, and the SIG. The SIG was observed to function as a central coordination and mediation platform.
KIIs provided insights into the strategic planning processes, with participants acknowledging sufficient opportunities for input but noting limited participation from partners. One participant stated, “I don’t think the NGOs are participating in finding solutions. Mainly the SIG is doing this. The SIG is doing a good job, so we feel relaxed somehow, so we don’t want to interfere in the system” (K-11). Another added, “It is positive that the implementing partners are only implementing the central plans” (K-06).
Workshop participants supported these findings, expressing trust in the SIG’s strategic planning but also highlighting the lack of engagement from partners in the decision-making process. One participant noted, “The SIG maintains the strategy and the quality of the strategy. In humanitarian crises and the Syrian context, we operate as organizations, but we established a central team” (W-04).
Our analysis suggests that while the SIG has a clear vision and structured strategic plans, the limited participatory strategy development may hinder broader ownership and engagement from all partners.
Limited transparency
Field observations noted a general perception of the SIG being approachable, but with limited transparency in documentation. Researchers observed that information sharing was mostly internal, with minimal public disclosure.
Document analysis highlighted the lack of an internet presence, financial disclosure, and public availability of strategic plans and annual reports. Information was primarily disseminated through internal reports and meetings, limiting access for external stakeholders.
KIIs revealed a discrepancy between perceived and actual transparency. One participant commented, “A normal Ministry of Health would not separately publish their vaccination results in so much detail” (K-03). Another stated, “Partners funded through the WHO share their financial data with the SIG, but privately funded partners do not” (K-02).
Workshop participants emphasized the need for greater transparency, particularly for stakeholders not directly involved in the SIG’s network. A participant remarked, “It is difficult to obtain information about the topic if one is not part of the network. Only the WHO and the Assistant Coordination Unit (ACU) additionally report on selected aspects of vaccination” (W-01).
Our analysis indicates that while the SIG is considered transparent by partners due to its approachability, the lack of public documentation and financial disclosure limits overall transparency. Enhanced public communication strategies could improve transparency and accountability.
Accountability and sustainability
Field observations underscored the complex collaboration of stakeholders underpinning vaccine provision, with no single body having legitimate oversight. Filed researchers noted the decentralised structure and reliance on various donors.
Document analysis highlighted the lack of enforcement mechanisms for medical guidelines and protocols. The SIG’s Statement of Principle lacked enforceable standards, leaving de facto power with diverse donors. This patchwork funding approach posed challenges to accountability and sustainability.
KIIs pointed to the absence of a central governance body, with one participant noting, “The donors know that the SIG is not officially on the papers, but they know there is a body called SIG responsible for reaching the target, achieving the indicators, and supervising technically” (K-07). Another participant identified potential risks, stating, “The cut of funds, war, and lack of stability of the security situation. We have the scenario, but we don’t know what will happen” (K-08).
Workshop participants discussed stabilising factors such as the system’s size, decentralized structure, and financial continuity. One participant remarked, “The system grows and becomes a stable system. Everyone is aware of how the system is growing, and this assists the continuity” (W-05).
Our analysis concludes that while there are significant challenges to accountability and sustainability, including fragmented oversight and reliance on diverse donors, stabilizing factors such as decentralization and financial continuity offer some resilience against potential disruptions. Capacity building at district and governorate levels is crucial for ensuring long-term stability and effectiveness.
The primary themes under investigation in this study encompassed the effectiveness and efficiency of the vaccination governance in northwest Syria; its responsiveness, inclusivity, and informed decision-making; its vision and strategy; transparency; and accountability and sustainability.
The management and coordination of vaccination in conflict-affected areas pose significant challenges to effectiveness and efficiency. In regions like northwest Syria, where government control is limited, the discontinuation of routine vaccination services exacerbates these challenges. Comparisons with other conflict-affected areas, such as Myanmar and Somalia, highlight the role of local organizations and international support in filling governance gaps [ 24 , 25 ]. However, research on vaccination coordination in northwest Syria remains sparse, underscoring the need for a deeper understanding of local structures and operations.
Prior to 2016, the health governance model followed a bottom-up approach, with local entities playing significant roles in vaccination activities. With the establishment of SIG, a hybrid top-down and bottom-up model emerged, shifting the focus to international support and coordination while preserving field connection. This model change reflects the unique challenges of vaccination services in conflict-affected regions and underscores the need for a collaborative approach under the United Nations’ umbrella.
The Syria Immunisation Group (SIG) plays a pivotal role in vaccination governance in northwest Syria, aiming to address these challenges. While SIG has gained internal legitimacy through collaboration with health directorates (HDs) and external legitimacy through collaborating with WHO and UNICEF, concerns regarding accountability and inclusivity persist. The lack of transparency and involvement of partners in strategic planning processes hinder informed decision-making. These finding are in line with a study by Alaref et al. in 2023 which evaluated six governance principles for central quasi-governmental institutions in northwest Syria, including SIG, and found that its legitimacy is fair and requires improvement, scoring 41–60% on a health system governance scale adapted for this paper. Accountability, transparency, effectiveness and efficiency were poor and required significant improvement, scoring 21–40%, while strategic vision was very poor or inactive, scoring 0–20% [ 26 ].
Despite having a strategic plan and receiving support from international organisations like the WHO and GAVI, SIG faces contradictions in its effectiveness and efficiency. The transition from emergency task forces to SIG was marked by power dynamics and challenges to local ownership, raising questions about sustainability and integration into national vaccination programs [ 9 ]. The potential transition of WHO operations further complicates the future of SIG, posing a key challenge to early recovery in Syria.
These findings raise questions about the future of the SIG body in light of the political and military changes in the region and the constant threat associated with cross-border operations. What would happen if the WHO ceased operations in Gaziantep and moved to Damascus, where a national vaccine program has been in place for decades? In such a scenario, would the SIG continue to carry out its activities in northwest Syria, or would it become a part of the national vaccine program? This is a key challenge for the transition to early recovery in Syria.
In conclusion, the governance of vaccination in conflict-affected areas of northwest Syria is complex, with multiple stakeholders involved and a lack of a legitimate government to fulfil essential functions. The success of the vaccination program heavily relies on the efforts of the Syria Immunisation Group (SIG), which acts as a trusted mediator between various stakeholders. However, the lack of transparency and accountability hinders the ability to assess the program’s effectiveness and efficiency. This calls for a push towards more localised ownership and transparency, with a hybrid top-down and bottom-up approach that addresses the unique context of conflict settings. Engaging local partners in decision-making and capacity building can improve sustainability and address issues surrounding legitimacy. Moreover, the responsibility to protect public health goes beyond national sovereignty, and the role of international bodies like the WHO becomes crucial in conflict areas. Inaction or delayed action can have catastrophic consequences, as witnessed in Syria with the emergence of diseases like polio and measles. It is essential to implement a structured feedback mechanism and transparent monitoring and evaluation processes to address challenges and foster trust among stakeholders and the community. Ultimately, the findings of this study inform debates around health governance in conflict settings, highlighting the need for more inclusive, transparent, and context-sensitive approaches to ensure the success and sustainability of vaccination programs.
Data availability
The datasets generated and/or analysed during the current study are not publicly available due to the sensitive nature of the data, but are available from the corresponding author on reasonable request.
Abbreviations
Syria Immunisation Group
Early Warning and Response Network
Expanded Programme on Immunization
World Health Organization
Global Alliance for Vaccines and Immunization
United Nations Office for the Coordination of Humanitarian Affairs
Health Directorates
Global Alliance for Vaccines and Immunisation
Key Informant Interviews
United Nations International Children’s Emergency Fund
Sato R. Effect of armed conflict on vaccination: evidence from the Boko Haram insurgency in northeastern Nigeria. Confl Health. 2019;13(1):1–10.
Article Google Scholar
Ngo NV, Pemunta NV, Muluh NE, Adedze M, Basil N, Agwale S. Armed conflict, a neglected determinant of childhood vaccination: some children are left behind. 2019;16(6):1454–63. https://doi.org/10.1080/2164551520191688043
Lam E, McCarthy A, Brennan M. Vaccine-preventable diseases in humanitarian emergencies among refugee and internally-displaced populations. Hum Vaccin Immunother. 2015;11(11):2627–36.
Article PubMed PubMed Central Google Scholar
Kennedy J, Michailidou D. Civil war, contested sovereignty and the limits of global health partnerships: a case study of the Syrian polio outbreak in 2013. Health Policy Plan. 2017;32(5):690–8.
Article PubMed Google Scholar
Pereira A, de Southgate L, Ahmed R, O’Connor H, Cramond P, Lenglet V. A. Infectious Disease Risk and Vaccination in Northern Syria after 5 years of Civil War: the MSF experience. PLoS Curr. 2018;10.
Tajaldin B, Almilaji K, Langton P, Sparrow A. Defining polio: closing the gap in global surveillance. Ann Glob Health. 2015;81(3):386–95.
Ahmad B, Bhattacharya S. Polio eradication in Syria. Lancet Infect Dis. 2014;14(7):547–8.
Meiqari L, Hoetjes M, Baxter L, Lenglet A. Impact of war on child health in northern Syria: the experience of Médecins sans Frontières. Eur J Pediatr. 2018;177(3):371–80.
Initiative GPE. In. Syrian Arab Republic. 2021. p. 191–191.
Alkhalil M, Alaref M, Mkhallalati H, Alzoubi Z, Ekzayez A. An analysis of humanitarian and health aid alignment over a decade (2011–2019) of the Syrian conflict. Confl Health. 2022.
Alkhalil M, Ekzayez A, Rayes D, Abbara A. Inequitable access to aid after the devastating earthquake in Syria. Lancet Glob Health. 2023;0(0).
OCHA. Northwest Syria Humanitarian Readiness and Response Plan. 2020.
Zulfiqar ABBC, Reality C. 2020 [cited 2020 May 2]. Syria: Who’s in control of Idlib? - BBC News. https://www.bbc.co.uk/news/world-45401474
EUAA. 1.3. Anti-government armed groups | European Union Agency for Asylum [Internet]. 2020 [cited 2023 Sep 10]. https://euaa.europa.eu/country-guidance-syria/13-anti-government-armed-groups
Alkhalil M, Alaref M, Ekzayez A, Mkhallalati H, El Achi N, Alzoubi Z, et al. Health aid displacement during a decade of conflict (2011–19) in Syria: an exploratory analysis. BMC Public Health. 2023;23(1):1–16.
Security Council Report. In. Hindsight: the demise of the Syria cross-border aid mechanism, August 2023 Monthly Forecast. Security Council Report; 2023.
WHO, Unicef. Immunization Summary: A statistical reference containing data through 2010. Vol. 2011. 2011.
Ekzayez A, Alkhalil M, Patel P, Bowsher G. Pandemic governance and community mobilization in conflict: a case study of Idlib, Syria. Inoculating cities: Case studies of the Urban response to the COVID-19 pandemic. 2024;61–80.
OECD. Creditor Reporting System (CRS) [Internet]. 2023 [cited 2023 Dec 1]. https://stats.oecd.org/Index.aspx?DataSetCode=CRS1
Kaddar M, Saxenian H, Senouci K, Mohsni E, Sadr-Azodi N. Vaccine procurement in the Middle East and North Africa region: challenges and ways of improving program efficiency and fiscal space. Vaccine. 2019;37(27):3520–8.
World Health Organization. World Health Organization Syrian Arab Republic [Internet]. 2020 [cited 2023 Sep 10]. http://apps.who.int/bookorders
ACU. Annual report 2019. Vol. 5, AIMS Mathematics. 2019.
Siddiqi S, Masud TI, Nishtar S, Peters DH, Sabri B, Bile KM, et al. Framework for assessing governance of the health system in developing countries: gateway to good governance. Health Policy. 2009;90(1):13–25.
Hugh Guan T, Htut HN, Davison CM, Sebastian S, Bartels SA, Aung SM, et al. Implementation of a neonatal hepatitis B immunization program in rural Karenni State, Myanmar: a mixed-methods study. PLoS ONE. 2021;16(12 December):e0261470.
Hugh Guan T, Htut HN, Davison CM, Sebastian S, Bartels SA, Aung SM et al. Implementation of a neonatal hepatitis B immunization program in rural Karenni State, Myanmar: A mixed-methods study. PLoS One [Internet]. 2021 Dec 1 [cited 2023 May 9];16(12 December):e0261470. https://journals.plos.org/plosone/article?id=10.1371/journal.pone.0261470
Alaref M, Al-Abdulla O, Al Zoubi Z, Al Khalil M, Ekzayez A. Health system governance assessment in protracted crisis settings: Northwest Syria. Health Res Policy Syst. 2023;21(1):1–13.
Download references
Acknowledgements
The authors acknowledge invaluable contributions of several staff based in Turkey and Syria for their input, access and support. We also wish to acknowledge in particularly contribution from Dr. Mahmoud Daher, then Head of the Gaziantep (Turkey) Office. Furthermore, appreciation is expressed for the contributions of the Assistance Coordination Unit staff, for the documents they made available for this study and their input in the analysis.
This publication is funded through the National Institute for Health Research (NIHR) 131207, Research for Health Systems Strengthening in northern Syria (R4HSSS), using UK aid from the UK Government to support global health research. The views expressed in this publication are those of the author(s) and do not necessarily reflect those of the NIHR or the UK government.
Author information
Ronja Kitlope Baatz and Abdulkarim Ekzayez are equal contributors to this work and designated as co-first authors.
Authors and Affiliations
Deventer Hospital, Deventer, Netherlands
Ronja Kitlope Baatz
Research for Health System Strengthening in northern Syria (R4HSSS), The Centre for Conflict & Health Research (CCHR), King’s College London, Strand, WC2R 2LS, London, UK
Abdulkarim Ekzayez & Preeti Patel
Syria Development Centre (SyriaDev), London, UK
Abdulkarim Ekzayez
Syria Immunisation Group (SIG), Gaziantep, Turkey
Yasser Najib & Mohammad Salem
Syria Public Health Network, London, UK
Munzer Alkhalil
Research for Health System Strengthening in Northern Syria (R4HSSS), UOSSM, Gaziantep, Turkey
Vascular Senior Clinical Fellow, Manchester Royal Infirmary, Manchester, UK
Mohammed Ayman Alshiekh
You can also search for this author in PubMed Google Scholar
Contributions
The initial framing, literature review, data collection and drafting of the study were carried out by RB and AE. AE contributed to the design, supervision, data collection, data analysis, and multiple rounds of editing. YN contributed to access to data, data collection, and data analysis. MS contributed to access to data and data analysis. PP contributed to analysis and multiple rounds of editing. Mohammed Ayman Alshiekh (MA) contributed to analysis and multiple rounds of editing. Munzer Alkhalil contributed to analysis and multiple rounds of editing. All authors read and approved the final manuscript. All authors read and approved the final manuscript.
Corresponding authors
Correspondence to Ronja Kitlope Baatz or Abdulkarim Ekzayez .
Ethics declarations
Ethics approval and consent to participate.
Ethical approval was obtained from the Institutional Review Board of King’s College London, under the approval number MRA-22/23-34048. Informed consent was obtained from all participants involved in the study. Participants were provided with detailed information regarding the study’s objectives, procedures, potential risks, and benefits. They were assured of their right to withdraw from the study at any time without any repercussions. All data collected were anonymised to ensure the confidentiality and privacy of the participants.
Consent for publication
Not applicable.
Competing interests
The authors declare no competing interests.
Additional information
Publisher’s note.
Springer Nature remains neutral with regard to jurisdictional claims in published maps and institutional affiliations.
Electronic supplementary material
Below is the link to the electronic supplementary material.
Supplementary Material 1
Rights and permissions.
Open Access This article is licensed under a Creative Commons Attribution 4.0 International License, which permits use, sharing, adaptation, distribution and reproduction in any medium or format, as long as you give appropriate credit to the original author(s) and the source, provide a link to the Creative Commons licence, and indicate if changes were made. The images or other third party material in this article are included in the article’s Creative Commons licence, unless indicated otherwise in a credit line to the material. If material is not included in the article’s Creative Commons licence and your intended use is not permitted by statutory regulation or exceeds the permitted use, you will need to obtain permission directly from the copyright holder. To view a copy of this licence, visit http://creativecommons.org/licenses/by/4.0/ .
Reprints and permissions
About this article
Cite this article.
Baatz, R.K., Ekzayez, A., Najib, Y. et al. Vaccination governance in protracted conflict settings: the case of northwest Syria. BMC Health Serv Res 24 , 1056 (2024). https://doi.org/10.1186/s12913-024-11413-1
Download citation
Received : 16 January 2024
Accepted : 07 August 2024
Published : 12 September 2024
DOI : https://doi.org/10.1186/s12913-024-11413-1
Share this article
Anyone you share the following link with will be able to read this content:
Sorry, a shareable link is not currently available for this article.
Provided by the Springer Nature SharedIt content-sharing initiative
- Immunisation
- Vaccination
- Health governance
- Conflict setting
- Localisation
BMC Health Services Research
ISSN: 1472-6963
- General enquiries: [email protected]
This week: the arXiv Accessibility Forum
Help | Advanced Search
Computer Science > Computation and Language
Title: can large language models unlock novel scientific research ideas.
Abstract: "An idea is nothing more nor less than a new combination of old elements" (Young, J.W.). The widespread adoption of Large Language Models (LLMs) and publicly available ChatGPT have marked a significant turning point in the integration of Artificial Intelligence (AI) into people's everyday lives. This study explores the capability of LLMs in generating novel research ideas based on information from research papers. We conduct a thorough examination of 4 LLMs in five domains (e.g., Chemistry, Computer, Economics, Medical, and Physics). We found that the future research ideas generated by Claude-2 and GPT-4 are more aligned with the author's perspective than GPT-3.5 and Gemini. We also found that Claude-2 generates more diverse future research ideas than GPT-4, GPT-3.5, and Gemini 1.0. We further performed a human evaluation of the novelty, relevancy, and feasibility of the generated future research ideas. This investigation offers insights into the evolving role of LLMs in idea generation, highlighting both its capability and limitations. Our work contributes to the ongoing efforts in evaluating and utilizing language models for generating future research ideas. We make our datasets and codes publicly available.
Comments: | 24 pages, 12 figures, 6 tables |
Subjects: | Computation and Language (cs.CL); Artificial Intelligence (cs.AI); Computers and Society (cs.CY); Human-Computer Interaction (cs.HC); Machine Learning (cs.LG) |
Cite as: | [cs.CL] |
(or [cs.CL] for this version) | |
Focus to learn more arXiv-issued DOI via DataCite (pending registration) |
Submission history
Access paper:.
- HTML (experimental)
- Other Formats
References & Citations
- Google Scholar
- Semantic Scholar
BibTeX formatted citation

Bibliographic and Citation Tools
Code, data and media associated with this article, recommenders and search tools.
- Institution
arXivLabs: experimental projects with community collaborators
arXivLabs is a framework that allows collaborators to develop and share new arXiv features directly on our website.
Both individuals and organizations that work with arXivLabs have embraced and accepted our values of openness, community, excellence, and user data privacy. arXiv is committed to these values and only works with partners that adhere to them.
Have an idea for a project that will add value for arXiv's community? Learn more about arXivLabs .

IMAGES
VIDEO
COMMENTS
The journal aims to be the major resource for statistical modelling, covering both methodology and practice. Its goal is to be multidisciplinary in nature, promoting the cross-fertilization of ideas between substantive research areas, as well as providing a common forum for the comparison, unification and nurturing of modelling issues across different subjects.
Overview. Statistical Papers is a forum for presentation and critical assessment of statistical methods encouraging the discussion of methodological foundations and potential applications. The Journal stresses statistical methods that have broad applications, giving special attention to those relevant to the economic and social sciences.
1. Introduction. Statistical modeling methods [Citation 1-17] are widely used in clinical science, epidemiology, and health services research to analyze and interpret data obtained from clinical trials as well as observational studies of existing data sources, such as claims files and electronic health records.Diagnostic and prognostic inferences from statistical models are critical if ...
Zero-inflated and hurdle models are widely applied to count data possessing excess zeros, where they can simultaneously model the process from how the zeros were generated and potentially help mitigate the eff... Yixuan Zou, Jan Hannig and Derek S. Young. Journal of Statistical Distributions and Applications 2021 8:5.
The innovative statistical algorithms of the past 50 years are statistical in the sense of being motivated and developed in the context of the structure of a statistical problem. The EM algorithm (Dempster, Laird, and Rubin 1977; Meng and van Dyk 1997), Gibbs sampler (Geman and Geman 1984; Gelfand and Smith 1990), particle filters (Kitagawa ...
Guogen Shan. Xinlin Lu. Samuel S. Wu. Regular Article 31 August 2024. Bayesian and frequentist inference derived from the maximum entropy principle with applications to propagating uncertainty about statistical methods. David R. Bickel. Short Communication 27 August 2024. Reduced bias estimation of the log odds ratio.
Abstract. Statistical modeling methods are widely used in clinical science, epidemiology, and health services research to analyze data that has been collected in clinical trials as well as ...
The baseline projection model using an age-period-cohort model or generalised linear model for each cancer type was selected based on model fit statistics and validation with pre-COVID-19 observed ...
This book focuses on methods and models in classification and data analysis and presents real-world applications at the interface with data science. Numerous topics are covered, ranging from statistical inference and modelling to clustering and factorial methods, and from directional data analysis to time series analysis and small area estimation.
spmodel is an R package used to fit, summarize, and predict for a variety spatial statistical models applied to point-referenced or areal (lattice) data. Parameters are estimated using various methods, including likelihood-based optimization and weighted least squares based on variograms. Additional modeling features include anisotropy, non-spatial random effects, partition factors, big data ...
An Introduction to Statistical. Modelling. Kelvin Jones, School of Geographical Sciences, University of Bristol, UK. Summary. ( Regression modelling. ( Researching 'cause and effect' relations ...
The research study adhered to a systematic methodology, delineated in Fig. 1, comprising several stages to ensure robustness.Initially, data retrieval involved extracting CRCP sections from both ...
Freedman makes a thorough appraisal of the statistical methods in these papers and in a variety of other examples. He illustrates the principles of modeling, and the pitfalls. The discussion shows you how to think about the critical issues - including the connection (or lack of it) between the statistical models and the real phenomena.
Introduction. Statistical analysis is necessary for any research project seeking to make quantitative conclusions. The following is a primer for research-based statistical analysis. It is intended to be a high-level overview of appropriate statistical testing, while not diving too deep into any specific methodology.
Statistical Models: Theory and Practice. This lively and engaging textbook explains the things you have to know in order to read empirical papers in the social and health sciences, as well as the techniques you need to build statistical models of your own. The author, David A. Freedman, explains the basic ideas of association and regression ...
Type and distribution of the data used. For the same objective, selection of the statistical test is varying as per data types. For the nominal, ordinal, discrete data, we use nonparametric methods while for continuous data, parametric methods as well as nonparametric methods are used.[] For example, in the regression analysis, when our outcome variable is categorical, logistic regression ...
The method pre-sented in this paper proposes to construct a faster running surrogate for such a computationally intensive nonlinear function, and to use it in a related non-linear statistical model that accounts for the uncertainty associated with this surrogate.
Research on the influencing factors of promoting flipped classroom teaching based on the integrated UTAUT model and learning engagement theory Yufan Pan & Wang He
The use of complex statistical models has recently increased substantially in the context of species distribution behavior. This complexity has made the inferential and predictive processes challenging to perform. The Bayesian approach has become a good option to deal with these models due to the ease with which prior information can be incorporated along with the fact that it provides a more ...
The statistical method used was the two-way mixed ANOVA with one within- and one between-factor, and post-hoc Bonferroni adjusted pairwise comparisons. There was statistical intergroup difference (F[1,112] = 6.542, P = 0.012) and a significant interaction between group and time (F[3, 336.4] = 3.535, P = 0.015).
Research Papers / Publications. Search Publication Type ... Ye Zhang (2024), Fundamental Limits of Spectral Clustering in Stochastic Block Models, IEEE Transactions on Information Theory (Accepted). Ye Zhang and Harrison H. Zhou (2024), Leave-one-out Singular Subspace Perturbation Analysis for Spectral Clustering, Annals of Statistics (Accepted).
This article is a practical introduction to statistical analysis for students and researchers. We'll walk you through the steps using two research examples. The first investigates a potential cause-and-effect relationship, while the second investigates a potential correlation between variables. Example: Causal research question.
emergence of Support Vector Machines (SVMs). Statistical learning methods serve as the foundation for developin g machine. intelligence and hold a pivotal position in a wide range of fields, s uch ...
Time Series Analysis and Modeling to Forecast: a Survey. Fatoumata Dama, Christine Sinoquet. View a PDF of the paper titled Time Series Analysis and Modeling to Forecast: a Survey, by Fatoumata Dama and 1 other authors. Time series modeling for predictive purpose has been an active research area of machine learning for many years.
CALL FOR PAPERS: 2026 SPECIAL ISSUE OF THE STATISTICS EDUCATION RESEARCH JOURNALThis special issue aims to showcase the diverse and innovative approaches to statistics education across the African continent, emphasising the unique challenges and opportunities faced by African countries. Within this special issue, the term statistics should be broadly viewed to include data science as well as ...
Effective vaccination governance in conflict-affected regions poses unique challenges. This study evaluates the governance of vaccination programs in northwest Syria, focusing on effectiveness, efficiency, inclusiveness, data availability, vision, transparency, accountability, and sustainability. Using a mixed-methods approach, and adapting Siddiqi's framework for health governance, data ...
Given that Large Language Models (LLMs) have made significant progress in writing code, can they now be used to autonomously reproduce results from research repositories? Such a capability would be a boon to the research community, helping researchers validate, understand, and extend prior work. To advance towards this goal, we introduce SUPER, the first benchmark designed to evaluate the ...
The Comprehensive ESRD Care (CEC) Model was designed to identify, test, and evaluate new ways to improve care for Medicare beneficiaries with End-Stage Renal Disease (ESRD). Through the CEC Model, CMS partnered with health care providers and suppliers to test the effectiveness of a new payment and service delivery model in providing beneficiaries with person-centered, high-quality care.
"An idea is nothing more nor less than a new combination of old elements" (Young, J.W.). The widespread adoption of Large Language Models (LLMs) and publicly available ChatGPT have marked a significant turning point in the integration of Artificial Intelligence (AI) into people's everyday lives. This study explores the capability of LLMs in generating novel research ideas based on information ...
Purpose: This paper is designed to critically review and analyze the body of research on a popular gang reduction strategy, implemented widely in the United States and a number of other countries, to: (1) assess whether researchers designed their evaluations to align with the theorized causal mechanisms that bring about reductions in violence; and (2) discuss how evidence on gang programs is ...