
An official website of the United States government
The .gov means it’s official. Federal government websites often end in .gov or .mil. Before sharing sensitive information, make sure you’re on a federal government site.
The site is secure. The https:// ensures that you are connecting to the official website and that any information you provide is encrypted and transmitted securely.
- Publications
- Account settings
Preview improvements coming to the PMC website in October 2024. Learn More or Try it out now .
- Advanced Search
- Journal List
- BMC Med Inform Decis Mak


A scoping review of cloud computing in healthcare
Lena griebel.
Department of Medical Informatics, Friedrich-Alexander-University Erlangen-Nürnberg, Wetterkreuz 13, Erlangen, D-91058 Germany
Hans-Ulrich Prokosch
Felix köpcke, dennis toddenroth, jan christoph, martin sedlmayr.
Cloud computing is a recent and fast growing area of development in healthcare. Ubiquitous, on-demand access to virtually endless resources in combination with a pay-per-use model allow for new ways of developing, delivering and using services. Cloud computing is often used in an “OMICS-context”, e.g. for computing in genomics, proteomics and molecular medicine, while other field of application still seem to be underrepresented. Thus, the objective of this scoping review was to identify the current state and hot topics in research on cloud computing in healthcare beyond this traditional domain.
MEDLINE was searched in July 2013 and in December 2014 for publications containing the terms “cloud computing” and “cloud-based”. Each journal and conference article was categorized and summarized independently by two researchers who consolidated their findings.
102 publications have been analyzed and 6 main topics have been found: telemedicine/teleconsultation, medical imaging, public health and patient self-management, hospital management and information systems, therapy, and secondary use of data. Commonly used features are broad network access for sharing and accessing data and rapid elasticity to dynamically adapt to computing demands. Eight articles favor the pay-for-use characteristics of cloud-based services avoiding upfront investments. Nevertheless, while 22 articles present very general potentials of cloud computing in the medical domain and 66 articles describe conceptual or prototypic projects, only 14 articles report from successful implementations. Further, in many articles cloud computing is seen as an analogy to internet-/web-based data sharing and the characteristics of the particular cloud computing approach are unfortunately not really illustrated.
Conclusions
Even though cloud computing in healthcare is of growing interest only few successful implementations yet exist and many papers just use the term “cloud” synonymously for “using virtual machines” or “web-based” with no described benefit of the cloud paradigm. The biggest threat to the adoption in the healthcare domain is caused by involving external cloud partners: many issues of data safety and security are still to be solved. Until then, cloud computing is favored more for singular, individual features such as elasticity, pay-per-use and broad network access, rather than as cloud paradigm on its own.
Electronic supplementary material
The online version of this article (doi:10.1186/s12911-015-0145-7) contains supplementary material, which is available to authorized users.
Medicine is an increasingly data-intensive and collaborative endeavor [ 1 ]. Advances in the OMICS-fields (genomics, proteomics and the like) generate considerable amounts of data to be processed and stored. Secondary use of clinical data with text-or data mining algorithms also entails a growing demand for dynamic, scalable resources. Often these resources are only utilized temporarily so that permanent infrastructure investments are hard to justify and flexible on-demand services are sought alternatively.
Cloud computing seems a viable solution to fulfill these demands. Commercial providers like Amazon and Microsoft promise to make hundreds of virtual machines available at ones’ fingertips, almost immediately and just for the time they are really needed. The advantage of such offers is, that such resources only have to be paid for the configuration, size and time they are actually used.
Thus, the term “cloud computing” is described by the National Institutes of Standards and Technology (NIST) [ 2 ] as a model for enabling ubiquitous, convenient, on-demand access to a shared pool of configurable computing resources. As essential characteristics of cloud computing Mell and Grance have listed (1) on demand self-service, (2) broad network access, (3) resource pooling with other tenants, (4) rapid elasticity, and (5) measured services. Clouds promise advantages in dynamic resources like computing power or storage capacities, ubiquitous access to resources at anytime from any place, and high flexibility and scalability of resources. These benefits have been the reason for increasing adoption of cloud computing in many business areas. In recent years this concept has seemingly also been introduced in the healthcare domain. At least, a continuously increasing number of articles and publications appears in the popular literature and is provided by healthcare IT companies, but also in the scientific literature cloud computing for healthcare applications is gaining attention.
When reviewing the large amount of most recent literature dealing with cloud approaches in healthcare it becomes obvious, that many reports are dealing with cloud-computing technologies as a replacement for grid computing in the OMICS-field, while other fields of application (e.g. health information systems, health information exchange or image processing and management) still seem to be underrepresented. In the popular literature the application of cloud computing for healthcare information system provision for example is often used as a buzz word, but real evidence on research in healthcare cloud computing (beside the big topic of OMICS) or even its successful and resource saving application is missing. Researchers have proposed cloud computing as a new business paradigm for biomedical information sharing [ 3 ]. Kuo asked “if cloud computing can benefit health services” [ 4 ] and described opportunities and challenges of healthcare cloud computing [ 5 ]. Ahuja and colleagues have recently tried to survey the current state of cloud computing in the healthcare domain [ 6 ]. However, their overview has by far neither been representative nor comprehensive (many of their limited number of 27 references were company website information or publications with a commercial background).
Thus, since currently no real overview on the application of cloud computing in healthcare exists, it is the objective of our scoping literature review, to uncover the current myth on healthcare cloud computing. It is our aim to provide a comprehensive overview on the existing literature and elicit the key messages of the current publications. Further, we want to identify “hot spots” within the healthcare domain (but outside of the OMICS area) where cloud computing concepts and applications have mostly been discussed. For the articles published as “cloud computing application for health care” we wanted to check if the typical cloud computing service models (software, platform or infrastructure as a service) as well as their respective deployment models (private, community, public or hybrid cloud) are differentiated. Finally, we wanted to verify, how far the buzz word “cloud computing” has really already achieved more than only the “conceptual design” and “challenges” state and entered into the status of routine daily application, hopefully even with measures on its proven value for the healthcare domain.
Thus, our review questions were:
- Does the existing literature provide enough evidence for the successful application of cloud computing in healthcare?
- What are the major application areas?
- Are particular types of cloud concepts (public clouds, private clouds or hybrid clouds) more dominant than others?
- Are particular cloud computing services (e.g. infrastructure as a service, software as a service, and platform as a service) more dominant than others?
- Is there evidence, that the benefits, advantages and cost savings, which are typically assigned to cloud computing, could already be realized in healthcare environments?
- What are the barriers, which still need to be overcome in order to make cloud computing a successful technology also in the healthcare domain?
Carrying out the review comprised the four stages of (1) collecting publications through a MEDLINE database search, (2) a first relevance screening to filter the results, (3) a review of the relevant papers and (4) a summarization of the content.
Within this review we consider the concept of healthcare to include all activities related to diagnosis, therapy and prevention of human diseases, or injuries, as well as clinical research and healthcare management. Publications on cloud computing for research in basic medical science (e.g. molecular medicine and genomics) however have not been considered.
Search strategy
We searched the MEDLINE database in July 2013 and conducted an updated MEDLINE literature research in December 2014 for the terms “cloud computing” and “cloud-based”. Further, articles were subsequently included based on references in the publications of this first search.
All references were imported into the literature management program EndNote. All results were screened for relevance against our inclusion criteria.
Selection of studies
The review team consisted of six researchers with expertise in medicine, computer science, medical informatics and statistics, working in groups of two. Each group was assigned one third of the papers in each round. Thus, each paper was reviewed independently by two reviewers. Conflicts between reviewers were resolved by short discussion rounds reaching a consensus.
At first, a relevance screening round based on the bibliographic data of a publication (type of publication, title, abstract, keywords) was conducted to remove obviously irrelevant papers. Details on this relevance screening are given in Additional file 1 .
Excluded were papers on clouds in a non-computing sense (e.g. scatter plot analyses, clouds in a meteorological context) as well as cloud-computing in non-healthcare related topics (e.g. clouds used for biological analyses or for veterinary medicine). For the remaining papers, full-texts were obtained. If full-text was not available the article was excluded.
In the next step, based on the available full texts, papers published in languages other than English, editorials, letters to the editor, commentaries and press articles were excluded as non-scientific and out of the scope of this review. Articles dealing with cloud computing in genomics without a concrete relevance for patient care were excluded as they were not in the scope of our review. Additional file 2 provides the eligibility screening form used in this full-text screening step.
For the remaining papers, the content has been extracted as described in the following section.
Full text screening and data extraction
The review protocol contained detailed instructions, inclusion/exclusion criteria, and a data extraction form (see Additional file 3 ). The data extraction form was handed to all reviewers in MS Office Excel 2010 format. This form included 14 closed and 8 open questions.
The closed questions captured e.g. the state of the described cloud computing system (i.e. theoretical, conceptual, prototype, successful), users addressed by the described system (e.g. physicians, patients, researchers), and the provider of the cloud (e.g. proprietary, i.e. self-constructed cloud computing solutions or commercially hosted solutions). Based upon NIST’s definition of cloud computing, its five essential characteristics (self-service, broad network access, resource pooling, rapid elasticity and measured service) [ 2 ] were checked for being mentioned by the authors. Besides advantages, also challenges were extracted, for example security concerns or dependencies on cloud providers.
Using open questions, the reviewers identified the main objective and the most important result of the article or of the described project, and in each case also summarized the specific usage of cloud computing. If mentioned, security concerns and countermeasures as well as cost considerations were noted. Finally, the definition of cloud computing, if it was used in a paper was collected.
Conducting this analysis of the articles enabled us to get an overview on the current state of research on cloud computing in healthcare and to collect the key messages of eligible publications.
Record selection and article type
Up to July 2013, 258 articles were found through literature research using the MEDLINE database. After the exclusion of one duplicate article and 63 articles, where title and abstract obviously illustrated that the contents of the article was from a completely different field, 194 remained for a cursory full text screening. Ten full texts were not available. 126 additional articles were removed during this step. 13 additional publications were identified from references of the screened literature, retrieved and included in the final analysis step. The first literature research thus resulted in 71 articles for the qualitative analysis. This literature research was updated in December 2014. During that research 200 further articles have been found in MEDLINE; 58 articles were removed due to their title and abstract. Of 21 articles the full text was not available; 90 further articles did not fit to the eligibility criteria. 31 new articles remained that were included in the qualitative analysis. Thus, in total 102 articles contributed to the subsequent qualitative synthesis. Of these 78 were journal papers, 24 were papers from conferences. The record selection process is shown in Figure 1 . Additional file 4 gives an overview of all 102 articles that were used for the qualitative synthesis and includes detailed results of the characterization of all eligible reviewed articles.

Scoping literature review flowchart.
Of the 102 articles only one has been published in the year 2008 and none in 2009. Seven articles have been published in 2010. From 2010 to 2011 the number of published articles concerning cloud computing in healthcare doubles up to 14 articles, and doubles again from 2011 to 2012 from 14 up to 29 articles. In 2013 27 articles have been published. Until December 2014 24 articles were identified–thus the trend seems not to be stable (Figure 2 ).

Yearly distribution of published articles.
Categorization of cloud computing research in healthcare: main domains
The final list of papers was screened again to identify any new topic complementing the MEDLINE result list. Each two reviewers independently tagged the articles in the qualitative synthesis with main domains included in the papers. The final set of topics was discussed by all reviewers and similar topics were grouped to one main topic. Finally the following six domains for the application of cloud computing to healthcare, sorted in descending order by the number of included articles, were identified (Figure 3 ):
- Telemedicine/Teleconsultation
- Medical Imaging
Public health and patients’ self-management
- Hospital management/clinical information systems
Secondary use of data

Cloud computing in healthcare–main domains.
Besides these categories we identified several articles that did not fit into one of the hot spots–these articles are explained in the “other domains” section.
In the following we describe the papers according to the identified categories, for more detailed information concerning MEDLINE articles’ content, please refer to the Additional file 4 .
Telemedicine/teleconsultation
Supporting communication and sharing data among stakeholders in healthcare is the most prominent domain including 34 articles. However, most publications describe just a typical telemedicine application when they report on the possibility to ubiquitously collect, access and share or analyze patient data from different hospitals or healthcare providers in dedicated health services networks.
Oshidori-Net2 for example is reported to be an “EPR and PACS sharing system” for six Japanese hospitals on an infrastructure which the authors call “server-based computing” and denote this to be cloud computing technology. The article further mentions that the server for this environment was built on virtual servers and virtual routers, but no further details on the cloud deployment or service model are given [ 7 ]. Similarly, Shih et al. [ 8 , 9 ] present a study in which 65 organ transplant healthcare professionals from China and Taiwan as well as 15 eHealth technology experts were questioned to identify pros and cons of so called “e-health documents” to be shared between institutions on a Web-platform. However, the article gives no information on why their proposal should be some type of cloud computing and not just a typical telemedicine platform for secure sharing of patient records for the respective organ transplant patients [ 8 , 9 ]. Rajkumar and Iyengar describe the concept of a Peer-to-Peer network to transfer medical resources like patient records and medical histories between diverse actors such as hospitals and ambulances in a medical emergency scenario [ 10 ]. In this scenario each hospital owns a community cloud to upload and share patient data with the nurse in the ambulance. A cloud application and an architecture test bed has been created, nevertheless the authors only present their concept and promise a reliable system to reduce the death rate in emergency care resulting from time delays during the patient transportation due to the missing opportunity to share important patient information with the hospital.
Also, Koufi et al. have named their concept “cloud emergency medical services” and provided a figure in which they depict their system components as infrastructure as a service, platform as a service and software as a service [ 11 ]. They further mention, that the system’s prototype implementation has been performed on a laboratory cloud computing infrastructure and that data are stored on multiple data centers in the cloud. Unfortunately, no further details on the specific type of cloud (private, community or public), the pay-for-use model or aspects of resource pooling with other tenants and rapid elasticity are given.
In another example Fujita et al. [ 12 ] called their implementation “Cloud Cardiology®”, mentioning, that “a cloud server enables to share ECG simultaneously inside and outside the hospital”. Nowhere in the further article itself, however, are any details presented why this server should really be a “cloud server” and not just a secured web-server for a telemedicine application, which provides a health information exchange platform in the internet.
Rao et al. propose a solution were also underserved, regions lacking infrastructure may benefit from cloud computing, without however illustrating in detail in which terms their approach should be a cloud application and not just a typical telemedicine service for rural areas [ 13 ]. Al-Zaiti et al. analyze the current problems and options for ECG transmission prior to hospitalization [ 14 ]. They see an option to standardize protocols used by different vendors and lower the investment cost for adopting the technology thanks to cloud services. One possible solution is presented by Fong and Chung [ 15 ] who describe a mobile cloud-based healthcare service by noncontact ECG monitoring. However, the software is described as client–server architecture implemented using standard web technologies and no cloud technologies are mentioned by the authors. Also Wang et al. [ 16 ] propose in their conceptual work a hybrid cloud computing environment to store data from personal health sensors worn at the body such as ECG sensors and to perform processing tasks. The purpose of the cloud is to accelerate computation intensive processing tasks by shifting them to the cloud server and therefore extend the battery life of mobile devices.
In contrast to the above examples, Hsieh and Hsu have presented a very comprehensive and detailed description of a 12-lead ECG telemedicine service based on cloud computing [ 17 ]. They have clearly described how the processing, visualization, management and e-learning services are deployed within the commercial Microsoft Azure cloud platform. They further present the reasons for adopting the Azure platform and the financial background of the implementation, based on the Azure pricing model with monthly costs directly related to CPU hours and GB storage used. As a second positive example we have identified the article of Hiden et al. who have described their development e-Science Central (a platform as a service which itself was built on an infrastructure as a service environment) [ 18 ]. Their article comprehensively illustrates not only the set of cloud services provided, which cover data storage services, but also service execution, workflow enactment and security. Finally as one of three case studies they present a medical pilot investigation (the MOVEeCloud project) where medical specialists assess the physical activity of patients based on data uploaded to the e-Science Central cloud by wearable accelerometers.
The improvement of the monitoring of discharged patients’ health-related quality of life and vital signs is the objective of caREMOTE, a prototype development of a cancer reporting and monitoring telemedicine system which is accessible by mobile devices [ 19 ]. For this prototype the cloud infrastructure was built on the Google App Engine (GAE) and data was stored in Google’s Big table technology. According to the authors, building such applications with GAEs sandbox technology leads to an isolation of the caREmote database within the cloud and secures the sensitive patient data from being violated. For a final routine application, this security aspect alone however, would by far not be sufficient. Therefore the authors intend to implement the Advanced Encryption Standard (AES) in a future version.
Similarily, Hussain et al. [ 20 ] implemented a system to use sensory data e.g. from smartphone sensors to detect activity patterns and ultimately lifestyle patterns. While the analysis was done on a local cluster of 4 host machines, the system is based on Hadoop as a typical big data technology which is easily scalable in clouds. Almashaqbeh el al. describe a cloud-based real-time remote health monitoring system (CHMS) which aims to integrate multi-hop sensor networks and cloud computing. However, the focus of the presentation is on routing the messages effectively (quality of service) through networked routers and computers and it therefore does not refer to any cloud or NIST characteristics [ 21 ].
The paper by Zao et al. puts a focus on telemonitoring in the neuroscience field [ 22 ]. The researchers present a prototypic online EEG-BCI (Brain Computer Interface) system based on wireless EEG headsets and mobile phones to predict users’ (patients, healthy persons) cognitive states in dynamic real-life situations. Cloud servers deliver the power to conduct semantic searches to find data segments matching with certain personal, environmental, and event specification used as a basis for the cognitive state prediction model.
Medical imaging
One of the second largest domains of use with 15 articles is medical imaging focusing on the storage, sharing and computation of images.
Kakadis [ 23 ] provides a more theoretical description of various aspects of cloud computing with a special focus on medical imaging. Computing intensive image processing, sharing/workflows and archiving are the three major application areas, security the major challenge. As a visionary paper it remains on a conceptual level and does not explicitly refer to implementations. Similarly, Gerard also motivates the utilization of cloud technologies in radiology in his extended outlook, if adequate service level agreements are in place to guarantee uptime and performance and security is granted [ 24 ].
A cloud-based Picture Archiving and Communication System (PACS) might enable the storage of medical images as “PACS-as-a-Service” [ 25 ] or even provide a highly flexible “radiology round-the-clock” [ 26 ]. Rostrom et al. [ 1 ] have built a proof-of-concept prototype to demonstrate that the secure exchange of images between a client and a DICOM server hosted in the Microsoft Azure cloud is possible. The development of a DICOM (Digital Imaging and Communications in Medicine) compliant bridge for easily sharing DICOM services across healthcare institutions supports the provision of medical imaging services across the different institutions [ 25 , 27 ]. Also an efficient transport of large image files between PACS and image analysis servers is under development [ 28 ]. Doukas [ 29 ] implemented an Android client to receive patient information and images from a central server that runs in an Amazon virtual machine and measured download times of images via 3G and WLAN. Besides the server being in the Internet, neither details on the particular cloud-features nor on data protection/safety are issues mentioned.
Especially computationally intensive tasks are predestined to be put in a cloud computing environment. Cloud computing with its ability to lease computing capacities can be a suitable solution due to its pay-for-use approach, its ubiquitous access to data and its elasticity [ 30 ]. Maratt [ 31 ] compared the accuracy and efficiency of templating as part of the preoperative planning for total hip arthroplasty between traditional printing and a digital SaaS. While the outcome confirms that digital templating is quite as good as traditional methods, the article does not focus on the cloud per se, but more on the medical outcome as prerequisite for the acceptance of digital service. Yoshida et al. [ 32 ] describe the implementation of a framework for distributed image processing and positively evaluated the performance gained by using more processing units. However, the evaluation used multi-core CPUs in a single machine and the transfer to cloud-environments is mentioned only as an additional conceptual possibility. Similarly, Qu et al. [ 33 ] evaluated five image texture analysis methods using a “CometCloud” called hybrid cloud-grid distribution framework. Despite the cloud features, the evaluation reported was performed on a local, grid-like cluster. In contrast, Meng et al. [ 34 ] implemented a cone-beam CT reconstruction algorithm using MapReduce and evaluated it on 10 to 200 Amazon cloud nodes experiencing a 1/n decrease of computing time.
Supporting research, Avila-Garcia [ 35 ] describes the objectives of a Microsoft-funded project to implement a virtual research environment to lower the barriers to cancer imaging. While the paper cites some grid frameworks and enlists some general features required by researchers, no explicit links to cloud technologies are given when describing the functions to be implemented.
Public Health is concerned with prevention, health promotion or improvement for individual citizens and patients but also for large population groups (epidemiology). Identically to the domain of medical imaging 15 articles belong to this domain.
Several papers include the idea that cloud computing might be used to support citizens and patients in managing their health status. Botts et al. [ 36 ] describe a pilot study named HealthATM which is a cloud-based personal health infrastructure to provide individuals from underserved population groups (i.e. people without health insurance) with instant access to their health information. The authors see cloud computing as a way to provide broad access to health data to population groups but do not explain how this highly scalable cloud architecture was implemented in detail, because the main focus of the paper was on the acceptance and usability of a personal electronic health records system in underserved populations.
The work of Piette et al. focusses on underserved patient groups as well. In two papers they describe how they created systems to inform underserved patient groups suffering from diabetes [ 37 ] resp. hypertension [ 38 ] with automated telephone calls to enable an improved self-management of the diseases. Although the authors mention that they use cloud computing to provide the application they do not differentiate between clouds and the Internet in general.
In their conference poster, Takeuchi et al. present a prototypic cloud-based system to store personal health and lifestyle data using mobile devices. In a cloud infrastructure they claim to have implemented data-mining technologies to extract individually important information such as lifestyle patterns. Although other persons like dietitians should have the possibility to add comments into the system it is not explained how data access in the cloud will be managed [ 39 ].
Similarly, the work of He et al. as well focusses on enabling citizens to manage their own health. They see cloud computing as a “component as a service” to develop a private healthcare cloud which should provide early warning of diseases [ 40 ]. Siddiqui et al. describe the concept of a Telecare Medical Information System (TMIS) which includes different medical services for patients and medical professionals such as a remote monitoring of physiological signals. The user should connect to the TMIS by using his/her smartphone and thus the smartphone needs to be equipped with authentication possibilities to ensure data privacy and data security. The authors propose a three-factor authentication (3FA) based on a dynamic cloud computing environment to enable the remote user authentication [ 41 ]. Van Gorp and Comuzzi discuss the prototype of MyPHRMachines where a cloud is used to deploy health-related data and the application software to view and analyze it in a personal health record system. After uploading their medical data to MyPHRMachines, patients can access them again from remote virtual machines that contain the right software to visualize and analyze them without any need for conversion. The patients should be able to can share their remote virtual machine session with selected caregivers [ 42 ].
Other projects are focused on specific user groups, such as the paper from Xu et al. [ 43 ] who worked on creating an automated cloud-based stress disorder monitor screening enabling patients suffering from Post-Traumatic Stress Disorder (PTSD) to monitor their progress during the treatment. According to the authors the so-called TPM (Tele-PTSD Monitor) system should be accessible via Public Switched Telephone Networks or via the Internet; latter might be realized using Amazon Elastic Compute Cloud. More information on the detailed cloud approach is not given to the reader.
Likewise, Su and Chiang describe IAServ (Intelligent Aging-in-place Home care Web Services) which is an electronic platform to provide healthcare services for elderly people at home. The objective of the platform is to prevent institutionalization of the users. Although the authors present an interesting architecture approach including an agent environment and a knowledge proceeding layer and explicitly mention the use of cloud computing services several times it remains unclear where a cloud computing system is used in the architecture of IAServ [ 44 ].
The work of Tseng and Wu as well focusses on enabling a healthy lifestyle of elderly people. They describe the prototype of iFit, which is a platform for the promotion of physical fitness to elder people through game-like activities. A so-called expert cloud is used to provide expert fitness diagnoses through a web service by receiving physiological data from the user and returning the corresponding fitness level and giving fitness suggestions to the user [ 45 ].
On a population level, Jalali et al. identified cloud computing as a solution to work with data of large populations by conceptualizing the use of virtual private clouds for public health reporting [ 46 ]. Price et al. worked on reducing execution time for epidemic analyses by using cloud structures [ 47 ]. Eriksson et al. describe a cloud-based architecture for simulating pandemic influenza outbreaks [ 48 ]. Ahnn et al. furthermore provide a theoretical paper on a way to create a cloud-based mobile health platform with a focus on energy efficiency [ 49 ].
Hospital management and clinical information systems
Another interesting field of cloud computing in healthcare described by 13 articles is the deployment of clinical information systems into clouds. Commercial HIS vendors (compare e.g. the CSC Health Cloud [ 3 ]) have started to propagate new managed HIS services for their customers and also offer infrastructure as a service on a monthly payment basis. According to Low and Chen the selection of such an outsourcing provider needs to be evaluated very well. They proposed a provider selection evaluation model based on the Fuzzy Delphi Method (FDM) and the Fuzzy Analytic Hierarchy Process (FAHP) and identified decision criteria such as system usefulness, ease of use and reliability, high service quality or professionalism of the outsourcing provider [ 50 ]. Yoo et al. have chosen a more conservative approach by establishing a private cloud within Seoul National University Bundang Hospital (Korea) based on virtualization technology, a virtual desktop infrastructure and 400 virtual machines, which supported easy and overall access to each of the hospital’s information systems from all devices throughout the hospital. For this implementation they performed a five year cost-benefit analysis and showed that their approach reached its break-even point in the fourth year of the investment [ 51 ].
Two publications [ 52 , 53 ] describe the environment of two Romanian hospital departments with two different clinical subsystems which are capable to exchange data between each other based on HL7 CDA. Even though the authors introduced their article with a general description of the different cloud deployment and service models, the remainder of the articles provides no evidence of cloud-use or requirement.
As the Malaysian government initiated a paradigm shift to use electronic hospital information and management systems (HIMS) cloud computing could be the method of choice to reduce the escalating costs of data storing and sharing according to Ratnam and Ramayah. Although the authors do not describe this cloud system in detail, they mention that a cloud platform using Microsoft Windows Azure was used as prototype architecture [ 54 ].
In China, Yao et al. [ 55 ] created a community cloud-based medical service delivery framework (CMSDF) to enable the exchange of resources between a large general hospital with its associated smaller healthcare institutions–so called Grassroot healthcare institutions being the smallest administrative level of medical institutions in China including for example community health service centers or rural clinics. In the prototype CMSDF a cloud-based Virtual Desktop Infrastructure is owned and managed by a large hospital which is able to share its medical software as SaaS with the Grassroot healthcare institutions. According to the author for the 34 cooperative sanatoriums that participated, 89.9% of investment and maintenance cost were saved because the smaller facilities had not to buy and host expensive software on their own.
Rodrigues et al. specifically address the risks of hosting electronic health records on cloud servers [ 56 ]. The authors conducted a review of papers about security and privacy issues which different cloud computing providers currently use for the development of their platforms. They emphasize that shifting health resources to cloud systems needs the consideration of several requirements regarding privacy and confidentiality of patient data and mention that an external company was needed to audit the cloud platform provider’s security mechanisms.
Seven papers describe applications for planning, managing or assessing therapeutic interventions.
Chang et al. [ 57 ] describe a website for access to information on drug compounds used in Traditional Chinese Medicine. In future, the iSMART portal shall provide genetic research features for drug research; however, until now only a webserver to the database exists publicly and no information on the cloud-specific development is given.
Dixon et al. describe a prototype of a clinical decision support system (CDS) that packages a patient’s data and sends it to a remote SaaS for analysis, i.e. rule application [ 58 ]; a comparison of the local assessments versus the remotely generated results are analyzed in [ 59 ]. While the service model for cloud computing seems fulfilled, no features of SaaS such as scalability or pay-per-use are mentioned. Another evaluation of a cloud-based decision support system for early recognition of sepsis is described by Amlad et al. [ 60 ]. An add-on to the Cerner EHR was used to continuously monitor patient to recognize possible outbreak of sepsis. While the system performed well, the added benefit of being cloud-based is not described.
A large part of the papers from this domain evaluate the performance gain when moving Monte Carlo simulations for radiation therapy planning into the cloud. Poole et al. [ 61 ] used the Amazon Cloud to simulate a clinical linear accelerator and experienced a 1/n reduction of computing time usage when up to 20 worker instances were instantiated. Similarly, Miras et al. [ 62 ] used Microsoft Azure with up to 64 virtual machines of different sizes to measure a speedup of up to 37x. A more complex calculation is performed by Na et al. [ 63 ] who uses Amazon cloud with up to 100 worker instances for a speedup of 10-14x. The difference in the speedup is caused by the ability to parallelize the algorithms and the overhead for worker management and data communication. Cost of routine use has been estimated by all three to be below or at par of an equivalent local hardware cluster. However, all three studies are limited as the use cases focused on the performance of the mathematical libraries outside real world applications.
Also the paper of Parsons et al. [ 64 ] includes a description of an Amazon cloud-based model for Monte Carlo simulation of radiation dose. They used a web application called VirtuaLinac to model radiation treatment components.
This domain includes articles describing cloud computing utilization for enabling secondary use of clinical data; e.g. for data analysis, text mining, or clinical research. Six papers belong to this domain.
Regola and Chawla discuss possibilities to store and share research health data and data from electronic health records in a cloud structure to reach an HIPAA (Health Insurance Portability and Accountability Act) complying environment [ 65 ]. For them, cloud computing offers the advantage of providing researchers with large computing resources. Data security can be achieved by providing proprietary cloud solutions where researchers can create their own customized networks and virtual servers.
Similarly, Chard et al. describe an approach to enable cloud-based services which should offer high scalability and HIPAA-compliant data security. They propose a cloud-based Software-as-a-Service NLP prototype to enable the extraction, procession, management, and comparison of medical data from several hospitals. Nevertheless, it does not become clear how data security should be achieved as-at the moment-the data in this cloud is not anonymous yet, but shall be accessible only to the particular data provider [ 66 ]. Also a cloud-based NLP service is described by Christoph et al. [ 67 ], here the free text is deidentified before put into the cloud. While the project described uses a community cloud, the OpenNebula-based implementation is said to run also in private or public scenarios. The main benefits of using cloud computing is in lowering the cost for processing data (no upfront investment, pay per use) and the managed services which enables the use of complex, computing intensive services by data providers with small IT departments.
Shen et al. describe generic standards-based services that can be transferred as virtual machines to other hospitals so that clinical pathways can be learned from order sets documented in EHRs. They mention that data mining models and results might be shared between different hospitals over a cloud-based server. Nevertheless the authors equal cloud computing with the Internet in general [ 68 ].
In the last article of this domain Rea et al. claim that they created a prototypic system that enables a cloud-based architecture to mine and normalize data for interchanging between hospitals [ 69 ]. The authors nevertheless do not explain how they face possible security and safety concerns when putting sensitive health data into a cloud, e.g. does this prototype include a private or a public cloud?
Other domains
The main topic of some papers could not be assigned to one of the other categories.
Doukas et al. describe an infrastructure for automated skin lesion classification to detect skin cancer in an early stage. This assessment system is based on mobile technologies used by patients–a cloud provides the essential data processing components for pattern recognition [ 70 ].
Shen et al. implemented a cloud bio-signal (e.g. electroencephalography, electrocardiograph) analysis system but it hard to identify where exactly the cloud component can be found in their system architecture [ 71 ]. Papakonstantinou et al. describe the prototype of a semantic wiki to support training in healthcare process management which allows cost savings, accelerated time to delivery, and offloaded maintenance [ 72 ].
Second Live as a virtual environment is mentioned in two publications. Garcia-Penalvo et al. describe an interesting training environment for ongoing and already skilled pharmacists in virtual worlds [ 73 ]. Their objective is that students and teachers get each an own avatar in the Second Life environment to practice and train laboratory work to assure a high education and work quality. In the authors’ conceptual paper cloud computing is thought to support the mechanisms of data recovery and analysis to proper evaluate the processes in Second Life. Also Stoicu-Tivadar et al. propose a medical education approach based on the Second Life environment. They describe an information system that provides training for medical students to treat patients using avatars. According to the authors cloud computing should be used to store data bases such as a medical guidelines database remotely but no further details on the use of clouds are given [ 74 ].
Medical students may profit from radiology cases provided for use on mobiles according to Balkman and Loehfelm [ 75 ]. They build a learning web-portal based on Googles App Engine which was perceived well, although the latency of bringing images to mobile devices is seen as a downside. In the end, a student must be evaluated by his performance. Ferenchick and Solomon have developed a mobile assessment tool (basically web based questionnaires) for observers to document proved student skills [ 76 ].
Another work that is not captured by the defined domains is dealing with mobile health applications that require data-intensive multimedia and security algorithms–the authors refer to the cloud-based provision as “Security as a Service” [ 77 ].
An interesting approach is the work of Nagata et al., who successfully implemented a cloud-based EHR for reducing adverse health consequences of the earthquake and nuclear disaster in Fukushima in 2011. To allow the emergency teams in Fukushima an efficient management and handling of patient data, access to EHRs for assessing patient data was provided in the form of software as service [ 78 ].
Furthermore, we found one article containing a short SWOT (strengths-weaknesses-opportunities-challenges) analysis of cloud computing in healthcare [ 4 ], and another dealing with implementation of strategic planning of organizations moving to a cloud [ 5 ]. Finally, two “overview articles” have been identified: one provided an overview on data privacy solutions in cloud computing [ 79 ] and secondly, the work of Ahuja et al. names several benefits and challenges of cloud computing [ 6 ]. Both such overview approaches however, are not performed systematically, but only include some major thoughts on cloud computing in healthcare, its advantages and disadvantages.
Implementation status
Our literature research revealed 22 theoretical papers that did not describe a specific cloud project but provided more common information on cloud computing in healthcare [ 4 - 6 , 8 , 14 , 23 , 24 , 26 , 49 - 52 , 56 , 79 - 87 ]. 12 articles include descriptions of basic conceptual work for cloud projects, but included no creation of a real system [ 16 , 30 , 35 , 46 , 53 , 54 , 63 , 77 , 88 - 92 ]. If applications are described they are usually in a prototype status [ 1 , 7 , 10 - 13 , 15 , 17 - 22 , 28 , 29 , 31 , 33 , 34 , 39 - 45 , 47 , 48 , 55 , 58 , 59 , 61 - 70 , 72 - 75 , 93 - 101 ]. Successful implementations of cloud systems in healthcare were only described in 13 of the 104 articles [ 9 , 25 , 27 , 32 , 36 - 38 , 51 , 57 , 60 , 71 , 78 , 102 ].
The distribution of the diverse implementation status is shown in Figure 4 .

Distribution of implementation status.
Definitions of cloud computing and NIST characteristics
Most articles rather describe features of the cloud than define it. These features include pay-as-you-go access to computing resources avoiding upfront investments and underutilizing private resources [ 1 , 21 , 25 , 30 , 31 , 53 , 62 , 65 ]. Scalability and flexibility are also presented as important characteristics for using cloud-based services, as system capabilities can easily adjust (scale) to momentary needs [ 1 , 25 , 50 , 62 , 82 , 84 ]. Availability and ubiquitous access are often mentioned [ 11 , 20 , 23 , 51 , 53 , 98 ] as well as the option to virtualize resources with distributed computing technologies, sometimes referred to as remote hosting [ 11 , 25 , 37 , 81 ].
These features can be linked to the five NIST characteristics of cloud-computing, i.e. rapid elasticity, followed by broad network access, resource pooling, on-demand self-service and measured service at least. But only eight publications directly cite NIST’s definition [ 4 , 5 , 18 , 23 , 24 , 40 , 52 , 55 , 63 , 67 , 75 , 81 , 83 , 85 , 100 ]. Thus, in most of the articles presenting cloud applications, details about the real deployment and service models remain unclear.
Some authors emphasize the more technical aspects of clouds: cloud computing is said to be more than just web-based applications, but also includes the necessary hardware, i.e. a physical network of many computers [ 5 , 26 , 77 , 84 ]. In five papers the cloud is even equated with the Internet in general [ 8 , 9 , 68 , 71 , 102 ].
Users and providers
Most cloud-based services are provided using own, proprietary infrastructure. If commercial services are used, Amazon services are applied most often: Elastic Computing Cloud (EC2) is referred to eleven times [ 13 , 34 , 48 , 61 , 63 , 66 , 72 , 90 , 93 , 97 ] and Amazon’s S3 service (Simple Storage Service) three times [ 25 , 29 , 40 ]. One application [ 65 ] used the Virtual Private Cloud (Amazon VPC) to provide more secure services. Cloud infrastructures by other vendors such as Microsoft or Google play a minor role [ 1 , 12 , 17 , 19 , 36 , 62 , 75 , 98 ].
End users of the applications described are from five main groups: physicians, other medical staff, patients, clinical researchers, and IT experts. Several articles describe physicians and other medical staff storing, sharing and analyzing patient data or medical images [ 1 , 7 - 9 , 11 - 13 , 17 , 19 , 25 , 27 - 29 , 31 , 36 , 46 , 51 , 58 , 69 , 71 , 78 , 88 , 90 , 92 , 94 , 96 , 102 ]. Also therapy planning or simulation of radiation dose might be enabled for physicians using cloud systems [ 64 ].
Patients are mainly focused in projects on personal healthcare management [ 16 , 19 , 22 , 29 , 36 - 43 , 49 , 96 ]. Medical researchers should be enabled to access large pools of data for medical research purposes [ 18 , 30 , 35 , 57 , 65 , 66 , 68 , 69 ], whereas programmers and hospital IT staff should be enabled to work on the creation of cloud-based solutions [ 8 , 34 , 53 , 62 , 93 , 95 ].
Challenges of cloud computing in healthcare
Three types of concerns using cloud computing in healthcare could be identified: safety/security of data as a threat to privacy, reliability and transparency of data handling by third parties, and lack of experience or evidence of a new technology.
First, in our literature we found that many authors mentioned data privacy and data confidentiality concerns. There is the fear that unauthorized persons might access sensible medical data in a cloud [ 1 , 5 , 13 , 18 , 24 , 25 , 28 , 29 , 31 , 46 , 51 , 52 , 63 , 65 - 67 , 72 , 81 , 83 , 85 , 88 , 94 , 96 ] which might hurt confidentiality of sensitive data about patients, therapies or physicians [ 41 , 103 ].
It is especially important that data security, privacy and confidentiality are focused [ 65 ] if handling of sensitive health data is outsourced to a commercial cloud, which means “that a third party now has control over the cloud-hosted area” [ 56 ]. Rodrigues et al. state that “cloud-based EHR must maintain the same level of data security as data stored in the servers of the health care provider” [ 56 ], but do not illustrate how this should be achieved.
In the US the Department of Health & Human Services has passed the Health Insurance Portability and Accountability Act (HIPAA) in 1996 which includes national standards for transactions in electronic healthcare concerning data privacy and security [ 104 ]. These standards provide a framework which should be considered when designing cloud services [ 1 , 63 , 65 , 66 , 83 ].
Many examples for improving data privacy and reducing confidentiality risks by authentication and authorization mechanisms are described [ 1 , 6 , 13 , 17 , 18 , 25 , 51 , 66 , 83 , 88 , 94 ]. For example, secure transmission protocols such as PCoIP could be employed, special security certificates could be utilized [ 66 ], access control lists (ACLs) can identify users’ role and the actions permitted [ 13 , 18 ], and licenses or electronic keys are handed over to authorized cloud users such as patients or physicians [ 88 ]. Further, a digital signature can ensure that data was entered or sent by the acclaimed person [ 56 ].
Data encryption is as well important to ensure data privacy [ 1 , 6 , 13 , 17 , 26 , 48 , 83 , 102 ]. Standardized encryption algorithms might be used [ 63 , 102 ] as well as secured data transmission using HTTPS [ 53 , 96 ]. Data encryption nevertheless can be problematic in emergency situations when physicians need instant access to patient data in a cloud and an access key is missing [ 58 ].
In principle, as few data as possible shall be put into the cloud [ 58 ]. Often it has to be anonymized before leaving an organization [ 30 , 65 ]. Sometimes it is possible to store identifiable data in separate entities to separate concerns [ 25 ]. Nevertheless, organizations often need to inform their patients before migrating their data to a third-party cloud computing provider [ 56 ]. The theoretical paper of Wang et al. focusses on the problem that cloud server providers might not be trustworthy (e.g. he might delete of modify some parts of the stored medical records). According to the authors an independent committee should be built to recover the original medical records from the cloud in the case of untrusted cloud providers [ 87 ].
Second, fears on technical issues exist when trying to implement a secure computing environment. Data might be lost due to technical problems with the cloud system [ 17 , 25 , 40 , 83 ] or vice versa, sensitive data cannot be fully deleted anymore once put into a remote cloud, leaving data in form of a fuzzy cloud structure [ 5 , 83 ]. In general, there is a fear of dependence on a cloud provider: a loss of control over their data [ 23 , 25 , 82 ]. Using audit trails might be a possibility to better control the use of a cloud system or facilitating data recovery [ 26 ]. This is why it is important to select only partners for outsourcing and cloud computing which can prove their security measures [ 56 , 89 , 100 ] and make handling of data completely transparent for the data owners [ 56 ]. Additionally, service-level agreements should be established between the customer organization and the cloud provider concerning data encryption and safety policies [ 23 , 100 ]. On the other hand clouds can even be utilized to store data using resource intensive security algorithms as kind of “Security-as-a-Service” [ 77 ].
Third, there are also concerns about the maturity of the cloud service–there might still be lack of evidence of successful cloud implementation in healthcare [ 4 , 83 ]. With regards to the economic advantages, Schweitzer proposes to conduct an economic analysis to ensure that savings through cloud computing are not overestimated because of hidden costs (e.g. cost for in-house IT support) [ 83 ].
Since for this relatively new domain with just emerging evidence standardized keywords and subject headings have yet not been well established, we decided on conducting a scoping review, as this approach is well suited for clarifying a complex concept and refine subsequent research inquiries [ 105 ]. While this approach yielded an overview on the status of cloud-computing in healthcare and identified the hot-topics, a systematic follow-up review could dig deeper into specific areas. Our review could help to focus on specific topics and to cope with the pace of publications.
The analysis of papers with regards to cloud features was hampered by the lack of information provided by the respective authors. Too often cloud was used synonymously with “Internet-based” or “running in a virtual machine” or “potentially scalable to a cloud” lacking any evidence of the real benefits. From the list of papers reviewed only eight papers refer explicitly to the NIST’s cloud computing definition itself. A large part of the papers do not even try to give a definition of cloud computing in general or describe in more detail what particularly makes their system to a cloud computing application. This is why we also conclude that future publications should more explicitly state their position with regards to the NIST characteristics.
Our findings may be limited by using MEDLINE as the main database as many publications especially in non-scientific media present cloud-based applications from a more practical or operational point of view. Searching the general Internet for cloud computing in healthcare reveals a very large number of hits of various kinds and qualities. Numerous cloud projects and offerings have not been scientifically published or evaluated. For example, CareCloud is a cloud-based software application including a complete infrastructure to document and facilitate caring processes in a hospital [ 106 ]; Box is a content sharing company which lately extends its cloud-based services to storage data to the healthcare sector enabling exchange of medical data between several physicians [ 107 ]. Well-known cloud services in health care are Microsoft’s Health Vault, a cloud-based platform to store and maintain health and fitness data [ 108 ] or the discontinued of Google Health service [ 109 ].
Of course, cloud computing is a hot topic and new papers are constantly published. Since 2010 the number of articles on cloud computing in healthcare has doubled almost every year. So the current review can only be a snapshot of a current state. However, comparing the publications date ranges of the topics shows no shift in the areas of research. A limitation is also that applications using cloud features may not be published with a title, abstract or keywords containing the word “cloud” and are thus not fitting our inclusion criteria.
The aim of this review was to get an overview on the status of cloud-computing in healthcare and to identify areas of interest beyond typical “OMICS” topics. We found that especially resource intensive (e.g. medical imaging) and communication intensive areas such as various kinds of “tele-”applications are predestined for cloud computing use.
Considering our research objectives, we were able to a) provide a comprehensive overview on the existing literature and elicit the key messages of the current publications and b) identify the “hot spots” within the healthcare domain where cloud computing concepts and applications have mostly been discussed.
The question, if the buzz word “cloud computing” has really already achieved more than just the “conceptual design” and “challenges” state and entered into the status of routine daily application still needs to be negated. Only 14 of the 102 publications have described successful applications. The vast majority of papers still was in an early prototype stage or only described potential options, challenges and risks of cloud services for the healthcare domain, but no actual application.
Thus, even though from 2010 to 2012 the number of articles on cloud computing in healthcare has doubled every year we had to realize, that many publications do not reference the characteristics of cloud computing as defined by NIST [ 2 ]. A large part of the papers do not even try to give a definition of cloud computing in general or describe in more detail what particularly makes their system to a cloud computing application.
It appeared to us, that many researchers do already declare their application as a cloud computing application, if only the two features of broad network access for data sharing among different stakeholders and data access from everywhere are given. Such type of applications, however, have already been implemented for a long time and–as long as the scenario has focused on supporting patient diagnostics and therapy–such approaches are typically named telemedicine applications, health information exchange or personal electronic health records.
In our opinion, an application which really enhances its provision by means of cloud computing should explicitly describe the cloud-specific characteristics of their application following the NIST definitions, such as rapid elasticity or measured service where a pay-per-use model supersedes upfront investments. Resource pooling helps organizations to consolidate and simplify infrastructure services and continue existing trends in virtualization. While in the consumer market on demand self-services are often used, in healthcare environments they only seem to play a minor role. Authors should also illustrate how this new technology/business model makes their application more cost effective than without cloud technology.
Further, if cloud computing is a major feature of a healthcare application, we recommend that in future publications, authors do describe the particular deployment model chosen (which often also relates to a description of data privacy measures applied, being very important for sensitive personal health data) and also which particular type of cloud service is applied. In too many of the recent publications those descriptions were missing and the impression remained, that authors often called a typical internet-/web-based telemedicine application now a cloud application, just because cloud computing is a current buzzword.
Acknowledgements
The work has been co-financed by the German Federal Ministry of Economics and Technology (BMWi) in the Trusted Cloud Initiative (Grant No FKZ 01MD11009). We acknowledge support by Deutsche Forschungsgemeinschaft and Friedrich-Alexander-Universität Erlangen-Nürnberg within the funding programme Open Access Publishing.
Abbreviations
Additional files.
Relevance screening form on basis of title and abstract. Shows how the large pool of MEDLINE articles found by keyword research was screened according to title and abstract.
Eligibility screening form on basis of full-text screening. Shows how the remained articles after the relevance screening where further screened on basis of eligibility criteria.
Characterization form on basis of full-text analysis. Shows how the content of the articles found eligible where characterized into several fields of interest.
Results from MEDLINE article analysis (n=102). Includes detailed results of the characterization of all eligible reviewed articles.
Competing interests
The authors declare that they have no competing interests.
Authors’ contributions
LG carried out the literature research and lead the study selection and screening process. FK, DT, IE, JC, IL, LG, and MS analyzed and categorized the identified articles. HUP, MS, and LG worked on drafting the manuscript. All authors read and approved the final manuscript.
Contributor Information
Lena Griebel, Email: [email protected] .
Hans-Ulrich Prokosch, Email: [email protected] .
Felix Köpcke, Email: [email protected] .
Dennis Toddenroth, Email: [email protected] .
Jan Christoph, Email: [email protected] .
Ines Leb, Email: [email protected] .
Igor Engel, Email: [email protected] .
Martin Sedlmayr, Email: [email protected] .
cloud computing Recently Published Documents
Total documents.
- Latest Documents
- Most Cited Documents
- Contributed Authors
- Related Sources
- Related Keywords
Simulation and performance assessment of a modified throttled load balancing algorithm in cloud computing environment
<span lang="EN-US">Load balancing is crucial to ensure scalability, reliability, minimize response time, and processing time and maximize resource utilization in cloud computing. However, the load fluctuation accompanied with the distribution of a huge number of requests among a set of virtual machines (VMs) is challenging and needs effective and practical load balancers. In this work, a two listed throttled load balancer (TLT-LB) algorithm is proposed and further simulated using the CloudAnalyst simulator. The TLT-LB algorithm is based on the modification of the conventional TLB algorithm to improve the distribution of the tasks between different VMs. The performance of the TLT-LB algorithm compared to the TLB, round robin (RR), and active monitoring load balancer (AMLB) algorithms has been evaluated using two different configurations. Interestingly, the TLT-LB significantly balances the load between the VMs by reducing the loading gap between the heaviest loaded and the lightest loaded VMs to be 6.45% compared to 68.55% for the TLB and AMLB algorithms. Furthermore, the TLT-LB algorithm considerably reduces the average response time and processing time compared to the TLB, RR, and AMLB algorithms.</span>
An improved forensic-by-design framework for cloud computing with systems engineering standard compliance
Reliability of trust management systems in cloud computing.
Cloud computing is an innovation that conveys administrations like programming, stage, and framework over the web. This computing structure is wide spread and dynamic, which chips away at the compensation per-utilize model and supports virtualization. Distributed computing is expanding quickly among purchasers and has many organizations that offer types of assistance through the web. It gives an adaptable and on-request administration yet at the same time has different security dangers. Its dynamic nature makes it tweaked according to client and supplier’s necessities, subsequently making it an outstanding benefit of distributed computing. However, then again, this additionally makes trust issues and or issues like security, protection, personality, and legitimacy. In this way, the huge test in the cloud climate is selecting a perfect organization. For this, the trust component assumes a critical part, in view of the assessment of QoS and Feedback rating. Nonetheless, different difficulties are as yet present in the trust the board framework for observing and assessing the QoS. This paper talks about the current obstructions present in the trust framework. The objective of this paper is to audit the available trust models. The issues like insufficient trust between the supplier and client have made issues in information sharing likewise tended to here. Besides, it lays the limits and their enhancements to help specialists who mean to investigate this point.
Cloud Computing Adoption in the Construction Industry of Singapore: Drivers, Challenges, and Strategies
An extensive review of web-based multi granularity service composition.
The paper reviews the efforts to compose SOAP, non-SOAP and non-web services. Traditionally efforts were made for composite SOAP services, however, these efforts did not include the RESTful and non-web services. A SOAP service uses structured exchange methodology for dealing with web services while a non-SOAP follows different approach. The research paper reviews the invoking and composing a combination of SOAP, non-SOAP, and non-web services into a composite process to execute complex tasks on various devices. It also shows the systematic integration of the SOAP, non-SOAP and non-web services describing the composition of heterogeneous services than the ones conventionally used from the perspective of resource consumption. The paper further compares and reviews different layout model for the discovery of services, selection of services and composition of services in Cloud computing. Recent research trends in service composition are identified and then research about microservices are evaluated and shown in the form of table and graphs.
Integrated Blockchain and Cloud Computing Systems: A Systematic Survey, Solutions, and Challenges
Cloud computing is a network model of on-demand access for sharing configurable computing resource pools. Compared with conventional service architectures, cloud computing introduces new security challenges in secure service management and control, privacy protection, data integrity protection in distributed databases, data backup, and synchronization. Blockchain can be leveraged to address these challenges, partly due to the underlying characteristics such as transparency, traceability, decentralization, security, immutability, and automation. We present a comprehensive survey of how blockchain is applied to provide security services in the cloud computing model and we analyze the research trends of blockchain-related techniques in current cloud computing models. During the reviewing, we also briefly investigate how cloud computing can affect blockchain, especially about the performance improvements that cloud computing can provide for the blockchain. Our contributions include the following: (i) summarizing the possible architectures and models of the integration of blockchain and cloud computing and the roles of cloud computing in blockchain; (ii) classifying and discussing recent, relevant works based on different blockchain-based security services in the cloud computing model; (iii) simply investigating what improvements cloud computing can provide for the blockchain; (iv) introducing the current development status of the industry/major cloud providers in the direction of combining cloud and blockchain; (v) analyzing the main barriers and challenges of integrated blockchain and cloud computing systems; and (vi) providing recommendations for future research and improvement on the integration of blockchain and cloud systems.
Cloud Computing and Undergraduate Researches in Universities in Enugu State: Implication for Skills Demand
Cloud building block chip for creating fpga and asic clouds.
Hardware-accelerated cloud computing systems based on FPGA chips (FPGA cloud) or ASIC chips (ASIC cloud) have emerged as a new technology trend for power-efficient acceleration of various software applications. However, the operating systems and hypervisors currently used in cloud computing will lead to power, performance, and scalability problems in an exascale cloud computing environment. Consequently, the present study proposes a parallel hardware hypervisor system that is implemented entirely in special-purpose hardware, and that virtualizes application-specific multi-chip supercomputers, to enable virtual supercomputers to share available FPGA and ASIC resources in a cloud system. In addition to the virtualization of multi-chip supercomputers, the system’s other unique features include simultaneous migration of multiple communicating hardware tasks, and on-demand increase or decrease of hardware resources allocated to a virtual supercomputer. Partitioning the flat hardware design of the proposed hypervisor system into multiple partitions and applying the chip unioning technique to its partitions, the present study introduces a cloud building block chip that can be used to create FPGA or ASIC clouds as well. Single-chip and multi-chip verification studies have been done to verify the functional correctness of the hypervisor system, which consumes only a fraction of (10%) hardware resources.
Study On Social Network Recommendation Service Method Based On Mobile Cloud Computing
Cloud-based network virtualization in iot with openstack.
In Cloud computing deployments, specifically in the Infrastructure-as-a-Service (IaaS) model, networking is one of the core enabling facilities provided for the users. The IaaS approach ensures significant flexibility and manageability, since the networking resources and topologies are entirely under users’ control. In this context, considerable efforts have been devoted to promoting the Cloud paradigm as a suitable solution for managing IoT environments. Deep and genuine integration between the two ecosystems, Cloud and IoT, may only be attainable at the IaaS level. In light of extending the IoT domain capabilities’ with Cloud-based mechanisms akin to the IaaS Cloud model, network virtualization is a fundamental enabler of infrastructure-oriented IoT deployments. Indeed, an IoT deployment without networking resilience and adaptability makes it unsuitable to meet user-level demands and services’ requirements. Such a limitation makes the IoT-based services adopted in very specific and statically defined scenarios, thus leading to limited plurality and diversity of use cases. This article presents a Cloud-based approach for network virtualization in an IoT context using the de-facto standard IaaS middleware, OpenStack, and its networking subsystem, Neutron. OpenStack is being extended to enable the instantiation of virtual/overlay networks between Cloud-based instances (e.g., virtual machines, containers, and bare metal servers) and/or geographically distributed IoT nodes deployed at the network edge.
Export Citation Format
Share document.
Research on the Application of Cloud Computing Technology in Computer Data Processing
Ieee account.
- Change Username/Password
- Update Address
Purchase Details
- Payment Options
- Order History
- View Purchased Documents
Profile Information
- Communications Preferences
- Profession and Education
- Technical Interests
- US & Canada: +1 800 678 4333
- Worldwide: +1 732 981 0060
- Contact & Support
- About IEEE Xplore
- Accessibility
- Terms of Use
- Nondiscrimination Policy
- Privacy & Opting Out of Cookies
A not-for-profit organization, IEEE is the world's largest technical professional organization dedicated to advancing technology for the benefit of humanity. © Copyright 2024 IEEE - All rights reserved. Use of this web site signifies your agreement to the terms and conditions.
A deep analysis of nature-inspired and meta-heuristic algorithms for designing intrusion detection systems in cloud/edge and IoT: state-of-the-art techniques, challenges, and future directions
- Published: 26 April 2024
Cite this article
- Wengui Hu 1 ,
- Qingsong Cao 2 ,
- Mehdi Darbandi 3 &
- Nima Jafari Navimipour 4 , 5
The number of cloud-, edge-, and Internet of Things (IoT)-based applications that produce sensitive and personal data has rapidly increased in recent years. The IoT is a new model that integrates physical objects and the Internet and has become one of the principal technological evolutions of computing. Cloud computing is a paradigm for centralized computing that gathers resources in one place and makes them available to consumers via the Internet. Despite the vast array of resources that cloud computing offers, real-time mobile applications might not find it acceptable because it is typically located far from users. However, in applications where low latency and high dependability are required, edge computing—which disperses resources to the network edge—is becoming more and more popular. Though it has less processing power than traditional cloud computing, edge computing offers resources in a decentralized way that can react to customers' needs more quickly. There has been a sharp increase in attackers stealing data from these applications since the data is so sensitive. Thus, a powerful Intrusion Detection System (IDS) that can identify intruders is required. IDS are essential for the cybersecurity of the IoT, cloud, and edge architectures. Investigators have mostly embraced the use of deep learning algorithms as a means of protecting the IoT environment. However, these techniques have some issues with computational complexity, long processing times, and poor precision. Feature selection approaches can be utilized to overcome these problems. Optimization methods, including bio-inspired algorithms, are applied as feature selection approaches to enhance the classification accuracy of IDS systems. Based on the cited sources, it appears that no study has looked into these difficulties in depth. This research thoroughly analyzes the current literature on intrusion detection and using nature-inspired algorithms to safeguard IoT and cloud/edge settings. This article examines pertinent analyses and surveys on the aforementioned subjects, dangers, and outlooks. It also examines many frequently used algorithms in the development of IDSs used in IoT security. The findings demonstrate their efficiency in addressing IoT and cloud/edge ecosystem security issues. Moreover, it has been shown that the methods put out in the literature might improve IDS security and dependability in terms of precision and execution speed.
This is a preview of subscription content, log in via an institution to check access.
Access this article
Price includes VAT (Russian Federation)
Instant access to the full article PDF.
Rent this article via DeepDyve
Institutional subscriptions
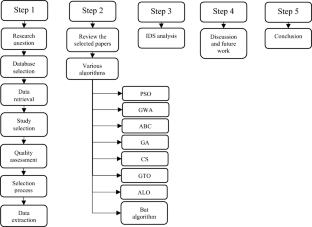

Data availability
Enquiries about data availability should be directed to the authors.
Balasubramanian, V., et al.: Low-latency vehicular edge: a vehicular infrastructure model for 5G. Simul. Model. Pract. Theory 98 , 101968 (2020)
Article Google Scholar
Al Ridhawi, I., et al.: A profitable and energy-efficient cooperative fog solution for IoT services. IEEE Trans. Ind. Inform. 16 (5), 3578–3586 (2019)
Liu, C., et al.: Robust online tensor completion for IoT streaming data recovery. IEEE Trans. Neural Netw. Learn. Syst. 34 (12), 10178–10192 (2022)
Article MathSciNet Google Scholar
Guo, Z., et al.: A security evaluation model for multi-source heterogeneous systems based on IoT and edge computing. Clust. Comput. 26 , 1–15 (2021)
Google Scholar
Almiani, M., et al.: Deep recurrent neural network for IoT intrusion detection system. Simul. Model. Pract. Theory 101 , 102031 (2020)
Zhang, G., Navimipour, N.J.: A comprehensive and systematic review of the IoT-based medical management systems: applications, techniques, trends and open issues. Sustain. Cities Soc. 82 , 103914 (2022)
Heidari, A., Jabraeil Jamali, M.A.: Internet of Things intrusion detection systems: a comprehensive review and future directions. Clust. Comput. 26 (6), 3753–3780 (2023)
Cheng, B., et al.: Situation-aware IoT service coordination using the event-driven SOA paradigm. IEEE Trans. Netw. Serv. Manag. 13 (2), 349–361 (2016)
Jiang, Y., Li, X.: Broadband cancellation method in an adaptive co-site interference cancellation system. Int. J. Electron. 109 (5), 854–874 (2022)
Sforzin, A., et al.: RPiDS: raspberry Pi IDS—a fruitful intrusion detection system for IoT. In: 2016 International IEEE Conferences on Ubiquitous Intelligence and Computing, Advanced and Trusted Computing, Scalable Computing and Communications, Cloud and Big Data Computing, Internet of People, and Smart World Congress (UIC/ATC/ScalCom/CBDCom/IoP/SmartWorld), 2016. IEEE (2016)
Heidari, A., et al.: Deep Q-Learning technique for offloading offline/online computation in blockchain-enabled green IoT-Edge scenarios. Appl. Sci. 12 (16), 8232 (2022)
Sefati, S., Navimipour, N.J.: A QoS-aware service composition mechanism in the Internet of Things using a hidden-Markov-model-based optimization algorithm. IEEE Internet Things J. 8 (20), 15620–15627 (2021)
Darbandi, M.: Kalman filtering for estimation and prediction servers with lower traffic loads for transferring high-level processes in cloud computing . Int. J. Technol. Innov. Res. 23 (1), 10–20 (2017). ISSN 2321-1814
Sun, G., et al.: Live migration for multiple correlated virtual machines in cloud-based data centers. IEEE Trans. Serv. Comput. 11 (2), 279–291 (2015)
Jannat, M.K.A., et al.: Efficient Wi-Fi-based human activity recognition using adaptive antenna elimination. IEEE Access 11 , 105440–105454 (2023)
Yan, A., et al.: FeMPIM: a FeFET-based multifunctional processing-in-memory cell. IEEE Trans. Circuits Syst. II (2023). https://doi.org/10.1109/TCSII.2023.3331267
Xie, Y., et al.: A two-stage estimation of distribution algorithm with heuristics for energy-aware cloud workflow scheduling. IEEE Trans. Serv. Comput. 16 , 4183–4197 (2023)
Hajimirzaei, B., Navimipour, N.J.: Intrusion detection for cloud computing using neural networks and artificial bee colony optimization algorithm. ICT Express 5 (1), 56–59 (2019)
Darbandi, M.: Proposing new intelligent system for suggesting better service providers in cloud computing based on Kalman filtering . Int. J. Technol. Innov. Res. 24 (1), 1–9 (2017). ISSN 2321-1814
Darbandi, M.: Proposing new intelligence algorithm for suggesting better services to cloud users based on Kalman filtering . J. Comput. Sci. Appl. 5(1), 11–16 (2017). ISSN 2328-7268.
Xiao, Z., et al.: Multi-objective parallel task offloading and content caching in D2D-aided MEC networks. IEEE Trans. Mob. Comput. 22 (11), 6599–6615 (2022)
Ghanbari, Z., et al.: The applications of the routing protocol for low-power and lossy networks (RPL) on the Internet of Mobile Things. Int. J. Commun. Syst. 35 (14), e5253 (2022)
Wang, S., et al.: Blockchain-empowered distributed multi-camera multi-target tracking in edge computing. IEEE Trans. Ind. Inform. 20 (1), 369–379 (2023)
Cao, B., et al.: Large-scale many-objective deployment optimization of edge servers. IEEE Trans. Intell. Transp. Syst. 22 (6), 3841–3849 (2021)
Cao, B., et al.: Mobility-aware multiobjective task offloading for vehicular edge computing in digital twin environment. IEEE J. Sel. Areas Commun. 41 (10), 3046–3055 (2023)
Shang, M., Luo, J.: The Tapio decoupling principle and key strategies for changing factors of Chinese urban carbon footprint based on cloud computing. Int. J. Environ. Res. Public Health 18 (4), 2101 (2021)
Cao, B., et al.: Edge-cloud resource scheduling in space–air–ground-integrated networks for Internet of Vehicles. IEEE Internet Things J. 9 (8), 5765–5772 (2021)
Tseng, L., et al.: Blockchain-based database in an IoT environment: challenges, opportunities, and analysis. Clust. Comput. 23 , 2151–2165 (2020)
Li, C., et al.: Efficient privacy-preserving in IoMT with blockchain and lightweight secret sharing. IEEE Internet Things J. 10 (24), 22051–22064 (2023)
Liang, W., Ji, N.: Privacy challenges of IoT-based blockchain: a systematic review. Clust. Comput. 25 (3), 2203–2221 (2022)
Yan, A., et al.: Designs of BCD adder based on excess-3 code in quantum-dot cellular automata. IEEE Trans. Circuits Syst. II 70 (6), 2256–2260 (2023)
Yan, A., et al.: Two double-node-upset-hardened flip-flop designs for high-performance applications. IEEE Trans. Emerg. Top. Comput. 11 , 1070–1081 (2023)
Khalid, U., et al.: A decentralized lightweight blockchain-based authentication mechanism for IoT systems. Clust. Comput. 23 (3), 2067–2087 (2020)
Hamza, A., Gharakheili, H.H., Sivaraman, V.: Combining MUD policies with SDN for IoT intrusion detection. In: Proceedings of the 2018 Workshop on IoT Security and Privacy, 2018 (2018)
Cao, B., et al.: A many-objective optimization model of Industrial Internet of Things based on private blockchain. IEEE Netw. 34 (5), 78–83 (2020)
Yan, A., et al.: Novel low cost, double-and-triple-node-upset-tolerant latch designs for nano-scale CMOS. IEEE Trans. Emerg. Top. Comput. 9 (1), 520–533 (2018)
Li, H., et al.: Public-key authenticated encryption with keyword search supporting constant trapdoor generation and fast search. IEEE Trans. Inf. Forensics Secur. 18 , 396–410 (2022)
Otoum, Y., Liu, D., Nayak, A.: DL-IDS: a deep learning-based intrusion detection framework for securing IoT. Trans. Emerg. Telecommun. Technol. 33 (3), e3803 (2022)
Yang, K., et al.: Deep network analyzer (DNA): a big data analytics platform for cellular networks. IEEE Internet Things J. 4 (6), 2019–2027 (2016)
Zarpelão, B.B., Miani, R.S.: Detection in internet of things. J. Netw. Comput. Appl. 84 , 25–37 (2017)
Verma, A., Ranga, V.: Machine learning based intrusion detection systems for IoT applications. Wirel. Pers. Commun. 111 , 2287–2310 (2020)
Heidari, A., Navimipour, N.J., Unal, M.: A secure intrusion detection platform using blockchain and radial basis function neural networks for Internet of drones. IEEE Internet Things J. 10 (10), 8445–8454 (2023)
Alhowaide, A., Alsmadi, I., Tang, J.: Ensemble detection model for IoT IDS. Internet Things 16 , 100435 (2021)
Smys, S., Basar, A., Wang, H.: Hybrid intrusion detection system for Internet of Things (IoT). J. ISMAC 2 (04), 190–199 (2020)
Lyu, T., et al.: Source selection and resource allocation in wireless powered relay networks: an adaptive dynamic programming based approach. IEEE Internet Things J. (2023). https://doi.org/10.1109/JIOT.2023.3321673
Aljawarneh, S., Aldwairi, M., Yassein, M.B.: Anomaly-based intrusion detection system through feature selection analysis and building hybrid efficient model. J. Comput. Sci. 25 , 152–160 (2018)
Kareem, S.S., et al.: An effective feature selection model using hybrid metaheuristic algorithms for IoT intrusion detection. Sensors 22 (4), 1396 (2022)
Xuemin, Z., et al.: Resource-constrained and socially selfish-based incentive algorithm for socially aware networks. J. Signal Process. Syst. 95 (12), 1439–1453 (2023)
Qi, M., et al.: Multi-region nonuniform brightness correction algorithm based on L-channel gamma transform. Secur. Commun. Netw. 2022 , 2675950 (2022)
Li, Q.-K., et al.: H ∞ consensus for multiagent-based supply chain systems under switching topology and uncertain demands. IEEE Trans. Syst. Man Cybern. Syst. 50 (12), 4905–4918 (2018)
Liu, X., Lou, S., Dai, W.: Further results on “System identification of nonlinear state-space models.” Automatica 148 , 110760 (2023)
Di, Y., et al.: A maneuvering target tracking based on fastIMM-extended Viterbi algorithm. Neural Comput. Appl. (2023). https://doi.org/10.1007/s00521-023-09039-1
Zheng, W., et al.: Design of a modified transformer architecture based on relative position coding. Int. J. Comput. Intell. Syst. 16 (1), 168 (2023)
Zheng, Y., et al.: Recognition and depth estimation of ships based on binocular stereo vision. J. Mar. Sci. Eng. 10 (8), 1153 (2022)
Yan, A., et al.: HITTSFL: design of a cost-effective HIS-Insensitive TNU-Tolerant and SET-Filterable latch for safety-critical applications. In: 2020 57th ACM/IEEE Design Automation Conference (DAC), 2020. IEEE (2020)
Zhou, G., et al.: PMT gain self-adjustment system for high-accuracy echo signal detection. Int. J. Remote Sens. 43 (19–24), 7213–7235 (2022)
Zhou, G., et al.: An RF amplifier circuit for enhancement of echo signal detection in bathymetric LiDAR. IEEE Sens. J. 22 (21), 20612–20625 (2022)
Kooktapeh, Z.G., et al.: In the line of fire: a systematic review and meta-analysis of job burnout among nurses. (2023). arXiv preprint arXiv:2312.14853
Liu, J., et al.: Research on intrusion detection based on particle swarm optimization in IoT. IEEE Access 9 , 38254–38268 (2021)
Kan, X., et al.: A novel IoT network intrusion detection approach based on adaptive particle swarm optimization convolutional neural network. Inf. Sci. 568 , 147–162 (2021)
Chaganti, R., et al.: A particle swarm optimization and deep learning approach for intrusion detection system in Internet of Medical Things. Sustainability 14 (19), 12828 (2022)
Shi, L., Li, K.: Privacy protection and intrusion detection system of wireless sensor network based on artificial neural network. Comput. Intell. Neurosci. 2022 , 1795454 (2022)
Ethala, S., Kumarappan, A.: A hybrid spider monkey and hierarchical particle swarm optimization approach for intrusion detection on Internet of Things. Sensors 22 (21), 8566 (2022)
Davahli, A., Shamsi, M., Abaei, G.: Hybridizing genetic algorithm and grey wolf optimizer to advance an intelligent and lightweight intrusion detection system for IoT wireless networks. J. Ambient. Intell. Humaniz. Comput. 11 , 5581–5609 (2020)
Keserwani, P.K., et al.: A smart anomaly-based intrusion detection system for the Internet of Things (IoT) network using GWO–PSO–RF model. J. Reliab. Intell. Environ. 7 , 3–21 (2021)
Otair, M., et al.: An enhanced grey wolf optimizer based particle swarm optimizer for intrusion detection system in wireless sensor networks. Wirel. Netw. 28 (2), 721–744 (2022)
Roopa Devi, E., Suganthe, R.: Enhanced transductive support vector machine classification with grey wolf optimizer cuckoo search optimization for intrusion detection system. Concurr. Comput. Pract. Exp. 32 (4), e4999 (2020)
Hajisalem, V., Babaie, S.: A hybrid intrusion detection system based on ABC–AFS algorithm for misuse and anomaly detection. Comput. Netw. 136 , 37–50 (2018)
Jesuretnam, J.B., Rose, J.J.: Performance analysis of optimal cluster selection and intrusion detection by hierarchical K-means clustering with hybrid ABC-DT. Int. J. Pervasive Comput. Commun. 17 (1), 49–63 (2021)
Mazini, M., Shirazi, B., Mahdavi, I.: Anomaly network-based intrusion detection system using a reliable hybrid artificial bee colony and AdaBoost algorithms . J. King Saud Univ. Comput. Inf. Sci. 31 (4): 541–553 (2019)
Ghanem, W.A.H., et al.: An efficient intrusion detection model based on hybridization of artificial bee colony and dragonfly algorithms for training multilayer perceptrons. IEEE Access 8 , 130452–130475 (2020)
Zhang, Y., Li, P., Wang, X.: Intrusion detection for IoT based on improved genetic algorithm and deep belief network. IEEE Access 7 , 31711–31722 (2019)
Mehanović, D., et al.: Feature selection using cloud-based parallel genetic algorithm for intrusion detection data classification. Neural Comput. Appl. 33 , 11861–11873 (2021)
Nguyen, M.T., Kim, K.: Genetic convolutional neural network for intrusion detection systems. Future Gener. Comput. Syst. 113 , 418–427 (2020)
Halim, Z., et al.: An effective genetic algorithm-based feature selection method for intrusion detection systems. Comput. Secur. 110 , 102448 (2021)
Qi, G., et al.: Intrusion detection for network based on elite clone artificial bee colony and back propagation neural network. Wirel. Commun. Mob. Comput. 2021 , 1–11 (2021)
Shen, Y., et al.: An ensemble method based on selection using bat algorithm for intrusion detection. Comput. J. 61 (4), 526–538 (2018)
Ahmed, I., et al.: Feature selection model based on gorilla troops optimizer for intrusion detection systems. J. Sens. 2022 , 1–12 (2022)
Natesan, P., et al.: Hadoop based parallel binary bat algorithm for network intrusion detection. Int. J. Parallel Program. 45 , 1194–1213 (2017)
Shitharth, S.: An enhanced optimization based algorithm for intrusion detection in SCADA network. Comput. Secur. 70 , 16–26 (2017)
Ghanem, W.A., Jantan, A.: A new approach for intrusion detection system based on training multilayer perceptron by using enhanced Bat algorithm. Neural Comput. Appl. 32 , 11665–11698 (2020)
Akinyelu, A.A., Adewumi, A.O.: On the performance of cuckoo search and bat algorithms based instance selection techniques for SVM speed optimization with application to e-fraud detection. KSII Trans. Internet Inf. Syst. 12 (3), 1348–1375 (2018)
Alsharafat, W.: The cuckoo feature filtration method for intrusion detection (cuckoo-ID) . Int. J. Adv. Comput. Sci. Appl. 11 (5) (2020)
Thirumalairaj, A., Jeyakarthic, M.: Hybrid cuckoo search optimization based tuning scheme for deep neural network for intrusion detection systems in cloud environment. J. Res. Lepidoptera 51 (2), 209–224 (2020)
Ghanem, W.A.H., et al.: Cyber intrusion detection system based on a multiobjective binary bat algorithm for feature selection and enhanced bat algorithm for parameter optimization in neural networks. IEEE Access 10 , 76318–76339 (2022)
Alrayes, F.S., et al.: Enhanced gorilla troops optimizer with deep learning enabled cybersecurity threat detection. Comput. Syst. Sci. Eng. 45 (3), 3037–3052 (2023)
Chen, J., et al.: Quantum-inspired ant lion optimized hybrid k-means for cluster analysis and intrusion detection. Knowl. Based Syst. 203 , 106167 (2020)
Samadi Bonab, M., et al.: A wrapper-based feature selection for improving performance of intrusion detection systems. Int. J. Commun. Syst. 33 (12), e4434 (2020)
Abu Khurma, R., Almomani, I., Aljarah, I.: IoT botnet detection using Salp swarm and ant lion hybrid optimization model. Symmetry 13 (8), 1377 (2021)
Bangui, H., Buhnova, B.: Lightweight intrusion detection for edge computing networks using deep forest and bio-inspired algorithms. Comput. Electr. Eng. 100 , 107901 (2022)
Lu, J., Osorio, C.: A probabilistic traffic-theoretic network loading model suitable for large-scale network analysis. Transp. Sci. 52 (6), 1509–1530 (2018)
Creech, G., Hu, J.: A semantic approach to host-based intrusion detection systems using contiguous and discontiguous system call patterns. IEEE Trans. Comput. 63 (4), 807–819 (2013)
Hoque, M.S., et al.: An implementation of intrusion detection system using genetic algorithm (2012). arXiv preprint arXiv:1204.1336
Elrawy, M.F., Awad, A.I., Hamed, H.F.: Intrusion detection systems for IoT-based smart environments: a survey. J. Cloud Comput. 7 (1), 1–20 (2018)
Wu, Z.-Y., Ismail, M., Wang, J.: Efficient exclusion strategy of shadowed RIS in dynamic indoor programmable wireless environments. IEEE Trans. Wirel. Commun. 23 (2), 994–1007 (2023)
Guo, R., Liu, H., Liu, D.: When deep learning-based soft sensors encounter reliability challenges: a practical knowledge-guided adversarial attack and its defense. IEEE Trans. Ind. Inform. 20 (2), 2702–2714 (2023)
Gao, J., et al.: MetaLoc: learning to learn wireless localization. IEEE J. Sel. Areas Commun. (2023). https://doi.org/10.48550/arXiv.2211.04258
Xu, H., et al.: Anomaly traffic detection based on communication-efficient federated learning in space–air–ground integration network. IEEE Trans. Wirel. Commun. 22 (12), 9346–9360 (2023)
Bratton, D., Kennedy, J.: Defining a standard for particle swarm optimization. In: 2007 IEEE Swarm Intelligence Symposium, 2007. IEEE (2007)
Krishna, E., Arunkumar, T.: Hybrid particle swarm and gray wolf optimization algorithm for IoT intrusion detection system. Int. J. Intell. Eng. Syst. 14 (4), 66–76 (2021)
Jung, S.-M.: Hyers-Ulam stability of a system of first order linear differential equations with constant coefficients. J. Math. Anal. Appl. 320 (2), 549–561 (2006)
Abbasi, M., Mohammadi Pasand, E., Khosravi, M.R.: Workload allocation in IoT-fog-cloud architecture using a multi-objective genetic algorithm. J. Grid Comput. 18 (1), 43–56 (2020)
Sun, X., et al.: State feedback control for a PM hub motor based on gray wolf optimization algorithm. IEEE Trans. Power Electron. 35 (1), 1136–1146 (2019)
Yang, H., Zhou, Z.: A novel intrusion detection scheme using cloud grey wolf optimizer. In: 2018 37th Chinese Control Conference (CCC), 2018. IEEE (2018)
Liu, D., et al.: Concurrent low-power listening: a new design paradigm for duty-cycling communication. ACM Trans. Sens. Netw. 19 (1), 1–24 (2022)
Zheng, W., et al.: An Abstract Syntax Tree based static fuzzing mutation for vulnerability evolution analysis. Inf. Softw. Technol. 158 , 107194 (2023)
Fan, X., et al.: Review and classification of bio-inspired algorithms and their applications. J. Bionic Eng. 17 , 611–631 (2020)
Nicklow, J., et al.: State of the art for genetic algorithms and beyond in water resources planning and management. J. Water Resour. Plan. Manag. 136 (4), 412–432 (2010)
Karaboga, D.: An Idea Based on Honey Bee Swarm for Numerical Optimization. Technical Report-tr06. Erciyes University, Engineering Faculty (2005)
Deng, X., et al.: An intelligent outlier detection method with one class support tucker machine and genetic algorithm toward big sensor data in Internet of Things. IEEE Trans. Ind. Electron. 66 (6), 4672–4683 (2018)
Liang, J., Yu, X., Li, H.: Collaborative energy-efficient moving in Internet of Things: genetic fuzzy tree versus neural networks. IEEE Internet Things J. 6 (4), 6070–6078 (2018)
Lin, N., et al.: An effective order-aware hybrid genetic algorithm for capacitated vehicle routing problems in Internet of Things. IEEE Access 7 , 86102–86114 (2019)
Jain, V., Agrawal, M.: Applying genetic algorithm in intrusion detection system of IoT applications. In: 2020 4th International Conference on Trends in Electronics and Informatics (ICOEI) (48184), 2020. IEEE (2020)
Yang, X.S., Hossein Gandomi, A.: Bat algorithm: a novel approach for global engineering optimization. Eng. Comput. 29 (5), 464–483 (2012)
Lu, S., Wang, S.-H., Zhang, Y.-D.: Detection of abnormal brain in MRI via improved AlexNet and ELM optimized by chaotic bat algorithm. Neural Comput. Appl. 33 , 10799–10811 (2021)
Yuvaraj, T., et al.: Comparative analysis of various compensating devices in energy trading radial distribution system for voltage regulation and loss mitigation using blockchain technology and bat algorithm. Energy Rep. 7 , 8312–8321 (2021)
Li, K., et al.: Couple-group consensus of cooperative–competitive heterogeneous multiagent systems: a fully distributed event-triggered and pinning control method. IEEE Trans. Cybern. 52 (6), 4907–4915 (2020)
Mostafa, R.R., et al.: An improved gorilla troops optimizer for global optimization problems and feature selection. Knowl. Based Syst. 269 , 110462 (2023)
Natarajan, E., et al.: Gorilla troops optimizer combined with ANFIS for wire cut EDM of aluminum alloy. Adv. Mater. Sci. Eng. 2022 , 3072663 (2022)
Mou, J., et al.: A machine learning approach for energy-efficient intelligent transportation scheduling problem in a real-world dynamic circumstances. IEEE Trans. Intell. Transp. Syst. 24 (12), 15527–15539 (2022)
Stallings, W., et al.: Computer Security: Principles and Practice, vol. 3. Pearson, Upper Saddle River (2012)
Zhang, H., et al.: Security defense decision method based on potential differential game for complex networks. Comput. Secur. 129 , 103187 (2023)
Yu, J., et al.: An indirect eavesdropping attack of keystrokes on touch screen through acoustic sensing. IEEE Trans. Mob. Comput. 20 (2), 337–351 (2019)
Fatani, A., et al.: IoT intrusion detection system using deep learning and enhanced transient search optimization. IEEE Access 9 , 123448–123464 (2021)
Chen, B., et al.: Finite-time velocity-free rendezvous control of multiple AUV systems with intermittent communication. IEEE Trans. Syst. Man Cybern. Syst. 52 (10), 6618–6629 (2022)
Sokolova, M., Japkowicz, N., Szpakowicz, S.: Beyond accuracy, F-score and ROC: a family of discriminant measures for performance evaluation. In: AI 2006: Advances in Artificial Intelligence: 19th Australian Joint Conference on Artificial Intelligence, Proceedings 19, Hobart, Australia, 4–8 December 2006. Springer (2006)
Congalton, R.G.: A review of assessing the accuracy of classifications of remotely sensed data. Remote Sens. Environ. 37 (1), 35–46 (1991)
Manel, S., Williams, H.C., Ormerod, S.J.: Evaluating presence–absence models in ecology: the need to account for prevalence. J. Appl. Ecol. 38 (5), 921–931 (2001)
Lipton, Z.C., Elkan, C., Naryanaswamy, B.: Optimal thresholding of classifiers to maximize F1 measure. In: Machine Learning and Knowledge Discovery in Databases: European Conference, ECML PKDD 2014, Proceedings, Part II 14, Nancy, France, 15–19 September 2014. Springer (2014)
Ahuja, N., et al.: Automated DDOS attack detection in software defined networking. J. Netw. Comput. Appl. 187 , 103108 (2021)
Liao, Q., et al.: An integrated multi-task model for fake news detection. IEEE Trans. Knowl. Data Eng. 34 (11), 5154–5165 (2021)
Jiang, H., et al.: A utility-aware general framework with quantifiable privacy preservation for destination prediction in LBSs. IEEE/ACM Trans. Netw. 29 (5), 2228–2241 (2021)
Li, L., Yao, L.: Fault tolerant control of fuzzy stochastic distribution systems with packet dropout and time delay. IEEE Trans. Autom. Sci. Eng. (2023). https://doi.org/10.1109/TASE.2023.3266065
Tan, J., et al.: WF-MTD: Evolutionary decision method for moving target defense based on Wright-Fisher process. IEEE Trans. Dependable Secure Comput. 20 (6), 4719–4732 (2022)
Hu, J., et al.: Consensus control of general linear multiagent systems with antagonistic interactions and communication noises. IEEE Trans. Autom. Control 64 (5), 2122–2127 (2018)
Li, S., et al.: A vehicle detection method based on disparity segmentation. Multimed. Tools Appl. 82 , 19643–19655 (2023)
Ding, Y., et al.: FraudTrip: taxi fraudulent trip detection from corresponding trajectories. IEEE Internet Things J. 8 (16), 12505–12517 (2020)
Hu, J., et al.: Sliding mode control for Markovian jump repeated scalar nonlinear systems with packet dropouts: the uncertain occurrence probabilities case. Appl. Math. Comput. 362 , 124574 (2019)
MathSciNet Google Scholar
Chen, Y., et al.: Risk propagation in multilayer heterogeneous network of coupled system of large engineering project. J. Manag. Eng. 38 (3), 04022003 (2022)
Dai, M., et al.: Maximize the Long-Term Average Revenue of Network Slice Provider via Admission Control Among Heterogeneous Slices (2023) . arXiv preprint arXiv:2304.10057
Dai, M., et al.: PSACCF: Prioritized online slice admission control considering fairness in 5G/B5G networks. IEEE Trans. Netw. Sci. Eng. 9 (6), 4101–4114 (2022)
Download references
This work was supported by 2022 Jiangxi Provincial Department of Education Science and Technology Research Project: Research on Robust Control Strategy of Intelligent Connected Vehicles for Information Security, GJJ2202610, The "Fourteenth Five-Year Plan" of Jiangxi Province's education discipline in 2022: Research on the construction of professional curriculum system integrating ideological and political elements from the perspective of "three complete education"- taking software engineering as an example, 22YB259.
Author information
Authors and affiliations.
School of Information Engineering, Jiangxi University of Technology, Nanchang, 330098, Jiangxi, China
School of Intelligent Engineering, Jiangxi University of Technology, Nanchang, 330098, Jiangxi, China
Qingsong Cao
Pôle Universitaire Léonard de Vinci, Paris, France
Mehdi Darbandi
Department of Computer Engineering, Kadir Has Universitesi, 34085, Istanbul, Turkey
Nima Jafari Navimipour
Future Technology Research Center, National Yunlin University of Science and Technology, Douliou, 64002, Yunlin, Taiwan
You can also search for this author in PubMed Google Scholar
Contributions
All authors reviewed the manuscript.
Corresponding authors
Correspondence to Wengui Hu or Nima Jafari Navimipour .
Ethics declarations
Conflict of interest.
The authors declare no competing interests.
Additional information
Publisher's note.
Springer Nature remains neutral with regard to jurisdictional claims in published maps and institutional affiliations.
Rights and permissions
Springer Nature or its licensor (e.g. a society or other partner) holds exclusive rights to this article under a publishing agreement with the author(s) or other rightsholder(s); author self-archiving of the accepted manuscript version of this article is solely governed by the terms of such publishing agreement and applicable law.
Reprints and permissions
About this article
Hu, W., Cao, Q., Darbandi, M. et al. A deep analysis of nature-inspired and meta-heuristic algorithms for designing intrusion detection systems in cloud/edge and IoT: state-of-the-art techniques, challenges, and future directions. Cluster Comput (2024). https://doi.org/10.1007/s10586-024-04385-8
Download citation
Received : 04 September 2023
Revised : 24 February 2024
Accepted : 26 February 2024
Published : 26 April 2024
DOI : https://doi.org/10.1007/s10586-024-04385-8
Share this article
Anyone you share the following link with will be able to read this content:
Sorry, a shareable link is not currently available for this article.
Provided by the Springer Nature SharedIt content-sharing initiative
- Nature-inspired algorithms
- Cloud computing
- Intrusion detection systems
- Intrusion detection
- Internet of Things
- Find a journal
- Publish with us
- Track your research
- All Research Labs
- 3D Deep Learning
- Applied Research
- Autonomous Vehicles
- Deep Imagination
- New and Featured
- AI Art Gallery
- AI & Machine Learning
- Computer Vision
- Academic Collaborations
- Government Collaborations
- Graduate Fellowship
- Internships
- Research Openings
- Research Scientists
- Meet the Team
- Publications
Stateful Conformer with Cache-based Inference for Streaming Automatic Speech Recognition
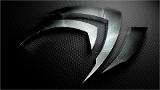
In this paper, we propose an efficient and accurate streaming speech recognition model based on the FastConformer architecture. We adapted the FastConformer architecture for streaming applications through: (1) constraining both the look-ahead and past contexts in the encoder, and (2) introducing an activation caching mechanism to enable the non-autoregressive encoder to operate autoregressively during inference. The proposed model is thoughtfully designed in a way to eliminate the accuracy disparity between the train and inference time which is common for many streaming models. Furthermore, our proposed encoder works with various decoder configurations including Connectionist Temporal Classification (CTC) and RNN-Transducer (RNNT) decoders. Additionally, we introduced a hybrid CTC/RNNT architecture which utilizes a shared encoder with both a CTC and RNNT decoder to boost the accuracy and save computation. We evaluate the proposed model on LibriSpeech dataset and a multi-domain large scale dataset and demonstrate that it can achieve better accuracy with lower latency and inference time compared to a conventional buffered streaming model baseline. We also showed that training a model with multiple latencies can achieve better accuracy than single latency models while it enables us to support multiple latencies with a single model. Our experiments also showed the hybrid architecture would not only speedup the convergence of the CTC decoder but also improves the accuracy of streaming models compared to single decoder models.
Publication Date
Research area, external links.
Advances, Systems and Applications
- Open access
- Published: 02 November 2023
Review on the application of cloud computing in the sports industry
- Lei Xiao 1 ,
- Yang Cao 2 ,
- Yihe Gai 1 ,
- Juntong Liu 3 ,
- Ping Zhong 4 &
- Mohammad Mahdi Moghimi 5
Journal of Cloud Computing volume 12 , Article number: 152 ( 2023 ) Cite this article
2088 Accesses
Metrics details
The transformative impact of cloud computing has permeated various industries, reshaping traditional business models and accelerating digital transformations. In the sports industry, the adoption of cloud computing is burgeoning, significantly enhancing efficiency and unlocking new potentials. This paper provides a comprehensive review of the applications of cloud computing in the sports industry, focusing on areas such as athlete performance tracking, fan engagement, operations management, sports marketing, and event hosting. Moreover, the challenges and potential future developments of cloud computing applications in this industry are also discussed. The purpose of this review is to provide a thorough understanding of the state-of-the-art applications of cloud computing in the sports industry and to inspire further research and development in this field.
Introduction
Background and importance of cloud computing.
Cloud computing has risen to prominence in the last two decades as a result of significant advances in digital technology. It is a computing paradigm that allows on-demand access to shared pools of configurable computing resources, such as servers, storage, applications, and services, that can be rapidly provisioned with minimal management effort [ 1 ]. This flexibility, scalability, and cost-effectiveness have made cloud computing an integral part of businesses across various sectors. Today, more and more business domains have adopted cloud computing paradigm to provide more economic, convenient and lightweight service provisions.
In the context of sports, cloud computing has the potential to transform many aspects of the industry. The advent of cloud technology has ushered in new opportunities, allowing sports organizations to improve their operations, optimize performance, enhance fan engagement, and open up new revenue streams. For instance, cloud-based solutions can efficiently handle large volumes of data generated during games, providing insightful analysis for strategy formulation and performance enhancement [ 2 ]. Moreover, cloud computing is at the core of many innovative technologies that are changing the face of the sports industry. From wearable technology that tracks athlete’s performance to virtual reality experiences that engage fans like never before, cloud computing is playing a pivotal role in driving these innovations [ 3 ]. It is also enabling sports organizations to transition from traditional operational methods to more efficient, scalable, and sustainable models, which are essential in today’s rapidly evolving digital landscape [ 4 ].
To further clarify the importance and significance of introducing cloud computing technology into sports industry, we present a typical application framework of cloud computing in Fig. 1 where a three-layer architecture is provided. In concrete, in the 3rd layer, various sensors or embedded devices are used to monitor and collect the real-time health conditions or signals of players involved in different sport items; in the 2nd layer, the collected sensor data of players are transmitted to nearby edge servers for initial processing and computation; in the 1st layer, the core data processed by edge servers are integrated together by a central cloud platform for uniform data analysis, mining and decision-makings.
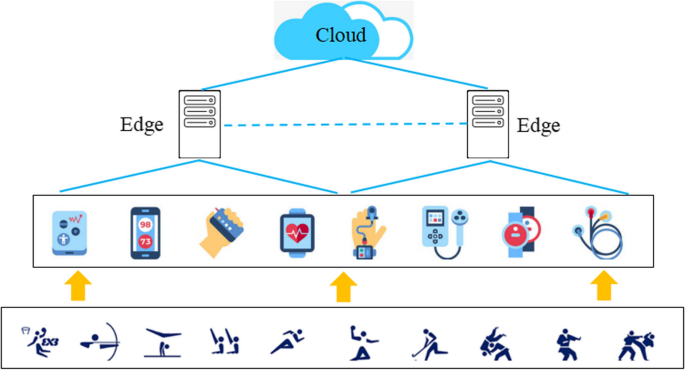
Three-layer data processing structure in cloud-aided sport industry
Overview of cloud computing in the sports industry
In recent years, the sports industry has increasingly adopted cloud computing, transforming multiple facets of the industry from athlete performance tracking to fan engagement, and operations management. Cloud computing technologies offer an effective solution for data storage and analytics in the sports industry. Large volumes of data can be generated from various sources, such as player tracking systems, ticket sales, and social media interactions. The cloud provides a platform where these vast amounts of data can be securely stored and effectively processed to derive actionable insights [ 5 ].
In the realm of athlete performance and health monitoring, the integration of cloud computing with wearable technology has been revolutionary. Wearable devices collect and transfer data to the cloud where sophisticated algorithms analyze the data and provide detailed performance reports and health assessments [ 6 ]. This empowers athletes and coaches to make data-driven decisions and develop personalized training regimens. Fan engagement has also been elevated by cloud computing, with platforms harnessing the cloud to deliver customized experiences. These range from interactive mobile applications providing real-time updates to virtual reality experiences immersing fans into the heart of the action [ 7 ]. Furthermore, cloud technology supports operational efficiency in sports organizations. It enables streamlined ticketing systems, better inventory management, and effective coordination of multi-faceted sporting events [ 8 ].
Despite these benefits, the adoption of cloud computing in sports is not without challenges. These range from data security concerns to the cost of technology implementation. This paper aims to offer a comprehensive review of these applications, highlighting both the opportunities and challenges that cloud computing presents to the sports industry.
Paper organization structure
This paper is organized as follows: “ Applications of cloud computing in the sports industry ” section provides a comprehensive examination of the applications of cloud computing in the sports industry, including athlete performance tracking, fan engagement, operations management, sports marketing, and event hosting. In “ Challenges of cloud computing in the sports industry ” section, we address the challenges associated with integrating cloud computing into the sports industry, such as data privacy, costs, and internet dependency. Following this, potential future developments and trends in the intersection of cloud computing and sports are discussed in “ Future trends and potential developments ” section, touching upon the integration with other technologies, customization, and sustainability. Finally, in “ Conclusion ” section, the paper concludes with a summary of key findings and suggestions for future research in this area.
Applications of cloud computing in the sports industry
Athlete performance tracking.
One of the key applications of cloud computing in the sports industry is the tracking of athlete performance. This involves capturing, storing, and analyzing vast amounts of data related to an athlete’s physical and physiological performance. With the integration of cloud technology, this process has become significantly more streamlined, enabling detailed, real-time performance monitoring and creating a data-driven approach to performance enhancement. Cloud-based performance tracking often employs wearable technology to gather real-time data from athletes during training and matches. For example, wearable devices can track parameters such as heart rate, acceleration, and GPS location, among others [ 9 ]. This data, often substantial in volume, is then transferred to the cloud where it is securely stored and processed.
Through the application of machine learning and artificial intelligence techniques on the cloud, the raw data can be transformed into meaningful insights. These insights include patterns and trends in an athlete’s performance, which can help to tailor training programs, identify areas for improvement, and anticipate potential injury risks [ 10 ]. Athletes wear devices (like smartwatches, fitness bands, or even smart clothing) can collect data on their heart rate, speed, acceleration, and more. This data is then uploaded to the cloud in real-time. Coaches and trainers can access this data from anywhere, analyze it using cloud-based software, and provide immediate feedback to the athlete. Over time, this data can be used to track performance trends, identify areas of improvement, and customize training regimens [ 11 ]. Furthermore, the remote and on-demand access to data and insights provided by the cloud allows coaches and sports scientists to monitor athlete performance and make timely interventions, irrespective of their physical location. This is particularly useful in the current era of global sports, where athletes and teams travel extensively [ 12 ].
Fan engagement
Cloud computing has a significant role in enhancing fan engagement in the sports industry, allowing fans to interact with their favorite sports and athletes in previously unimaginable ways. By providing fans with personalized and immersive experiences, cloud computing is transforming how fans consume sports. One of the primary areas where cloud computing impacts fan engagement is through the use of social media. Social media platforms provide a space where fans can engage with their favorite teams and athletes and share their experiences with others. Cloud computing enables these platforms to handle massive amounts of data and deliver personalized content to individual users. For example, the use of data analytics can help sports organizations understand fans’ behavior, allowing them to provide fans with tailored content that meets their preferences and enhances their engagement [ 13 ].
In addition, cloud computing is enabling the creation of advanced mobile applications that provide fans with real-time updates, video content, and opportunities for interaction. Sports organizations can use these applications to engage fans during live events, providing them with real-time statistics, instant replays, and interactive features such as voting systems or quizzes [ 14 ]. Beyond mobile applications, the intersection of cloud computing and virtual reality (VR) technology is providing fans with immersive experiences. For example, fans can use VR headsets to experience a live game from the best seats in the stadium, or even from the perspective of a player on the field, all from the comfort of their own homes [ 15 ]. Cloud computing underpins these experiences by providing the necessary processing power and data storage capacity. Furthermore, the ability of cloud platforms to integrate various types of data has opened up new possibilities for fan engagement. For example, by combining data from different sources, such as ticket sales, social media interactions, and online merchandise purchases, sports organizations can gain a more comprehensive understanding of their fans’ behavior. This can inform marketing strategies and create personalized fan experiences [ 16 ].
Fans can use VR headsets or AR-enabled smartphones to access cloud-hosted virtual stadiums, watch games from unique angles, or even walk on the field with their favorite players. The cloud ensures that these experiences are smooth and high-quality by handling the heavy computational load and delivering content seamlessly to users worldwide.However, while cloud computing offers tremendous opportunities to enhance fan engagement, it also raises concerns related to data privacy and security. Sports organizations must ensure they adhere to data protection regulations and take appropriate steps to protect their fans’ data. In summary, cloud computing is significantly enhancing fan engagement in the sports industry by enabling personalized, interactive, and immersive fan experiences. As technologies continue to evolve, it is anticipated that the role of cloud computing in fan engagement will become increasingly integral.
- Operations management
The application of cloud computing in operations management is shaping the sports industry by introducing efficient, scalable, and flexible solutions that transform traditional operational processes. From inventory management to ticketing systems, cloud computing’s robust capabilities are driving operational efficiency and improving overall performance. In terms of inventory and facility management, sports organizations often grapple with managing vast inventories of equipment, merchandise, and food and beverage supplies. The adoption of cloud-based inventory management systems allows these organizations to accurately track inventory in real-time, streamline procurement processes, and minimize waste, resulting in significant cost savings [ 17 ]. Similarly, for facility management, cloud-based solutions can assist in scheduling, maintaining, and managing sports facilities more efficiently, resulting in improved utilization and cost effectiveness [ 18 ].
Ticketing and registration systems have also benefited from cloud technology. Traditional ticketing systems often involve labor-intensive processes and are prone to inefficiencies and inaccuracies. However, cloud-based ticketing solutions offer a more efficient approach, allowing fans to purchase and validate tickets digitally, reducing the likelihood of counterfeit tickets and enhancing the fan experience [ 19 ]. Moreover, these systems can handle large volumes of transactions simultaneously, a critical feature during high-demand periods. The coordination and management of sports events, particularly large-scale events like the Olympics or the FIFA World Cup, can be exceedingly complex. Cloud computing provides a platform for effective coordination of all aspects of these events, from logistics and security to volunteer management and media coverage. For example, during the 2020 Tokyo Olympics, cloud technology was used to integrate and manage data from various sources, enabling efficient operations and real-time decision-making [ 20 ]. Cloud-based systems can handle ticket sales, reservations, and access control for large-scale sports events. Fans can purchase tickets online, receive digital tickets, and use their smartphones or QR codes for entry. The cloud system can handle peak loads (like during a major game’s ticket release) and ensure that operations run smoothly.
Cloud computing is also facilitating collaborative work environments in the sports industry. Cloud-based platforms allow staff to access necessary documents and applications from any device, promoting productivity and flexibility in workflows [ 21 ]. Despite these advantages, transitioning to cloud-based operations management can be challenging, involving significant costs and requiring a change in organizational culture and workflows. Also, the reliance on internet connectivity and concerns about data security need to be addressed. Cloud computing is transforming operations management in the sports industry, providing efficient, scalable, and flexible solutions that enhance organizational performance. As the industry continues to adapt to digital transformation, the use of cloud technology in operations management is expected to become more prevalent.
Sports marketing
Cloud computing has introduced a transformative shift in sports marketing, enabling strategies to become more targeted, personalized, and data-driven [ 22 ]. The unprecedented levels of connectivity and data accessibility provided by cloud computing are paving the way for more effective marketing strategies and more lucrative sponsorship opportunities.
Targeted marketing strategies
In the realm of sports marketing, cloud computing’s capacity to handle large volumes of data and conduct sophisticated data analytics has enabled highly targeted marketing strategies. These strategies are underpinned by the analysis of a myriad of data sources, from social media interactions and online merchandise purchases to ticketing information and fan app usage [ 23 ]. By analyzing this data, sports organizations can gain valuable insights into fans’ behavior and preferences, enabling them to create highly targeted and personalized marketing campaigns. For instance, clubs can use these insights to tailor email marketing content to individual fans, promoting merchandise or tickets based on their past behavior and preferences [ 24 ]. Moreover, cloud-based platforms can enable real-time marketing, allowing organizations to react instantly to events on and off the field. For example, a memorable moment in a game can be instantly converted into a marketing opportunity, with relevant content quickly created and distributed across digital platforms [ 25 ].
Data-driven sponsorship opportunities
In terms of sponsorship opportunities, the adoption of cloud computing allows sports organizations to provide potential sponsors with detailed, data-driven insights into their fan base. This ability to quantitatively demonstrate fan engagement levels and demographic breakdowns makes sports organizations more appealing to sponsors, as they can better assess the potential return on investment and align their marketing efforts with the right audience [ 26 ]. Furthermore, during live events, cloud technology can facilitate dynamic sponsorship opportunities. By analyzing real-time data, digital advertising hoardings can display personalized advertisements tailored to the audience watching at home, opening up a new dimension to sports sponsorships [ 26 ].
Sports teams and organizations can collect data on fan preferences, online interactions, merchandise purchases, and more. This data, stored and analyzed in the cloud, can be used to create personalized marketing campaigns. For example, a fan who frequently watches a particular player’s highlights might receive special merchandise offers related to that player. Nevertheless, with these new opportunities come challenges related to data privacy and security [ 27 , 28 , 29 ]. Organizations must balance their marketing strategies and sponsorship opportunities with the ethical and legal requirements of data protection, underlining the importance of robust data governance policies. Cloud computing is driving a transformative shift in sports marketing, enabling more targeted, personalized, and data-driven strategies. As cloud technology continues to evolve, it is expected to play an increasingly integral role in sports marketing and sponsorship.
Event hosting
Cloud computing has significantly revolutionized the process of hosting sports events. From the planning phase through execution to the post-event analysis, cloud computing provides robust, scalable, and cost-effective solutions that enhance efficiency, engagement, and experience [ 30 , 31 ]. Cloud-based event management tools simplify the planning process by providing a central platform where all aspects of event planning can be coordinated. Tasks such as scheduling, resource allocation, volunteer management, and participant registration can all be managed effectively on these platforms. By using the cloud, these processes can be automated, tracked, and updated in real-time, enhancing efficiency and communication among the event management team [ 32 ].
The actual execution of the event can also benefit from cloud technology. The integration of ticketing systems, access control, real-time information updates, and security management into a unified cloud platform can improve event operations, resulting in a smoother and more enjoyable experience for attendees [ 33 ]. Moreover, cloud-based systems can handle the surge in internet traffic during the event, ensuring seamless access to information for attendees, staff, and online viewers. One of the most transformative applications of cloud computing in event hosting is the provision of live streaming services. Through cloud platforms, sports events can be broadcast live to viewers worldwide, dramatically expanding the reach of the event. The scalability of the cloud ensures that the streaming service can handle large viewer numbers, providing a smooth viewing experience [ 34 ].
Furthermore, cloud computing enables the real-time analysis of event data. Data gathered from ticket sales, social media interactions, and audience engagement can be analyzed to provide valuable insights during the event. This allows event organizers to make data-driven decisions to enhance the ongoing event experience [ 35 ]. Post-event, the cloud facilitates efficient wrap-up procedures, including financial reconciliation, feedback collection, and performance analysis. The data gathered before and during the event can be further analyzed to evaluate the event’s success and inform the planning of future events [ 36 ].
During large sports events, organizers can use cloud-connected cameras and sensors to monitor crowd movements, identify potential congestion points, and ensure safety protocols are followed. The cloud processes this data in real-time, allowing event managers to make quick decisions, like redirecting foot traffic or dispatching security to specific locations. However, the integration of cloud computing into event hosting presents challenges. These include concerns over data security, reliability of internet connectivity, and the requirement for significant upfront investment in cloud technology.
Challenges of cloud computing in the sports industry
Data privacy and security.
While cloud computing offers significant benefits to the sports industry, it also presents certain challenges, with data privacy and security being paramount among them [ 37 ]. With the vast amounts of data generated and stored in the cloud, including sensitive personal data, health information, and performance metrics, it is crucial that these are securely managed and protected. Data privacy represents a significant concern, as information stored in the cloud can potentially be accessed from anywhere, by anyone with the correct credentials. This increases the risk of unauthorized access and privacy breaches. The situation is compounded by the fact that the data involved often includes sensitive personal information, such as names, addresses, health data, and credit card information [ 38 ]. Especially in the big data context, the traditional centralized data processing paradigm with cloud is not efficient enough. Therefore, to alleviate the heavy burden of cloud platform, many edge servers are often used to make initial data preprocessing before the massive data are directly sent to the cloud platform. In this situation, private user data are probably disclosed to other parties during the data transmission among cloud, edge and users.
Security is another major challenge in cloud computing. Despite robust security measures, no system is completely immune to security threats. These threats can include hacking attempts, data breaches, and other cyber-attacks. Such incidents not only compromise the privacy of individuals but can also significantly damage the reputation of sports organizations [ 39 ]. The use of third-party cloud service providers further complicates matters. Organizations often have limited control over their data security when using third-party services, which can result in potential vulnerabilities [ 40 ]. Sports organizations are also faced with the complex task of navigating global data protection regulations. Laws such as the General Data Protection Regulation (GDPR) in Europe impose stringent requirements on how personal data is handled, including how it is collected, stored, and shared. Non-compliance can lead to significant penalties [ 41 ].
To address these challenges, sports organizations need to ensure they have robust data governance policies in place and that they are using secure and reliable cloud services. This includes ensuring appropriate encryption, access controls, and intrusion detection systems are in place. They must also ensure they are transparent with their stakeholders about their data handling practices and that they are in compliance with all relevant regulations [ 42 ]. In conclusion, while cloud computing offers vast potential for the sports industry, it is not without its challenges. Data privacy and security issues need to be thoroughly addressed to ensure the benefits of cloud computing can be realized without compromising the privacy and security of stakeholders.
Cost and complexity of implementation
Implementing cloud computing in the sports industry can be both costly and complex. While cloud computing promises cost savings over time due to reduced need for hardware and physical infrastructure, the upfront costs can be significant. These costs can include the implementation of the cloud solution itself, staff training, and ongoing maintenance and support [ 43 ]. The cost can be particularly challenging for smaller sports organizations, which may not have the necessary budget to invest in advanced cloud solutions. Although cloud services are generally billed on a usage basis, which allows for scaling according to need, the initial investment can still be a barrier for these organizations [ 44 ].
Moreover, migrating to a cloud-based system can be a complex process, requiring specialized knowledge and expertise. Depending on the size of the organization and the extent of its data, the migration process can be time-consuming and potentially disruptive to operations. This process often requires the support of external IT specialists, which adds to the overall cost of implementation [ 45 ]. The complexity of implementation also extends to the integration of the cloud solution with existing systems. This can be particularly challenging if the organization’s existing IT infrastructure is outdated or incompatible with the cloud solution. In such cases, a complete overhaul of the IT infrastructure may be required, adding further to the cost and complexity [ 43 ].
There’s also the issue of vendor lock-in. When sports organizations commit to a particular cloud provider’s platform, they may find it difficult to migrate their services to another provider later on. This can limit the organization’s flexibility and could lead to increased costs over time [ 46 ]. While cloud computing offers many potential benefits to the sports industry, the cost and complexity of implementation are significant challenges that need to be carefully managed. To successfully implement cloud solutions, sports organizations need to carefully plan their cloud adoption strategies, taking into account their specific needs, budget constraints, and IT capabilities.
Dependence on internet connectivity
The sports industry’s reliance on cloud computing also means a dependency on consistent and robust internet connectivity. This dependence on internet connectivity is one of the fundamental challenges associated with the adoption of cloud computing. For sports organizations, the reliance on internet connectivity means that any disruption in their internet service could potentially bring their operations to a halt. This is a particular concern for live events, where a disruption in internet service could affect everything from ticketing to live streaming, potentially damaging the reputation of the event and the organization [ 47 ].
Similarly, for athletes and coaches who rely on cloud-based systems for performance tracking and analytics, a lack of internet connectivity could mean lost data or an inability to access crucial information. For example, a coach might be unable to access real-time data about an athlete’s performance during a training session or a game if there is a disruption in internet service [ 48 ]. In areas with weak or inconsistent internet coverage, the adoption of cloud-based solutions can be particularly challenging. This is especially true in developing countries or rural areas where internet infrastructure may not be robust. The digital divide can limit the reach and effectiveness of cloud-based solutions in these areas [ 49 ]. Additionally, the reliance on internet connectivity also has implications for data security. An insecure internet connection can expose data to potential security threats, underlining the importance of secure and reliable internet connectivity [ 50 ]. Mitigating these challenges requires investment in robust, reliable, and secure internet infrastructure. Where possible, organizations might also consider hybrid cloud solutions, which combine private and public cloud services. These solutions can offer offline capabilities, reducing the dependence on constant internet connectivity [ 51 ].
Future trends and potential developments
Integration with other technologies (ai, iot, etc.).
The convergence of cloud computing with other advanced technologies such as Artificial Intelligence (AI), Internet of Things (IoT), and Blockchain presents significant opportunities for the sports industry [ 52 , 53 ]. This integration can amplify the benefits of each technology, driving innovation and creating new ways to enhance athletic performance, fan engagement, and operational efficiency. AI and cloud computing are increasingly becoming interdependent. AI applications, ranging from predictive analytics to automated decision-making systems, rely on the vast computational resources offered by the cloud. On the other hand, the cloud benefits from AI’s ability to process and analyze large datasets, optimize system performance, and improve data security [ 54 ]. In sports, AI can enhance cloud-based athlete performance tracking systems, allowing for real-time analysis and feedback. It can also enhance fan experience by enabling personalized content delivery and predictive services, such as predicting game outcomes or player performance [ 55 ].
IoT, which refers to the network of physical devices connected to the internet, can benefit significantly from integration with cloud computing [ 56 ]. Cloud platforms can store and analyze the large amounts of data generated by IoT devices, allowing for real-time decision-making and enhancing the value of IoT applications in sports [ 57 , 58 ]. IoT devices, such as wearable technology and smart equipment, can provide detailed data about athletes’ performance, health, and safety. When combined with cloud-based analytics, these insights can inform training strategies, injury prevention measures, and even tactics during competitions [ 59 ]. Blockchain technology, known for its security and transparency features, can also complement cloud computing. In sports, blockchain can enhance cloud-based ticketing systems by preventing fraud and ensuring transparency. It can also create new possibilities for fan engagement, such as token-based reward systems or secure voting platforms for fan decisions [ 60 ].
While these integrations offer promising potential, they also introduce additional complexities and challenges, particularly in terms of data privacy, system integration, and technology management [ 61 ]. Future research and innovation should focus on addressing these challenges to fully realize the potential of these integrated technologies in the sports industry.
Customization and personalization
The future of cloud computing in the sports industry points towards a greater focus on customization and personalization [ 62 , 63 , 64 , 65 ]. As the volume of available data continues to increase, there is a growing opportunity for sports organizations to create personalized experiences and offerings for their stakeholders [ 66 ], including athletes, fans, and sponsors. For athletes, cloud-based tools can provide personalized training plans, nutritional advice, and injury prevention strategies. These customized solutions can be developed based on a variety of data, including historical performance data, real-time tracking data, health data, and even genetic information. Such personalization can optimize athlete performance and promote long-term athlete health and wellbeing [ 67 ].
Fans also stand to benefit from more personalized experiences [ 68 , 69 ]. Using data collected from various sources, such as ticketing systems, social media, merchandise sales, and digital platform interactions, sports organizations can create highly targeted content and marketing campaigns. For instance, fans could receive personalized match updates, tailored merchandise recommendations, and bespoke content featuring their favorite players. This level of personalization can improve fan engagement, deepen fan loyalty, and increase revenue from fan-related activities [ 25 ]. For sponsors and partners, personalization can lead to more effective collaboration and improved return on investment. By leveraging the data stored and analyzed in the cloud, sports organizations can provide sponsors with detailed insights into their fan base, enabling the creation of highly targeted marketing strategies. This data-driven approach can enhance the value of sponsorships and partnerships, leading to mutually beneficial relationships [ 14 ].
Looking forward, advancements in AI and machine learning are expected to further enhance the customization and personalization possibilities in sports [ 70 ]. These technologies can help to analyze and interpret the vast amounts of data generated in the sports industry, leading to more accurate insights and more effective personalization strategies [ 55 ]. The future of cloud computing in the sports industry is likely to be characterized by a greater focus on customization and personalization. As technology continues to advance, the possibilities for creating personalized experiences for athletes, fans, and sponsors are expected to grow.
Sustainability and green IT
The role of cloud computing in promoting sustainability and green IT practices within the sports industry is another emerging trend [ 71 , 72 , 73 ]. As the societal focus on environmental sustainability continues to grow, so does the pressure on sports organizations to reduce their environmental impact. Here, cloud computing can be an essential tool. Cloud computing can contribute to sustainability in several ways. Firstly, it reduces the need for physical IT infrastructure, which in turn reduces the energy consumption associated with running and cooling these systems. Cloud data centers benefit from economies of scale and can operate more efficiently than smaller, organization-specific data centers, leading to a smaller carbon footprint [ 74 , 75 , 76 ].
Secondly, the scalability of cloud computing means that resources are only used when needed, preventing the waste associated with underutilized infrastructure. As a result, cloud computing can contribute to more sustainable IT practices within sports organizations [ 77 ]. Moreover, cloud computing can also support sustainability in the sports industry beyond IT practices. The data processing and analysis capabilities of the cloud can support the implementation of other environmentally friendly practices. For example, it can help optimize travel schedules for teams and fans, reducing carbon emissions associated with transportation. It can also enable smarter management of facilities, such as predictive maintenance and energy management, contributing to greener operations [ 78 ]. Looking forward, we can expect further advancements in green cloud computing technologies. For instance, improvements in energy-efficient data center design, renewable energy use, and energy-aware scheduling algorithms will continue to enhance the environmental sustainability of cloud computing [ 75 ].
As environmental sustainability becomes an increasingly important concern for society and for the sports industry, the role of cloud computing in promoting green IT practices and broader sustainability efforts is set to grow. Embracing this trend will not only contribute to environmental protection efforts but also build a positive reputation for sports organizations among increasingly eco-conscious stakeholders.
This study was motivated by the rising prominence of cloud computing and its profound influence on the sports industry. The purpose of this review was to provide a comprehensive overview of the applications of cloud computing in sports, discuss the challenges faced, and identify future trends and potential developments. The research highlights several key applications of cloud computing in sports, including athlete performance tracking, fan engagement, operations management, sports marketing, and event hosting. These applications illustrate how cloud computing has transformed traditional sports practices by facilitating data collection and analysis, enhancing communication, and optimizing operations.
Cloud computing often works well in business systems involving big data processing due to the high computational capability of cloud platforms. Inspired by this observation, cloud computing technology is introduced in sport industries in this paper to deal with big sport data and has achieved good performances in sport industries. However, not all sport items can produce big volume of data that need to be processed by powerful cloud platforms; in such cases, cloud computing platforms are not a necessity since small sport data can be processed by local clients such as computer or laptops.
Alongside the benefits of cloud computing, the research also illuminated several challenges associated with the adoption of cloud computing in the sports industry, including issues of data privacy and security, cost and complexity of implementation, and dependence on internet connectivity. In addition, how to extend the traditional cloud-based sport data processing systems to more flexible and cost-effective edge-based systems to adapt time-efficient and cost-efficient business applications is still a challenging task in future study. These challenges underscore the need for careful planning, robust security measures, and continuous monitoring and adjustment of cloud solutions.
Availability of data and materials
Not applicable.
Wang C, Wang D (2023) Managing the integration of teaching resources for college physical education using intelligent edge-cloud computing. J Cloud Comput 12:82
Article Google Scholar
Cortsen K, Rascher DA (2018) The application of sports technology and sports data for commercial purposes. The use of technology in sport: Emerging challenges, pp 47–84
Camomilla V, Bergamini E, Fantozzi S, Vannozzi G (2018) Trends supporting the in-field use of wearable inertial sensors for sport performance evaluation: A systematic review. Sensors 18(3):873
Svantesson D, Clarke R (2010) Privacy and consumer risks in cloud computing. Comp Law Sec Rev 26(4):391–397. https://doi.org/10.1016/j.clsr.2010.05.005
Webster J, Watson RT (2002) Analyzing the Past to Prepare for the Future: writing a literature review. MIS Quarterly 26(2):xiii–xxiii. http://www.jstor.org/stable/4132319
of Sports Medicine AC, et al (2013) ACSM’s guidelines for exercise testing and prescription. Lippincott Williams & Wilkins
Garatea J, Muñoz I, Ala S, Cardoso F, Gelautz M, Seitner F, Kapeller C, Brosch N, Buresova I, Huteckova S, et al (2017) International conference on Technology and Innovation in Sports, Health and Wellbeing (TISHW) Vila Real, Portugal. 01‐03 December 2016 Abstracts", Bmc Sports Science Medicine And Rehabilitation, vol. 9. p. 2017
Varmus M, Kubina M, Miciak M, Sarlak M, Klampar P, Strba P (2023) Education and knowledge in the field of sponsorship and general funding of sports infrastructure. Economic and Social Development: Book of Proceedings, pp 128–136
Seshadri DR, Li RT, Voos JE, Rowbottom JR, Alfes CM, Zorman CA, Drummond CK (2017) Wearable sensors for monitoring the internal and external workload of the athlete. NPJ Digit Med 2(1):1–12
Google Scholar
Karakaya A, Akleylek S (2021) A novel IoT-based health and tactical analysis model with fog computing. PeerJ Comput Sci 7:342
Kos A, Umek A (2018) Wearable sensor devices for prevention and rehabilitation in healthcare: Swimming exercise with real-time therapist feedback. IEEE Internet Things J 6(2):1331–1341
Van Eetvelde H, Mendonça LD, Ley C, Seil R, Tischer T (2021) Machine learning methods in sport injury prediction and prevention: a systematic review. J Exp Orthop 8:1–15
Hambrick ME, Simmons JM, Greenhalgh GP, Greenwell TC (2019) Understanding professional athletes’ use of twitter: A content analysis of athlete tweets. Int J Sport Commun 2(4):454–471
Chase C (2020) The data revolution: Cloud computing, artificial intelligence, and machine learning in the future of sports. 21st Century Sports: How Technologies Will Change Sports in the Digital Age, pp 175–189
Pantano E, Pizzi G, Scarpi D, Dennis C (2020) Competing during a pandemic? retailers’ ups and downs during the covid-19 outbreak. J Bus Res 116:209–213
Skinner J, Smith AC (2021) Introduction: sport and covid-19: impacts and challenges for the future (volume 1). Eur Sport Manag Q 21(3):323–332
Mauricette J, Wells P, Haar J (2022) User perceptions of cloud-based small business accounting software. Pac Account Rev 34(4):595–613
Wu JC, Lee SM, Chen CJ (2023) Exploring the context with factors of cloud computing to digital transformation and innovation. In: International Conference on Knowledge Management in Organizations, Springer, pp 115–136
Regner F, Urbach N, Schweizer A (2019) NFTs in practice – non-fungible tokens as core component of a blockchain-based event ticketing application. ICIS 2019 Proceedings. 1. https://aisel.aisnet.org/icis2019/blockchain_fintech/blockchain_fintech/1
Du Y, Li Y, Chen J, Hao Y, Liu J (2023) Edge computing-based digital management system of game events in the era of internet of things. J Cloud Comput 12(1):44
Alashhab ZR, Anbar M, Singh MM, Leau YB, Al-Sai ZA, Alhayja’a SA, (2021) Impact of coronavirus pandemic crisis on technologies and cloud computing applications. J Electron Sci Technol 19(1):100059
Han H, Fei S, Yan Z, Zhou X (2022) A survey on blockchain-based integrity auditing for cloud data. Digital Commun Netw 8(5):591–603
Nguyen B, Simkin L, Canhoto AI (2018) The internet of things (IoT) and marketing: the state of play, future trends and the implications for marketing. J Mark Manag 34(1–2):1–6
Thaichon P, Ratten V (2021) Developing digital marketing: Relationship perspectives. Emerald Publishing Limited
Naraine ML, Bakhsh JT, Wanless L (2022) Th e impact of sponsorship on social media engagement: A longitudinal examination of professional sport teams. Sport Mark Q 31(3):239–252
Mandrikov V, Zamyatina N, Zubarev Y, Komleva L, Vakalova L, Vinichenko A et al (2020) Advertising and sponsorship activities in the field of physical education, sports and the Olympic movement. Bioethics 14(2):42–45
Wang F, Wang L, Li G, Wang Y, Lv C, Qi L (2022) Edge-cloud-enabled matrix factorization for diversified apis recommendation in mashup creation. World Wide Web J 25(5):1809–1829
Zhang S, Liu C, Li X, Han Y (2022) Runtime reconfiguration of data services for dealing with out-of-range stream fluctuation in cloud-edge environments. Digit Commun Netw 8(6):1014–1026
Kong L, Li G, Rafique W, Shen S, He Q, Khosravi MR, Wang R, Qi L (2022) Time-aware missing healthcare data prediction based on arima model. IEEE/ACM Trans Comput Biol Bioinforma. https://doi.org/10.1109/TCBB.2022.3205064
Song W, Wu Y, Cui Y, Liu Q, Shen Y, Qiu Z, Yao J, Peng Z (2022) Public integrity verification for data sharing in cloud with asynchronous revocation. Digit Commun Netw 8(1):33–43
Xu Z, Zhu D, Chen J, Yu B (2022) Splitting and placement of data-intensive applications with machine learning for power system in cloud computing. Digit Commun Netw 8(4):476–484
Anshari M, Alas Y, Guan LS (2016) Developing online learning resources: Big data, social networks, and cloud computing to support pervasive knowledge. Educ Inf Technol 21:1663–1677
Al-Dosari K (2020) Analysis of the anticipated and potential economic impacts of mega sporting events on developing countries: a case of FIFA 2020 world cup in Qatar. J Bus Manag (COES &RJ-JBM) 8(3):156–176
Daim TU, Rueda G, Martin H, Gerdsri P (2018) Forecasting emerging technologies: Use of bibliometrics and patent analysis. Technol Forecast Soc Chang 80(8):1482–1492
Santoro G, Vrontis D, Thrassou A, Dezi L (2021) The internet of things: Building a knowledge management system for open innovation and knowledge management capacity. Technol Forecast Soc Chang 153:119254
Vanos JK, Thomas WM, Grundstein AJ, Hosokawa Y, Liu Y, Casa DJ (2020) A multi-scalar climatological analysis in preparation for extreme heat at the Tokyo 2020 Olympic and paralympic games. Temperature 7(2):191–214
Kong L, Wang L, Gong W, Yan C, Duan Y, Qi L (2022) Lsh-aware multitype health data prediction with privacy preservation in edge environment. World Wide Web J 25(5):1793–1808
Chen J, Li K, Zhang Z, Li K, Yu PS (2021) A survey on applications of artificial intelligence in fighting against covid-19. ACM Comput Surv (CSUR) 54(8):1–32
Zhang X, Cui L, Shen W, Zeng J, Du L, He H, Cheng L (2023) File processing security detection in multi-cloud environments: a process mining approach. J Cloud Comput 12:100
Alotaibi AF et al (2021) A comprehensive survey on security threats and countermeasures of cloud computing environment. Turk J Comput Math Educ (TURCOMAT) 12(9):1978–1990
Russo B, Valle L, Bonzagni G, Locatello D, Pancaldi M, Tosi D (2018) Cloud computing and the new EU general data protection regulation. IEEE Cloud Comput 5(6):58–68
Ahmed M, Hossain MA (2014) Cloud computing and security issues in the cloud. Int J Netw Secur Appl 6(1):25
Emeakaroha VC, Bullman M, Morrison JP (2016) Towards automated cost-efficient data management for federated cloud services. In: 2016 5th IEEE International Conference on Cloud Networking (Cloudnet), IEEE, pp 158–163
Sivarajah U, Kamal MM, Irani Z, Weerakkody V (2017) Critical analysis of big data challenges and analytical methods. J Bus Res 70:263–286
Moghavvemi S, Paramanathan T, Rahin NM, Sharabati M (2018) The impact of perceived enjoyment, perceived reciprocal benefits and knowledge power on students’ knowledge sharing through facebook. Int J Manag Educ 16(1):1–12
Serrador P, Pinto JK (2015) Does agile work?—a quantitative analysis of agile project success. Int J Proj Manag 33(5):1040–1051
Botta A, De Donato W, Persico V, Pescapé A (2016) Integration of cloud computing and internet of things: A survey. Futur Gener Comput Syst 56:684–700
Chellaswamy C, Saravanan M, Abirami M, Boosuphasri R, Balaji M, et al (2020) Machine learning based condition recognition system for bikers. In: 2020 7th International Conference on Smart Structures and Systems (ICSSS), IEEE, pp 1–6
Dwivedi YK, Hughes DL, Coombs C, Constantiou I, Duan Y, Edwards JS, Kar AK (2019) Impact of covid-19 pandemic on information management research and practice: Transforming education, work and life. Int J Inf Manag 55(102):211
Kim HW, Jeong YS (2018) Secure authentication-management human-centric scheme for trusting personal resource information on mobile cloud computing with blockchain. Hum-Centric Comput Inf Sci 8(1):1–13
Dillon T, Wu C, Chang E (2016) Cloud computing: Issues and challenges. In: 2010 24th IEEE International Conference on Advanced Information Networking and Applications, IEEE, pp 27–33
Dai H, Xu Y, Chen G, Dou W, Tian C, Wu X, He T (2022) Rose: Robustly safe charging for wireless power transfer. IEEE Trans Mob Comput 21(6):2180–2197
Gu R, Chen Y, Liu S, Dai H, Chen G, Zhang K, Che Y, Huang Y (2021) Liquid: Intelligent resource estimation and network-efficient scheduling for deep learning jobs on distributed GPU clusters. IEEE Trans Parallel Distrib Syst. https://doi.org/10.1109/TPDS.2021.3138825
Bughin J, Hazan E, Ramaswamy S, Chui M, Allas T, Dahlström P, Trench M (2017) Artificial intelligence: the next digital frontier? McKinsey Global Institute
Novatchkov H, Baca A (2013) Artificial intelligence in sports on the example of weight training. J Sports Sci Med 12(1):27
Dai H, Wang X, Lin X, Gu R, Liu Y, Dou W, Chen G (2021) Placing wireless chargers with limited mobility. IEEE Trans Mob Comput. https://doi.org/10.1109/TMC.2021.3136967
Al-Fuqaha A, Guizani M, Mohammadi M, Aledhari M, Ayyash M (2015) Internet of things: A survey on enabling technologies, protocols, and applications. IEEE Commun Surv Tutor 17(4):2347–2376
Gu R, Zhang K, Xu Z, Che Y, Fan B, Hou H, Dai H, Yi L, Ding Y, Chen G, Huang Y (2022) Fluid: Dataset abstraction and elastic acceleration for cloud-native deep learning training jobs. In: 38th IEEE International Conference on Data Engineering, pp 2183–2196
Islam SMR, Kwak D, Kabir MH, Hossain M, Kwak KS (2015) The internet of things for health care: a comprehensive survey. IEEE Access 3:678–708
Casino F, Dasaklis TK, Patsakis C (2019) A systematic literature review of blockchain-based applications: current status, classification and open issues. Telematics Inform 36:55–81
Dai H, Yu J, Li M, Wang W, Liu A, Ma J, Qi L, Chen G (2022) Bloom filter with noisy coding framework for multi-set membership testing. IEEE Trans Knowl Data Eng. https://doi.org/10.1109/TKDE.2022.3199646
Wang S, Chen X, Jannach D, Yao L (2023) Causal decision transformer for recommender systems via offline reinforcement learning. In: The 46th International ACM SIGIR Conference on Research and Development in Information Retrieval
Wang F, Zhu H, Srivastava G, Li S, Khosravi MR, Qi L (2022) Robust collaborative filtering recommendation with user-item-trust records. IEEE Trans Comput Soc Syst 9(4):986–996
Zhang S, Yao L, Sun A, Tay Y (2018) Deep learning based recommender system: a survey and new perspectives. ACM Comput Surv 52:1–38
Qi L, Lin W, Zhang X, Dou W, Xu X, Chen J (2023) A correlation graph based approach for personalized and compatible web apis recommendation in mobile app development. IEEE Trans Knowl Data Eng 35(6):5444–5457
Wang S, Xu X, Zhang X, Wang Y, Song W (2022) Veracity-aware and event-driven personalized news recommendation for fake news mitigation. In: Proceedings of the ACM Web Conference, pp 3673–3684
Bourdon PC, Cardinale M, Murray A, Gastin P, Kellmann M, Varley MC, Gabbett TJ, Coutts AJ, Burgess DJ, Gregson W et al (2017) Monitoring athlete training loads: consensus statement. Int J Sports Physiol Perform 12(s2):S2-161
Cao Y, Chen X, Yao L, Wang X, Zhang WE (2020) Adversarial attack and detection on reinforcement learning based recommendation system. In: The 43rd Annual ACM SIGIR Conference on Research and Development in Information Retrieval
Wang N, Wang S, Wang Y, Sheng QZ, Orgun MA (2022) Exploiting intra-and inter-session dependencies for session-based recommendations. World Wide Web 25:425–443
Wu S, Shen S, Xu X, Chen Y, Zhou X, Liu D, Xue X, Qi L (2023) Popularity-aware and diverse web apis recommendation based on correlation graph. IEEE Trans Comput Soc Syst 10(2):771–782
Wang F, Li G, Wang Y, Rafique W, Khosravi MR, Liu G, Liu Y, Qi L (2022) Privacy-aware traffic flow prediction based on multi-party sensor data with zero trust in smart city. ACM Trans Internet Technol. https://doi.org/10.1145/3511904
Minahil Ayub MF, Mahmood K, Kumari S, Sangaiah AK (2021) Lightweight authentication protocol for e-health clouds in IoT-based applications through 5g technology. Digit Commun Netw 7(2):235–244
Yang Y, Yang X, Heidari M, Srivastava G, Khosravi MR, Qi L (2022) Astream: Data-stream-driven scalable anomaly detection with accuracy guarantee in IIoT environment. IEEE Trans Netw Sci Eng. https://doi.org/10.1109/TNSE.2022.3157730
He Q, Tan S, Chen F, Xu X, Qi L, Hei X, Zomaya A, Jin H, Yang Y (2023) Edindex: Enabling fast data queries in edge storage systems. In: The 43rd Annual ACM SIGIR Conference on Research and Development in Information Retrieval
Chambers F, Sandford R (2019) Learning to be human in a digital world: a model of values fluency education for physical education. Sport Educ Soc 24(9):925–938
Yuan L, He Q, Chen F, Zhang J, Qi L, Xu X, Xiang Y, Yang Y (2022) Csedge: Enabling collaborative edge storage for multi-access edge computing based on blockchain. IEEE Trans Parallel Distrib Syst 33(8):1873–1887
Marston HR, van Hoof J, Chua RYJ (2020) Digital transformations in the sport and physical activity landscape: A burgeoning research agenda. Front Sports Active Living 2:100
Caniato F, Caridi M, Crippa L, Moretto A (2012) Environmental sustainability in fashion supply chains: An exploratory case based research. Int J Prod Econ 135(2):659–670
Download references
Acknowledgements
Author information, authors and affiliations.
Chengdu University of Technology, Chengdu, China
Lei Xiao & Yihe Gai
Xindu Research Institute of Educational Science, Chengdu, China
Chengdu University of Information Technology, Chengdu, China
Juntong Liu
Ganxian Middle School (West Campus), Ganzhou, China
Department of Electrical Engineering, Yazd Branch, Islamic Azad University, Yazd, Iran
Mohammad Mahdi Moghimi
You can also search for this author in PubMed Google Scholar
Contributions
L. X. : English writing of the whole paper; Y. C. : Literature searching about “Applications of Cloud Computing in the Sports Industry”; Y. G. : Literature searching about “Challenges of Cloud Computing in the Sports Industry”; J. L. : Literature searching about “Future Trends and Potential Developments”; P. Z. : Proofread the entire manuscript for coherence and grammar; M. M. : Conduct the final review and approve the manuscript before submission.
Corresponding author
Correspondence to Mohammad Mahdi Moghimi .
Ethics declarations
Ethics approval and consent to participate, consent for publication.
All authors agree on the publication of this paper if accepted.
Competing interests
The authors declare no competing interests.
Additional information
Publisher’s note.
Springer Nature remains neutral with regard to jurisdictional claims in published maps and institutional affiliations.
Rights and permissions
Open Access This article is licensed under a Creative Commons Attribution 4.0 International License, which permits use, sharing, adaptation, distribution and reproduction in any medium or format, as long as you give appropriate credit to the original author(s) and the source, provide a link to the Creative Commons licence, and indicate if changes were made. The images or other third party material in this article are included in the article's Creative Commons licence, unless indicated otherwise in a credit line to the material. If material is not included in the article's Creative Commons licence and your intended use is not permitted by statutory regulation or exceeds the permitted use, you will need to obtain permission directly from the copyright holder. To view a copy of this licence, visit http://creativecommons.org/licenses/by/4.0/ .
Reprints and permissions
About this article
Cite this article.
Xiao, L., Cao, Y., Gai, Y. et al. Review on the application of cloud computing in the sports industry. J Cloud Comp 12 , 152 (2023). https://doi.org/10.1186/s13677-023-00531-6
Download citation
Received : 28 July 2023
Accepted : 11 October 2023
Published : 02 November 2023
DOI : https://doi.org/10.1186/s13677-023-00531-6
Share this article
Anyone you share the following link with will be able to read this content:
Sorry, a shareable link is not currently available for this article.
Provided by the Springer Nature SharedIt content-sharing initiative
- Cloud computing
- Sports industry
- Digital transformation
- Performance tracking
Cybersecurity professor and Ph.D. students to present autonomous driving research at ACM MobiSys ’24 Conference
Paper marks first time someone from rit has published at highly selective conference.
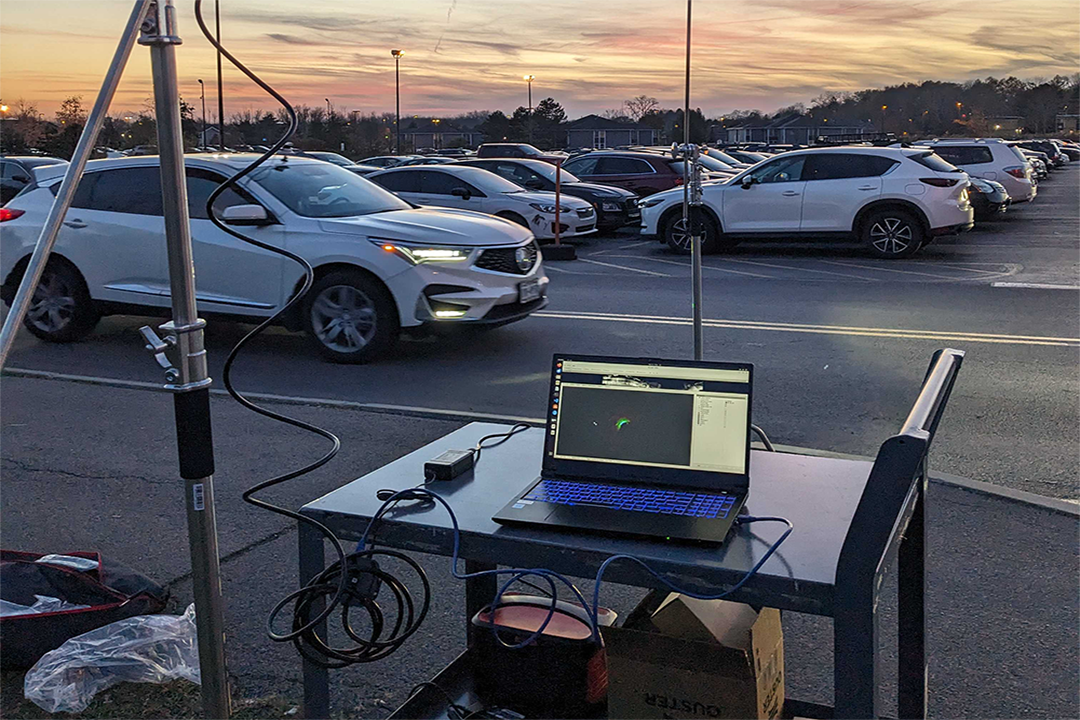
Fawad Ahmad
The paper addresses solutions for occlusions and blind spots that hinder autonomous driving.
Fawad Ahmad , assistant professor in the Department of Computer Science and ESL Global Cybersecurity Institute at RIT, along with computing and information sciences Ph.D. candidates Kaleem Nawaz Khan and Ali Khalid, will present new research at the prestigious ACM International Conference on Mobile Systems, Applications, and Services ( ACM MobiSys’24 Conference ) in Tokyo , Japan in June. The conference is highly selective with an acceptance rate of only 16%. Their paper, VRF: Vehicle Road-side Point Cloud Fusion , marks the first time RIT researchers have published at the conference.
The paper addresses solutions for occlusions and blind spots that hinder autonomous driving. The RIT team, along with collaborators Yash Turkar and Karthik Dantu from the University at Buffalo have developed a system that enables road-side mounted sensors to share and combine their 3D data with the vehicle’s own sensors. This creates a more complete picture of the surroundings, extending the car's "vision" beyond its own limitations.
“Our system, VRF, shares and fuses 3D views from a road-side sensors to a vehicle in real-time with high accuracy,” said Ahmad. “VRF is particularly impressive in that can share and fuse 3D data in under 20 milliseconds, which is crucial for real-time decision making in self-driving cars. At the same time, it maintains high accuracy, achieving positioning within 5 centimeters.”
With VRF, vehicles will have a more complete understanding of their surroundings. With this, vehicles will have more time to react to external events, make more informed decisions, and hence drive safer.
The conference takes place June 3 – 7, 2024.
Recommended News
April 26, 2024
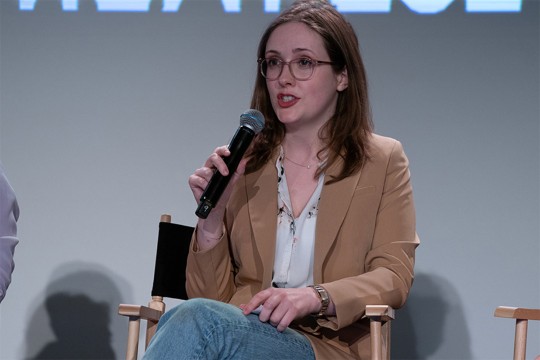
Color Chat: Disney’s Image and Color Engineer Molly Hill
postPerspective talks to Molly Hill '18 (motion picture science), '18 (film and animation), about her experience working as a senior image and color engineer at The Walt Disney Studios.
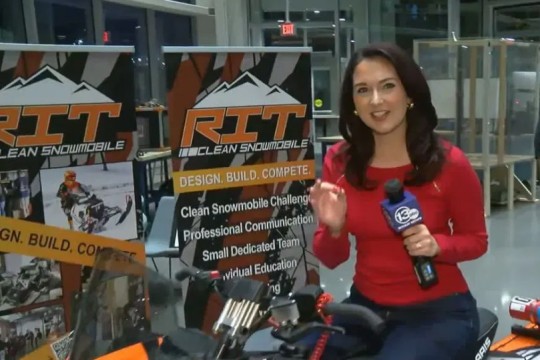
Showcasing creativity and innovation at Imagine RIT
13WHAM/Fox Rochester previews Imagine RIT in eight segments.
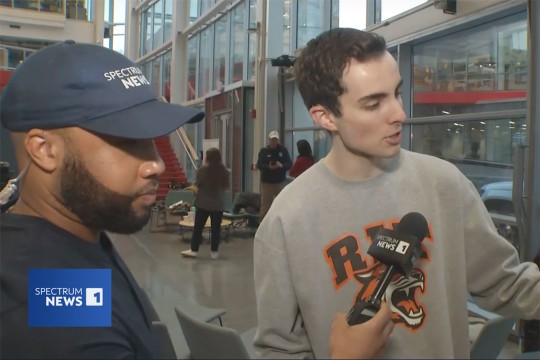
Imagine RIT festival returns to Rochester this weekend
Spectrum News showcases exhibits appearing at Imagine RIT.
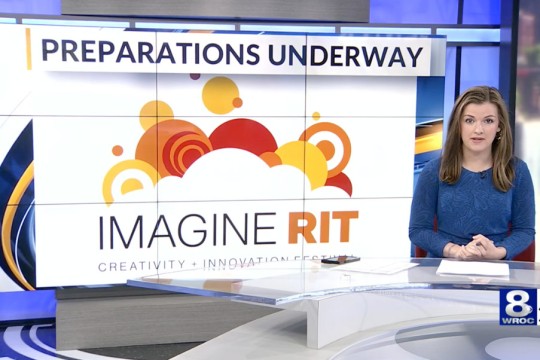
‘I’m hoping this makes a difference in little kids:’ Imagine RIT is this Saturday
WROC-TV highlights exhibits that will be on display at Imagine RIT.

IMAGES
VIDEO
COMMENTS
7.1. Challenges. Via analysis and contrast, we observe that cloud computing security protection work has achieved satisfactory research results. However, many problems remain, which prompt the consideration of a variety of security factors and continuous improvements in defense technology and security strategies. 1.
Research themes analyzed under cloud computing literature are business and technology issues, conceptualization as well as cloud computing application domains. The business issues theme considers research related to the socio-technical aspect of cloud computing such as adoption, cost, privacy, legislation, and ethics.
We also explore the cybersecurity elements associated with cloud computing, focusing on intrusion detection and prevention and understanding how that can be applied in the cloud. Finally, we investigate the future research directions for cloud computing and expand this paper into further articles with experiments and results.
The Journal of Cloud Computing, Advances, Systems and Applications (JoCCASA) has been launched to offer a high quality journal geared entirely towards the research that will offer up future generations of Clouds. The journal publishes research that addresses the entire Cloud stack, and as relates Clouds to wider paradigms and topics.
Cloud computing can be offered as "SaaS (S oftware as a. Service) such as data storag e, computing power and PaaS. (Platform as a Service) such as web devel opment platform.". [1]. Table 2 [5 ...
This paper provides a review of cloud computing technology, cloud models, deployment and cloud applications such as types of applications, reliability, and security. Finally, open research issues ...
The smart collection and sharing of data is an important part of cloud-based systems, since huge amounts of data are being created all the time. This feature allows users to distribute data to particular recip... S. Velmurugan, M. Prakash, S. Neelakandan and Arun Radhakrishnan. Journal of Cloud Computing 2024 13 :86.
2.1. Multi-cloud. The traditional notion of multi-cloud was leveraging resources from multiple data centers of a provider. Then applications were hosted to utilise resources from multiple providers [4], [5].Rightscale estimates that current businesses use an average of six separate clouds. 1 The use of multi-clouds are increasing, but there are hurdles that will need to be overcome.
Abstract -- Cloud computing is a recent development in the world of Information Technology offering IT. capabilities as services. It involves parallel computing, distributed co mputing, grid ...
This research paper presents what cloud computing is, the various cloud models and the overview of the cloud computing architecture, and analyzes the key research challenges present in cloud computing and offers best practices to service providers and enterprises hoping to leverage cloud service to improve their bottom line in this severe economic climate.
In a world with intensive computational services and require optimal solutions, cloud security is a critical concern. As a known fact, the cloud is a diverse field in which data is crucial, and as a result, it invites the dark world to enter and create a virtual menace to businesses, governments, and technology that is facilitated by the cloud. This article addresses the fundamentals of cloud ...
Cloud Computing: Applications, Challenges and Open Issues ... His research interests include cloud computing, recommender systems and big data analytics. Dr. Sanjaya Kumar Panda ... CSI Paper Presenter Award at International Conference and CSI Distinguished Speaker Award. He has published more than 60 papers in reputed journals and conferences.
Research papers for the literature review were downloaded from Scopus database from which specific keywords pertaining to our research topic were used. The keywords that were used are big data, cloud computing, business and management. ... This chapter explores the various application of cloud computing and big data in business and management ...
The authors in have conducted a systematic exploration of serverless computing-related research papers. As they mentioned, their work is not a survey, but it is a supporting source for future research papers. ... Visualizing serverless cloud application logs for program understanding In: 2017 IEEE Symposium on Visual Languages and Human-Centric ...
Well-known cloud services in health care are Microsoft's Health Vault, a cloud-based platform to store and maintain health and fitness data [ 108] or the discontinued of Google Health service [ 109 ]. Of course, cloud computing is a hot topic and new papers are constantly published.
In this paper, we provide an overview of the cloud computing model and discuss its applications for collaboration between academic institutions. Cloud computing is considered a typical paradigm that provides suitable, efficient network login to an appropriate pool of computing resources which can be provided and released with just nominal assiduity and service providers reciprocity. However ...
The paper further compares and reviews different layout model for the discovery of services, selection of services and composition of services in Cloud computing. Recent research trends in service composition are identified and then research about microservices are evaluated and shown in the form of table and graphs. Download Full-text.
A Feature Paper should be a substantial original Article that involves several techniques or approaches, provides an outlook for future research directions and describes possible research applications. Feature papers are submitted upon individual invitation or recommendation by the scientific editors and must receive positive feedback from the ...
1. Introduction. Cloud computing is a paradigm that provides on-demand computing resources such as storage, network, servers, databases, platforms, and so on as a service to its users via the internet, using a pay-as-you-go model [1], [2].Cloud computing has changed the way Micro, Small, and Medium Enterprises provide and use computing services.
This study is intended to quantitatively analyze the research trends in the domain of cloud computing in agriculture by conducting the first ever bibliometric analysis in this domain. This paper analyzed 565 research articles published during 2010-2021 in this area. Cloud computing in agriculture has been found to be a research area with promising growth with various technologies and ...
The author puts forward the problems existing in the application of current cloud computing technology. In order to better play the value of cloud computing technology in computer data processing, the author of this article has given effective measures to ensure cloud computing security, strengthen research and development of hybrid cloud ...
The number of cloud-, edge-, and Internet of Things (IoT)-based applications that produce sensitive and personal data has rapidly increased in recent years. The IoT is a new model that integrates physical objects and the Internet and has become one of the principal technological evolutions of computing. Cloud computing is a paradigm for centralized computing that gathers resources in one place ...
This paper aims to develop a cloud-based intelligent machine monitoring and control system (CIM-MCS) framework, the service structure, and approach to deploying the CIM-MCS in a public cloud infrastructure platform and service provider.
This paper explores the synergies between artificial intellig ence (AI) and cloud computing, focusing on how their integration enhances intellige nce and efficiency across diverse applications ...
Cloud computing is a fast-growing market with consistent advancements throughout India. Gartner predicted end-user spending on cloud services in India would total 7.3 billion USD in 2022 [].Initiatives to develop cloud-skilled talent and encourage a cloud infrastructure in India are helping the country continue its momentum in cloud computing.].
In this paper, we propose an efficient and accurate streaming speech recognition model based on the FastConformer architecture. We adapted the FastConformer architecture for streaming applications through: (1) constraining both the look-ahead and past contexts in the encoder, and (2) introducing an activation caching mechanism to enable the non-autoregressive encoder to operate ...
The transformative impact of cloud computing has permeated various industries, reshaping traditional business models and accelerating digital transformations. In the sports industry, the adoption of cloud computing is burgeoning, significantly enhancing efficiency and unlocking new potentials. This paper provides a comprehensive review of the applications of cloud computing in the sports ...
This approach aims to achieve efficient search by attributes in encrypted datasets. ABSE has a wide range of applications in the fields of privacy protection, data sharing, and cloud computing. In this paper, we describe the trends in development, focusing on enhancing security, improving computational efficiency, and increasing flexibility.
Fawad Ahmad, assistant professor in the Department of Computer Science and ESL Global Cybersecurity Institute at RIT, along with computing and information sciences Ph.D. candidates Kaleem Nawaz Khan and Ali Khalid, will present new research at the prestigious ACM International Conference on Mobile Systems, Applications, and Services (ACM MobiSys'24 Conference) in June.
With the kick-off in March 2024, the Bosch Research team officially began taking part in the project. As the project manager and driver, Bosch Research is developing methods, modules and tools together with the Bosch Power Solutions and Cross-Domain Solutions divisions and the Bosch subsidiary ETAS to ensure resource-saving, energy-efficient and safe vehicle operation.