Information
- Author Services

Initiatives
You are accessing a machine-readable page. In order to be human-readable, please install an RSS reader.
All articles published by MDPI are made immediately available worldwide under an open access license. No special permission is required to reuse all or part of the article published by MDPI, including figures and tables. For articles published under an open access Creative Common CC BY license, any part of the article may be reused without permission provided that the original article is clearly cited. For more information, please refer to https://www.mdpi.com/openaccess .
Feature papers represent the most advanced research with significant potential for high impact in the field. A Feature Paper should be a substantial original Article that involves several techniques or approaches, provides an outlook for future research directions and describes possible research applications.
Feature papers are submitted upon individual invitation or recommendation by the scientific editors and must receive positive feedback from the reviewers.
Editor’s Choice articles are based on recommendations by the scientific editors of MDPI journals from around the world. Editors select a small number of articles recently published in the journal that they believe will be particularly interesting to readers, or important in the respective research area. The aim is to provide a snapshot of some of the most exciting work published in the various research areas of the journal.
Original Submission Date Received: .
- Active Journals
- Find a Journal
- Proceedings Series
- For Authors
- For Reviewers
- For Editors
- For Librarians
- For Publishers
- For Societies
- For Conference Organizers
- Open Access Policy
- Institutional Open Access Program
- Special Issues Guidelines
- Editorial Process
- Research and Publication Ethics
- Article Processing Charges
- Testimonials
- Preprints.org
- SciProfiles
- Encyclopedia
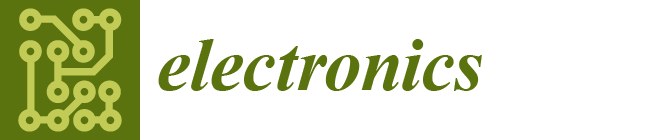
Article Menu

- Subscribe SciFeed
- Recommended Articles
- Google Scholar
- on Google Scholar
- Table of Contents
Find support for a specific problem in the support section of our website.
Please let us know what you think of our products and services.
Visit our dedicated information section to learn more about MDPI.
JSmol Viewer
Artificial intelligence as a disruptive technology—a systematic literature review.
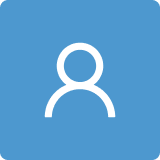
1. Introduction
2. materials and methods.
- First exclusion: ○ Document types—the Editorial Materials and Meeting Abstracts were removed (WoS—38, S—42), leaving 124 (WoS) + 142 (S) = 266 papers; ○ All publishers with only 1 article, as we considered that they did not have a serious approach toward this topic, were removed (WoS—20, S—23), leaving 104 (WoS) + 119 (S). Further, at this stage, the intermediary results (1) were merged into the same file, resulting in 223 articles.
- Second exclusion: ○ With the support of EndNote (used for reference management), it was possible to identify duplicate records (196) originating from the two databases and retain only 1 entry (98). In this manner, we obtained the intermediary results (2), with a total of 125 references.
- Third exclusion: ○ The remaining list was evaluated for relevance based on title, keyword, and abstract analysis, and the articles that did not fit the purpose of the research were eliminated (−28), leaving a total of 97 papers included in the study.
3.1. AI as a Disruptive Technology in Healthcare (Medicine)
3.1.1. disruptive features in the applications to surgery, 3.1.2. disruptive features in the applications to healthcare, 3.2. ai as a disruptive technology in business—logistics and transportation and the labor market, 3.2.1. logistics, 3.2.2. labor market, 3.3. ai as a disruptive technology in agriculture, 3.3.1. smart farming, 3.3.2. digital twins, 3.3.3. the fourth industrial revolution (4ir), 3.4. ai as a disruptive technology in education, 3.5. ai as a disruptive technology with respect to urban development—society, smart cities, and smart government, 3.5.1. disruptive technology’s impact on society, 3.5.2. smart cities, 3.5.3. smart government, 4. discussion and conclusions.
- Enhanced diagnosis, as AI algorithms can examine a large number of medical data to help clinicians make more accurate diagnoses, thus minimizing the possibility of misdiagnosis;
- Personalized medicine, since by using a patient’s particular medical history and genetic data, AI can aid the development of individualized treatment approaches;
- Superior patient outcomes, as AI may be used to track patients, anticipate future health difficulties, and alert medical professionals to take preventative action before significant health issues arise;
- Expedite drug development, because AI can analyze massive volumes of data to hasten the process of developing new drugs and bringing them to market;
- Improved clinical trials, due to the fact that data from clinical trials may be analyzed using AI algorithms, thus assisting in the selection of the most efficient therapies and enhancing patient results.
- The development of AI in healthcare creates ethical issues, such as the issue of responsibility in situations of misdiagnosis or treatment suggestions;
- Limited clinical validity poses a serious problem, because in certain complicated medical situations, AI algorithms may not be as accurate as human specialists and may not be completely verified for assessing all medical disorders;
- Healthcare professionals and patients who are suspicious about the accuracy and dependability of the technology can be resistant to the adoption of AI in the industry.
- For improved supply chain management, AI may aid routing, scheduling, and delivery optimization, which lowers transportation costs and increases delivery times;
- Transportation safety may be improved by using AI to track and improve driver behavior, reduce collisions, and increase road safety;
- AI can enhance logistics efficiency, as it may be used to improve inventory management, optimize storage and picking procedures, and expedite warehouse operations;
- AI is transforming the labor sector by replacing many old manual jobs while also opening up new career prospects in programming and data analysis;
- AI may improve customer experience as it can be used to offer updates on tracking and delivery in real-time, thereby reducing wait times and raising satisfaction;
- AI may aid the maximization of fuel use and the cutting of emissions through effective vehicle scheduling and routing and thus contribute to minimized environmental impacts;
- Many laborious and repetitive tasks will be automated, which may result in fewer jobs and employment possibilities, particularly in sectors such as logistics and transportation;
- As the demand for more high-skilled positions in AI and data analysis increases and fewer low-skilled occupations are automated, the rising usage of AI may worsen already-existing income discrepancies;
- The widespread usage of autonomous cars may result in substantial social and cultural changes, such as the loss of individual driving abilities and the demise of the automobile culture.
- Improved agricultural yields and less waste are possible with the use of AI, which may help farmers optimize planting, irrigation, and fertilization;
- Better resource management may help farmers conserve energy, water, and other resources while decreasing waste and enhancing sustainability;
- Enhanced food safety can be enforced by tracking the whole food production chain from farm to table, while AI can assist in the identification and prevention of food-borne diseases;
- AI can provide real-time analysis of crop, soil, and weather variables, thus enabling farmers to make educated decisions;
- Predictive maintenance may reduce downtime and boost production by predicting when machines and equipment need maintenance.
- AI systems are not immune to technical glitches or malfunctions, and the agricultural sector might suffer significantly as a result, leading to crop losses and possible food shortages;
- The usage of AI in agriculture may have unforeseen environmental effects, including increased pesticide and herbicide use, degraded soil, and the loss of biodiversity.
- A decrease in dropout rates and improved student results due to AI’s ability to detect students’ areas of need and offer focused support;
- Education that is customized to each student’s requirements, interests, and learning preferences may be achieved by using AI to deliver personalized learning experiences for students;
- Improved assessment and feedback due to AI’s ability to automate, enhance, and optimize the grading and feedback process and provide students faster, more precise, and more thorough feedback on their work;
- Lifelong learning is possible because of AI, which can help people continue to learn and advance their expertise.
- Education quality may suffer due to the usage of AI in the classroom when human interaction, creativity, and critical thinking abilities are substituted by automated procedures;
- A lack of critical thinking abilities may be precipitated by AI because the use of AI-powered tools and resources may lessen the necessity for critical thinking and problem-solving abilities, which may retard the development of these skills among students;
- The dependence on technology due to an overreliance on AI in the classroom may result in a lack of creativity, independence, and decision-making abilities, which will reduce students’ capacity to think and work independently.
- An increase in transparency, as by using AI to render governmental processes more open and accountable, individuals will be able to better understand how choices are made;
- Enhanced fraud detection, since AI may be used to identify and stop corruption and fraud in government systems, thus increasing public confidence in these organizations;
- Better resource allocation, because governmental organizations may use AI to more effectively direct resources, including money and staff, to the areas where they are most needed;
- The introduction of predictive analytics, as through the use of AI, government agencies may employ predictive analytics to proactively address prospective concerns before they become problems.
- Privacy issues—Government entities frequently deploy AI algorithms that rely on substantial volumes of personal data, which raises privacy concerns regarding how these data are gathered, kept, and used;
- Lack of transparency—AI technologies employed by government agencies may be opaque, making it difficult for the public to comprehend how and why choices are being made;
- The employment of AI in governmental affairs may result in greater control and surveillance, which may have detrimental effects on free expression and civil rights;
- When an AI system utilized by a government errs or causes harm, it may be challenging to pinpoint the culprit, which results in a lack of accountability.
Author Contributions
Data availability statement, conflicts of interest.
- Bower, L.J.; Christensen, M.C. Disruptive technologies: Catching the wave. Har. Buss. Rev. 1995 , 73 , 43–53. [ Google Scholar ]
- Christensen, C.M.; Bower, J.L. Customer power, strategic investment, and the failure of leading firms. Strateg. Manag. J. 1996 , 17 , 197–218. [ Google Scholar ]
- Christensen, C.M. The Innovator’s Dilemma: When New Technologies Cause Great Firms to Fail ; Harvard Business School Press: Boston, MA, USA, 1997; 179p. [ Google Scholar ]
- O’Connor, S.; Sidorko, P. Chapter 2—The complexities of our informational environment. In Imagine Your Library’s Future ; Chandos Publishing: Oxford, UK, 2010; pp. 33–60. [ Google Scholar ]
- Laukyte, M. Disruptive Technologies and the Sport Ecosystem: A Few Ethical Questions. Philosophies 2020 , 5 , 24. [ Google Scholar ] [ CrossRef ]
- Jekov, B.; Petkova, P.; Parusheva, Y.; Shoikova, E. Disruptive Technologies—Artificial Intelligence and Blockchain in Education. In Proceedings of the 11th Annual International Conference of Education, Research and Innovation (ICERI), Seville, Spain, 7–9 November 2022; pp. 6784–6793. [ Google Scholar ]
- Bongomin, O.; Ocen, G.G.; Nganyi, E.O.; Musinguzi, A.; Omara, T. Exponential Disruptive Technologies and the Required Skills of Industry 4.0. J. Eng. 2020 , 2020 , 4280156. [ Google Scholar ] [ CrossRef ] [ Green Version ]
- Hernández, R. World Standards Day 2018 Puts the Spotlight on the Fourth Industrial Revolution. Available online: https://www.iso.org/news/ref2333.html (accessed on 19 January 2023).
- Bird, K. Four Trends Will Impact ISO’s Future Strategy. Available online: https://www.iso.org/news/ref2436.html (accessed on 19 January 2023).
- Bublitz, F.M.; Oetomo, A.; Sahu, K.S.; Kuang, A.; Fadrique, L.X.; Velmovitsky, P.E.; Nobrega, R.M.; Morita, P.P. Disruptive Technologies for Environment and Health Research: An Overview of Artificial Intelligence, Blockchain, and Internet of Things. Int. J. Environ. Res. Public Health 2019 , 16 , 3847. [ Google Scholar ] [ CrossRef ] [ Green Version ]
- Chang, N.; Zhang, Y.; Lu, D.; Zheng, X.; Xue, J. Is a Disruptive Technology Disruptive? The Readiness Perspective Based on TOE. In Proceedings of the 2020 IEEE International Conference on Industrial Engineering and Engineering Management (IEEM), Singapore, 14–17 December 2020; pp. 893–897. [ Google Scholar ]
- Cartaxo, B.; Pinto, G.; Soares, S. The role of rapid reviews in supporting decision-making in software engineering practice. In Proceedings of the 22nd International Conference on Evaluation and Assessment in Software Engineering—EASE’18, Christchurch, New Zealand, 28–29 June 2018. [ Google Scholar ]
- Christensen, C.M.; McDonald, R.; Altman, E.J.; Palmer, J.E. Disruptive innovation: An intellectual history and directions for future research. J. Manag. Stud. 2018 , 55 , 1043–1078. [ Google Scholar ] [ CrossRef ] [ Green Version ]
- Popescul, D.; Radu, L.D.; Păvăloaia, V.D.; Georgescu, M.R. Psychological Determinants of Investor Motivation in Social Media-Based Crowdfunding Projects: A Systematic Review. Front. Psychol. 2020 , 11 , 588121. [ Google Scholar ] [ CrossRef ]
- Radu, L.D. Disruptive Technologies in Smart Cities: A Survey on Current Trends and Challenges. Smart Cities 2020 , 3 , 1022–1038. [ Google Scholar ] [ CrossRef ]
- VOSViewer. Visualizing Scientific Landscapes. Available online: https://www.vosviewer.com/features/highlights (accessed on 20 July 2022).
- Basmmi, A.B.M.N.; Abd Halim, S.; Saadon, N.A. Comparison of web services for sentiment analysis in social networking sites. In Proceedings of the IOP Conference Series: Materials Science and Engineering, Tangerang, Indonesia, 18–20 November 2020. [ Google Scholar ]
- Abdullah, N.S.D.; Zolkepli, I.A. Sentiment Analysis of Online Crowd Input towards Brand Provocation in Facebook, Twitter, and Instagram. In Proceedings of the International Conference on Big Data and Internet of Thing—BDIOT2017, London, UK, 20–22 December 2017. [ Google Scholar ]
- Byrne, M.; O’Malley, L.; Glenny, A.M.; Pretty, I.; Tickle, M. Assessing the reliability of automatic sentiment analysis tools on rating the sentiment of reviews of NHS dental practices in England. PLoS ONE 2021 , 16 , e0259797. [ Google Scholar ] [ CrossRef ]
- Saura, J.R.; Reyes-Menendez, A.; Alvarez-Alonso, C. Do Online Comments Affect Environmental Management? Identifying Factors Related to Environmental Management and Sustainability of Hotels. Sustainability 2018 , 10 , 3016. [ Google Scholar ] [ CrossRef ] [ Green Version ]
- Pahuriray, A.V.; Basanta, J.D.; Arroyo, J.C.T.; Delima, A.J.P. Flexible Learning Experience Analyzer (FLExA): Sentiment Analysis of College Students through Machine Learning Algorithms with Comparative Analysis using WEKA. Int. J. Emerg. Technol. Adv. Eng. 2022 , 12 , 1–15. [ Google Scholar ] [ CrossRef ]
- Stoiber, C.; Walchshofer, C.; Pohl, M.; Potzmann, B.; Grassinger, F.; Stitz, H.; Streit, M.; Aigner, W. Comparative evaluations of visualization onboarding methods. Vis. Inform. 2022 , 6 , 34–50. [ Google Scholar ] [ CrossRef ]
- Zhuang, Y. Emotional analysis of sentences based on machine learning. In Big Data Analytics for Cyber-Physical System in Smart City ; Advances in Intelligent Systems and Computing; Atiquzzaman, M., Yen, N., Xu, Z., Eds.; Springer: Singapore, 2020; pp. 813–820. [ Google Scholar ] [ CrossRef ]
- Elangovan, K.; JasmineRani, L.P.; Karthikeyan, M.P.; Therasa, M. Analysis of Social Network with Ontology and Deep Sentiment Durability Detection (SSD) Model for Green Community. J. Green Eng. 2020 , 10 , 2661–2677. [ Google Scholar ]
- Monkeylearn. No-Code Text Analytics. Available online: https://monkeylearn.com (accessed on 20 July 2022).
- Contreras, D.; Wilkinson, S.; Alterman, E.; Hervás, J. Accuracy of a pre-trained sentiment analysis (SA) classification model on tweets related to emergency response and early recovery assessment: The case of 2019 Albanian earthquake. Nat. Hazards 2022 , 113 , 403–421. [ Google Scholar ] [ CrossRef ] [ PubMed ]
- Contreras, D.; Wilkinson, S.; Balan, N.; James, P. Assessing post-disaster recovery using sentiment analysis: The case of L’Aquila. Earthq. Spectra 2022 , 38 , 81–108. [ Google Scholar ] [ CrossRef ]
- Sadriu, S.; Nuci, K.P.; Imran, A.S.; Uddin, I.; Sajjad, M. An Automated Approach for Analysing Students Feedback Using Sentiment Analysis Techniques. In Mediterranean Conference on Pattern Recognition and Artificial Intelligence ; Springer International Publishing: Cham, Switzerland, 2021; pp. 228–239. [ Google Scholar ]
- Bredava, A. A Guide to Sentiment Analysis: What Is It and How Does It Work? Available online: https://awario.com/blog/sentiment-analysis (accessed on 15 January 2023).
- Ryman-Tubb, N.F.; Krause, P.; Garn, W. How Artificial Intelligence and machine learning research impacts payment card fraud detection: A survey and industry benchmark. Eng. Appl. Artif. Intell. 2018 , 76 , 130–157. [ Google Scholar ] [ CrossRef ]
- Aria, M.; Cuccurullo, C. Bibliometrix: An R-tool for comprehensive science mapping analysis. J. Informetr. 2017 , 11 , 959–975. [ Google Scholar ] [ CrossRef ]
- International Organization for Standardization. ISO Standardization Foresight Framework—Trend Report 2022. Available online: https://www.iso.org/files/live/sites/isoorg/files/store/en/PUB100470.pdf (accessed on 19 January 2023).
- Dal Mas, F.; Piccolo, D.; Cobianchi, L.; Edvinsson, L.; Presch, G.; Massaro, M.; Skrap, M.; Vajana, A.F.D.; D’Auria, S.D.S.; Bagnoli, C. The Effects of Artificial Intelligence, Robotics, and Industry 4.0 Technologies. Insights from the Healthcare Sector. In Proceedings of the European Conference on the Impact of Artificial Intelligence and Robotics (ECIAIR), EM Normandie Business Sch, Oxford, UK, 31 Octorber–1 November 2022; pp. 88–95. [ Google Scholar ]
- Manickam, P.; Mariappan, S.A.; Murugesan, S.M.; Hansda, S.; Kaushik, A.; Shinde, R.; Thipperudraswamy, S.P. Artificial Intelligence (AI) and Internet of Medical Things (IoMT) Assisted Biomedical Systems for Intelligent Healthcare. Biosensors 2022 , 12 , 562. [ Google Scholar ] [ CrossRef ]
- Kelly, J.T.; Collins, P.F.; McCamley, J.; Ball, L.; Roberts, S.; Campbell, K.L. Digital disruption of dietetics: Are we ready? J. Hum. Nutr. Diet. 2021 , 34 , 134–146. [ Google Scholar ] [ CrossRef ]
- Joda, T.; Yeung, A.W.K.; Hung, K.; Zitzmann, N.U.; Bornstein, M.M. Disruptive Innovation in Dentistry: What It Is and What Could Be Next. J. Dent. Res. 2021 , 100 , 448–453. [ Google Scholar ] [ CrossRef ]
- Ahmad, P.; Alam, M.; Aldajani, A.; Alahmari, A.; Alanazi, A.; Stoddart, M.; Sghaireen, M. Dental Robotics: A Disruptive Technology. Sensors 2021 , 21 , 3308. [ Google Scholar ] [ CrossRef ]
- McBee, M.P.; Wilcox, C. Blockchain Technology: Principles and Applications in Medical Imaging. J. Digit. Imaging 2020 , 33 , 726–734. [ Google Scholar ] [ CrossRef ] [ PubMed ]
- Rasouli, J.J.; Shao, J.N.; Neifert, S.; Gibbs, W.N.; Habboub, G.; Steinmetz, M.P.; Benzel, E.; Mroz, T.E. Artificial Intelligence and Robotics in Spine Surgery. Glob. Spine J. 2021 , 11 , 556–564. [ Google Scholar ] [ CrossRef ] [ PubMed ] [ Green Version ]
- Dorweiler, B.; Wegner, M.; Salem, O.; Murtaja, A.; Schäfers, J.F.; Oberhuber, A. Innovation, disruptive technologies and transformation in vascular surgery. Gefasschirurgie 2022 , 27 , 561–568. [ Google Scholar ] [ CrossRef ]
- Mohanty, K.; Subiksha, S.; Kirthika, S.; Bh, S.; Sokkanarayanan, S.; Bose, P.; Sathiyanarayanan, M.; IEEE. Opportunities of Adopting AI-Powered Robotics to Tackle COVID-19. In Proceedings of the International Conference on COMmunication Systems and NETworkS (COMSNETS), Bangalore, India, 5–9 January 2022; pp. 703–708. [ Google Scholar ]
- Jabarulla, M.Y.; Lee, H.N. A Blockchain and Artificial Intelligence-Based, Patient-Centric Healthcare System for Combating the COVID-19 Pandemic: Opportunities and Applications. Healthcare 2021 , 9 , 1019. [ Google Scholar ] [ CrossRef ]
- Saraswat, D.; Bhattacharya, P.; Verma, A.; Prasad, V.K.; Tanwar, S.; Sharma, G.; Bokoro, P.N.; Sharma, R. Explainable AI for Healthcare 5.0: Opportunities and Challenges. IEEE Access 2022 , 10 , 84486–84517. [ Google Scholar ] [ CrossRef ]
- Mesko, B.; Hetenyi, G.; Gyorffy, Z. Will artificial intelligence solve the human resource crisis in healthcare? BMC Health Serv. Res. 2018 , 18 , 545. [ Google Scholar ] [ CrossRef ] [ Green Version ]
- Maliha, G.; Gerke, S.; Cohen, G.; Parikh, R.B. Artificial Intelligence and Liability in Medicine: Balancing Safety and Innovation. Milbank Q. 2021 , 99 , 629–647. [ Google Scholar ] [ CrossRef ]
- Khatab, Z.; Yousef, G.M. Disruptive innovations in the clinical laboratory: Catching the wave of precision diagnostics. Crit. Rev. Clin. Lab. Sci. 2021 , 58 , 546–562. [ Google Scholar ] [ CrossRef ]
- Brunelle, F.; Brunelle, P. Artificial Intelligence and Medical Imaging: Definition, State of the Art and Perspectives. Bull. Acad. Natl. Med. 2019 , 203 , 683–687. [ Google Scholar ]
- Garbuio, M.; Lin, N. Artificial Intelligence as a Growth Engine for Health Care Startups: Emerging Business Models. Calif. Manag. Rev. 2019 , 61 , 59–83. [ Google Scholar ] [ CrossRef ] [ Green Version ]
- Prakash, S.; Balaji, J.N.; Joshi, A.; Surapaneni, K.M. Ethical Conundrums in the Application of Artificial Intelligence (AI) in Healthcare—A Scoping Review of Reviews. J. Pers. Med. 2022 , 12 , 1914. [ Google Scholar ] [ CrossRef ] [ PubMed ]
- Mesko, B. Future Directions of Digital Health. In Digital Health: Scaling Healthcare to the World ; Rivas, H., Wac, K., Eds.; Springer: Berlin/Heidelberg, Germany, 2018; pp. 339–363. [ Google Scholar ] [ CrossRef ]
- Ojo, A. Next Generation Government—Hyperconnected, Smart and Augmented. In Proceedings of the 20th IFIP WG 5.5 Working Conference on Virtual Enterprises (PRO-VE), Turin, Italy, 23–25 September 2019; pp. 285–294. [ Google Scholar ]
- Ljepava, N. AI-Enabled Marketing Solutions in Marketing Decision Making: AI Application in Different Stages of Marketing Process. TEM J. 2022 , 11 , 1308–1315. [ Google Scholar ] [ CrossRef ]
- Wiegandt, D. Blockchain and Smart Contracts and the Role of Arbitration. J. Int. Arbitr. 2022 , 39 , 671–690. [ Google Scholar ] [ CrossRef ]
- Dong, C.W.; Akram, A.; Andersson, D.; Arnas, P.O.; Stefansson, G. The impact of emerging and disruptive technologies on freight transportation in the digital era: Current state and future trends. Int. J. Logist. Manag. 2021 , 32 , 386–412. [ Google Scholar ] [ CrossRef ]
- Noor, A. Adoption of Blockchain Technology Facilitates a Competitive Edge for Logistic Service Providers. Sustainability 2022 , 14 , 15543. [ Google Scholar ] [ CrossRef ]
- Ugochukwu, N.A.; Goyal, S.B.; Rajawat, A.S.; Islam, S.M.N.; He, J.; Aslam, M. An Innovative Blockchain-Based Secured Logistics Management Architecture: Utilizing an RSA Asymmetric Encryption Method. Mathematics 2022 , 10 , 4670. [ Google Scholar ] [ CrossRef ]
- Zondervan, N.A.; Tolentino-Zondervan, F.; Moeke, D. Logistics Trends and Innovations in Response to COVID-19 Pandemic: An Analysis Using Text Mining. Processes 2022 , 10 , 2667. [ Google Scholar ] [ CrossRef ]
- Hong, Z.F.; Zhang, H.Y.; Gong, Y.M.; Yu, Y.G. Towards a multi-party interaction framework: State-of-the-art review in sustainable operations management. Int. J. Prod. Res. 2022 , 60 , 2625–2661. [ Google Scholar ] [ CrossRef ]
- Yu, H. Modeling a remanufacturing reverse logistics planning problem: Some insights into disruptive technology adoption. Int. J. Adv. Manuf. Technol. 2022 , 123 , 4231–4249. [ Google Scholar ] [ CrossRef ]
- Jia, W.; Wang, S.; Xie, Y.; Chen, Z.; Gong, K. Disruptive technology identification of intelligent logistics robots in AIoT industry: Based on attributes and functions analysis. Syst. Res. Behav. Sci. 2022 , 39 , 557–568. [ Google Scholar ] [ CrossRef ]
- Cukier, W. Disruptive processes and skills mismatches in the new economy Theorizing social inclusion and innovation as solutions. J. Glob. Responsib. 2019 , 10 , 211–225. [ Google Scholar ] [ CrossRef ]
- Oosthuizen, R.M. The Fourth Industrial Revolution—Smart Technology, Artificial Intelligence, Robotics and Algorithms: Industrial Psychologists in Future Workplaces. Front. Artif. Intell. 2022 , 5 , 913168. [ Google Scholar ] [ CrossRef ] [ PubMed ]
- Khatri, S.; Pandey, D.K.; Penkar, D.; Ramani, J. Impact of Artificial Intelligence on Human Resources. In Data Management, Analytics and Innovation ; Advances in Intelligent Systems and Computing; Sharma, N., Chakrabarti, A., Balas, V., Eds.; Springer: Singapore, 2019. [ Google Scholar ]
- Burden, K. Impact of disruptive technologies on sourcing and outsourcing transactions. Comput. Law Secur. Rev. 2018 , 34 , 886–889. [ Google Scholar ] [ CrossRef ]
- Miklosik, A.; Evans, N. Impact of Big Data and Machine Learning on Digital Transformation in Marketing: A Literature Review. IEEE Access 2020 , 8 , 101284–101292. [ Google Scholar ] [ CrossRef ]
- Koizumi, S. The Light and Shadow of the Fourth Industrial Revolution. In Innovation beyond Technology: Science for Society and Interdisciplinary Approaches ; Lechevalier, S., Ed.; Springer: Berlin/Heidelberg, Germany, 2019; pp. 63–86. [ Google Scholar ] [ CrossRef ]
- Rosales, M.A.; Magsumbol, J.A.V.; Palconit, M.G.B.; Culaba, A.B.; Dadios, E.P. Artificial Intelligence: The Technology Adoption and Impact in the Philippines. In Proceedings of the IEEE 12th International Conference on Humanoid, Nanotechnology, Information Technology, Communication and Control, Environment, and Management (HNICEM), Manila, Philippines, 3–7 December 2020. [ Google Scholar ]
- Anitha, J.; Saranya, N. Cassava Leaf Disease Identification and Detection Using Deep Learning Approach. Int. J. Comput. Commun. Control 2022 , 17 , 1–7. [ Google Scholar ] [ CrossRef ]
- Ortola, A. Unlocking the full potential of artificial intelligence in PH. Philippine Daily Inquirer , 24 June 2019. [ Google Scholar ]
- Karimanzira, D.; Rauschenbach, T. An intelligent management system for aquaponics. at-Automatisierungstechnik 2021 , 69 , 345–350. [ Google Scholar ] [ CrossRef ]
- Jacobs, M.; Remus, A.; Gaillard, C.; Menendez, H.M.; Tedeschi, L.O.; Neethirajan, S.; Ellis, J.L. ASAS-NANP symposium: Mathematical modeling in animal nutrition: Limitations and potential next steps for modeling and modelers in the animal sciences. J. Anim. Sci. 2022 , 100 , skac132. [ Google Scholar ] [ CrossRef ]
- De Bernardi, P.; Azucar, D.; Forliano, C.; Franco, M. Innovative and Sustainable Food Business Models. In Innovation in Food Ecosystems: Entrepreneurship for a Sustainable Future ; Springer: Berlin/Heidelberg, Germany, 2020; pp. 189–221. [ Google Scholar ] [ CrossRef ]
- Neethirajan, S.; Kemp, B. Digital Twins in Livestock Farming. Animals 2021 , 11 , 1008. [ Google Scholar ] [ CrossRef ]
- Spanaki, K.; Sivarajah, U.; Fakhimi, M.; Despoudi, S.; Irani, Z. Disruptive technologies in agricultural operations: A systematic review of AI-driven AgriTech research. Ann. Oper. Res. 2022 , 308 , 491–524. [ Google Scholar ] [ CrossRef ]
- Kamble, S.; Gunasekaran, A.; Sharma, R. Modeling the blockchain enabled traceability in agriculture supply chain. Int. J. Inf. Manag. 2020 , 52 , 101967. [ Google Scholar ] [ CrossRef ]
- Wamba, S.F.; Queiroz, M.M. Blockchain in the operations and supply chain management: Benefits, challenges and future research opportunities. Int. J. Inf. Manag. 2020 , 52 , 102064. [ Google Scholar ] [ CrossRef ]
- Moore, W.B.; Felo, A. The evolution of accounting technology education: Analytics to STEM. J. Educ. Bus. 2022 , 97 , 105–111. [ Google Scholar ] [ CrossRef ]
- Brito, C.R.; Ciampi, M.M.; Sluss, J.J.; Santos, H.D. Trends in Engineering Education: A Disruptive View for not so far Future. In Proceedings of the 18th International Conference on Information Technology Based Higher Education and Training (ITHET), Magdeburg, Germany, 26–27 September 2019. [ Google Scholar ]
- Ghani, A. Engineering education at the age of Industry 5.0—Higher education at the crossroads. World Trans. Eng. Technol. Educ. 2022 , 20 , 112–117. [ Google Scholar ]
- Bühler, M.M.; Jelinek, T.; Nübel, K. Training and Preparing Tomorrow’s Workforce for the Fourth Industrial Revolution. Educ. Sci. 2022 , 12 , 782. [ Google Scholar ] [ CrossRef ]
- Chaka, C. Is Education 4.0 a Sufficient Innovative, and Disruptive Educational Trend to Promote Sustainable Open Education for Higher Education Institutions? A Review of Literature Trends. Front. Educ. 2022 , 7 , 226. [ Google Scholar ] [ CrossRef ]
- Choi, T.-M.; Kumar, S.; Yue, X.; Chan, H.-L. Disruptive Technologies and Operations Management in the Industry 4.0 Era and Beyond. Prod. Oper. Manag. 2022 , 31 , 9–31. [ Google Scholar ] [ CrossRef ]
- Bengoechea, J.; Bell, A. The Impact of 21st Century Technology in Higher Education: The Role of Artificial Intelligence. Int. J. Educ. Pedagog. Sci. 2022 , 16 , 493–496. [ Google Scholar ]
- Miao, F.; Wayne, H.; Ronghuai, H.; Hui, Z. AI and Education: A Guidance for Policymakers ; UNESCO Publishing: Paris, France, 2021. [ Google Scholar ]
- Pornpongtechavanich, P.; Eambunnapong, K.; Daengsi, T.; Nilsook, P. Critical success factors for smart-professional disruptor in university. Int. J. Eval. Res. Educ. 2022 , 11 , 1696–1703. [ Google Scholar ] [ CrossRef ]
- Molenaar, I. Towards hybrid human-AI learning technologies. Eur. J. Educ. 2022 , 57 , 632–645. [ Google Scholar ] [ CrossRef ]
- Holmes, W.; Tuomi, I. State of the art and practice in AI in education. Eur. J. Educ. 2022 , 53 , 542–570. [ Google Scholar ] [ CrossRef ]
- Zeeshan, K.; Hämäläinen, T.; Neittaanmäki, P. Internet of Things for Sustainable Smart Education: An Overview. Sustainability 2022 , 14 , 4293. [ Google Scholar ] [ CrossRef ]
- Ciolacu, M.; Svasta, P.M.; Berg, W.; Popp, H.; IEEE. Education 4.0 for Tall Thin Engineer in a Data Driven Society. In Proceedings of the 23rd IEEE International Symposium for Design and Technology in Electronic Packaging (SIITME), Constanta, Romania, 26–29 October 2022; pp. 438–443. [ Google Scholar ]
- Ahmad, T. Scenario based approach to re-imagining future of higher education which prepares students for the future of work. High. Educ. Ski. Work-Based Learn. 2019 , 10 , 217–238. [ Google Scholar ] [ CrossRef ]
- Yang, K.; Shi, Y.M.; Zhou, Y.; Yang, Z.P.; Fu, L.Q.; Chen, W. Federated Machine Learning for Intelligent IoT via Reconfigurable Intelligent Surface. IEEE Netw. 2020 , 34 , 16–22. [ Google Scholar ] [ CrossRef ]
- Kasinathan, P.; Pugazhendhi, R.; Elavarasan, R.M.; Ramachandaramurthy, V.K.; Ramanathan, V.; Subramanian, S.; Kumar, S.; Nandhagopal, K.; Raghavan, R.R.V.; Rangasamy, S.; et al. Realization of Sustainable Development Goals with Disruptive Technologies by Integrating Industry 5.0, Society 5.0, Smart Cities and Villages. Sustainability 2022 , 14 , 15258. [ Google Scholar ] [ CrossRef ]
- Sgantzos, K.; Grigg, I. Artificial Intelligence Implementations on the Blockchain. Use Cases and Future Applications. Future Internet 2019 , 11 , 170. [ Google Scholar ] [ CrossRef ] [ Green Version ]
- Corchado, J.M.; Trabelsi, S. Advances in Sustainable Smart Cities and Territories. Electronics 2022 , 11 , 1280. [ Google Scholar ] [ CrossRef ]
- Xu, Y. Programmatic Dreams: Technographic Inquiry into Censorship of Chinese Chatbots. Soc. Media + Soc. 2018 , 4 , 2056305118808780. [ Google Scholar ] [ CrossRef ] [ Green Version ]
- Zweispace. Zweispace Won the Best Startup Award for Real Estate and Construction Batch at the Plug and Play Summer Summit 2020. Available online: https://en.zweispace.co.jp/2020/08/04/zweispace-won-the-best-startup-award-for-real-estate-and-construction-batch-at-the-plug-and-play-summer-summit-2020/ (accessed on 4 August 2022).
- Ortega-Fernández, A.; Martín-Rojas, R.; García-Morales, V. Artificial Intelligence in the Urban Environment: Smart Cities as Models for Developing Innovation and Sustainability. Sustainability 2020 , 12 , 7860. [ Google Scholar ] [ CrossRef ]
- Schintler, L.A.; McNeely, C.L. Artificial intelligence, institutions, and resilience: Prospects and provocations for cities. J. Urban Manag. 2022 , 11 , 256–268. [ Google Scholar ] [ CrossRef ]
- Fu, G.; Jin, Y.; Sun, S.; Yuan, Z.; Butler, D. The role of deep learning in urban water management: A critical review. Water Res. 2022 , 223 , 118973. [ Google Scholar ] [ CrossRef ]
- Ullah, F. Smart Tech 4.0 in the Built Environment: Applications of Disruptive Digital Technologies in Smart Cities, Construction, and Real Estate. Buildings 2022 , 12 , 1516. [ Google Scholar ] [ CrossRef ]
- Ullah, F.; Sepasgozar, S.M.E.; Wang, C.X. A Systematic Review of Smart Real Estate Technology: Drivers of, and Barriers to, the Use of Digital Disruptive Technologies and Online Platforms. Sustainability 2018 , 10 , 3142. [ Google Scholar ] [ CrossRef ] [ Green Version ]
- Ghobakhloo, M. Industry 4.0, digitization, and opportunities for sustainability. J. Clean. Prod. 2020 , 252 , 119869. [ Google Scholar ] [ CrossRef ]
- Khanna, A.; Sah, A.; Bolshev, V.; Jasinski, M.; Vinogradov, A.; Leonowicz, Z.; Jasiński, M. Blockchain: Future of e-Governance in Smart Cities. Sustainability 2021 , 13 , 11840. [ Google Scholar ] [ CrossRef ]
- McLean, J.; Mackenzie, R. Digital justice in Australian visa application processes? Altern. Law J. 2019 , 44 , 291–296. [ Google Scholar ] [ CrossRef ]
- Ishengoma, F.R.; Shao, D.; Alexopoulos, C.; Saxena, S.; Nikiforova, A. Integration of artificial intelligence of things (AIoT) in the public sector: Drivers, barriers and future research agenda. Digit. Policy Regul. Gov. 2022 , 24 , 449–462. [ Google Scholar ] [ CrossRef ]
- Rapanyane, M.B.; Sethole, F.R. The rise of artificial intelligence and robots in the 4th Industrial Revolution: Implications for future South African job creation. Contemp. Soc. Sci. 2020 , 15 , 489–501. [ Google Scholar ] [ CrossRef ]
- Mesko, B. The role of artificial intelligence in precision medicine. Expert Rev. Precis. Med. Drug Dev. 2017 , 2 , 239–241. [ Google Scholar ] [ CrossRef ]
- Brougham, D.; Haar, J. Smart Technology, Artificial Intelligence, Robotics, and Algorithms (STARA): Employees’ perceptions of our future workplace. J. Manag. Organ. 2018 , 24 , 239–257. [ Google Scholar ] [ CrossRef ] [ Green Version ]
- Frey, C.B.; Osborne, M.A. The future of employment: How susceptible are jobs to computerisation? Technol. Forecast. Soc. Chang. 2017 , 114 , 254–280. [ Google Scholar ] [ CrossRef ]
Click here to enlarge figure
Manuscript-Selected Keyword | Frequency in Abstract | Frequency in Keywords | Frequency in Titles | Total | Frequency (Total) | Rank |
---|---|---|---|---|---|---|
AI | 194 | 17 | 41 | 252 | 481 | 1 |
Artificial intelligence | 125 | 66 | 38 | 229 | ||
IoT | 33 | 11 | 6 | 50 | 89 | 2 |
Internet of things | 27 | 7 | 5 | 39 | ||
BlockChain | 55 | 11 | 9 | 75 | 75 | 3 |
6G | 16 | 15 | 4 | 35 | 35 | 4 |
5G | 9 | 5 | 3 | 17 | 17 | 5 |
3D Printing | 5 | 3 | 4 | 12 | 12 | 6 |
Cluster | Domain-Related Keywords | Technology-Related Keywords |
---|---|---|
Blue | Healthcare (Digital heath), Medicine, Dentistry | AI (Machine learning), Robotics, digitalization, new technology |
Green | Business, Organizations, Logistics, Government | AI (Augmented reality), Digital, Automation, RPA |
Yellow | Agriculture, Smart farming, Industry | AI (Deep learning), Internet technology, Internet of things |
Red | Education, Society, Smart city, Environment, | AI (applications), Cloud computing, Big Data, Blockchain |
Aspect | Positive Impact | Negative Impact |
---|---|---|
Diagnosis | Improved accuracy, velocity, and consistency of medical actions. | Limited clinical validity in certain complex cases. |
Treatment | Personalized treatment plans for patient’s particular situation. | Ethical concerns and accountability in cases of misdiagnosis. |
Clinical Trials | Are efficient and cost-effective due to AI. | - |
Predictive Medicine | Improved early intervention, reliable and fast screening. | - |
Healthcare Access | Improved access to medical services due to lower costs. | - |
Operations | Streamlined workflows and resource management. | Job losses in certain areas. |
Research | Enhanced medical research. | - |
Data Privacy | - | Concerns over data privacy and security. |
Adoption | - | Resistance to change and skepticism from healthcare employees |
Cost | - | High cost, in the short run, for development and implementation. |
Impact on | Disruptive Feature | Disruptive Technologies | Reference |
---|---|---|---|
Healthcare: patient data such as laboratory results, wearable devices’ data, genomic data, medical imaging | Has positive aspects such as improved management of patient medical history but also generates plenty of legal and ethical issues. | Blockchain and AI | [ ] |
Medicine: guided surgery and advanced imaging | Development of new surgical methods based on previous procedures, a revolution in spinal care via AI, Robotic assistance decreases surgeon fatigue. | AI: Robots, ML, and DL | [ , , ] |
Healthcare in COVID-19 pandemic | Robots used intensively for distribution of food and medicine to ill persons, assisting elderly people, biopsies (with Endoscopy bots); 3D prosthetics printing. | AI: Robots and 3D printing AI and blockchain | [ , ] |
Healthcare support in HR process of hiring medical personnel | AI aids HR with respect to finding and vetting potential healthcare workers. In addition, it has great potential as a cognitive assistant but cannot replace humans. | AI | [ ] |
Healthcare by Healthcare 5.0 | EXAI is a revolutionary AI innovation that enhances clinical healthcare procedures and provides transparency to predictive analysis. | AI: Explainable AI, Healthcare 5.0 | [ ] |
Medicine by Surgery 4.0 | The digital transformation of surgery. | AI: AR/VR, 3D printing | [ ] |
Dentistry | Revolutionizes dental medicine’s diagnostic and therapeutic procedures. | AI | [ , ] |
Medicine: ethical issues | AI algorithms can be inaccurate, which leads to low clinical judgment and unfavorable patient outcomes. | AI and ML | [ ] |
Disruptive Technology | Impact on Logistics | Impacts on Transportation | References |
---|---|---|---|
AI | Terminal operation (e.g., identifying ill passengers and luggage controls to facilitate efficiency in terms of human logistics within railways and airports), congestion mitigation, and traffic flow prediction | Vehicle routing, optimal route suggestion | [ , ] |
Autonomous vehicles | Indirect impacts | Individual vehicles and groups of vehicles traveling together, e.g., platoons; features wireless communication | [ ] |
Automated robots | Short-distance deliveries | Mainly based on economic viability, accessibility to the public, acceptance by different stakeholders, and benefits associated with their use | [ , ] |
Drones | Low impact | Provide access to unreachable areas and future use in last-mile delivery | [ , ] |
3D printing | Disrupts traditional manufacturing and logistics processes | Indirect impacts/consequences | [ , , ] |
Big Data | Enhance collaborative shipping, forecast demand, and manage supply chains | Real-time traffic flows, aid the navigation of ocean vessels, forecast train delays, adjust ocean vessel speeds, manage infrastructure maintenance, optimize truck fill rates, increase transport safety, locate charging stations, improve parking policies | [ ] |
IoT | Low impact | IoT is the backbone that supports vehicle-to-vehicle, vehicle-to-person, and vehicle-to-infrastructure communications | [ ] |
Blockchain | Exacerbates data-sharing provenance issues, ownership registry issues, and issues including trust, privacy, and transparency | Track-and-trace affordances; credit evaluation; increases transportation visibility; strengthens transportation security—including with respect to shipping and ports—regarding the tracking of goods; reduces inefficiencies due to extensive paperwork; and reduces disputes regarding logistics of goods | [ ] |
Electric Vehicles | Impacts on urban consolidation centers, off-peak distribution (wherein its environmental benefits are important) | City deliveries involving small vehicles—vans and bikes—as well as medium-duty trucks and also heavy-duty trucks | [ , ] |
Aspect | Positive Impact(s) | Negative Impact(s) |
---|---|---|
Fleet Management | Decreased downtime; increased efficiency through vehicle allocation optimization. | System failures may occur; increased costs for installation and maintenance may be incurred. |
Product’s delivery | Maximized efficiency; minimized delivery time and costs. | Delivery workers may lose their jobs. |
Supply Chain Management | Route optimization; reduced consumption; facilitates cleaner environment. | Ethical issues such as lack of accountability for supply chain disruptions. |
Traffic Management | Optimized traffic flow; reduced congestion; optimized routes. | Privacy concerns due to surveillance; potential job losses for traffic officers. |
Environmental Sustainability | Reduced carbon emissions; increased efficiency of fuel consumption. | Dependence on technology leads to greater energy consumption. |
Safeness | Superior driver assistance; fewer accidents. | Ethical issues regarding autonomous vehicles; potential job losses for drivers. |
Impact on | Disruptive Feature | Disruptive Technologies | Reference |
---|---|---|---|
Logistics and Transportation | Impacts L and T and the opportunities to support management decisions in the L industry. | Autonomous vehicles, automated robots, drones, 3D printing, big data, IoT, blockchain, electric vehicles | [ , ] |
Enhance the sustainability and resilience of L and green L (green distribution, reverse L, and green warehousing) | Blockchain, Internet of Things (IoT), smart robots | [ , , , ] | |
Logistics by LSP | Expand the boundaries of supply chain traceability, transparency, accuracy, and safety | Blockchain, IoT, and bigdata | [ ] |
Labor market: new jobs created | Require specialized technical knowledge to develop and operate them; new jobs are being created; new skills need to be developed | NLP, ML, reasoning, computer vision | [ , ] |
Labor market: jobs taken | Replacing human laborers to reduce expenditures | RPA | [ ] |
Aspect | Positive Impact(s) | Negative Impact(s) |
---|---|---|
Job Creation | New AI-related jobs. | Job losses due to tasks replaced by AI. |
Skill Development | Opportunities for skill development and upskilling. | Reduced demand for certain skills and job losses for workers. |
Productivity | Automation increases efficiency and reduces manual labor. | Increased dependence on technology. |
Wage disparities | Wage raises for high-skilled workers. | Wage decreases for low-skilled workers. |
Working Conditions | Improved safety; reduced physical labor. | Technological addiction; ethical implications related to AI. |
Sectors | Positive Impacts | Negative Impacts |
---|---|---|
Agricultural research | Innovations in predictive analytics, disease control, and breeding programs. | Disparities with respect to access to research. |
Labor force in Agriculture | Reduced manual labor tasks | Job losses due to task automation. |
Livestock management | Improved decision making through data analysis | Privacy concerns regarding data collection and analysis. |
Crop production and Precision agriculture | Increased crop yields and profitability. | Potential system failures; high costs of implementation. |
Smart farming | Water is saved via smart irrigation; crop diseases can be identified on site. | Limited access to Internet; chaotic regional development. |
Impact on | Disruptive Feature | Disruptive Technologies | Reference |
---|---|---|---|
Farming | Smart irrigation systems (Skydrop) | AI and weather forecast | [ ] |
Keeps track of the mental and emotional states of animals | AI-based recognition technology | [ , ] | |
Innovations in the market of aquaponics: intelligent management system for aquaculture | AI | [ , ] | |
Krops: disrupts the old buying and selling practices | AI techniques and Azzure | [ , ] | |
Identification of pest and crop diseases and provision of vigor and water stress indices | AI-based image recognition via satellite or drone image analysis | [ , ] | |
Smart farming and urban farming | AI and blockchain | [ ] | |
Agriculture Supply Chain (ASC) | Real-time, data-driven ASC | Blockchain, AI, IoT, and 3D printing | [ , ] |
Impact on | Disruptive Feature | Disruptive Technologies | Reference |
---|---|---|---|
Education: management of academic organizations | Lack of physical (human) supervisor. | AI, blockchain | [ , ] |
Education: Sports | AI poses unethical concerns involving the transformation of athletes into cyborgs (1) and the robotization of training and judgement processes (2). | AI: robotics, enhanced vision, AR/VR | [ ] |
Education: emergence of Education 4.0 | A lack of interaction between students and professors, robotization of education. | AI, robotics, blockchain, 3D printing, 5G, IoT, digital twins, and augmented reality | [ , , , ] |
Education 4.0 should integrate Industry 4.0 concepts into academic curricula | Rapid and massive disruption to all sectors in terms of demand for occupations and skills | 13 key technologies: IoT, big data, 3D printing, cloud computing, AR, VR/AR, cyber-physical systems, AI, smart sensors, simulation, nanotechnology, drones, and biotechnology | [ ] |
Education: Instructors and students | Enhances the integrity of educational experiences | IoT | [ ] |
Education: engineering students and professors | Generates a paradigm shift in engineering education | 4IR boosted by AI | [ , ]. |
Education: dentistry students | Dental students can be trained using full-body robots | Robotics | [ , ] |
Aspect | Positive Impact | Negative Impact |
---|---|---|
Personalized Learning | Customized learning experiences for students. | Eliminates social interactions. |
Skill Development | AI-based skill development for instructors and students. | Reduced demand for certain skills and job losses for educators. |
Teaching | Improved teaching efficiency and effectiveness. | Decreased face-to-face interaction; automation leads to job losses for educators. |
Assessment | More accurate and efficient assessments. | Lack of accountability for assessment outcomes, i.e., who is to blame in case of errors? |
Equity | Improved equity in education; reduced educational disparities. | Data collection and analysis create privacy concerns. |
Accessibility | Improved accessibility to education; reduced costs of education. | Dependence on technology may lead to potential system failures and unavailability of data. |
Aspect | Positive Impact | Negative Impact |
---|---|---|
Employment | decrease in manual labor; development of new jobs. | some professions may become obsolete; pay gap between low- and high-skilled individuals. |
Healthcare | enhanced patient care; lower medical expenses. | health data privacy issues; job losses for healthcare workers. |
Education | customized learning; minimized educational costs. | technology dependency; possible loss of teaching positions. |
Entertainment | enhanced production and distribution of content. | reduced face-to-face engagement and social skills. |
Communication | high accessibility; fewer language obstacles | addiction to technology. |
Privacy | enhanced data security | privacy issues due to data collection and analysis |
Aspect Impacted | Positive Impact | Negative Impact |
---|---|---|
Urban planning | effective urban planning. | benefit- and access-related disparities. |
Environmental sustainability | better air quality; low carbon emissions. | technological addiction may lead to system breakdowns. |
Traffic management | improved traffic flow; less congestion; route optimization. | surveillance privacy concerns; job losses for traffic officers. |
Waste management | enhanced waste collection and management; waste reduction. | job loss; potential system failures. |
Citizen’s Satisfaction | improved quality of life. | ethical and moral issues. |
Energy management | Energy benefits via AI-monitored energy usage; reduced energy consumption. | AI systems consume more energy, which might negate any environmental benefits. |
Aspects | Positive Impact | Negative Impact |
---|---|---|
Public Service Delivery | reduced wait times; customized public services. | privacy issues concerning data collection; job losses for government employees. |
Public Safety | predictive policing; improved emergency response times. | ethical concerns regarding biased algorithms and predictive policing. |
Public Decision Making | high accuracy and reduced bias; enhanced data analysis. | Algorithm-related ethical concerns; lack of accountability for decisions made by AI. |
Elections | increased participation; reduced voting fraud. | Algorithm-related ethical concerns; lack of accountability for AI decisions. |
Public Fraud Detection | high accuracy of detection; fewer fraudulent activities. | data collection concerns. |
Impact on | Disruptive Feature(s) | Disruptive Technologies | Reference |
---|---|---|---|
Society | It is an essential tool to national security and a major element of achieving the country’s dream of national rejuvenation | AI chatbots: AI and big data | [ ] |
Society 5.0—a highly integrated cyber and physical platform—is constructed, with people playing a prominent role | Industry 5.0/Society 5.0 | [ ] | |
AIoT is disrupting the public sector. | Artificial Intelligence of Things (AIoT) | [ ] | |
Smart cities | Precipitates both positive and negative effects in the business world | Blockchain combined with AI, Cloud and IoT | [ ] |
Integration between smart cities, construction, and real estate | Smart Tech 4.0 | [ , ] | |
The development of a prosperous and powerful smart city economy | CNN and/or AIA | [ ] | |
Smart government | humans replaced by machines (negation of 3000 jobs) | AI, RPA, and Big data | [ ] |
The statements, opinions and data contained in all publications are solely those of the individual author(s) and contributor(s) and not of MDPI and/or the editor(s). MDPI and/or the editor(s) disclaim responsibility for any injury to people or property resulting from any ideas, methods, instructions or products referred to in the content. |
Share and Cite
Păvăloaia, V.-D.; Necula, S.-C. Artificial Intelligence as a Disruptive Technology—A Systematic Literature Review. Electronics 2023 , 12 , 1102. https://doi.org/10.3390/electronics12051102
Păvăloaia V-D, Necula S-C. Artificial Intelligence as a Disruptive Technology—A Systematic Literature Review. Electronics . 2023; 12(5):1102. https://doi.org/10.3390/electronics12051102
Păvăloaia, Vasile-Daniel, and Sabina-Cristiana Necula. 2023. "Artificial Intelligence as a Disruptive Technology—A Systematic Literature Review" Electronics 12, no. 5: 1102. https://doi.org/10.3390/electronics12051102
Article Metrics
Article access statistics, further information, mdpi initiatives, follow mdpi.
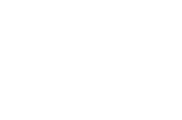
Subscribe to receive issue release notifications and newsletters from MDPI journals
Thank you for visiting nature.com. You are using a browser version with limited support for CSS. To obtain the best experience, we recommend you use a more up to date browser (or turn off compatibility mode in Internet Explorer). In the meantime, to ensure continued support, we are displaying the site without styles and JavaScript.
- View all journals
- Explore content
- About the journal
- Publish with us
- Sign up for alerts
- Published: 04 January 2023
Papers and patents are becoming less disruptive over time
- Michael Park ORCID: orcid.org/0000-0001-8373-5480 1 ,
- Erin Leahey 2 &
- Russell J. Funk ORCID: orcid.org/0000-0001-6670-4981 1
Nature volume 613 , pages 138–144 ( 2023 ) Cite this article
377k Accesses
258 Citations
4582 Altmetric
Metrics details
- Scientific community
Theories of scientific and technological change view discovery and invention as endogenous processes 1 , 2 , wherein previous accumulated knowledge enables future progress by allowing researchers to, in Newton’s words, ‘stand on the shoulders of giants’ 3 , 4 , 5 , 6 , 7 . Recent decades have witnessed exponential growth in the volume of new scientific and technological knowledge, thereby creating conditions that should be ripe for major advances 8 , 9 . Yet contrary to this view, studies suggest that progress is slowing in several major fields 10 , 11 . Here, we analyse these claims at scale across six decades, using data on 45 million papers and 3.9 million patents from six large-scale datasets, together with a new quantitative metric—the CD index 12 —that characterizes how papers and patents change networks of citations in science and technology. We find that papers and patents are increasingly less likely to break with the past in ways that push science and technology in new directions. This pattern holds universally across fields and is robust across multiple different citation- and text-based metrics 1 , 13 , 14 , 15 , 16 , 17 . Subsequently, we link this decline in disruptiveness to a narrowing in the use of previous knowledge, allowing us to reconcile the patterns we observe with the ‘shoulders of giants’ view. We find that the observed declines are unlikely to be driven by changes in the quality of published science, citation practices or field-specific factors. Overall, our results suggest that slowing rates of disruption may reflect a fundamental shift in the nature of science and technology.
Similar content being viewed by others
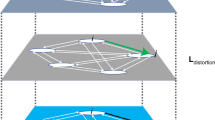
Leading countries in global science increasingly receive more citations than other countries doing similar research
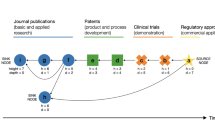
Dynamics of technology emergence in innovation networks
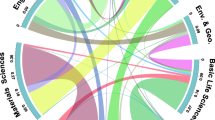
Interdisciplinarity revisited: evidence for research impact and dynamism
Although the past century witnessed an unprecedented expansion of scientific and technological knowledge, there are concerns that innovative activity is slowing 18 , 19 , 20 . Studies document declining research productivity in semiconductors, pharmaceuticals and other fields 10 , 11 . Papers, patents and even grant applications have become less novel relative to prior work and less likely to connect disparate areas of knowledge, both of which are precursors of innovation 21 , 22 . The gap between the year of discovery and the awarding of a Nobel Prize has also increased 23 , 24 , suggesting that today’s contributions do not measure up to the past. These trends have attracted increasing attention from policymakers, as they pose substantial threats to economic growth, human health and wellbeing, and national security, along with global efforts to combat grand challenges such as climate change 25 , 26 .
Numerous explanations for this slowdown have been proposed. Some point to a dearth of ‘low-hanging fruit’ as the readily available productivity-enhancing innovations have already been made 19 , 27 . Others emphasize the increasing burden of knowledge; scientists and inventors require ever more training to reach the frontiers of their fields, leaving less time to push those frontiers forward 18 , 28 . Yet much remains unknown, not merely about the causes of slowing innovative activity, but also the depth and breadth of the phenomenon. The decline is difficult to reconcile with centuries of observation by philosophers of science, who characterize the growth of knowledge as an endogenous process, wherein previous knowledge enables future discovery, a view captured famously in Newton’s observation that if he had seen further, it was by ‘standing on the shoulders of giants’ 3 . Moreover, to date, the evidence pointing to a slowdown is based on studies of particular fields, using disparate and domain-specific metrics 10 , 11 , making it difficult to know whether the changes are happening at similar rates across areas of science and technology. Little is also known about whether the patterns seen in aggregate indicators mask differences in the degree to which individual works push the frontier.
We address these gaps in understanding by analysing 25 million papers (1945–2010) in the Web of Science (WoS) ( Methods ) and 3.9 million patents (1976–2010) in the United States Patent and Trademark Office’s (USPTO) Patents View database ( Methods ). The WoS data include 390 million citations, 25 million paper titles and 13 million abstracts. The Patents View data include 35 million citations, 3.9 million patent titles and 3.9 million abstracts. Subsequently, we replicate our core findings on four additional datasets—JSTOR, the American Physical Society corpus, Microsoft Academic Graph and PubMed—encompassing 20 million papers. Using these data, we join a new citation-based measure 12 with textual analyses of titles and abstracts to understand whether papers and patents forge new directions over time and across fields.
Measurement of disruptiveness
To characterize the nature of innovation, we draw on foundational theories of scientific and technological change 2 , 29 , 30 , which distinguish between two types of breakthroughs. First, some contributions improve existing streams of knowledge, and therefore consolidate the status quo. Kohn and Sham (1965) 31 , a Nobel-winning paper used established theorems to develop a method for calculating the structure of electrons, which cemented the value of previous research. Second, some contributions disrupt existing knowledge, rendering it obsolete, and propelling science and technology in new directions. Watson and Crick (1953) 32 , also a Nobel winner, introduced a model of the structure of DNA that superseded previous approaches (for example, Pauling’s triple helix). Kohn and Sham and Watson and Crick were both important, but their implications for scientific and technological change were different.
We quantify this distinction using a measure—the CD index 12 —that characterizes the consolidating or disruptive nature of science and technology (Fig. 1 ). The intuition is that if a paper or patent is disruptive, the subsequent work that cites it is less likely to also cite its predecessors; for future researchers, the ideas that went into its production are less relevant (for example, Pauling’s triple helix). If a paper or patent is consolidating, subsequent work that cites it is also more likely to cite its predecessors; for future researchers, the knowledge upon which the work builds is still (and perhaps more) relevant (for example, the theorems Kohn and Sham used). The CD index ranges from −1 (consolidating) to 1 (disruptive). We measure the CD index five years after the year of each paper’s publication (indicated by CD 5 , see Extended Data Fig. 1 for the distribution of CD 5 among papers and patents and Extended Data Fig. 2 for analyses using alternative windows) 33 . For example, Watson and Crick and Kohn and Sham both received over a hundred citations within five years of being published. However, the Kohn and Sham paper has a CD 5 of −0.22 (indicating consolidation), whereas the Watson and Crick paper has a CD 5 of 0.62 (indicating disruption). The CD index has been validated extensively in previous research, including through correlation with expert assessments 12 , 34 .
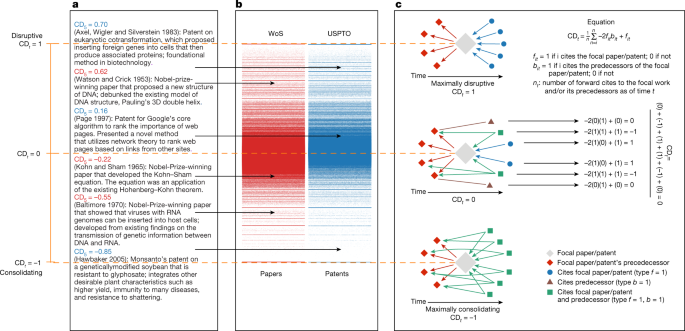
This figure shows a schematic visualization of the CD index. a , CD index value of three Nobel Prize-winning papers 31 , 32 , 58 and three notable patents 59 , 60 , 61 in our sample, measured as of five years post-publication (indicated by CD5). b , Distribution of CD 5 for papers from WoS ( n = 24,659,076) between 1945 and 2010 and patents from Patents View ( n = 3,912,353) between 1976 and 2010, where a single dot represents a paper or patent. The vertical (up–down) dimension of each ‘strip’ corresponds to values of the CD index (with axis values shown in orange on the left). The horizontal (left–right) dimension of each strip helps to minimize overlapping points. Darker areas on each strip plot indicate denser regions of the distribution (that is, more commonly observed CD 5 values). Additional details on the distribution of the CD index are given in Extended Data Fig. 1 . c , Three hypothetical citation networks, where the CD index is at the maximally disruptive value (CD t = 1), midpoint value (CD t = 0), and maximally consolidating value (CD t = −1). The panel also provides the equation for the CD index and an illustrative calculation.
Declining disruptiveness
Across fields, we find that science and technology are becoming less disruptive. Figure 2 plots the average CD 5 over time for papers (Fig. 2a ) and patents (Fig. 2b ). For papers, the decrease between 1945 and 2010 ranges from 91.9% (where the average CD 5 dropped from 0.52 in 1945 to 0.04 in 2010 for ‘social sciences’) to 100% (where the average CD 5 decreased from 0.36 in 1945 to 0 in 2010 for ‘physical sciences’); for patents, the decrease between 1980 and 2010 ranges from 78.7% (where the average CD 5 decreased from 0.30 in 1980 to 0.06 in 2010 for ‘computers and communications’) to 91.5% (where the average CD 5 decreased from 0.38 in 1980 to 0.03 in 2010 for ‘drugs and medical’). For both papers and patents, the rates of decline are greatest in the earlier parts of the time series, and for patents, they appear to begin stabilizing between the years 2000 and 2005. For papers, since about 1980, the rate of decline has been more modest in ‘life sciences and biomedicine’ and physical sciences, and most marked and persistent in social sciences and ‘technology’. Overall, however, relative to earlier eras, recent papers and patents do less to push science and technology in new directions. The general similarity in trends we observe across fields is noteworthy in light of ‘low-hanging fruit’ theories 19 , 27 , which would probably predict greater heterogeneity in the decline, as it seems unlikely fields would ‘consume’ their low-hanging fruit at similar rates or times.
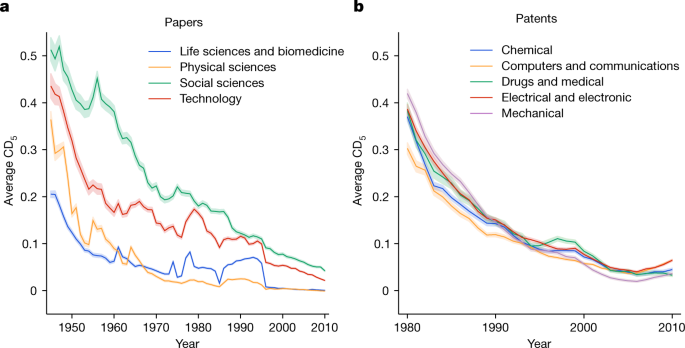
a , b , Decline in CD 5 over time, separately for papers ( a , n = 24,659,076) and patents ( b , n = 3,912,353). For papers, lines correspond to WoS research areas; from 1945 to 2010 the magnitude of decline ranges from 91.9% (social sciences) to 100% (physical sciences). For patents, lines correspond to National Bureau of Economic Research (NBER) technology categories; from 1980 to 2010 the magnitude of decline ranges from 93.5% (computers and communications) to 96.4% (drugs and medical). Shaded bands correspond to 95% confidence intervals. As we elaborate in the Methods , this pattern of decline is robust to adjustment for confounding from changes in publication, citation and authorship practices over time.
Source data
Linguistic change.
The decline in disruptive science and technology is also observable using alternative indicators. Because they create departures from the status quo, disruptive papers and patents are likely to introduce new words (for example, words used to create a new paradigm might differ from those that are used to develop an existing paradigm) 35 , 36 . Therefore, if disruptiveness is declining, we would expect a decline in the diversity of words used in science and technology. To evaluate this, Fig. 3a , d documents the type-token ratio (that is, unique/total words) of paper and patent titles over time (Supplementary Information section 1 ). We observe substantial declines, especially in the earlier periods, before 1970 for papers and 1990 for patents. For paper titles (Fig. 3a ), the decrease (1945–2010) ranges from 76.5% (social sciences) to 88% (technology); for patent titles (Fig. 3d ), the decrease (1980–2010) ranges from 32.5% (chemical) to 81% (computers and communications). For paper abstracts (Extended Data Fig. 3a ), the decrease (1992–2010) ranges from 23.1% (life sciences and biomedicine) to 38.9% (social sciences); for patent abstracts (Extended Data Fig. 3b ), the decrease (1980–2010) ranges from 21.5% (mechanical) to 73.2% (computers and communications). In Fig. 3b , e , we demonstrate that these declines in word diversity are accompanied by similar declines in combinatorial novelty; over time, the particular words that scientists and inventors use in the titles of their papers and patents are increasingly likely to have been used together in the titles of previous work. Consistent with these trends in language, we also observe declining novelty in the combinations of previous work cited by papers and patents, based on a previously established measure of ‘atypical combinations’ 14 (Extended Data Fig. 4 ).
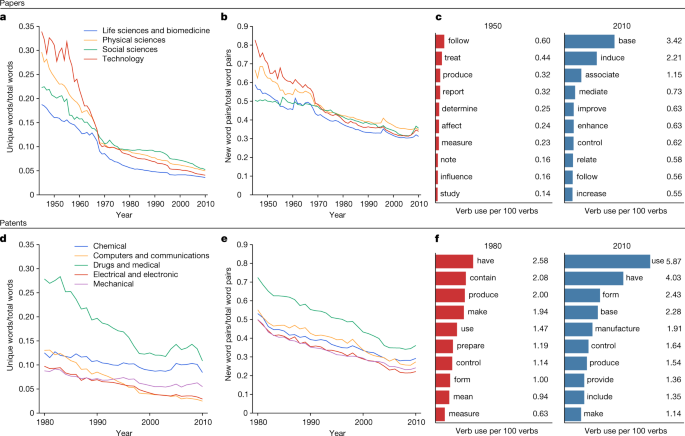
a , d , Figures showing a decline in the diversity of language used in science and technology based on the unique/total words of paper titles from 1945 to 2010 ( a , n = 24,659,076) and of patent titles from 1980 to 2010 ( d , n = 3,912,353). b , e , Figures showing a decline in the novelty of language used in science and technology based on the number of new word pairs/total word pairs introduced each year in WoS paper titles from 1945 to 2010 ( b ) and in Patents View patent titles from 1980 to 2010 (refs. 1 , 17 ) ( e ). For papers in both a and b , lines correspond to WoS research areas ( n = 264 WoS research area × year observations). For patents in both d and e , lines correspond to NBER technology categories ( n = 229 NBER technology category × year observations). c , f , Figures showing the frequency of the most commonly used verbs in paper titles for the first (red) and last (blue) decades of the observation period in paper ( c , n = 24,659,076) and patent ( f , n = 3,912,353) titles.
The decline in disruptive activity is also apparent in the specific words used by scientists and inventors. If disruptiveness is declining, we reasoned that verbs alluding to the creation, discovery or perception of new things should be used less frequently over time, whereas verbs alluding to the improvement, application or assessment of existing things may be used more often 35 , 36 . Figure 3 shows the most common verbs in paper (Fig. 3c ) and patent titles (Fig. 3f ) in the first and last decade of each sample (Supplementary Information section 2 ). Although precisely and quantitatively characterizing words as ‘consolidating’ or ‘disruptive’ is challenging in the absence of context, the figure highlights a clear and qualitative shift in language. In the earlier decades, verbs evoking creation (for example, ‘produce’, ‘form’, ‘prepare’ and ‘make’), discovery (for example, ‘determine’ and ‘report’) and perception (for example, ‘measure’) are prevalent in both paper and patent titles. In the later decades, however, these verbs are almost completely displaced by those tending to be more evocative of the improvement (for example, ‘improve’, ‘enhance’ and ‘increase’), application (for example, ‘use’ and ‘include’) or assessment (for example, ‘associate’, ‘mediate’ and ‘relate’) of existing scientific and technological knowledge and artefacts. Taken together, these patterns suggest a substantive shift in science and technology over time, with discovery and invention becoming less disruptive in nature, consistent with our results using the CD index.
Conservation of highly disruptive work
The aggregate trends we document mask considerable heterogeneity in the disruptiveness of individual papers and patents and remarkable stability in the absolute number of highly disruptive works ( Methods and Fig. 4 ). Specifically, despite large increases in scientific productivity, the number of papers and patents with CD 5 values in the far right tail of the distribution remains nearly constant over time. This ‘conservation’ of the absolute number of highly disruptive papers and patents holds despite considerable churn in the underlying fields responsible for producing those works (Extended Data Fig. 5 , inset). These results suggest that the persistence of major breakthroughs—for example, measurement of gravity waves and COVID-19 vaccines—is not inconsistent with slowing innovative activity. In short, declining aggregate disruptiveness does not preclude individual highly disruptive works.
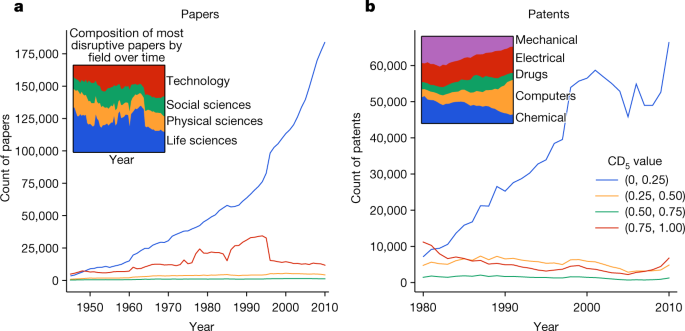
This figure shows the number of disruptive papers ( a , n = 5,030,179) and patents ( b , n = 1,476,004) across four different ranges of CD 5 (papers and patents with CD 5 values in the range [−1.0, 0) are not represented in the figure). Lines correspond to different levels of disruptiveness as measured by CD 5 . Despite substantial increases in the numbers of papers and patents published each year, there is little change in the number of highly disruptive papers and patents, as evidenced by the relatively flat red, green and orange lines. This pattern helps to account for simultaneous observations of both aggregate evidence of slowing innovative activity and seemingly major breakthroughs in many fields of science and technology. The inset plots show the composition of the most disruptive papers and patents (defined as those with CD 5 values >0.25) by field over time. The observed stability in the absolute number of highly disruptive papers and patents holds despite considerable churn in the underlying fields of science and technology responsible for producing those works. ‘Life sciences’ denotes the life sciences and biomedicine research area; ‘electrical’ denotes the electrical and electronic technology category; ‘drugs’ denotes the drugs and medical technology category; and ‘computers’ denotes the computers and communications technology category.
Alternative explanations
What is driving the decline in disruptiveness? Earlier, we suggested our results are not consistent with explanations that link slowing innovative activity to diminishing ‘low-hanging fruit’. Extended Data Fig. 5 shows that the decline in disruptiveness is unlikely to be due to other field-specific factors by decomposing variation in CD 5 attributable to field, author and year effects ( Methods ).
Declining rates of disruptive activity are unlikely to be caused by the diminishing quality of science and technology 22 , 37 . If they were, then the patterns seen in Fig. 2 should be less visible in high-quality work. However, when we restrict our sample to articles published in premier publication venues such as Nature , Proceedings of the National Academy of Sciences and Science or to Nobel-winning discoveries 38 (Fig. 5 ), the downward trend persists.
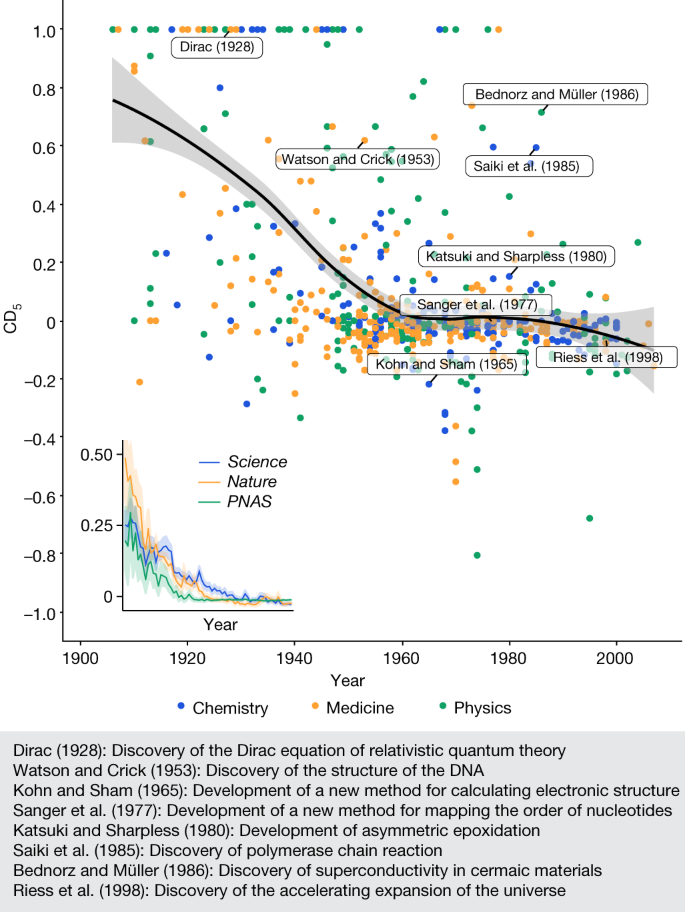
This figure shows changes in CD 5 over time for papers published in Nature , Proceedings of the National Academy of Sciences ( PNAS ) and Science (inset plot, n = 223,745) and Nobel Prize-winning papers (main plot, n = 635), with several notable examples 31 , 32 , 58 , 62 , 63 , 64 , 65 , 66 highlighted. Colours indicate the three different journals in the inset plot; colours indicate the three different fields in which the Nobel Prize is awarded in the main plot. Shaded bands correspond to 95% confidence intervals. For historical completeness, we plot CD index scores for all Nobel papers back to 1900 (the first year in which the prize was awarded); however, our main analyses begin in the post-1945 era, when the WoS data are generally more reliable. The figure indicates that changes in the quality of published science over time is unlikely to be responsible for the decline in disruption.
Furthermore, the trend is not driven by characteristics of the WoS and UPSTO data or our particular derivation of the CD index; we observe similar declines in disruptiveness when we compute CD 5 on papers in JSTOR, the American Physical Society corpus, Microsoft Academic Graph and PubMed ( Methods ), the results of which are shown in Extended Data Fig. 6 . We further show that the decline is not an artefact of the CD index by reporting similar patterns using alternative derivations 13 , 15 ( Methods and Extended Data Fig. 7 ).
Declines in disruptiveness are also not attributable to changing publication, citation or authorship practices ( Methods ). First, using approaches from the bibliometrics literature 39 , 40 , 41 , 42 , 43 , we computed several normalized versions of the CD index that adjusted for the increasing tendency for papers and patents to cite previous work 44 , 45 . Results using these alternative indicators (Extended Data Fig. 8a , d ) were similar to those we reported in Fig. 2 . Second, using regression, we estimated models of CD 5 as a function of indicator variables for each paper or patent’s publication year, along with specific controls for field × year level—number of new papers/patents, mean number of papers/patents cited, mean number of authors or inventors per paper—and paper or patent-level—number of papers or patents cited—factors. Predictions from these models indicated a decline in disruptive papers and patents (Extended Data Fig. 8b , e and Supplementary Table 1 ) that was consistent with our main results. Finally, using Monte Carlo simulations, we randomly rewired the observed citation networks while preserving key characteristics of scientists’ and inventors’ citation behaviour, including the number of citations made and received by individual papers and patents and the age gap between citing and cited works. We find that observed CD 5 values are lower than those from the simulated networks (Extended Data Fig. 8 c, f ), and the gap is widening: over time, papers and patents are increasingly less disruptive than would be expected by chance. Taken together, these additional analyses indicate that the decline in CD 5 is unlikely to be driven by changing publication, citation or authorship practices.
Growth of knowledge and disruptiveness
We also considered how declining disruptiveness relates to the growth of knowledge (Extended Data Fig. 9 ). On the one hand, scientists and inventors face an increasing knowledge burden, which may inhibit discoveries and inventions that disrupt the status quo. On the other hand, as previously noted, philosophers of science suggest that existing knowledge fosters discovery and invention 3 , 6 , 7 . Using regression models, we evaluated the relationship between the stock of papers and patents (a proxy for knowledge) within fields and their CD 5 (Supplementary Information section 3 and Supplementary Table 2 ). We find a positive effect of the growth of knowledge on disruptiveness for papers, consistent with previous work 20 ; however, we find a negative effect for patents.
Given these conflicting results, we considered the possibility that the availability of knowledge may differ from its use. In particular, the growth in publishing and patenting may lead scientists and inventors to focus on narrower slices of previous work 18 , 46 , thereby limiting the ‘effective’ stock of knowledge. Using three proxies, we document a decline in the use of previous knowledge among scientists and inventors (Fig. 6 ). First, we see a decline in the diversity of work cited (Fig. 6a,d ), indicating that contemporary science and technology are engaging with narrower slices of existing knowledge. Moreover, this decline in diversity is accompanied by an increase in the share of citations to the 1% most highly cited papers and patents (Fig. 6a (i) , d(i) ), which are also decreasing in semantic diversity (Fig. 6a (ii) , d (ii) ). Over time, scientists and inventors are increasingly citing the same previous work, and that previous work is becoming more topically similar. Second, we see an increase in self-citation (Fig. 6b,e ), a common proxy for the continuation of one’s pre-existing research stream 47 , 48 , 49 , which is consistent with scientists and inventors relying more on highly familiar knowledge. Third, the mean age of work cited, a common measure for the use of dated knowledge 50 , 51 , 52 , is increasing (Fig. 6c,f ), suggesting that scientists and inventors may be struggling to keep up with the pace of knowledge expansion and instead relying on older, familiar work. All three indicators point to a consistent story: a narrower scope of existing knowledge is informing contemporary discovery and invention.
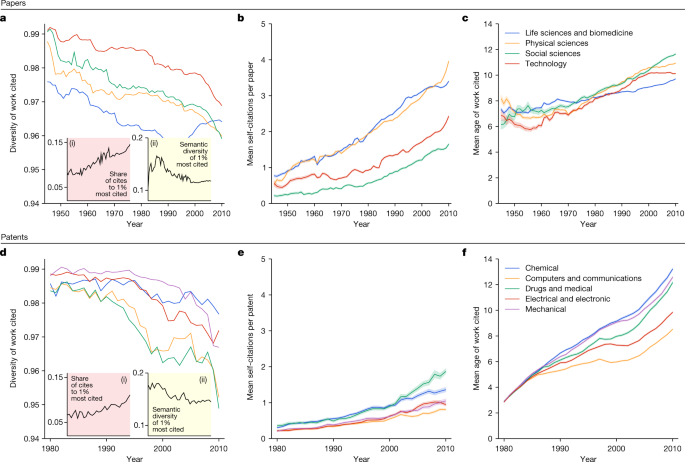
a – f , Changes in the level of diversity of existing scientific and technological knowledge use among papers ( a , n = 264 WoS research area × year observations; b and c , n = 24,659,076 papers) and patents ( d , 229 NBER technology category × year observations; e and f , n = 3,912,353 patents) based on following measures: diversity of work cited ( a and d ), mean number of self-citations ( b and e ) and mean age of cited work ( c and f ). Shaded bands ( b , c , e and f ) correspond to 95% confidence intervals. The inset plots of a and d show changes in the share of citations to the top 1% most highly cited papers ( a (i) and d (i)) and in the semantic diversity of the top 1% most cited over time ( a (ii) and d (ii)). Values of both measures are computed within field and year, and are subsequently averaged across fields for plotting. Semantic diversity is based on paper and patent titles; values correspond to the ratio of the standard deviation to the mean pairwise cosine similarity (that is, the coefficient of variation) among the titles of the 1% most cited papers and patents by field and year. To enable semantic comparisons, titles were vectorized using pretrained word embeddings. For papers, lines are shown for each WoS research area; for patents, lines are shown for each NBER technology category. In subsequent regression analyses using these measures, we find that using less diverse work, more of one’s own work and older work is associated with less disruptive papers and patents ( Methods and Extended Data Table 1 ).
Results from a subsequent series of regression models suggest that use of less diverse work, more of one’s own work and older work are all negatively associated with disruption ( Methods , Extended Data Table 1 and Supplementary Table 3 ), a pattern that holds even after accounting for the average age and number of previous works produced by team members. When the range of work used by scientists and inventors narrows, disruptive activity declines.
In summary, we report a marked decline in disruptive science and technology over time. Our analyses show that this trend is unlikely to be driven by changes in citation practices or the quality of published work. Rather, the decline represents a substantive shift in science and technology, one that reinforces concerns about slowing innovative activity. We attribute this trend in part to scientists’ and inventors’ reliance on a narrower set of existing knowledge. Even though philosophers of science may be correct that the growth of knowledge is an endogenous process—wherein accumulated understanding promotes future discovery and invention—engagement with a broad range of extant knowledge is necessary for that process to play out, a requirement that appears more difficult with time. Relying on narrower slices of knowledge benefits individual careers 53 , but not scientific progress more generally.
Moreover, even though the prevalence of disruptive works has declined, we find that the sheer number has remained stable. On the one hand, this result may suggest that there is a fixed ‘carrying capacity’ for highly disruptive science and technology, in which case, policy interventions aimed at increasing such work may prove challenging. On the other hand, our observation of considerable churn in the underlying fields responsible for producing disruptive science and technology suggests the potential importance of factors such as the shifting interests of funders and scientists and the ‘ripeness’ of scientific and technologicalknowledge for breakthroughs, in which case the production of disruptive work may be responsive to policy levers. In either case, the stability we observe in the sheer number of disruptive papers and patents suggests that science and technology do not appear to have reached the end of the ‘endless frontier’. Room remains for the regular rerouting that disruptive works contribute to scientific and technological progress.
Our study is not without limitations. Notably, even though research to date supports the validity of the CD index 12 , 34 , it is a relatively new indicator of innovative activity and will benefit from future work on its behaviour and properties, especially across data sources and contexts. Studies that systematically examine the effect of different citation practices 54 , 55 , which vary across fields, would be particularly informative.
Overall, our results deepen understanding of the evolution of knowledge and may guide career planning and science policy. To promote disruptive science and technology, scholars may be encouraged to read widely and given time to keep up with the rapidly expanding knowledge frontier. Universities may forgo the focus on quantity, and more strongly reward research quality 56 , and perhaps more fully subsidize year-long sabbaticals. Federal agencies may invest in the riskier and longer-term individual awards that support careers and not simply specific projects 57 , giving scholars the gift of time needed to step outside the fray, inoculate themselves from the publish or perish culture, and produce truly consequential work. Understanding the decline in disruptive science and technology more fully permits a much-needed rethinking of strategies for organizing the production of science and technology in the future.
We limit our focus to research papers published between 1945 and 2010. Although the WoS data begin in the year 1900, the scale and social organization of science shifted markedly in the post-war era, thereby making comparisons with the present difficult and potentially misleading 67 , 68 , 69 . We end our analyses of papers in 2010 because some of our measures require several subsequent years of data following paper publication. The WoS data archive 65 million documents published in 28,968 journals between 1900 and 2017 and 735 million citations among them. In addition, the WoS data include the titles and the full text of abstracts for 65 and 29 million records, respectively, published between 1913 and 2017. After eliminating non-research documents (for example, book reviews and commentaries) and subsetting the data to the 1945–2010 window, the analytical sample consists of n = 24,659,076 papers.
Patents View data
We limit our focus to patents granted from 1976, which is the earliest year for which machine-readable records are available in the Patents View data. As we did with papers, we end our analyses in 2010 because some measures require data from subsequent years for calculation. The Patents View data are the most exhaustive source of historical data on inventions, with information on 6.5 million patents granted between 1976 and 2017 and their corresponding 92 million citations. The Patents View data include the titles and abstracts for 6.5 million patents granted between 1976 and 2017. Following previous work 12 , we focused our attention on utility patents, which cover the vast majority (91% in our data) of patented inventions. After eliminating non-utility patents and subsetting the data to the 1976–2010 window, the analytical sample consists of n = 3,912,353 patents.
Highly disruptive papers and patents
Observations (and claims) of slowing progress in science and technology are increasingly common, supported not only by the evidence we report, but also by previous research from diverse methodological and disciplinary perspectives 10 , 11 , 18 , 19 , 20 , 21 , 22 , 23 , 24 . Yet as noted in the main text, there is a tension between observations of slowing progress from aggregate data on the one hand, and continuing reports of seemingly major breakthroughs in many fields of science and technology—spanning everything from the measurement of gravity waves to the sequencing of the human genome—on the other. In an effort to reconcile this tension, we considered the possibility that whereas overall, discovery and invention may be less disruptive over time, the high-level view taken in previous work may mask considerable heterogeneity. Put differently, aggregate evidence of slowing progress does not preclude the possibility that some subset of discoveries and inventions is highly disruptive.
To evaluate this possibility, we plot the number of disruptive papers (Fig. 4a ) and patents (Fig. 4b ) over time, where disruptive papers and patents are defined as those with CD 5 values >0. Within each panel, we plot four lines, corresponding to four evenly spaced intervals—(0, 0.25], (0.25, 0.5], (0.5, 0.75], (0.75, 1.00]—over the positive values of CD 5 . The first two intervals therefore correspond to papers and patents that are relatively weakly disruptive, whereas the latter two correspond to those that are more strongly so (for example, where we may expect to see major breakthroughs such as some of those mentioned above). Despite major increases in the numbers of papers and patents published each year, we see little change in the number of highly disruptive papers and patents, as evidenced by the relatively flat red, green and orange lines. Notably, this ‘conservation’ of disruptive work holds even despite fluctuations over time in the composition of the scientific and technological fields responsible for producing the most disruptive work (Fig. 4 , inset plots). Overall, these results help to account for simultaneous observations of both major breakthroughs in many fields of science and technology and aggregate evidence of slowing progress.
Relative contribution of field, year and author or inventor effects
Our results show a steady decline in the disruptiveness of science and technology over time. Moreover, the patterns we observe are generally similar across broad fields of study, which suggests that the factors driving the decline are not unique to specific domains of science and technology. The decline could be driven by other factors, such as the conditions of science and technology at a point in time or the particular individuals who produce science and technology. For example, exogenous factors such as economic conditions may encourage research or invention practices that are less disruptive. Similarly, scientists and inventors of different generations may have different approaches, which may result in greater or lesser tendencies for producing disruptive work. We therefore sought to understand the relative contribution of field, year and author (or inventor) factors to the decline of disruptive science and technology.
To do so, we decomposed the relative contribution of field, year and author fixed effects to the predictive power of regression models of the CD index. The unit of observation in these regressions is the author (or inventor) × year. We enter field fixed effects using granular subfield indicators (that is, 150 WoS subject areas for papers, 138 NBER subcategories for patents). For simplicity, we did not include additional covariates beyond the fixed effects in our models. Field fixed effects capture all field-specific factors that do not vary by author or year (for example, the basic subject matter); year fixed effects capture all year-specific factors that do not vary by field or author (for example, the state of communication technology); author (or inventor) fixed effects capture all author-specific factors that do not vary by field or year (for example, the year of PhD awarding). After specifying our model, we determine the relative contribution of field, year and author fixed effects to the overall model adjusted R 2 using Shapley–Owen decomposition. Specifically, given our n = 3 groups of fixed effects (field, year and author) we evaluate the relative contribution of each set of fixed effects by estimating the adjusted R 2 separately for the 2 n models using subsets of the predictors. The relative contribution of each set of fixed effects is then computed using the Shapley value from game theory 70 .
Results of this analysis are shown in Extended Data Fig. 5 , for both papers (top bar) and patents (bottom bar). Total bar size corresponds to the value of the adjusted R 2 for the fully specified model (that is, with all three groups of fixed effects). Consistent with our observations from plots of the CD index over time, we observe that for both papers and patents, field-specific factors make the lowest relative contribution to the adjusted R 2 (0.02 and 0.01 for papers and patents, respectively). Author fixed effects, by contrast, appear to contribute much more to the predictive power of the model, for both papers (0.20) and patents (0.17). Researchers and inventors who entered the field in more recent years may face a higher burden of knowledge and thus resort to building on narrower slices of existing work (for example, because of more specialized doctoral training), which would generally lead to less disruptive science and technology being produced in later years, consistent with our findings. The pattern is more complex for year fixed effects; although year-specific factors that do not vary by field or author hold more explanatory power than field for both papers (0.02) and patents (0.16), they appear to be substantially more important for the latter than the former. Taken together, these findings suggest that relatively stable factors that vary across individual scientists and inventors may be particularly important for understanding changes in disruptiveness over time. The results also confirm that domain-specific factors across fields of science and technology play a very small role in explaining the decline in disruptiveness of papers and patents.
Alternative samples
We also considered whether the patterns we document may be artefacts of our choice of data sources. Although we observe consistent trends in both the WoS and Patents View data, and both databases are widely used by the Science of Science community, our results may conceivably be driven by factors such as changes in coverage (for example, journals added or excluded from WoS over time) or even data errors rather than fundamental changes in science and technology. To evaluate this possibility, we therefore calculated CD 5 for papers in four additional databases—JSTOR, the American Physical Society corpus, Microsoft Academic Graph and PubMed. We included all records from 1930 to 2010 from PubMed (16,774,282 papers), JSTOR (1,703,353 papers) and American Physical Society (478,373 papers). The JSTOR data were obtained via a special request from ITHAKA, the data maintainer ( http://www.ithaka.org ), as were the American Physical Society data ( https://journals.aps.org/datasets ). We downloaded the Microsoft Academic Graph data from CADRE at Indiana University ( https://cadre.iu.edu/ ). The PubMed data were downloaded from the National Library of Medicine FTP server ( ftp://ftp.ncbi.nlm.nih.gov/pubmed/baseline ). Owing to the exceptionally large scale of Microsoft Academic Graph and the associated computational burden, we randomly extracted 1 million papers. As shown in Extended Data Fig. 6 , the downward trend in disruptiveness is evident across all samples.
Alternative bibliometric measures
Several recent papers have introduced alternative specifications of the CD index 12 . We evaluated whether the declines in disruptiveness we observe are corroborated using two alternative variations. One criticism of the CD index has been that the number of papers that cite only the focal paper’s references dominates the measure 13 . Bornmann et al. 13 proposes \({{\rm{DI}}}_{l}^{{\rm{nok}}}\) as a variant that is less susceptible to this issue. Another potential weakness of the CD index is that it could be very sensitive to small changes in the forward citation patterns of papers that make no backward citations 15 . Leydesdorff et al. 15 suggests DI* as an alternate indicator of disruption that addresses this issue. Therefore, we calculated \({{\rm{DI}}}_{l}^{{\rm{nok}}}\) where l = 5 and DI* for 100,000 randomly drawn papers and patents each from our analytic sample. Results are presented in Extended Data Fig. 7a (papers) and b (patents). The blue lines indicate disruption based on Bornmann et al. 13 and the orange lines indicate disruption based on Leydesdorff et al. 15 . Across science and technology, the two alternative measures both show declines in disruption over time, similar to the patterns observed with the CD index. Taken together, these results suggest that the declines in disruption we document are not an artefact of our particular operationalization.
Robustness to changes in publication, citation and authorship practices
We also considered whether our results may be attributable to changes in publication, citation or authorship practices, rather than by substantive shifts in discovery and invention. Perhaps most critically, as noted in the main text, there has been a marked expansion in publishing and patenting over the period of our study. This expansion has naturally increased the amount of previous work that is relevant to current science and technology and therefore at risk of being cited, a pattern reflected in the marked increase in the average number of citations made by papers and patents (that is, papers and patents are citing more previous work than in previous eras) 44 , 45 . Recall that the CD index quantifies the degree to which future work cites a focal work together with its predecessors (that is, the references in the bibliography of the focal work). Greater citation of a focal work independently of its predecessors is taken to be evidence of a social process of disruption. As papers and patents cite more previous work, however, the probability of a focal work being cited independently of its predecessors may decline mechanically; the more citations a focal work makes, the more likely future work is to cite it together with one of its predecessors, even by chance. Consequently, increases in the number of papers and patents available for citing and in the average number of citations made by scientists and inventors may contribute to the declining values of the CD index. In short, given the marked changes in science and technology over our long study window, the CD index of papers and patents published in earlier periods may not be directly comparable to those of more recent vintage, which could in turn render our conclusions about the decline in disruptive science and technology suspect. We addressed these concerns using three distinctive but complementary approaches—normalization, regression adjustment and simulation.
Verification using normalization
First, following common practice in bibliometric research 39 , 40 , 41 , 42 , 43 , we developed two normalized versions of the CD index, with the goal of facilitating comparisons across time. Among the various components of the CD index, we focused our attention on the count of papers or patents that only cite the focal work’s references ( N k ), as this term would seem most likely to scale with the increases in publishing and patenting and in the average number of citations made by papers and patents to previous work 13 . Larger values of N k lead to smaller values of the CD index. Consequently, marked increases in N k over time, particularly relative to other components of the measure, may lead to a downward bias, thereby inhibiting our ability to accurately compare disruptive science and technology in later years with earlier periods.
Our two normalized versions of the CD index aim to address this potential bias by attenuating the effect of increases in N k . In the first version, which we call ‘Paper normalized’, we subtract from N k the number of citations made by the focal paper or patent to previous work ( N b ). The intuition behind this adjustment is that when a focal paper or patent cites more previous work, N k is likely to be larger because there are more opportunities for future work to cite the focal paper or patent’s predecessors. This increase in N k would result in lower values of the CD index, although not necessarily as a result of the focal paper or patent being less disruptive. In the second version, which we call ‘field × year normalized’, we subtract N k by the average number of backward citations made by papers or patents in the focal paper or patent’s WoS research area or NBER technology category, respectively, during its year of publication (we label this quantity \({N}_{{\rm{b}}}^{{\rm{m}}{\rm{e}}{\rm{a}}{\rm{n}}}\) ). The intuition behind this adjustment is that in fields and time periods in which there is a greater tendency for scientists and inventors to cite previous work, N k is also likely to be larger, thereby leading to lower values of the CD index, although again not necessarily as a result of the focal paper or patent being less disruptive. In cases in which either N b or \({N}_{{\rm{b}}}^{{\rm{m}}{\rm{e}}{\rm{a}}{\rm{n}}}\) exceed the value of N k , we set N k to 0 (that is, N k is never negative in the normalized measures). Both adaptations of the CD index are inspired by established approaches in the scientometrics literature, and may be understood as a form of ‘citing side normalization’ (that is, normalization by correcting for the effect of differences in lengths of references lists) 40 .
In Extended Data Fig. 8 , we plot the average values of both normalized versions of the CD index over time, separately for papers (Extended Data Fig. 8a ) and patents (Extended Data Fig. 8d ). Consistent with our findings reported in the main text, we continue to observe a decline in the CD index over time, suggesting that the patterns we observe in disruptive science and technology are unlikely to be driven by changes in citation practices.
Verification using regression adjustment
Second, we adjusted for potential confounding using a regression-based approach. This approach complements the bibliometric normalizations just described by allowing us to account for a broader array of changes in publication, citation and authorship practices in general (the latter of which is not directly accounted for in either the normalization approach or the simulation approach described next), and increases the amount of previous work that is relevant to current science and technology in particular. In Supplementary Table 1 , we report the results of regression models predicting CD 5 for papers (Models 1–4) and patents (Models 5–8), with indicator variables included for each year of our study window (the reference categories are 1945 and 1980 for papers and patents, respectively). Models 1 and 4 are the baseline models, and include no other adjustments beyond the year indicators. In Models 2 and 5, we add subfield fixed effects (WoS subject areas for papers and NBER technology subcategories for patents). Finally, in Models 3–4 and 7–8, we add control variables for several field × year level—number of new papers orpatents, mean number of papers or patents cited, mean number of authors or inventors per paper—and paper- or patent-level—number of papers or patents cited—characteristics, thereby enabling more robust comparisons in patterns of disruptive science and technology over the long time period spanned by our study. For the paper models, we also include a paper-level control for the number of unlinked references (that is, the number of citations to works that are not indexed in WoS). We find that the inclusion of these controls improves model fit, as indicated by statistically significant Wald tests presented below the relevant models.
Across all eight models shown in Supplementary Table 1 , we find that the coefficients on the year indicators are statistically significant and negative, and growing in magnitude over time, which is consistent with the patterns we reported based on unadjusted CD 5 values index in the main text (Fig. 2 ). In Extended Data Fig. 8 , we visualize the results of our regression-based approach by plotting the predicted CD 5 values separately for each of the year indicators included in Models 4 (papers) and 8 (patents). To enable comparisons with raw CD 5 values shown in the main text, we present the separate predictions made for each year as a line graph. As shown in the figure, we continue to observe declining values of the CD index across papers and patents, even when accounting for changes in publication, citation and authorship practices.
Verification using simulation
Third, following related work in the Science of Science 14 , 71 , 72 , 73 , we considered whether our results may be an artefact of changing patterns in publishing and citation practices by using a simulation approach. In essence, the CD index measures disruption by characterizing the network of citations around a focal paper or patent. However, many complex networks, even those resulting from random processes, exhibit structures that yield non-trivial values on common network measures (for example, clustering) 74 , 75 , 76 . During the period spanned by our study, the citation networks of science and technology experienced significant change, with marked increases in both the numbers of nodes (that is, papers or patents) and edges (that is, citations). Thus, rather than reflecting a meaningful social process, the observed declines in disruption may result from these structural changes in the underlying citation networks.
To evaluate this possibility, we followed standard techniques from network science 75 , 77 and conducted an analysis in which we recomputed the CD index on randomly rewired citation networks. If the patterns we observe in the CD index are the result of structural changes in the citation networks of science and technology (for example, growth in the number of nodes or edges) rather than a meaningful social process, then these patterns should also be visible in comparable random networks that experience similar structural changes. Therefore, finding that the patterns we see in the CD index differ for the observed and random citation networks would serve as evidence that the decline in disruption is not an artefact of the data.
We began by creating copies of the underlying citation network on which the values of the CD index used in all analyses reported in the main text were based, separately for papers and patents. For each citation network (one for papers, one for patents), we then rewired citations using a degree-preserving randomization algorithm. In each iteration of the algorithm, two edges (for example, A–B and C–D) are selected from the underlying citation network, after which the algorithm attempts to swap the two endpoints of the edges (for example, A–B becomes A–D, and C–D becomes C–B). If the degree centrality of A, B, C and D remains the same after the swap, the swap is retained; otherwise, the algorithm discards the swap and moves on to the next iteration. When evaluating degree centrality, we consider ‘in-degree’ (that is, citations from other papers or patents to the focal paper or patent) and ‘out-degree’ (that is, citations from the focal paper or patent to other papers or patents) separately. Furthermore, we also required that the age distribution of citing and cited papers or patents was identical in the original and rewired networks. Specifically, swaps were only retained when the publication year of the original and candidate citations was the same. In light of these design choices, our rewiring algorithm should be seen as fairly conservative, as it preserves substantial structure from the original network. There is no scholarly consensus on the number of swaps necessary to ensure the original and rewired networks are sufficiently different from one another; the rule we adopt here is 100 × m , where m is the number of edges in the network being rewired.
Following previous work 14 , we created ten rewired copies of the observed citation networks for both papers and patents. After creating these rewired citation networks, we then recomputed CD 5 . Owing to the large scale of the WoS data, we base our analyses on a random subsample of ten million papers; CD 5 was computed on the rewired network for all patents. For each paper and patent, we then compute a z score that compares the observed CD 5 value to those of the same paper or patent in the ten rewired citation networks. Positive z scores indicate that the observed CD 5 value is greater (that is, more disruptive) than would be expected by chance; negative z scores indicate that the observed values are lesser (that is, more consolidating).
The results of these analyses are shown in Extended Data Fig. 8 , separately for papers (Extended Data Fig. 8c ) and patents (Extended Data Fig. 8f ). Lines correspond to the average z score among papers or patents published in the focal year. The plots reveal a pattern of change in the CD index over and beyond that ‘baked in’ to the changing structure of the network. We find that on average, papers and patents tend to be less disruptive than would be expected by chance, and moreover, the gap between the observed CD index values and those from the randomly rewired networks is increasing over time, which is consistent with our findings of a decline in disruptive science and technology.
Taken together, the results of the foregoing analyses suggest that although there have been marked changes in science and technology over the course of our long study window, particularly with respect to publication, citation and authorship practices, the decline in disruptive science and technology that we document using the CD index is unlikely to be an artefact of these changes, and instead represents a substantive shift in the nature of discovery and invention.
Regression analysis
We evaluate the relationship between disruptiveness and the use of previous knowledge using regression models, predicting CD 5 for individual papers and patents, based on three indicators of previous knowledge use—the diversity of work cited, mean number of self-citations and mean age of work cited. Our measure of the diversity of work cited is measured at the field × year level; all other variables included in the regressions are defined at the level of the paper or patent. To account for potential confounding factors, our models included year and field fixed effects. Year fixed effects account for time variant factors that affect all observations (papers or patents) equally (for example, global economic trends). Field fixed effects account for field-specific factors that do not change over time (for example, some fields may intrinsically value disruptive work over consolidating ones). In contrast to our descriptive plots, for our regression models, we adjust for field effects using the more granular 150 WoS ‘extended subjects’ (for example, ‘biochemistry and molecular biology’, ‘biophysics’, ‘biotechnology and applied microbiology’, ‘cell biology’, ‘developmental biology’, ‘evolutionary biology’ and ‘microbiology’ are extended subjects within the life sciences and biomedicine research area) and 38 NBER technology subcategories (for example, ‘agriculture’, ‘food’, ‘textile’; ‘coating’; ‘gas’; ‘organic’; and ‘resins’ are subcategories within the chemistry technology category).
In addition, we also include controls for the ‘mean age of team members’ (that is, ‘career age’, defined as the difference between the publication year of the focal paper or patent and the first year in which each author or inventor published a paper or patent) and the ‘mean number of previous works produced by team members’. Although increases in rates of self-citations may indicate that scientists and inventors are becoming more narrowly focused on their own work, these rates may also be driven in part by the amount of previous work available for self-citing. Similarly, although increases in the age of work cited in papers and patents may indicate that scientists and inventors are struggling to keep up, they may also be driven by the rapidly aging workforce in science and technology 78 , 79 . For example, older scientists and inventors may be more familiar with or more attentive to older work, or may actively resist change 80 . These control variables help to account for these alternative explanations.
Supplementary Table 3 shows summary statistics for variables used in the ordinary-least-squares regression models. The diversity of work cited is measured by normalized entropy, which ranges from 0 to 1. Greater values on this measure indicate a more uniform distribution of citations to a wider range of existing work; lower values indicate a more concentrated distribution of citations to a smaller range of existing work. The tables show that the normalized entropy in a given field and year has a nearly maximal average entropy of 0.98 for both science and technology. About 16% of papers cited in a paper are by an author of the focal paper; the corresponding number for patents is about 7%. Papers tend to rely on older work and work that varies more greatly in age (measured by standard deviation) than patents. In addition, the average CD 5 of a paper is 0.04 whereas the average CD 5 of a patent is 0.12, meaning that the average paper tends to be less disruptive than the average patent.
We find that using more diverse work, less of one’s own work and older work tends to be associated with the production of more disruptive science and technology, even after accounting for the average age and number of previous works produced by team members. These findings are based on our regression results, shown in Extended Data Table 1 . Models 6 and 12 present the full regression models. The models indicate a consistent pattern for both science and technology, wherein the coefficients for diversity of work cited are positive and significant for papers (0.159, P < 0.01) and patents (0.069, P < 0.01), indicating that in fields in which there is more use of diverse work, there is greater disruption. Holding all other variables at their means, the predicted CD 5 of papers and patents increases by 303.5% and 1.3%, respectively, when the diversity of work cited increases by 1 s.d. The coefficients of the ratio of self-citations to total work cited is negative and significant for papers (−0.011, P < 0.01) and patents (−0.060, P < 0.01), showing that when researchers or inventors rely more on their own work, discovery and invention tends to be less disruptive. Again holding all other variables at their means, the predicted CD 5 of papers and patents decreases by 622.9% and 18.5%, respectively, with a 1 s.d. increase in the ratio. The coefficients of the interaction between mean age of work cited and dispersion in age of work cited is positive and significant for papers (0.000, P < 0.01) and patents (0.001, P < 0.01), suggesting that—holding the dispersion of the age of work cited constant—papers and patents that engage with older work are more likely to be disruptive. The predicted CD 5 of papers and patents increases by a striking 2,072.4% and 58.4%, respectively, when the mean age of work cited increases by 1 s.d. (about nine and eight years for papers and patents, respectively), again holding all other variables at their means. In summary, the regression results suggest that changes in the use of previous knowledge may contribute to the production of less disruptive science and technology.
Reporting summary
Further information on research design is available in the Nature Portfolio Reporting Summary linked to this article.
Data availability
Data associated with this study are freely available in a public repository at https://doi.org/10.5281/zenodo.7258379 . Our study draws on data from six sources: the American Physical Society, JSTOR, Microsoft Academic Graph, Patents View, PubMed and WoS. Data from Microsoft Academic Graph, Patents View and PubMed are publicly available, and our repository includes complete data for analyses from these sources. Data from the American Physical Society, JSTOR and WoS are not publicly available, and were used under licence from their respective publishers. To facilitate replication, our repository includes limited versions of the data from these sources, which will enable calculation of basic descriptive statistics. The authors will make full versions of these data available upon request and with permission from their respective publishers. Source data are provided with this paper.
Code availability
Open-source code related to this study is available at https://doi.org/10.5281/zenodo.7258379 and http://www.cdindex.info . We used Python v.3.10.6 (pandas v.1.4.3, numpy v.1.23.1, matplotlib v.3.5.2, seaborn v.0.11.2, spacy v.2.2, jupyterlab v.3.4.4) to wrangle, analyse and visualize data and to conduct statistical analyses. We used MariaDB v.10.6.4 to wrangle data. We used R v.4.2.1 (ggplot2 v.3.36, ggrepel v.0.9.0) to visualize data. We used StataMP v.17.0 (reghdfe v.5.7.3) to conduct statistical analyses.
Fleming, L. Recombinant uncertainty in technological search. Manage. Sci. 47 , 117–132 (2001).
Article Google Scholar
Schumpeter, J. Capitalism, Socialism and Democracy (Perennial, 1942).
Koyré, A. An unpublished letter of Robert Hooke to Isaac Newton. ISIS 43 , 312–337 (1952).
Article MathSciNet MATH Google Scholar
Popper, K. Conjectures and Refutations: The Growth of Scientific Knowledge (Routledge, 2014).
Fleck, L. Genesis and Development of a Scientific Fact (Univ. Chicago Press, 2012).
Acemoglu, D., Akcigit, U. & Kerr, W. R. Innovation network. Proc. Natl Acad. Sci. USA 113 , 11483–11488 (2016).
Article ADS CAS Google Scholar
Weitzman, M. L. Recombinant growth. Q. J. Econ. 113 , 331–360 (1998).
Tria, F., Loreto, V., Servedio, V. D. P. & Strogatz, S. H. The dynamics of correlated novelties. Sci. Rep. 4 , 1–8 (2014).
Fink, T. M. A., Reeves, M., Palma, R. & Farr, R. S. Serendipity and strategy in rapid innovation. Nat. Commun. 8 , 1–9 (2017).
Article CAS Google Scholar
Pammolli, F., Magazzini, L. & Riccaboni, M. The productivity crisis in pharmaceutical R&D. Nat. Rev. Drug Discov. 10 , 428–438 (2011).
Bloom, N., Jones, C. I., Van Reenen, J. & Webb, M. Are ideas getting harder to find? Am. Econ. Rev. 110 , 1104–1144 (2020).
Funk, R. J. & Owen-Smith, J. A dynamic network measure of technological change. Manage. Sci. 63 , 791–817 (2017).
Bornmann, L., Devarakonda, S., Tekles, A. & Chacko, G. Are disruption index indicators convergently valid? The comparison of several indicator variants with assessments by peers. Quant. Sci. Stud. 1 , 1242–1259 (2020).
Uzzi, B., Mukherjee, S., Stringer, M. & Jones, B. Atypical combinations and scientific impact. Science 342 , 468–472 (2013).
Leydesdorff, L., Tekles, A. & Bornmann, L. A proposal to revise the disruption index. Prof. Inf. 30 , e300121 (2021).
Lu, C. et al. Analyzing linguistic complexity and scientific impact. J. Informetr. 13 , 817–829 (2019).
Hofstra, B. et al. The diversity–innovation paradox in science. Proc. Natl Acad. Sci. USA 117 , 9284–9291 (2020).
Jones, B. F. The burden of knowledge and the ‘death of the renaissance man’: is innovation getting harder? Rev. Econ. Stud. 76 , 283–317 (2009).
Article MATH Google Scholar
Gordon, R. J. The Rise and Fall of American Growth (Princeton Univ. Press, 2016).
Chu, J. S. G. & Evans, J. A. Slowed canonical progress in large fields of science. Proc. Natl Acad. Sci. USA 118 , e2021636118 (2021).
Packalen, M. & Bhattacharya, J. NIH funding and the pursuit of edge science. Proc. Natl Acad. Sci. USA 117 , 12011–12016 (2020).
Jaffe, A. B. & Lerner, J. Innovation and its Discontents: How Our Broken Patent System Is Endangering Innovation and Progress, and What To Do About It (Princeton Univ. Press, 2011).
Horgan, J. The End of Science: Facing the Limits of Knowledge in the Twilight of the Scientific Age (Basic Books, 2015).
Collison, P. & Nielsen, M. Science Is Getting Less Bang for its Buck (Atlantic, 2018).
Nolan, A. Artificial intelligence and the future of science. oecd.ai , https://oecd.ai/en/wonk/ai-future-of-science (25 October 2021).
Effective Policies to Foster High-risk/High-reward Research. OECD Science, Technology, and Industry Policy Papers (OECD, 2021).
Cowen, T. The Great Stagnation: How America Ate All the Low-Hanging Fruit of Modern History, Got Sick, and Will (Eventually) Feel Better (Penguin, 2011).
Einstein, A. The World As I See It (Citadel Press, 1949).
Arthur, W. B. The structure of invention. Res. Policy 36 , 274–287 (2007).
Tushman, M. L. & Anderson, P. Technological discontinuities and organizational environments. Adm. Sci. Q. 31 , 439–465 (1986).
Kohn, W. & Sham, L. J. Self-consistent equations including exchange and correlation effects. Phys. Rev. 140 , A1133 (1965).
Article ADS MathSciNet Google Scholar
Watson, J. D. & Crick, F. H. C. Molecular structure of nucleic acids: a structure for deoxyribose nucleic acid. Nature 171 , 737–738 (1953).
Bornmann, L. & Tekles, A. Disruption index depends on length of citation window. Prof. Inf. 28 , e280207 (2019).
Wu, L., Wang, D. & Evans, J. A. Large teams develop and small teams disrupt science and technology. Nature 566 , 378–382 (2019).
Kuhn, T. S. The Structure of Scientific Revolutions (Univ. Chicago Press, 1962).
Brad Wray, K. Kuhn and the discovery of paradigms. Philos. Soc. Sci. 41 , 380–397 (2011).
Ioannidis, J. P. A. Why most published research findings are false. PLoS Med. 2 , e124 (2005).
Li, J., Yin, Y., Fortunato, S. & Wang, D. A dataset of publication records for Nobel laureates. Sci. Data 6 , 1–10 (2019).
Bornmann, L. & Marx, W. Methods for the generation of normalized citation impact scores in bibliometrics: which method best reflects the judgements of experts? J. Informetr. 9 , 408–418 (2015).
Waltman, L. A review of the literature on citation impact indicators. J. Informetr. 10 , 365–391 (2016).
Waltman, L. & van Eck, N. J. in Springer Handbook of Science and Technology Indicators (eds. Glänzel, W. et al.) 281–300 (Springer, 2019).
Bornmann, L. How can citation impact in bibliometrics be normalized? A new approach combining citing-side normalization and citation percentiles. Quant. Sci. Stud. 1 , 1553–1569 (2020).
Petersen, A. M., Pan, R. K., Pammolli, F. & Fortunato, S. Methods to account for citation inflation in research evaluation. Res. Policy 48 , 1855–1865 (2019).
Bornmann, L. & Mutz, R. Growth rates of modern science: a bibliometric analysis based on the number of publications and cited references. J. Assoc. Inf. Sci. Technol. 66 , 2215–2222 (2015).
Bornmann, L., Haunschild, R. & Mutz, R. Growth rates of modern science: a latent piecewise growth curve approach to model publication numbers from established and new literature databases. Humanit. Soc. Sci. Commun. 8 , 1–15 (2021).
Jones, B. F. & Weinberg, B. A. Age dynamics in scientific creativity. Proc. Natl Acad. Sci. USA 108 , 18910–18914 (2011).
Bonzi, S. & Snyder, H. Motivations for citation: a comparison of self citation and citation to others. Scientometrics 21 , 245–254 (1991).
Fowler, J. & Aksnes, D. Does self-citation pay? Scientometrics 72 , 427–437 (2007).
King, M. M., Bergstrom, C. T., Correll, S. J., Jacquet, J. & West, J. D. Men set their own cites high: gender and self-citation across fields and over time. Socius 3 , 2378023117738903 (2017).
Mukherjee, S., Romero, D. M., Jones, B. & Uzzi, B. The nearly universal link between the age of past knowledge and tomorrow’s breakthroughs in science and technology: the hotspot. Sci. Adv. 3 , e1601315 (2017).
Article ADS Google Scholar
Merton, R. K. Singletons and multiples in scientific discovery: a chapter in the sociology of science. Proc. Am. Philos. Soc. 105 , 470–486 (1961).
Google Scholar
Wang, D., Song, C. & Barabási, A.-L. Quantifying long-term scientific impact. Science 342 , 127–132 (2013).
Leahey, E. Not by productivity alone: how visibility and specialization contribute to academic earnings. Am. Sociol. Rev. 72 , 533–561 (2007).
Tahamtan, I. & Bornmann, L. Core elements in the process of citing publications: conceptual overview of the literature. J. Informetr. 12 , 203–216 (2018).
Tahamtan, I. & Bornmann, L. What do citation counts measure? An updated review of studies on citations in scientific documents published between 2006 and 2018. Scientometrics 121 , 1635–1684 (2019).
Bhattacharya, J. & Packalen, M. Stagnation and Scientific Incentives (Working Paper 26752) , https://www.nber.org/papers/w26752 (2020).
Azoulay, P., Graff Zivin, J. S. & Manso, G. Incentives and creativity: evidence from the academic life sciences. RAND J. Econ. 42 , 527–554 (2011).
Baltimore, D. Viral RNA-dependent DNA polymerase: RNA-dependent DNA polymerase in virions of RNA tumour viruses. Nature 226 , 1209–1211 (1970).
Page, L. Method for node ranking in a linked database. US patent 6,285,999 (2001).
Axel, R., Wigler, M. H. & Silverstein, S. J. Processes for inserting DNA into eucaryotic cells and for producing proteinaceous materials. US patent 4,634,665 (1983).
Hawbaker, M. S. Soybean variety SE90346. US patent 6,958,436 (2005).
Katsuki, T. & Sharpless, K. B. The first practical method for asymmetric epoxidation. J. Am. Chem. Soc. 102 , 5974–5976 (1980).
Riess, A. G., et al. Observational evidence from supernovae for an accelerating universe and a cosmological constant. Astron. J. 116 , 1009 (1998).
Dirac, P. A. M. The quantum theory of the electron. Proc. R. Soc. Lond. A Math. Phys. Sci. 117 , 610–624 (1928).
ADS MATH Google Scholar
Sanger, F., Nicklen, S. & Coulson, A. R. DNA sequencing with chain-terminating inhibitors. Proc. Natl Acad. Sci. USA 74 , 5463–5467 (1977).
Bednorz, J. G. & Müller, K. A. Possible high T c superconductivity in the Ba-La-Cu-O system. Z. Phys. B Condens. Matter 64 , 189–193 (1986).
Wuchty, S., Jones, B. F. & Uzzi, B. The increasing dominance of teams in production of knowledge. Science 316 , 1036–1039 (2007).
Guimera, R., Uzzi, B., Spiro, J. & Amaral, L. A. N. Team assembly mechanisms determine collaboration network structure and team performance. Science 308 , 697–702 (2005).
Jones, B. F., Wuchty, S. & Uzzi, B. Multi-university research teams: shifting impact, geography, and stratification in science. Science 322 , 1259–1262 (2008).
Grömping, U. Estimators of relative importance in linear regression based on variance decomposition. Am. Stat. 61 , 139–147 (2007).
Article MathSciNet Google Scholar
Mukherjee, S., Uzzi, B., Jones, B. & Stringer, M. A new method for identifying recombinations of existing knowledge associated with high-impact innovation. J. Prod. Innov. Manage. 33 , 224–236 (2016).
Christianson, N. H., Sizemore Blevins, A. & Bassett, D. S. Architecture and evolution of semantic networks in mathematics texts. Proc. R. Soc. A 476 , 20190741 (2020).
Article ADS MathSciNet MATH Google Scholar
Newman, M. E. J. The structure of scientific collaboration networks. Proc. Natl Acad. Sci. USA 98 , 404–409 (2001).
Article ADS MathSciNet CAS MATH Google Scholar
Newman, M. E. J. Scientific collaboration networks. I. Network construction and fundamental results. Phys. Rev. E 64 , 016131 (2001).
Uzzi, B. & Spiro, J. Collaboration and creativity: the small world problem. Am. J. Sociol. 111 , 447–504 (2005).
Funk, R. J. Making the most of where you are: geography, networks, and innovation in organizations. Acad. Manage. J. 57 , 193–222 (2014).
Barabási, A.-L. Network Science (Cambridge Univ. Press, 2016).
Blau, D. M. & Weinberg, B. A. Why the US science and engineering workforce is aging rapidly. Proc. Natl Acad. Sci. USA 114 , 3879–3884 (2017).
Cui, H., Wu, L. & Evans, J. A. Aging scientists and slowed advance. Preprint at https://doi.org/10.48550/arXiv.2202.04044 (2022).
Azoulay, P., Fons-Rosen, C. & Graff Zivin, J. S. Does science advance one funeral at a time? Am. Econ. Rev. 109 , 2889–2920 (2019).
Download references
Acknowledgements
This study was supported by the National Science Foundation (grant Nos. 1829168, 1932596 and 1829302).
Author information
Authors and affiliations.
Carlson School of Management, University of Minnesota, Minneapolis, MN, USA
Michael Park & Russell J. Funk
School of Sociology, University of Arizona, Tucson, AZ, USA
Erin Leahey
You can also search for this author in PubMed Google Scholar
Contributions
R.J.F. and E.L. collaboratively contributed to the conception and design of the study. R.J.F. and M.P. collaboratively contributed to the acquisition, analysis and interpretation of the data. R.J.F. created software used in the study. R.J.F., E.L. and M.P. collaboratively drafted and revised the manuscript.
Corresponding author
Correspondence to Russell J. Funk .
Ethics declarations
Competing interests.
The authors declare no competing interests.
Peer review
Peer review information.
Nature thanks Diana Hicks and the other, anonymous, reviewer(s) for their contribution to the peer review of this work. Peer reviewer reports are available.
Additional information
Publisher’s note Springer Nature remains neutral with regard to jurisdictional claims in published maps and institutional affiliations.
Extended data figures and tables
Extended data fig. 1 distribution of cd 5 ..
This figure gives an overview of the distribution of CD 5 for papers (n = 24,659,076) and patents (n = 3,912,353). Panels a and c show counts of papers and patents over discrete intervals of CD 5 . Panels b and d show the distribution of CD 5 over time, within 10 (papers) and 5 (patents) year intervals, using letter-value plots. These plots are similar to boxplots, but generally provide more reliable summaries for large datasets. They are drawn by identifying the median of the underlying distribution and then recursively drawing boxes outward from there in either direction that encompass half of the remaining data.
Extended Data Fig. 2 CD index measured using alternative forward citation windows.
This figure evaluates the sensitivity of our results to the use of different forward citation windows when computing the CD index for papers (n = 24,659,076) and patents (n = 3,912,353). In the main text, the index is computed based on citations made to papers and patents and their backward references as of 5 years after the year of publication. a and c plot the CD index using a longer, 10 year forward window, for papers and patents, respectively. b and d plot the CD index using all forward citations made to sample papers and patents as of the year 2017. Shaded bands correspond to 95% confidence intervals. Overall, the results mirror those reported in the main text, although the decline is somewhat steeper using longer forward citation windows, suggesting our primary results may represent a more conservative estimate.
Extended Data Fig. 3 Diversity of language use in science and technology over time.
This figure shows changes in the ratio of unique to total words (also known as the type-token ratio) over time based on data from the abstracts of papers ( a , n = 76 WoS research area × year observations) and patents ( b , n = 229 NBER technology category × year observations). For papers, lines correspond to WoS research areas; for patents, lines correspond to NBER technology categories. For paper abstracts, lines begin in 1992 because WoS does not reliably record abstracts for papers published prior to the early 1990s. The ratio of unique to total words is computed separately by field (i.e., the uniqueness of words and total word counts are determined within WoS research areas and NBER technology categories). If disruption is decreasing, we may plausibly expect to see a decrease in the diversity of words used by scientists and inventors, as discoveries and inventions will be less likely to create departures from the status quo, and will therefore be less likely to need to introduce new terminology. For both papers and patents, we observe declining diversity in word use over time, which is consistent with this expectation and corroborates our findings using the CD index.
Extended Data Fig. 4 Declining combinatorial novelty.
This figure shows changing patterns in the combinatorial novelty/conventionality of papers ( a , n = 24,659,076) and patents ( b , n = 3,912,353), using a previously proposed measure of “atypical combinations” 14 . The measure quantifies the degree to which the prior work cited by a paper or patent would be expected by chance. For papers, we follow prior work 14 and consider combinations of cited journals. If a paper made three citations to prior work, and that work was published in three different journals— Nature , Cell , and Science —then there are three combinations— Nature × Cell , Nature × Science , and Science × Cell . To determine the degree to which each combination would be expected by chance, the frequency of observed pairings is compared to those in 10 “rewired” copies of the overall citation network, using a z-score. For patents, there is no natural analogue to journals, and therefore we consider pairings of primary United States Patent Classification (USPC) system codes. We present the results of this analysis following the approach of prior work 14 , which plots the cumulative distribution function of the measure. In general, there is a rightward shift in the cumulative distributions over time, suggesting that for both papers and patents, combinations are more conventional than would be expected by chance, consistent with what we would anticipate based on our results using the CD index. For patents, there is also a smaller shift in the opposite direction on the left side of the distribution, suggesting that novel patents in recent decades are somewhat more novel than novel patents in earlier decades. Overall, however, the bulk of the distribution is moving rightward, indicating greater conventionality.
Extended Data Fig. 5 Contribution of field, year, and author effects.
This figure shows the relative contribution of field, year, and author fixed effects to the adjusted R 2 in regression models predicting CD 5 . The top bar shows the results for papers (n = 80,607,091 paper × author observations); the bottom bar shows the results for patents (n = 8,319,826 patent × inventor observations). The results suggest that for both papers and patents, stable characteristics of authors contribute significantly to patterns of disruptiveness. Moreover, relatively little of the variation is accounted for by field-specific factors.
Extended Data Fig. 6 CD index over time across data sources.
This figure shows changes in CD 5 over time across four additional data sources (the WoS [n = 24,659,076] and Patents View [n = 3,912,353] lines are included for reference): JSTOR (n = 1,703,353), the American Physical Society corpus (n = 478,373), Microsoft Academic Graph (n = 1,000,000), and PubMed (n = 16,774,282). Colours indicate the six different data sources. Shaded bands correspond to 95% confidence intervals. The figure indicates that the decline in disruption is unlikely to be driven by our sample choice of WoS papers and Patents View patents.
Extended Data Fig. 7 Alternative measures of disruption.
This figure shows the decline in the disruption of papers ( a , n = 100,000) and patents ( b , n = 100,000) based on two alternative measures of disruption. The blue lines calculate disruption using a measure proposed in Bornmann et al. 13 , \({{DI}}_{l}^{{nok}}\) where l = 5, which makes the measure more resilient to marginal changes in the number of papers or patents that only cite the focal work’s references. The orange lines calculate disruption using a measure proposed in Leydesdorff et al. 15 , DI*, which makes the measure less sensitive to small changes in the forward citation patterns of papers or patents that make no backward citations. Shaded bands correspond to 95% confidence intervals. With both alternative measures, we observe decreases in disruption for papers and patents, suggesting that the decline is not an artefact of our operationalization of disruption.
Extended Data Fig. 8 Robustness to changes in publication, citation, and authorship practices.
This figure evaluates whether declines in disruptiveness may be attributable to changes in publication, citation, and authorship practices for papers (n = 24,659,076) and patents (n = 3,912,353). Panels a and d adjust for these changes using a normalization approach. We present two alternative versions of the CD index, both of which account for the tendency for papers and patents to cite more prior work over time. Blue lines indicate normalization at the paper level (accounting for the number of citations made by the focal paper/patent). Orange lines indicate normalization at the field and year level (accounting for the mean number of citations made by papers/patents in the focal field and year). Panels b (papers) and e (patents) adjust for changes in publication, citation, and authorship practices using a regression approach. The panels show predicted values of CD 5 based on regressions reported in Models 4 (papers) and 8 (patents) of Supplementary Table 1 , which adjust for field × year— Number of new papers/patents , Mean number of papers/patents cited , Mean number of authors/inventors per paper/patent —and paper/patent-level— Number of papers/patents cited , Number of unlinked references —characteristics. Predictions are made separately for each year indicator included in the models; we then connect these separate predictions with lines to aid interpretation. Finally, Panels c (papers) and f (patents) adjust for changes in publication, citation, and authorship practices using a simulation approach. The panels plot z-scores that compare values of CD 5 obtained from the observed citation networks to those obtained from randomly rewired copies of the observed networks. Across all six panels, shaded bands correspond to 95% confidence intervals.
Extended Data Fig. 9 Growth of scientific and technological knowledge.
This figure shows the number of papers (n = 24,659,076) published ( a ) and patents (n = 3,912,353) granted ( b ) over time. For papers, lines correspond to WoS research areas; for patents, lines correspond to NBER technology categories.
Supplementary information
Supplementary information.
Supplementary Sections 1–3, Tables 1–3 and References.
Peer Review File
Source data fig. 2., source data fig. 3., source data fig. 4., source data fig. 5., source data fig. 6., source data extended data fig. 1., source data extended data fig. 2., source data extended data fig. 3., source data extended data fig. 5., source data extended data fig. 6., source data extended data fig. 7., source data extended data fig. 8., source data extended data fig. 9., rights and permissions.
Springer Nature or its licensor (e.g. a society or other partner) holds exclusive rights to this article under a publishing agreement with the author(s) or other rightsholder(s); author self-archiving of the accepted manuscript version of this article is solely governed by the terms of such publishing agreement and applicable law.
Reprints and permissions
About this article
Cite this article.
Park, M., Leahey, E. & Funk, R.J. Papers and patents are becoming less disruptive over time. Nature 613 , 138–144 (2023). https://doi.org/10.1038/s41586-022-05543-x
Download citation
Received : 14 February 2022
Accepted : 08 November 2022
Published : 04 January 2023
Issue Date : 05 January 2023
DOI : https://doi.org/10.1038/s41586-022-05543-x
Share this article
Anyone you share the following link with will be able to read this content:
Sorry, a shareable link is not currently available for this article.
Provided by the Springer Nature SharedIt content-sharing initiative
This article is cited by
Recsoi: recommending research directions using statements of ignorance.
- Adrien Bibal
- Nourah M. Salem
- Lawrence E. Hunter
Journal of Biomedical Semantics (2024)
Mapping the scientific output of organoids for animal and human modeling infectious diseases: a bibliometric assessment
- Jean Monlong
- Agnès Wiedemann
Veterinary Research (2024)
Redefining cancer research for therapeutic breakthroughs
- Arseniy E. Yuzhalin
British Journal of Cancer (2024)
In search of innovative potential
- Lutz Bornmann
- Christoph Ettl
- Christian Leibel
EMBO Reports (2024)
BrainSwarming, blockchain, and bioethics: applying Innovation Enhancing Techniques to healthcare and research
- Anuraag A. Vazirani
- Tony McCaffrey
- Sebastian Porsdam Mann
Scientific Reports (2024)
By submitting a comment you agree to abide by our Terms and Community Guidelines . If you find something abusive or that does not comply with our terms or guidelines please flag it as inappropriate.
Quick links
- Explore articles by subject
- Guide to authors
- Editorial policies
Sign up for the Nature Briefing newsletter — what matters in science, free to your inbox daily.

This week: the arXiv Accessibility Forum
Help | Advanced Search
Computer Science > Software Engineering
Title: a disruptive research playbook for studying disruptive innovations.
Abstract: As researchers, we are now witnessing a fundamental change in our technologically-enabled world due to the advent and diffusion of highly disruptive technologies such as generative AI, Augmented Reality (AR) and Virtual Reality (VR). In particular, software engineering has been profoundly affected by the transformative power of disruptive innovations for decades, with a significant impact of technical advancements on social dynamics due to its the socio-technical nature. In this paper, we reflect on the importance of formulating and addressing research in software engineering through a socio-technical lens, thus ensuring a holistic understanding of the complex phenomena in this field. We propose a research playbook with the goal of providing a guide to formulate compelling and socially relevant research questions and to identify the appropriate research strategies for empirical investigations, with an eye on the long-term implications of technologies or their use. We showcase how to apply the research playbook. Firstly, we show how it can be used retrospectively to reflect on a prior disruptive technology, Stack Overflow, and its impact on software development. Secondly, we show it can be used to question the impact of two current disruptive technologies: AI and AR/VR. Finally, we introduce a specialized GPT model to support the researcher in framing future investigations. We conclude by discussing the broader implications of adopting the playbook for both researchers and practitioners in software engineering and beyond.
Subjects: | Software Engineering (cs.SE) |
Cite as: | [cs.SE] |
(or [cs.SE] for this version) | |
Focus to learn more arXiv-issued DOI via DataCite |
Submission history
Access paper:.
- HTML (experimental)
- Other Formats
References & Citations
- Google Scholar
- Semantic Scholar
BibTeX formatted citation


Bibliographic and Citation Tools
Code, data and media associated with this article, recommenders and search tools.
- Institution
arXivLabs: experimental projects with community collaborators
arXivLabs is a framework that allows collaborators to develop and share new arXiv features directly on our website.
Both individuals and organizations that work with arXivLabs have embraced and accepted our values of openness, community, excellence, and user data privacy. arXiv is committed to these values and only works with partners that adhere to them.
Have an idea for a project that will add value for arXiv's community? Learn more about arXivLabs .
Disruptive Technologies - Science topic

- Recruit researchers
- Join for free
- Login Email Tip: Most researchers use their institutional email address as their ResearchGate login Password Forgot password? Keep me logged in Log in or Continue with Google Welcome back! Please log in. Email · Hint Tip: Most researchers use their institutional email address as their ResearchGate login Password Forgot password? Keep me logged in Log in or Continue with Google No account? Sign up

- SUGGESTED TOPICS
- The Magazine
- Newsletters
- Managing Yourself
- Managing Teams
- Work-life Balance
- The Big Idea
- Data & Visuals
- Reading Lists
- Case Selections
- HBR Learning
- Topic Feeds
- Account Settings
- Email Preferences
What Is Disruptive Innovation?
- Clayton M. Christensen,
- Michael E. Raynor,
- Rory McDonald

For the past 20 years, the theory of disruptive innovation has been enormously influential in business circles and a powerful tool for predicting which industry entrants will succeed. Unfortunately, the theory has also been widely misunderstood, and the “disruptive” label has been applied too carelessly anytime a market newcomer shakes up well-established incumbents.
In this article, the architect of disruption theory, Clayton M. Christensen, and his coauthors correct some of the misinformation, describe how the thinking on the subject has evolved, and discuss the utility of the theory.
They start by clarifying what classic disruption entails—a small enterprise targeting overlooked customers with a novel but modest offering and gradually moving upmarket to challenge the industry leaders. They point out that Uber, commonly hailed as a disrupter, doesn’t actually fit the mold, and they explain that if managers don’t understand the nuances of disruption theory or apply its tenets correctly, they may not make the right strategic choices. Common mistakes, the authors say, include failing to view disruption as a gradual process (which may lead incumbents to ignore significant threats) and blindly accepting the “Disrupt or be disrupted” mantra (which may lead incumbents to jeopardize their core business as they try to defend against disruptive competitors).
The authors acknowledge that disruption theory has certain limitations. But they are confident that as research continues, the theory’s explanatory and predictive powers will only improve.
Twenty years after the introduction of the theory, we revisit what it does—and doesn’t—explain.
Please enjoy this HBR Classic.
- Clayton M. Christensen was the Kim B. Clark Professor of Business Administration at Harvard Business School and a frequent contributor to Harvard Business Review.
- Michael E. Raynor is a director at Deloitte Consulting LLP. He is the coauthor, with Mumtaz Ahmed, of The Three Rules: How Exceptional Companies Think (New York: Penguin Books, 2013).
- Rory McDonald is the Thai-Hi T. Lee (MBA 1985) Associate Professor of Business Administration in the Technology and Operations Management unit at Harvard Business School.

Partner Center
Click through the PLOS taxonomy to find articles in your field.
For more information about PLOS Subject Areas, click here .
Loading metrics
Open Access
Peer-reviewed
Research Article
Research on potential disruptive technology identification based on technology network
Roles Conceptualization, Data curation, Formal analysis, Funding acquisition, Investigation, Methodology, Project administration, Resources, Software, Supervision, Validation, Visualization, Writing – original draft, Writing – review & editing
Affiliation Intellectual Property Information Services Center, Jingdezhen Ceramic University, Jingdezhen, China

Roles Funding acquisition, Methodology, Resources, Software, Supervision, Validation
* E-mail: [email protected]
Affiliations Intellectual Property Information Services Center, Jingdezhen Ceramic University, Jingdezhen, China, School of Information Engineering, Jingdezhen Ceramic University, Jingdezhen, China
Roles Resources, Software, Validation
Roles Resources, Software, Supervision, Validation
Roles Resources, Software, Supervision, Visualization
- Mingli Ding,
- Wangke Yu,
- Ran Li,
- Zhenzhen Wang,
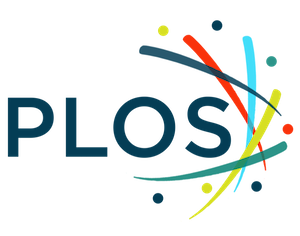
- Published: April 4, 2024
- https://doi.org/10.1371/journal.pone.0298098
- Reader Comments
Three evident and meaningful characteristics of disruptive technology are the zeroing effect that causes sustaining technology useless for its remarkable and unprecedented progress, reshaping the landscape of technology and economy, and leading the future mainstream of technology system, all of which have profound impacts and positive influences. The identification of disruptive technology is a universally difficult task. Therefore, this paper aims to enhance the technical relevance of potential disruptive technology identification results and improve the granularity and effectiveness of potential disruptive technology identification topics. According to the life cycle theory, dividing the time stage, then constructing and analyzing the dynamic of technology networks to identify potential disruptive technology. Thereby, using the Latent Dirichlet Allocation (LDA) topic model further to clarify the topic content of potential disruptive technologies. This paper takes the large civil unmanned aerial vehicles (UAVs) as an example to prove the feasibility and effectiveness of the model. The results show that the potential disruptive technology in this field is the data acquisition, main equipment, and ground platform intelligence.
Citation: Ding M, Yu W, Li R, Wang Z, Li J (2024) Research on potential disruptive technology identification based on technology network. PLoS ONE 19(4): e0298098. https://doi.org/10.1371/journal.pone.0298098
Editor: Sérgio Duarte Correia, Polytechnic Institute of Portalegre: Instituto Politecnico de Portalegre, PORTUGAL
Received: October 16, 2023; Accepted: January 17, 2024; Published: April 4, 2024
Copyright: © 2024 Ding et al. This is an open access article distributed under the terms of the Creative Commons Attribution License , which permits unrestricted use, distribution, and reproduction in any medium, provided the original author and source are credited.
Data Availability: Data files are available from the dryad database ( https://doi.org/10.5061/dryad.z612jm6j8 ).
Funding: WY is the recipient of the Jiangxi Province key S&T cooperation project (20212BDH80021). MD is the recipient of the Special Project for Scientific and Technological Cooperation of Jiangxi Province Grant Number (YC2023-S831). The funders had no role in study design, data collection and analysis, decision to publish, or preparation of the manuscript.
Competing interests: The authors have declared that no competing interests exist.
1. Introduction
Disruptive technology has a high value in technology strategies and investment decisions [ 1 ]. As a great development and growing route of traditional technologies, disruptive technology plays an important role in advancing human society, high-quality economic progress, and improving living conditions and national security. The report of the 19th National Congress of the Communist Party of China put forward corresponding requirements, focusing on key common technologies, frontier-leading technologies, modern engineering technologies, and disruptive technological innovation [ 2 ]. The " 13th Five-Year National Science and Technology Innovation Plan " and the " National Innovation-Driven Development Strategy Outline " included disruptive technological innovation. Beijing municipal party committee and municipal government attached great importance to constructing science and technology innovation. The moon landing plan proposed by Japan, the Russian Forward-looking Research Foundation, the British Advanced Research and Invention Agency, and the US Defense Advanced Research Projects Agency. Many countries and regions, government departments, and large enterprises have participated in the party to promote disruptive technological innovation [ 3 ].
However, disruptive technology in most cases is not easy to judge or identify, because of its long brewing and germination process. Thus, compared to existing sustaining technologies, it has fallen behind in core indicators from the early stage. Still, for its significant and rigid advantages in certain non-core performance indexes, disruptive technology can exist for a long time and not be replaced by any other technologies. Once, it enters the development period, the field will promote sound and rapidly. Therefore, disruptive technology identification is a necessary research task.
2. Literature review
’Disruptive technology’ [ 4 ] is a kind of new and imaginative technology, which has a disruptive effect on existing traditional or mainstream technologies. At present, there are three main methods concerning disruptive technology identification: technology roadmap and expert experience combined analysis, index evaluation analysis, and model-based analysis [ 5 ]. Among them, Vojak and Chambers (2004) [ 6 ], Kostoff (2004) [ 7 ], and Walsh (2004) [ 8 ] combined analysis of technology roadmap and expert experience towards disruptive technology identification based on the development or improvement of the technology roadmap. Carlsen (2010) [ 9 ], Lu Guangsong and Lu Ping (2011) [ 10 ] discussed and evaluated the effect of technology roadmap analysis in the identification of potentially disruptive technologies. Based on the index evaluation analysis method, Sainio and Puumalainen (2007) [ 11 ], Keller and Hüsig (2009) [ 12 ], Ganguly (2010) [ 13 ], Hang (2011) [ 14 ], Hardman et al. (2013) [ 15 ], Jia Weifeng et al. (2022) [ 16 ], and Chen Xiaoli et al. (2019) [ 17 ] put forward the corresponding subversive technical index standard theory. In terms of model analysis, Lim and Anderson (2016) [ 18 ], Adner (2002) [ 19 ], Su Qilin (2006) [ 20 ], Linton (2002) [ 21 ], A. Momenni (2016) [ 22 ], and Jia Weifeng et al. (2021) [ 23 ] established corresponding models to explore the identification of disruptive technologies.
In detail, Ganguly has implemented a potential disruptive technology evaluation system, and developed indicators of technology adoption rate, target market segmentation, technology expected utility, and existing technology maturity. Taking the online music industry as an example, it compares the cost per channel, flexibility, acquisition speed, accessibility, and robustness with existing technologies. However, there are still some limitations in the evaluation system of some indicators that still need to be combined with more qualitative recognition, music quality portability, functionality (feature + performance), and analysis. Moreover, the defined indicators lack versatility and must be customized and adjusted to apply to different industries or products. Lu Songguang and Lu Ping formulated a technology roadmap to identify the potential disruptive technologies, then pointed out the problems of stakeholder participation, technology uncertainty, market uncertainty, infrastructure uncertainty, path dependence, and path creation in this method. A.Momenni advocated identifying potential disruptive technologies through topic modeling based on k-core analysis, patent development paths, and past and current trends in technology development. This study only analyzed some invention patents, and there was information loss in the analysis process. In addition, due to the limitations of the algorithm, the number of patents analysis is also limited. At the same time, there are still some shortcomings, such as the technical classification is not clear and comprehensive, and the number of citations between different patent offices is different. From this point of view, there is still some research value in the identification method of disruptive technology.
Considering the above problems, below will explore to identify disruptive technology with patent data in the technology network. The patent literature is a major carrier of scientific and technological information. It has always been the object of analysis that researchers strived to mine and analyze disruptive technologies from it. In recent years, researchers have relied on patent data to continue to explore the direction of disruptive prediction through data mining, and have successively provided a number of innovative solutions. In the past, the analysis of disruptive technology co-occurrence networks mainly focused on the citation network, which stressed the classification of high-value patents, and had little direct correlation with technology, but the International Patent Classification (IPC) codes were closely related to technology. In the meantime, the LDA topic model was also used to explain and refine the results, to improve the accuracy and possibility of disruptive technology identification.
3. Research design
As an important indicator and carrier for measuring technology, patents contain more than 90% of the world’s technical information [ 24 ]. Considering the diffusion and transition of disruptive technological innovation, the hierarchical analysis method is used to realize technology identification from three levels: knowledge flow, invention patent, and technology theme. First, collecting the technical development data of the whole large-scale civil UAVs field, dividing the life cycle according to the trend of patent application, and grasping the development trend of this field. With the accumulation of basic knowledge theory in the early stage, technology gradually awakens and develops to generate invention patents and transition diffusion. Through the analysis of the network at the end of the germination period and the early stage of the development period, could measure the changes and transitions of each node. The rapid development and application diffusion of invention patents will continue for a long time, and many broad invention patents will gradually clarify and converge on the specific leading technologies.
The three different analyses lay the basis for identifying potential disruptive technologies and play an active role in scientific research and technology discovery. Knowledge Flow: This involves understanding existing theoretical knowledge, analyzing the technological life cycle in the field to gain in-depth learning, and applying knowledge practically. This approach facilitates knowledge reproduction and co-production. Invention Patents: These patents encompass both existing scientific and technological achievements as well as the latest breakthroughs, reflecting the pulse and direction of technological development. They serve as a crucial entry point for discovering technological innovation points and emerging solutions. Technological Themes: With continuous development, rapid growth, and integration of related technologies, these themes can be transformed into actual productivity and contribute to the sustainability of patent value. Through this method, we can identify potential disruptive technology themes from a vast expanse of knowledge. Furthermore, this provides a reference for enterprise technological research and development, as well as the positive development of the industry.
Mining the contained meaning in the feature items and the content of the information, and quantifying the information analysis carrier of the technology network. IPC represents the relationship and strength between specific technical topics. The influence of IPC cannot be measured individually. Only in the co-occurrence network can show its influence in the process of technical cooperation or technology absorption and diffusion. Therefore, taking advantage of IPC to construct a technology co-occurrence network for analysis and using its correlation degree and co-occurrence frequency to mine potential disruptive technology. Based on the theory of co-occurrence network and previous studies, the information of each node in the technology network is measured by four indicators: degree centrality, closeness centrality, betweenness centrality, and eccentricity.
The influence of IPC is determined by their control ability in the technology network and their dependence on other IPC codes, see Eqs ( 1 ) to ( 4 ). Degree centrality can calculate the degree to which a node is associated with all other nodes in the network and is the simplest index to quantify the influence of nodes. The larger the degree of a node is, the higher the degree centrality of the node is, which means that the node is more important in the network and the cohesion is stronger. The betweenness centrality depends on the degree to which a node is at the center of multiple nodes, which can be measured according to the mediating role of nodes in the network. The high betweenness centrality of the node means it is located on the shortest path of multiple other nodes, which indicates that the node has strong technical or resource control ability in the technology network. Closeness centrality can be used to check whether the node is at the core of the technology network, intending to the closeness of the node to all other nodes in the network. The eccentricity implies the distance from a given starting node to the farthest node from it.
The Eq ( 1 ) is the calculation formula of the degree centrality of each node, represents the connection relationship between node i and node j, and N represents the total number of network nodes; the Eq ( 2 ) is the calculation formula of the betweenness centrality of each node, represents the number of shortest paths that node j and node t are connected and pass through i, represents the number of all shortest paths, and N represents the total number of network nodes. Eq ( 3 ) is the calculation formula of closeness centrality, represents the network distance between node i and node j, and N is the total number of network nodes. The Eq ( 4 ) is the eccentricity calculation formula, is defined as the same Eq ( 3 ), and N is the total number of network nodes. In the germination stage, according to the relevant indicators, the low-impact high-potential nodes are divided into two types: the nodes in type 1 have the characteristics of low degree centrality, low betweenness centrality, and high closeness centrality. After entering the technology network, they are connected with important nodes and closer to the center position; the nodes in type 2 have the characteristics of low degree centrality, low betweenness centrality, and high eccentricity. When entering the technology network, they are not connected to the important nodes of the existing network and are in a more remote network position.
In order to further analyze the potential disruptive technologies in the field, the LDA topic model is used to interpret the invention patent abstracts mapped by the transition diffusion IPC. On the basis of the identification of the transition and diffusion of the technology network nodes, a more fine-grained analysis is carried out to verify the results of the analysis obtained before, furthermore to determine and refine the specific research and development direction of the previous identification technology results. The overall analysis process is shown in Fig 1 .
- PPT PowerPoint slide
- PNG larger image
- TIFF original image
https://doi.org/10.1371/journal.pone.0298098.g001
4. Empirical analysis
4.1 data acquisition and processing.
Firstly, Familiar with the development status of this field is preliminarily, and the technical decomposition of the required research field through the paper and patent query. Meanwhile, constructing the retrieval strategy, in consideration of the previous decomposition of the key technologies of large civil UAV patents [ 25 ]. Moreover, obtaining the relevant invention patent data through the Incopat patent database, and then preprocessing the data to facilitate the analysis of the subsequent technology network.
The UAVs have the advantages of low cost, small size, flexible maneuverability, convenience to use, strong combat survivability, and low combat environment requirements, which are valued by domestic and foreign research and development teams. After more than 30 years of accumulation and development of the domestic UAV application market, the demand scenario has gradually expanded from the initial military to consumption. According to the application field of UAVs, it can be roughly divided into military type and civil type. Large civil UAVs are one of the future development hotspots in the field of UAVs. With the continuous research and development of civil UAVs in various countries, it has been widely used in agricultural plant protection, film and television aerial photography, transportation, emergency search and rescue, power inspection, communication relay, meteorological monitoring, environmental protection, public safety, and other fields. Therefore, it is of great significance to identify the potential disruptive technology of large civil UAVs.
4.2 Life cycle analysis
Disruptive technology essentially belongs to technologies, which is in line with the general law of technological development. The evolution rules are consistent with the general law of the technology life cycle, and show different characteristics at different stages. Therefore, the technology life cycle is used to divide the development stage and show the dynamic development process of disruptive technology innovation. Due to the small number of patents in the field before 1970 and the slow development, the patent data from 1971 to 2020 are selected for patent life cycle analysis. According to the search results, this paper uses Loget Lab 4.0 software to carry out logistic curve fitting and divides the patent life cycle of a large civil UAV into four cycles, namely the germination period, growth period, maturity period, and decline period. Among them, the germination period is 1973–2012, the growth period is 2013–2016, the maturity period is 2017–2034, and the recession period is after 2035. According to the characteristics of slow development in its germination period and gradual expansion in its growth period, the whole germination period from 2003 to 2007 was selected as the first stage, from 2008 to 2012 as the second stage, from 2013 to 2015 as the third stage in the early growth period, and from 2016 to 2018 as the fourth stage. The technology network was constructed and the relevant index data were analyzed.
According to the change in the position of the technology nodes in the network in the germination stage and the growth stage, the IPC codes with subversive potential are selected.
4.3 Potential disruptive technology identification results
According to the technology network constructed in the germination stage, the indicators of each node are measured to determine the distribution of each node in the technology network. In the first phase, G05D1 / 00 (control of the position, course, altitude, or attitude of a vehicle on land, in water, in the air, or in space, such as an autopilot (a radio navigation system or a similar system using other waves to enter G01S) [2006.01]) occupies the core position of the network and represents the flight control technology of a large civil UAV. The flight control system is the key technology of application and development in the field of UAVs [ 26 ]. The flight control system is the key core part of UAV control. As the brain of a UAV, it has an irreplaceable position, and generally includes three parts: sensor, airborne computer, and servo action equipment. The functions realized mainly include three categories: UAV attitude stability and control, UAV mission equipment management and emergency control, as shown in Table 1 .
https://doi.org/10.1371/journal.pone.0298098.t001
Through calculation, it is found that the number of nodes in the germination stage conforming to the two types described in Table 1 is 148. Furthermore, according to the technical development procedures, the degree centrality and betweenness centrality of the above nodes in the technology network of the two stages of the growth period are measured to test whether one or more indicators exceed the average level of the current period, so as to determine the expansion progress and influence degree of the nodes. As a result, at least one of the 91 node influence indicators was selected to exceed the average level of the current period, that is, at least one expansion transition occurred during the growth period. Therefore, compared with other nodes, they have the possibility of subverting the existing knowledge structure and theoretical basis in the field. Further found that H04N7 / 00 (TV system (components into the H04N3 / 00, H04N5 / 00: for digital video signal encoding, decoding, compression or decompression method or device; the degree centrality and closeness centrality of H04N7 / 00 increase from 0.03 and 0.41 in the first stage to 0.41 and 0.6 in the fourth stage. At the same time, in the fourth stage, it has a high betweenness centrality, which controls and restricts the development of other technologies in the field. This shows that image communication technology has potential subversiveness in the field of large civil UAVs.
In addition, from the overall observation of the technology network, it can be found that there is a possibility of important technological innovation in the field of large civil UAVs. Taking the first stage of the germination period as an example, its overall network presents a state where the center is more concentrated and the edge is scattered, and the IPC codes of the nodes with a higher degree of central technology integration is mostly concentrated on aircraft and regulation control. Distributed in the edge of the more dispersed nodes, such as the transmission of digital information; survey navigation; video metrology, or photogrammetry; measuring azimuth information such as level, distance or azimuth is also an important technology in the field of UAVs. It can be seen that in the early invention patent technology network, there are certain emerging technologies that disperse the edge of the network and have the possibility of diffusion and transition, as shown in Fig 2 .
https://doi.org/10.1371/journal.pone.0298098.g002
Finally, the IPC nodes of the transition diffusion in the knowledge flow are mapped to invention patents, and 1271 related invention patents are required to be analyzed specifically. There are several technical topics and research directions in this part of the invention patent, which need to be further focused and explained.
4.4 The interpretation and test of the LDA topic model
Extract the abstracts of 1271 selected inventions, and combine the LDA topic model for technical topic clustering. The Python software Jieba and Gensim are used to segment words and remove stop words. The term frequency–inverse document frequency (TF-IDF) algorithm is used to calculate the frequency weight of technical keywords in the patent literature, and then the model is used to sort out the technical topics, and the perplexity value of each topic is calculated, as shown in Fig 3 .
https://doi.org/10.1371/journal.pone.0298098.g003
At the same time, as the intertopic distance map shows, according to the size and number of bubbles, found that the frequency of themes 1, 2, and 3 are higher. In addition, based on the analysis of the distribution dimension and location of the theme, noticed that theme 2 and theme 3 have the feature word intersection and close connection; compared with Topic 1, the Jensen-Shannon divergence (JSD) distance between the two topics is far, and the content is quite different, as shown in Fig 4 .
https://doi.org/10.1371/journal.pone.0298098.g004
Combined with the confusion curve and the actual analysis results, the optimal number of topics is finally selected to be 3, as shown in Table 2 .
https://doi.org/10.1371/journal.pone.0298098.t002
Potential disruptive technology identification and interpretation of clustering topic results:
Theme 1 includes image equipment, information data, target area monitoring, and user terminal interaction are all associated with data acquisition, especially in the application of vehicle-mounted drones and autonomous driving. Further analysis of Theme 1 is provided below.
National deployment: in 2002, the U.S. Department of Transportation and the National Aeronautics and Space Administration focused on launching the application of UAVs in the field of transportation. In 2010, Shanghai purchased two small UAVs to participate in environmental resource management, maritime search and rescue, law enforcement investigation and evidence collection, traffic flow monitoring, and other work content, focusing on public emergencies and on-site emergency command of the information collection [ 27 ]. In the same year, China’s State Council and the Central Military Commission issued the Opinions on Deepening the Reform of China’s Low Altitude Airspace Management, which provided an opportunity for the promotion of drones in the civil sector, such as border monitoring, disaster monitoring, and assessment. Consumer drones are mainly used for leisure purposes such as aerial photography and gaming, and most of them adopt multi-rotor platforms with lower cost of construction. Enterprise R&D: In 2014, Renault released a KWID concept car, equipped with a quadcopter drone, which was usually automatically stowed on the roof of the car, and could be automatically flown and lifted into the air when needed through the built-in GPS and camera, combined with the tablet operation of the console. In 2015, Gopro, Inc. entered the "Small Drone Alliance" and disclosed several drones for use in aerial photography and gaming., and officially released the first multi-motor driven drone. Shenzhen DJI Innovation Technology Co., Ltd (DJI) also announced that it would apply self-developed cameras to its future drone products [ 28 ]. In early 2016, DJI released the Elf Phantom 4 drone, which was the first time it successfully introduced binocular vision technology in a drone, achieving autonomous following and autonomous obstacle avoidance, which was the key factor that prompted DJI’s decision to enter the field of intelligent driving. In 2017, Land Rover launched a production model of the vehicle drone, the Discovery SOV Edition. It was equipped with an onboard drone, which could take off and land the roof drone at high speeds on the car, through an electromagnetic holding and automatic positioning system. Industry development: Data Society and Digital Economy and the "Belt and Road" Cooperation International Academic Conference mentioned the role of information technology upgrades in the expansion of drone application scenarios. In 2019, the Ministry of Industry and Information Technology issued 5G licenses to China Telecom Corp Ltd, China Mobile Communications Corporation, China United Network Communication Group Co., Ltd, and other communication units, the construction of the network and the application of innovation go hand in hand [ 29 ]. In 2020, the civil drone industry launched the "Five-Year Action Programme for Promoting the Construction of a New Infrastructure for Civil Aviation", which proposed to expand the application scenarios of UAV logistics and transportation, terminal distribution, etc. At Some airports, it has achieved the related infrastructure of unmanned aerial vehicles, unmanned vehicles, and other operating conditions, to explore the military and civil aviation cooperation, manned aircraft unmanned aircraft fusion operation, and air-ground integration operation.
Theme 2 includes major airframe equipment such as airframe, motor, rotor, controller, remote control, controller, propeller, etc., involving airframe structure, electrical system, flight control system, and power system. The fuselage platform is the bearing platform of civil UAVs, involving the fuselage, arm, frame, landing gear, other structural components, and their connecting structures. The power system is the key guarantee for the endurance of civil UAVs, including piston, fuel gas, oil-electric hybrid, electric motors, and other modes. The aerodynamic layout is the vital assurance for the success of the flight of civil UAVs, and it can be classified into rotary wing, fixed wing, and mixed wing, the rotary wing can be specifically classified into single rotor, fixed wing, and hybrid wing [ 30 ]. The research and development direction of the UAV control system is closely related to the above theme, and the development of UAV technology and market demand [ 31 ]. The UAV control station is the command center where the operator can control and supervise the UAV remotely. At the same time, the control station is also equipped with a series of functions such as online mission planning, data control, image and intelligence processing, and view display [ 32 ]. One of the main reasons for civil UAVs to malfunction and go out of control is mechanical failure. In recent years, there have been several incidents at home and abroad in which drones crashed and hurt people due to battery or motor failures. The Karma drone launched by the foreign company GoPro, Inc. was also recalled because of the basic battery fixation problem. Therefore, the quality of the body structure, material, and manufacturing process of civil UAVs will directly affect the safety and operational efficiency of their operation. Without the influence of external factors, the internal stability of the UAV itself is also extremely important. Moreover, the quality of the materials, the compatibility of the internal structures, and the manufacturing process are all key elements that affect the safety and stability of UAVs [ 33 ].
Theme 3 indicates the detection and intelligence of the ground platform, especially in the application of emergency and patrol of electric power. The U.S. Federal Aviation Administration (FAA) proposed 19 types of services for Unmanned Aerial System Traffic Management. The European Single European Sky ATM Research (SESAR) listed 30 functions that U-space should have. The vast majority of the functions need to be newly defined and developed, and they were all based on digital and automated services for intelligent system function development. 2009 was the beginning year of inspection drones in China, with the State Grid formally setting up a project to develop a drone inspection system. 17 August 2021, San Diego—following the critical breakthrough of the Qualcomm Flight platform, which enabled the successful unmanned flight of the "Witty" helicopter on Mars. Thus, Qualcomm Technologies, Inc. continued to strengthen its leadership in the UAV space with the introduction of the world’s first 5G and AI-enabled drone platform, and the reference design of the Qualcomm Flight RB5 5G platform. In 2023, Foia Intelligent Technology Development (Jiangsu) Co., Ltd. (Fioa) launched the Zhifang A30EC series of UAV nests to become the ground infrastructure that enabled fully automated UAV operations. The compact and lightweight design paired with Fioa Intelligence’s RuiYun control system realized the automatic power change, battery management, temperature control and dehumidification, uninterruptible power supply, remote communication, intelligent lighting, and weather sensing. This innovative equipment completely changed the mode of manual on-site operation of UAVs, improved operational efficiency, and realized fully automatic operation of UAVs.
In summary, three potential disruptive technology directions are selected through technology network analysis and the LDA topic model. The future development trend of large civil UAVs is roughly the same as the identified technical topics, thus the identification method has certain effectiveness. Moreover, for the above technology direction, enterprises are active in R&D and innovation, patent applications are gradually increasing, technology development tends to be mature, and the market tends to be stable and saturated, thus it is more difficult to enter. The main patent applicants have rapid momentum of technology research and development, and the technology competition in the large civil UAV industry is more intense. It is recommended that they strengthen cooperation between industry, academia, and research to form a synergy in technology development and achieve breakthroughs in disruptive technologies.
4.5 Empirical test
To test the validity of the empirical data, use the spss26 software for the Mann-Whitney U test. First, the patents that do not have potential disruptive technologies in the germination period are set to 1 group and otherwise set to 2 groups. Secondly, the Citation Index and Inventor Index are selected as indicators, and the entropy weighting method and linear weighting method are adopted to calculate the corresponding index scores of patents. Among them, the Citation Index normalizes the patent age of each patent, which represents technological innovation and has a significant impact on subsequent technological development, the more often a patent is cited, the greater its patent value, see Eq ( 5 ). The Inventor Index measures the inventor of a patent, the greater the number of inventors on a patent, the greater the creative effort to develop the patents, see Eq ( 6 ).
After testing, the p-value was 0.034, lower than 0.05, the Z value was 2.118, the average rank of group 1 was 494.57, and the average rank of group 2 was 541.62. Therefore, the original hypothesis is rejected, and it is concluded that there is a significant difference between the two sets of data and the inventor in terms of the number of citations. In summary, the patents of the two groups are superior and subversive in terms of patent value, continuous influence, and creativity.
5. Conclusion
Main contributions and innovations: This paper simulates the development path of disruptive technology from knowledge literature, invention patents, and technology topics through an analytic hierarchy process to identify potential technology topics in the early stage of its development. At the same time, the technology network is constructed with the IPC codes of patents as the entry point, which enhances the degree of correlation between the recognition results and the technology compared with the citation network analysis. Meanwhile, in order to avoid the problem that the IPC codes range is relatively large, the LDA topic model is used to extract the technical topics that should be mapped to the invention patent, which improves the granularity and accuracy of the analysis results.
(1) According to the life cycle theory, the invention patent information from 2003 to 2018 is intercepted, and the technical network construction and dynamic analysis are carried out according to the invention patent IPC, and the node-image communication technology with prominent diffusion and significant transition characteristics is selected as the potential disruptive technology.
(2) Search for the invention patent information mapped by the potential disruptive IPC, and obtain the technical topic module of the data acquisition, main equipment, and ground platform intelligence through LDA topic model clustering. After querying and verifying the literature in the field, the topic identification results of the technology have a certain correlation with the development trend of the industry.
In view of the above analysis, there are still some shortcomings: this method only considers the IPC codes of patents and does not involve the technology market, consumers, policies, and other factors, so it has certain limitations. In addition, due to the time lag of patent data, the analysis method in this paper has limitations in the prediction of disruptive technologies. At the same time, it can be further combined with other literature, evaluation indicators, theme evolution analysis, and other comprehensive analyses and research.
- View Article
- Google Scholar
- 5. WANG, B. The diffusion mechanism of disruptive technology innovation from the perspective of complex networks[D]. Thesis, Jilin university, 2022. https://doi.org/10.27162/d.cnki.gjlin.2022.007770 .
- 17. Chen X.; Han T. Disruptive Technology Forecasting Based on Gartner Hype Cycle. In 2019 IEEE TECHNOLOGY & ENGINEERING MANAGEMENT CONFERENCE (TEMSCON); IEEE: New York, 2019. https://doi.org/10.1109/temscon.2019.8813649 .
- 27. SHANG F.; JIAN C. Analysis of UAV signal characteristics[C]. In 2020 China Information and Communication Conference (CICC 2020); China Institute of Communications, 2020; pp 389–391. https://doi.org/10.26914/c.cnkihy.2020.055855 .
- 28. PENG B.; ZHAGN Y.; CAI X.; Li S. Research status and prospect of traffic monitoring based on UAV[C]. In Proceedings of the 11th China Intelligent Transportation Annual Conference; China Intelligent Transportation Systems Association, 2016; pp 111–120.
- 29. SHI C.; DU B.; LI J. The digital economy has driven the civil UAV industry to jump from 1.0 to 3.0. In Proceedings of the International Symposium on Data Society and Digital Economy and the Belt and Road Cooperation[C]; Intelligent Oilfield Institute of Chang’an University、Digital experiment and Research Center of Chang’an University, School of Digital Economics·GDUFE、China Institute of Data Research, Guangdong University of Finance and Economics, 2022; pp 104–110. https://doi.org/10.26914/c.cnkihy.2022.040373 .
- 30. Zhao Y. (2020). Research on Development Trends and Countermeasures of Global Civil UAV Industry Based on Patent Analysis [Degree thesis, Chongqing University of Technology]. https://doi.org/10.27753/d.cnki.gcqgx.2020.000204 .
- Browse All Articles
- Newsletter Sign-Up
Disruption →
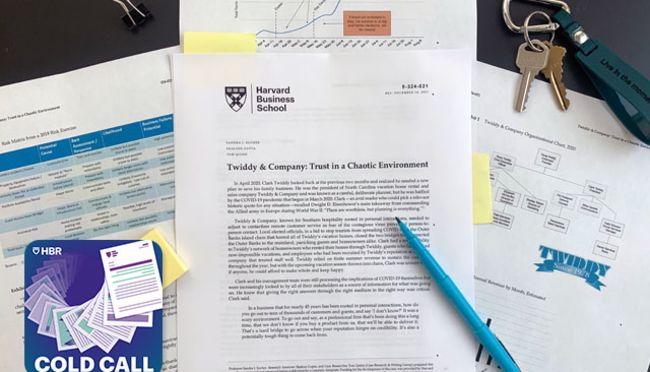
- 21 May 2024
- Cold Call Podcast
The Importance of Trust for Managing through a Crisis
In March 2020, Twiddy & Company, a family-owned vacation rental company known for hospitality rooted in personal interactions, needed to adjust to contactless, remote customer service. With the upcoming vacation season thrown into chaos, President Clark Twiddy had a responsibility to the company’s network of homeowners who rented their homes through the company, to guests who had booked vacations, and to employees who had been recruited by Twiddy’s reputation for treating staff well. Who, if anyone, could he afford to make whole and keep happy? Harvard Business School professor Sandra Sucher, author of the book The Power of Trust: How Companies Build It, Lose It, Regain It, discusses how Twiddy leaned into trust to weather the COVID-19 pandemic in her case, “Twiddy & Company: Trust in a Chaotic Environment.”
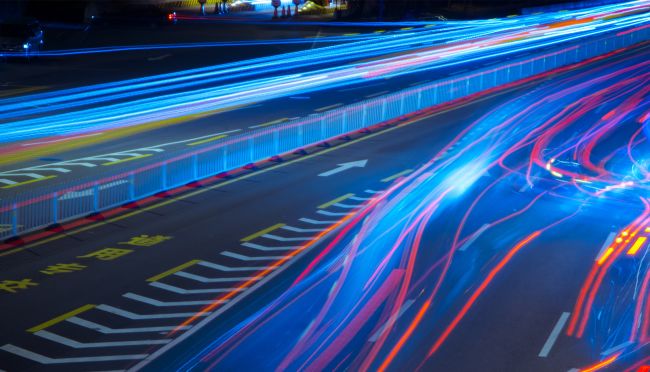
- 07 Feb 2022
- Research & Ideas
Digital Transformation: A New Roadmap for Success
Is your company reaping the rewards of digital transformation yet? Linda Hill and colleagues offer seven guiding principles for transformations at any stage—nascent, progressing, or stalled. Open for comment; 0 Comments.
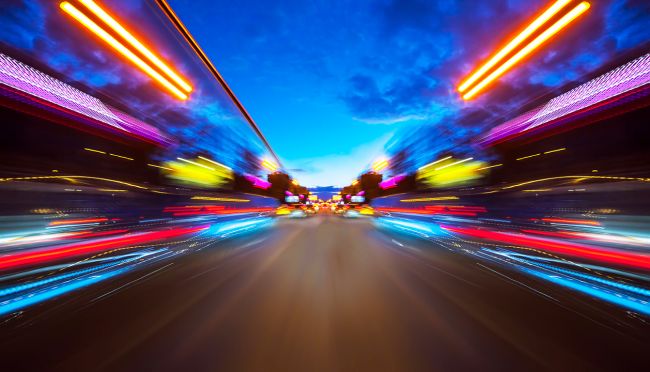
- 31 Jan 2022
Where Can Digital Transformation Take You? Insights from 1,700 Leaders
Digital transformation seems like a journey without end, but many companies are forging ahead. Linda Hill and colleagues reveal six qualities that set digitally mature organizations apart. Open for comment; 0 Comments.
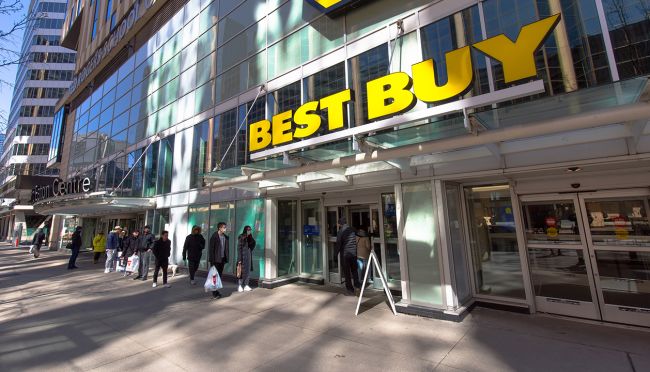
- 04 May 2021
Best Buy: How Human Connection Saved a Failing Retailer
In The Heart of Business, former Best Buy CEO Hubert Joly shares how he revived the ailing electronics chain, offering a guide to leaders facing seemingly insurmountable challenges. Open for comment; 0 Comments.
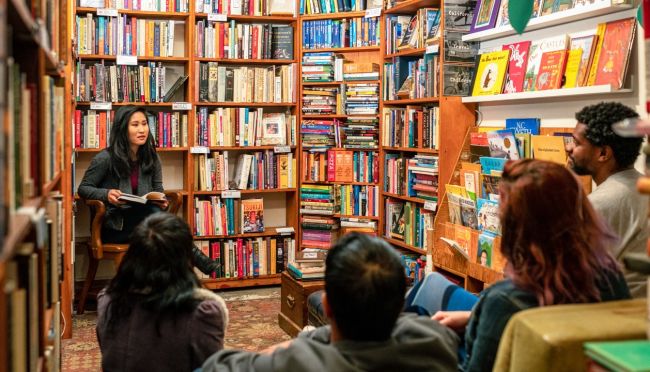
- 21 Jan 2020
Lessons for Retailers from the Rebirth of Indie Bookstores
Independent bookstores are resurging. Their strategies offer lessons for many disrupted industries to compete against Amazon and other digital retailers, says Ryan Raffaelli. Open for comment; 0 Comments.
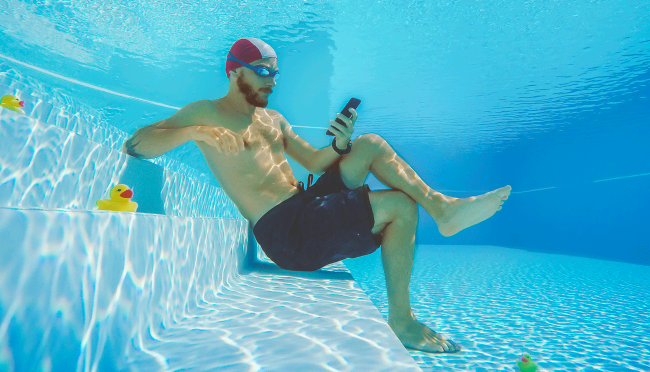
- 14 Aug 2019
- Sharpening Your Skills
The Manager's Guide to Leveraging Disruption
Clayton M. Christensen's seminal book, The Innovator's Dilemma, helped ignite the idea of innovative disruption. His Harvard Business School colleagues have been adding to innovation research ever since. Open for comment; 0 Comments.
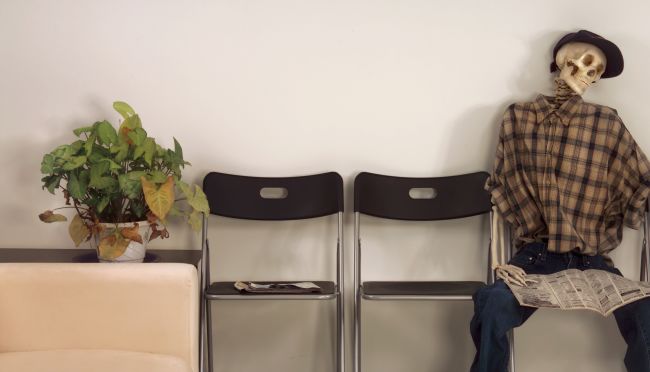
- 08 Jul 2019
Are Paywalls Saving Newspapers?
Newspapers with reputable brands and unique content are finding success behind paywalls. For most papers, however, the future is not so clear, says Doug J. Chung. Open for comment; 0 Comments.
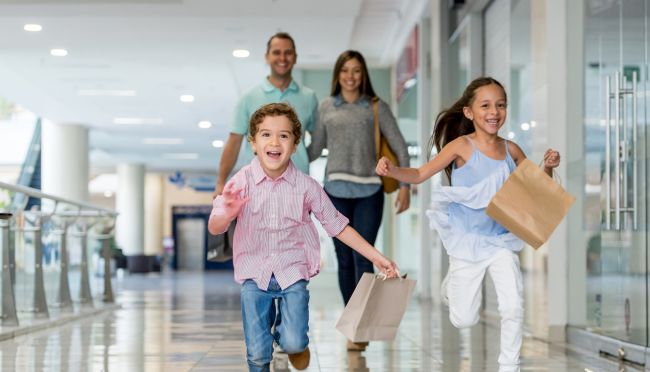
- 18 Feb 2019
What’s Really Disrupting Business? It’s Not Technology
Technology doesn't drive disruption—customers do. In a new book, marketing professor Thales Teixeira argues that successful disruptors are faster to spot and serve emerging customer needs than larger competitors. Open for comment; 0 Comments.
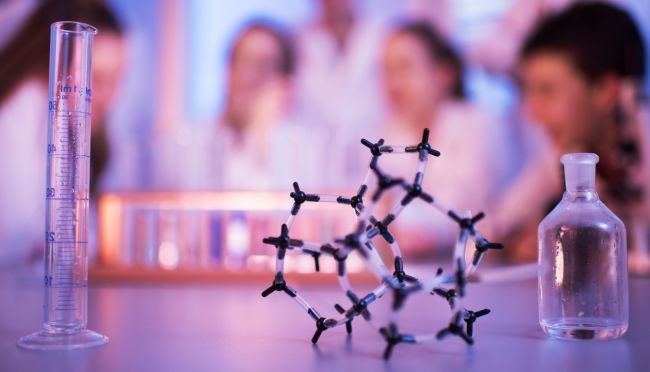
- 07 Feb 2019
How Big Companies Can Outrun Disruption
Large companies can be easy targets for disruption, but Gary Pisano says there are steps that can keep them ahead of the innovation curve. Rule 1: Don't emulate startup cultures. Open for comment; 0 Comments.
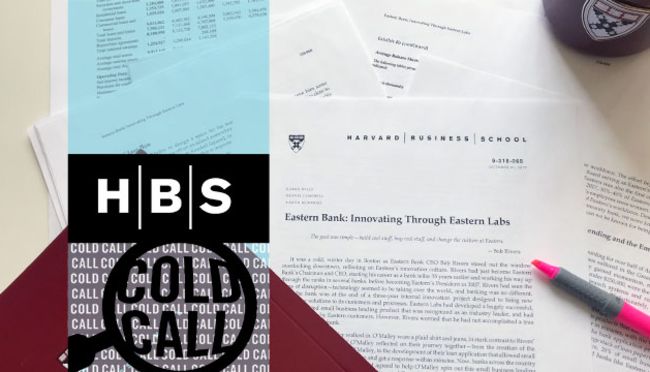
- 20 Dec 2018
Using Fintech to Disrupt Eastern Bank from Within
When Eastern Bank decided to battle a threat from new competitors, it hired a fintech executive to set up Eastern Labs and start innovating. Karen Mills discusses her case study on what happened next. Open for comment; 0 Comments.
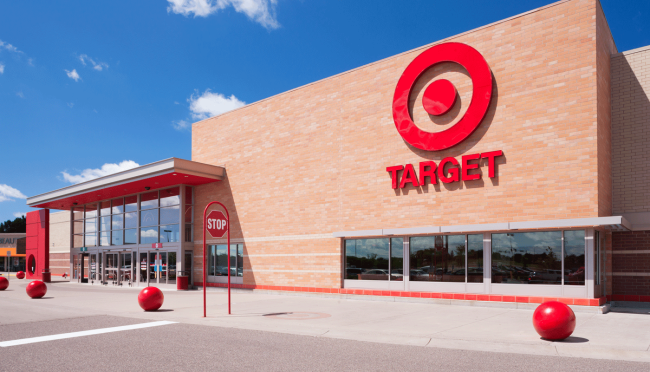
- 28 Nov 2018
On Target: Rethinking the Retail Website
Target is one big-brand retailer that seems to have survived and even thrived in the apocalyptic retail landscape. What's its secret? Srikant Datar discusses the company's relentless focus on online data. Open for comment; 0 Comments.
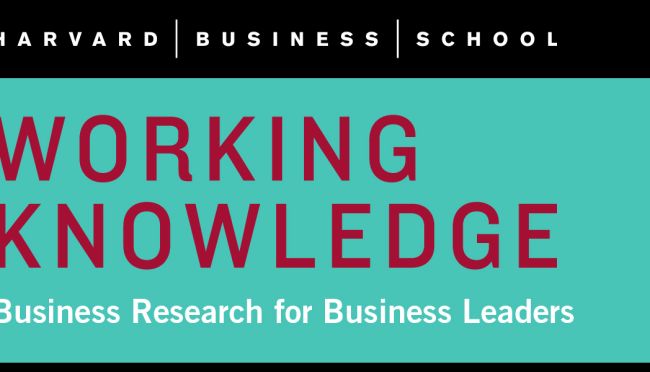
- 20 Apr 2018
- Working Paper Summaries
Executive Education in the Digital Vortex: The Disruption of the Supply Landscape
The competitive landscape of executive education is feeling a tectonic shift even as demand grows for managerial skills. This study maps and analyzes the major providers of executive education programs, including business schools, consultancies, and corporate universities, to better understand and explain the industry’s present and future dynamics.
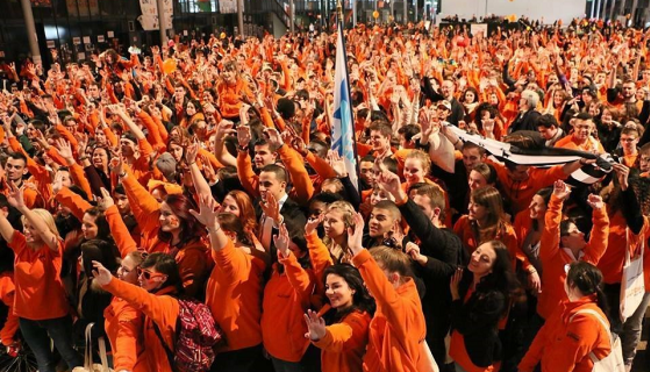
- 20 Sep 2017
The Three Types of Leaders Who Create Radical Change
Every successful social movement requires three distinct leadership roles: the agitator, the innovator, and the orchestrator, according to institutional change expert Julie Battilana. Open for comment; 0 Comments.
- 02 Mar 2015
Retail Reaches a Tipping Point—Which Stores Will Survive?
Part 1: The new book Retail Revolution: Will Your Brick and Mortar Store Survive? argues that ecommerce is about to deal severe blows to many familiar store-based brands—even including Walmart. Here's how retailers can fight back, according to Rajiv Lal, José Alvarez, and Dan Greenberg. Open for comment; 0 Comments.
- 25 Jul 2011
How Disruptive Innovation is Remaking the University
In The Innovative University, authors Clayton M. Christensen and Henry J. Eyring take Christensen's theory of disruptive innovation to the field of higher education, where new online institutions and learning tools are challenging the future of traditional colleges and universities. Key concepts include: A disruptive innovation brings to market a product or service that isn't as good as the best traditional offerings, but is less expensive and easier to use. Online learning is a disruptive technology that is making colleges and universities reconsider their higher education models. Closed for comment; 0 Comments.
- 05 Apr 2010
HBS Cases: iPads, Kindles, and the Close of a Chapter in Book Publishing
Book publishing is changing before our very eyes, even if the industry itself is fighting the transition with every comma it can muster. Harvard Business School professor Peter Olson, former CEO of Random House, wonders if books themselves may be in jeopardy. Key concepts include: The traditional book publishing and distribution system is under pressure to change to digital e-books. Publishers should consider a strategy of cooperation rather than competition with online retailers. Adding video and other multimedia capabilities will make e-books more attractive in the textbook industry. The fundamental question to be asked in the Internet age is, how popular will books remain? Closed for comment; 0 Comments.
- 24 Apr 2009
Corporate Social Entrepreneurship
Accelerated organizational transformation faces a host of obstacles well-documented in the change management literature. Because corporate social entrepreneurship (CSE) expands the core purpose of corporations and their organizational values, it constitutes fundamental change that can be particularly threatening and resisted. Furthermore, it pushes the corporation's actions more broadly and deeply into the area of social value creation where the firm's experiences and skill sets are less developed. The disruptive social innovations intrinsic to the CSE approach amplify this zone of discomfort. Fortunately, the experiences of innovative companies such as Timberland and Starbucks show how these challenges may be overcome. Key concepts include: Values-based leadership, the synergistic generation of social and economic value, and strategic cross-sector alliances are key ingredients to achieving a sustainably successful business. For companies to move their corporate social responsibility (CSR) activities to the next level, they need to rethink their current approaches to CSR, tapping into the creativity of each individual. Like all entrepreneurship, CSE is about creating disruptive change in the pursuit of new opportunities. It combines the willingness and desire to create joint economic and social value with the entrepreneurial redesign, systems development, and action necessary to carry it out. Closed for comment; 0 Comments.
- 08 Apr 2009
Clayton Christensen on Disrupting Health Care
In The Innovator's Prescription, Clayton Christensen and his coauthors target disruptive innovations that will make health care both more affordable and more effective. From the HBS Alumni Bulletin. Closed for comment; 0 Comments.
- 04 Sep 2007
Jumpstarting Innovation: Using Disruption to Your Advantage
Fostering innovation in a mature company can often seem like a swim upstream—the needs of the existing business often overwhelm attempts to create something new. Harvard Business School professor Lynda M. Applegate shows how one of the forces that threatens established companies can also be a source of salvation: disruptive change. Plus: Innovation worksheets. Key concepts include: Jumpstarting innovation is a critical business imperative. Executives realize that radical change is needed but do not feel equipped to make such change. Disruptions in the business environment allow new entrants or forward-thinking established players to introduce innovations that transform the way companies do business and consumers behave. Disruptive changes that might serve as the source of innovation include technology shifts, new business models, industry dynamics, global opportunities, and regulatory changes. Closed for comment; 0 Comments.
- 15 Sep 2003
The Lessons of New-Market Disruption
Teradyne was successful. Hewlett-Packard was not. Professor Clark Gilbert writes about how two companies had such different results with disruptive innovation. Closed for comment; 0 Comments.
ENHANCING TOURISM INNOVATION THROUGH DISRUPTIVE TECHNOLOGY AND STRATEGIC ALLIANCES: EVIDENCE FROM POPULAR DESTINATIONS IN WEST JAVA
Description.
ENHANCING TOURISM INNOVATION THROUGH DISRUPTIVE TECHNOLOGY AND STRATEGIC ALLIANCES: EVIDENCE FROM POPULAR DESTINATIONS IN WEST JAVA The research objects focus on the following construct: 1. Disruptive technology 2. tourism innovation 3. Strategic alliances 4. Local cultural engagement This study employs a quantitative, explanatory survey design to investigate the role of disruptive technology and strategic alliances in fostering tourism innovation in popular destinations in West Java. This approach is chosen to quantify relationships between variables and to provide a clear explanatory framework for understanding the impact of these factors on tourism innovation. The primary instrument used for data collection is a structured questionnaire, utilizing a nine-point (1 – 9) Likert scale to measure respondents' perceptions and attitudes. The questionnaire encompasses various indicators related to disruptive technology (enhanced visitor experience, operational efficiency, sustainability impact, employee productivity, and accessibility) and strategic alliances (compatible goals, complementary skills, cooperative cultures, and commensurate risk). A pilot test was conducted to ensure the validity and reliability of the instrument, involving a small sample of respondents similar to the study population. The unit of analysis for this study comprises approximately 310 popular tourist destinations in West Java. These destinations were selected based on their popularity and significance to the region's tourism industry. Data collected from the survey were analyzed using Partial Least Squares Structural Equation Modeling (PLS-SEM). This method was chosen due to its suitability for analyzing complex models and its ability to handle small to medium-sized samples effectively. The analysis involved two main stages: the measurement model and the structural model. The measurement model assessed the reliability and validity of the constructs, while the structural model evaluated the hypothesized relationships between disruptive technology, strategic alliances, and tourism innovation. The PLS-SEM approach provided robust insights into the direct and moderating effects of local cultural engagement on the studied relationships, offering a comprehensive understanding of the factors driving tourism innovation in West Java.
Technology and Business Strategy
Digital Uncertainty and Digital Solutions
- © 2021
- Igor Stepnov 0
Moscow State Institute of International Relations (MGIMO University), Moscow, Russia Financial University under the Government of the Russian Federation, Moscow, Russia
You can also search for this editor in PubMed Google Scholar
- Presents a truly international perspective by representing viewpoints from Russia, China, Hungary, Italy, Serbia and other countries
- Examines the impact of new technologies over a wide variety of industries, including city management and supply chains
- Looks at how digitization and other advances in technology have informed new legislation and governance
25k Accesses
36 Citations
This is a preview of subscription content, log in via an institution to check access.
Access this book
Subscribe and save.
- Get 10 units per month
- Download Article/Chapter or eBook
- 1 Unit = 1 Article or 1 Chapter
- Cancel anytime
- Available as EPUB and PDF
- Read on any device
- Instant download
- Own it forever
- Compact, lightweight edition
- Dispatched in 3 to 5 business days
- Free shipping worldwide - see info
- Durable hardcover edition
Tax calculation will be finalised at checkout
Other ways to access
Licence this eBook for your library
Institutional subscriptions
About this book
This book examines how new technologies have transformed global markets, as well as global business strategy. It explores how digitalization, artificial intelligence, virtual reality, and other changes in technology have led both to new opportunities but also to increased uncertainty within both business and legislature.
By pulling together academics from Russia, China, France, Hungary, Azerbaijan, Tajikistan and other countries, this book provides a truly international perspective on the impact of new technologies across areas including smart cities, corporate governance, EU legislation and logistical enterprise. It will be valuable reading for academics interested in digitization, digital business, digital entrepreneurship and the way that technological change has informed strategy.
Similar content being viewed by others
Digitalization: A Literature Review and Research Agenda
Introduction to Digitalization Cases Vol. 2: Mastering Digital Transformation for Global Business
Shifts in Digital Technologies’ Implementation During the COVID-19 Pandemic: The Case of Russia
- Technological Leadership
- Globalization
- Digitalization
- Economic Barriers
- Future Uncertainty
Table of contents (22 chapters)
Front matter, introduction: the limits of digital leadership: from “agile” to “great leap”.
Igor Stepnov
Digital Breakthrough
The uncertainty of the technological future, a future with artificial intelligence: strategy for success.
- Nidjad Asadli
Technological Revolution in Financial Intermediation
- Galina Panova, Irina Larionova, Istvan Lengyel
The Digital Vector of Ensuring Economic Security of the Company
- Artem Krivtsov, Leyla Berdnikova
Modernization or New Engineering: Models of Leadership in the Global Civil Aviation Market
- Anna Kolesnikova, Julia Kovalchuk
Digital Twins Application in Managing the Scientific and Technological Development of High-Tech Industries
- Vladislav Klochkov, Irina Selezneva, Julia Kovalchuk
Individualization of Approaches in Scenarios of Survival and Development for Companies in the Digital Environment
- Zhaklin Sarkisyan, Maya Tikhonova
Artificial Intelligence in Public Governance
- Sergey Kamolov, Kirill Teteryatnikov
Global Navigation Satellite Systems as Digital Solutions for Smart Cities
- Sergey Kamolov, Grigory Tarasov
The Digital Trade Route to an Economic Space in the Eurasian Economic Union: Institutions and Technology
- Kobilzhon Zoidov, Alekxey Medkov
Digitalization as Objective Factor of the Substitution of the Labor by the Capital
- Vladimir Osipov, Yuan Lunqu, Liu Dun, Geng Yuan
Spurt of Economic Systems
Investment in the modernization and reconstruction of industrial equipment: an evaluation of multiplier effects in russia.
- Nina Goridko, Elena Krasina
Risks of Modernizing National Economies in the Conditions of the Technological Leap
- Zhaklin Sarkisyan
Imperfect Mechanisms for the Use and Protection of Intellectual Property as an Organizational Barrier in the Movement to Global Technological Leadership
- Alexander Litvinenko, Julia Lozina, Lyudmila Chernikova
Smart Cities’ Hyper-Economies as the Reflection of Digital Governance Leadership
- Sergey Kamolov, Alexander Blokhin
Smart Contracts and Corporate Governance: Prospects and Risks of Business Digitalization
- Alexander Yukhno, Vladimir Osipov
Editors and Affiliations
Moscow state institute of international relations (mgimo university), moscow, russia, financial university under the government of the russian federation, moscow, russia, about the editor, bibliographic information.
Book Title : Technology and Business Strategy
Book Subtitle : Digital Uncertainty and Digital Solutions
Editors : Igor Stepnov
DOI : https://doi.org/10.1007/978-3-030-63974-7
Publisher : Palgrave Macmillan Cham
eBook Packages : Business and Management , Business and Management (R0)
Copyright Information : The Editor(s) (if applicable) and The Author(s), under exclusive license to Springer Nature Switzerland AG 2021
Hardcover ISBN : 978-3-030-63973-0 Published: 02 February 2021
Softcover ISBN : 978-3-030-63976-1 Published: 03 February 2022
eBook ISBN : 978-3-030-63974-7 Published: 01 February 2021
Edition Number : 1
Number of Pages : XXI, 314
Number of Illustrations : 29 b/w illustrations
Topics : Business and Management, general , Entrepreneurship
- Publish with us
Policies and ethics
- Find a journal
- Track your research

IMAGES
VIDEO
COMMENTS
Abstract —the term "disruptive technology" as coined by Christensen (1997) refers to a new technology having lower cost and. performance measured b y traditional criteria, but having higher ...
From Fig. 1, we see that the research on disruptive innovation has begun to gain its popularity since 2013, with the articles published in SSCI journals beginning to reach more than 10 pieces each and every year.And the number of related articles has peaked at 45 in 2018. The developing trajectory of the published articles also indicates the upward trend of discussion and development of this ...
Disruptive technologies are related to a country's competitiveness and international status. Accurately identifying and predicting the trends in disruptive technologies through scientific methods can effectively grasp the dynamics of technological development, adjust the national science and technology strategic layout, and better seize the high ground in international competition. Based on ...
Students may leverage GPT's advanced language-generation capabilities to craft essays and research papers, bypassing the critical learning process involved in such tasks. Educators fear that this disruptive innovation might erode educational standards and foster a culture of dishonesty (Greitemeyer & Kastenmüller, Citation 2023).
This research is by far the only research record in the last decade that provides a systematic literature review of disruptive technologies with an extensive focus on customers. Considering the methodological perspective, this research uses topic modelling as a new literature review model, enabling analysing more research outputs and yielding ...
The greatest technological changes in our lives are predicted to be brought about by Artificial Intelligence (AI). Together with the Internet of Things (IoT), blockchain, and several others, AI is considered to be the most disruptive technology, and has impacted numerous sectors, such as healthcare (medicine), business, agriculture, education, and urban development. The present research aims ...
Fig. 2: Decline of disruptive science and technology. a, b, Decline in CD 5 over time, separately for papers (a, n = 24,659,076) and patents (b, n = 3,912,353). For papers, lines correspond to WoS ...
Disruptive changes, understood as changes in a company and its operating environment caused by digitalization, possibly leading to the current business becoming obsolete (Parviainen et al., 2017), trigger DT in different environments due to rapid or disruptive innovations in digital technologies.These changes create high levels of uncertainty, and industries and companies try to adapt to these ...
This paper provides a comprehensive analysis for challenges and opportunities that arise from the integration of disruptive technologies. This includes areas such as energy efficiency, governance, social profiling, tenancy and storage management, and several others (Fig. 55.3). Fig. 55.1. Disruptive technologies.
A Disruptive Research Playbook for Studying Disruptive Innovations. As researchers, we are now witnessing a fundamental change in our technologically-enabled world due to the advent and diffusion of highly disruptive technologies such as generative AI, Augmented Reality (AR) and Virtual Reality (VR). In particular, software engineering has been ...
This review paper strives to analyze published literature on disruptive technologies in diverse sectors and how they impacted the markets and major market players with focus on the satisfaction of ...
Explore the latest full-text research PDFs, articles, conference papers, preprints and more on DISRUPTIVE TECHNOLOGIES. Find methods information, sources, references or conduct a literature review ...
Information technologies (ITs) have played an increasingly important role in generating disruptive innovations. Telephones, for instance, disrupted the market for telegraphy and made the service obsolete. In a more recent example, share-riding applications, such as Uber and Didi, effectively disrupted the taxi market and, in some cities, began ...
The article provides an overview of emerging disruptive technologies. These technical innovations render existing technologies and social relations obsolete or radically altered, possibly driving harmful direct and indirect social, economic and ecological disruptions. Creating taxonomic order for technology innovations and being able to parse out their key features starts the path to ...
Summary. For the past 20 years, the theory of disruptive innovation has been enormously influential in business circles and a powerful tool for predicting which industry entrants will succeed ...
2. Literature review 'Disruptive technology' [] is a kind of new and imaginative technology, which has a disruptive effect on existing traditional or mainstream technologies.At present, there are three main methods concerning disruptive technology identification: technology roadmap and expert experience combined analysis, index evaluation analysis, and model-based analysis [].
Identifying disruptive technologies has important value for the decision-making in technology layout and investment. The identification methods of disruptive technologies based on data mining have attracted much attention recently, but most of the existing studies use single data for the identification, that may cause bias. Therefore, this paper uses multi-source data which represent the ...
New research on disruption from Harvard Business School faculty on issues including how disruptive innovation is remaking businesses, health care organizations, and universities; insights from observations by the theory's founder, Clayton Christensen; and how to use disruption to your advantage. Page 1 of 22 Results →. 21 May 2024.
The evolving field of disruptive technologies has recently gained significant interest in various industries, including agriculture. The fourth industrial revolution has reshaped the context of agricultural technology (AgriTech) with applications of artificial intelligence (AI) and a strong focus on data-driven analytical techniques. Motivated by the advances in AgriTech for agrarian ...
ENHANCING TOURISM INNOVATION THROUGH DISRUPTIVE TECHNOLOGY AND STRATEGIC ALLIANCES: EVIDENCE FROM POPULAR DESTINATIONS IN WEST JAVA The research objects focus on the following construct: 1. Disruptive technology 2. tourism innovation 3. Strategic alliances 4. Local cultural engagement This study employs a quantitative, explanatory survey design to investigate the role of disruptive technology ...
Reflective e-paper displays show promising prospects with the increasing demand for energy-efficient and sustainable technologies. However, the intrinsic incapability to display in dark environments hinders their broader application, and it is significantly important to enable reflective displays to achieve good display effects under various lighting conditions.
Artificial Intelligence as a Disruptive Technology for Digital Marketing Vladislav Rutskiy1,7, Reza Mousavi2, Nikita Chudopal1, Younes El Amrani3, Valentina Everstova4, and Roman Tsarev5,6(B) 1 Siberian Federal University, Krasnoyarsk, Russia 2 PBXDom, Walnut, USA 3 Abdelmalek Essaâdi University, Tangier, Morocco 4 North-Eastern Federal University, Yakutsk, Russia
This research unveils a transformative methodology for fabricating flexible printed circuit boards (PCBs), focusing on the unique attributes of filter paper substrates. A meticulous parametric exploration scrutinizes critical aspects such as buckling resistance, charging current, plating time, and electrode configurations for copper electroplating.
An increasing number of companies are beginning to use artificial intelligence technology in the field of marketing to overcome this problem and gain competitive advantages in the market, both in terms of advertising quality and its costs. Only a few years ago the largest companies such as Netflix, Amazon, Google started to use it [1].
Digital technologies are key to achieving competitive advantage across marketing and retailing contexts. At the same time, marketing managers are confronted with a variety of challenges surrounding the strategic use of these technologies and the need to re-think their digital strategies. Importantly, managers need to develop a deeper understanding of consumer attitudes towards and engagement ...
About this book. This book examines how new technologies have transformed global markets, as well as global business strategy. It explores how digitalization, artificial intelligence, virtual reality, and other changes in technology have led both to new opportunities but also to increased uncertainty within both business and legislature.