Click through the PLOS taxonomy to find articles in your field.
For more information about PLOS Subject Areas, click here .
Loading metrics
Open Access
Peer-reviewed
Research Article

Math and language gender stereotypes: Age and gender differences in implicit biases and explicit beliefs
Roles Conceptualization, Data curation, Formal analysis, Investigation, Writing – original draft, Writing – review & editing
* E-mail: [email protected]
Affiliation Department of Psychological and Brain Sciences, Indiana University Bloomington, Bloomington, Indiana, United States of America

Roles Conceptualization, Data curation, Formal analysis, Funding acquisition, Investigation, Methodology, Project administration, Resources, Supervision, Writing – original draft, Writing – review & editing
Affiliation Department of Psychology and Neuroscience, University of North Carolina at Chapel Hill, Chapel Hill, North Carolina, United States of America
Roles Data curation, Investigation, Methodology, Writing – review & editing
Affiliation Department of Psychological and Brain Sciences, Colgate University, Hamilton, New York, United States of America
Roles Conceptualization, Formal analysis, Funding acquisition, Investigation, Methodology, Resources, Software, Supervision, Writing – original draft, Writing – review & editing
- Heidi A. Vuletich,
- Beth Kurtz-Costes,
- Erin Cooley,
- B. Keith Payne
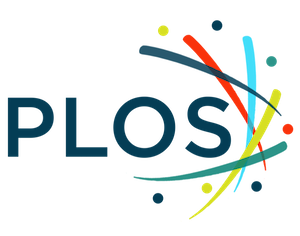
- Published: September 8, 2020
- https://doi.org/10.1371/journal.pone.0238230
- Peer Review
- Reader Comments
In a cross-sectional study of youth ages 8–15, we examined implicit and explicit gender stereotypes regarding math and language abilities. We investigated how implicit and explicit stereotypes differ across age and gender groups and whether they are consistent with cultural stereotypes. Participants ( N = 270) completed the Affect Misattribution Procedure (AMP) and a survey of explicit beliefs. Across all ages, boys showed neither math nor language implicit gender biases, whereas girls implicitly favored girls in both domains. These findings are counter to cultural stereotypes, which favor boys in math. On the explicit measure, both boys’ and girls’ primary tendency was to favor girls in math and language ability, with the exception of elementary school boys, who rated genders equally. We conclude that objective gender differences in academic success guide differences in children’s explicit reports and implicit biases.
Citation: Vuletich HA, Kurtz-Costes B, Cooley E, Payne BK (2020) Math and language gender stereotypes: Age and gender differences in implicit biases and explicit beliefs. PLoS ONE 15(9): e0238230. https://doi.org/10.1371/journal.pone.0238230
Editor: Jennifer Steele, York University, CANADA
Received: September 18, 2019; Accepted: August 12, 2020; Published: September 8, 2020
Copyright: © 2020 Vuletich et al. This is an open access article distributed under the terms of the Creative Commons Attribution License , which permits unrestricted use, distribution, and reproduction in any medium, provided the original author and source are credited.
Data Availability: All relevant data are uploaded to the Open Science Framework database and publicly accessible via the following URL: https://osf.io/fv5h8/ .
Funding: This research was supported by a grant from the National Institute of Child Health and Human Development ( https://www.nichd.nih.gov/ )#1R03HD072025-01A1 awarded to BKC and KP. The first author was supported by a National Science Foundation Graduate Fellowship and the Paul and Daisy Soros Fellowships for New Americans. The funders had no role in study design, data collection and analysis, decision to publish, or preparation of the manuscript.
Competing interests: The authors have declared that no competing interests exist.
Introduction
Children’s perceptions of gender differences in cognitive abilities are important because they may lead boys and girls to develop different interests and different areas of achievement [ 1 – 3 ]. For example, stereotypic perceptions of academic abilities have been implicated in gender differences in course selections and career trajectories, contributing to the underrepresentation of women in science, technology, engineering and math (STEM) [ 4 , 5 ]. Much of the research devoted to this topic has examined children’s self-reported explicit beliefs about gender differences in abilities. As summarized below, explicit beliefs may differ in systematic ways from implicit gender biases , which are automatically activated associations to gender categories. In this study, we examined children’s implicit biases and their explicit beliefs regarding gender differences in math and language abilities.
Math is directly relevant to students’ interest in and preparation for STEM careers, but biases favoring girls in language could also contribute to STEM disparities by disproportionately attracting girls and deterring boys from the humanities [ 6 ]. To identify if, and when, changes in explicit beliefs and implicit biases are taking place, we examined age differences in these phenomena, using a sample that ranged in age from 8 to 15 years. We also examined relations between youth’s implicit biases and their explicit beliefs.
Possible influences on children’s perceptions of gender differences in academic abilities
According to social identity theory, identifying with a social category leads to in-group preferences because doing so protects people’s self-esteem [ 7 ]. Gender is one of the first social categories to develop, and children as young as 3 to 5 years old express preferences for their own gender [ 8 , 9 ]. Same-gender preferences typically result in more generalized positive evaluations of one’s gender group, particularly among children [ 10 ]. For these reasons, one would predict that children’s explicit reports of competence in academic domains would be biased by their gender, resulting in in-group preferences. Other factors that may influence children’s perceptions of academic abilities, particularly among older children, are the need to be fair [ 11 ], the development of more accurate evaluations [ 12 ], and the need to present themselves in socially desirable ways [ 13 ].
As children age, they also become aware of cultural stereotypes that contradict their positive evaluations of the in-group. Two traditional gender stereotypes are that boys are more talented than girls are in math, whereas girls are more talented than boys in language domains [ 14 ]. Sociocultural views of knowledge construction suggest that children’s awareness of these kinds of cultural stereotypes increases with maturation [ 15 ], and empirical studies have shown support for this idea [ 16 – 18 ]. Therefore, as children grow older and have more experiences in which stereotypes are discussed or endorsed by others, these stereotypes may become a more salient source informing children’s own attitudes. In particular, when children encounter negative stereotypes about their social group, those stereotypes may either temper their in-group favoritism, yielding more egalitarian attitudes, or guide their attitudes such that they endorse the negative stereotypes about their group. Research suggests that the way children respond often depends on where their group is positioned within the larger social hierarchy [ 19 ]. Children belonging to groups of high status are more likely to endorse negative stereotypes about their in-group, presumably because they do not have a strong need to self-enhance. Children belonging to low-status groups are more resistant to endorsing negative stereotypes and either downplay them or opt for egalitarian views instead. For instance, in two studies, boys and Whites endorsed traditional gender and race stereotypes regardless of whether those stereotypes favored their in-group, but girls and Black children were more selective, endorsing stereotypes that favored their in-group while denying stereotypes that portrayed their in-group negatively [ 18 , 19 ].
Another factor influencing children and adolescents’ beliefs is their personal experience. Although in most countries worldwide men are more likely than women to pursue careers in STEM domains [ 20 ], girls tend to receive higher grades than boys throughout primary and secondary school across academic subjects [ 21 , 22 ]. Particularly as youth enter middle school, where achievement becomes more salient because of academic tracking and public posting of honor rolls, they are likely to become aware of differences in academic performance favoring girls. Indeed, using a photo-identification task in which youth were asked to pair photos with verbal descriptions of individuals, middle school youth were more likely to choose photos of girls than of boys for depictions of high-achieving youth [ 23 ].
Girls’ relative superiority over boys in academic settings is robust, as reflected by school grades, high school and college completion rates, and teachers’ ratings of school engagement [ 21 , 24 , 25 ]. Therefore, although gender differences persist in career choices, and stereotypes are still widely endorsed that favor boys in math, youths’ awareness of gender differences in school success might lead to implicit biases and/or explicit reports that are either egalitarian or that favor girls across domains. For instance, one research study with children ages 4–10 found that, with age, boys increasingly endorse personal beliefs about girls’ academic superiority and also beliefs that adults see girls as academically superior [ 26 ].
Explicit beliefs regarding gender differences in academic abilities
As outlined in the previous section, several factors potentially influence children’s perceptions of gender differences in academic abilities. Unfortunately, research studies investigating children’s beliefs through explicit reports have not demonstrated consistent findings in support of any one explanation. Regarding math ability, several studies have shown that young boys (approximately ages 6–11) show in-group preferences [ 27 , 28 ]. In two different studies, Italian boys in first grade were more likely to point to a picture of a boy, rather than a girl, when asked who is especially good at math [ 28 , 29 ]. Italian boys in third and fifth grade were also more likely to say that boys, rather than girls, were better at math [ 30 ]. In U.S., German, and French samples, boys in fourth grade rated boys as more gifted than girls in math [ 31 – 33 ]. Singaporean boys in first, third, and fifth grade were more likely to point to a picture of a boy, rather than a girl, when asked who liked math more [ 27 ]. These results are consistent with the idea that in-group preferences dominate in early and middle childhood. However, this explanation does not hold for girls. Although some of the studies just discussed reported in-group math preferences among young girls (ages 6–11) [ 28 – 30 , 32 , 33 ], others found that young girls hold egalitarian views [ 27 ] or even endorse stereotypes that boys are more able than girls in math [ 31 ]. The findings also do not uniformly support other explanations such as social status, cultural stereotypes, or actual differences in performance as factors influencing children’s beliefs. Findings with older children are even less straightforward. Some studies show older boys and girls (ages ranging from 12–15) favor their own gender, some find youth are egalitarian, and yet others find that they favor the outgroup, with no clear age trends [ 30 – 32 , 34 – 37 ].
Research results regarding boys’ and girls’ gendered beliefs about language ability are also mixed. To our knowledge, no studies have reported girls favoring boys in language domains across any age range, but a few studies have found that young girls (ages ranging from 6–11) reported no differences between boys and girls in their liking of, or competence in, language [ 27 , 38 ]. Italian first grade girls did not show a gender preference when choosing between a picture of a boy and that of a girl when selecting who was better at language [ 38 ]. Singaporean girls in first, third, and fifth grade also did not show a gender preference when rating boys’ and girls’ liking of language [ 27 ]. Boys, on the other hand, more consistently endorse the female-language stereotype, but exceptions have been found whereby boys report egalitarian beliefs or even favor boys in language. For instance, first grade Italian boys did not show a gender preference when choosing between a picture of a boy and one of a girl when selecting who was better at language [ 29 , 38 ]. In a U.S. sample, fourth grade boys were egalitarian regarding their beliefs about who was better at reading and writing [ 32 ]. Therefore, the influences of in-group preferences versus cultural stereotypes, social status, or other factors on children’s explicit beliefs about math and language abilities remain unclear.
A problematic aspect of research on academic stereotypes focused on explicit beliefs is that it relies on self-report measures, which allow participants to control their responses. Children may report beliefs that match perceived social expectations or self-presentation goals, regardless of their own beliefs. For instance, 6- to 8-year-old children who thought they were being videotaped suppressed their explicit in-group preference and outgroup prejudice, whereas those who thought the camera was off did not [ 39 ]. The mixed findings in explicit attitudes, then, might reflect variability in children’s personal beliefs, but they could also be an artifact of children’s reactance to being questioned about a socially sensitive topic.
Examining children’s implicit biases circumvents the self-presentation problems associated with explicit measures. Implicit biases are automatic associations that are measured using cognitive tests that capitalize on reaction times or priming procedures. Responses to these tests are difficult to control and thus, are often independent of intent [ 40 – 42 ]. Such associations are not necessarily indicative of explicit beliefs, and may even be inconsistent with them [ 43 ]. Although explicit measures are informative in their own right, implicit measures can provide additional information regarding the impact of socially sensitive topics, such as stereotypes, that may not be readily accessible through explicit reports. In this study, we explored both children’s explicit beliefs and their implicit biases regarding math and language ability among boys and girls. Our hypotheses regarding explicit beliefs were that in-group preferences would be evident in the youngest age group (elementary school children) regarding math ability. We did not have specific hypotheses regarding older children, as multiple factors can affect their responses on explicit tests, including societal stereotypes, social status differences, and social desirability, among others. Regarding language ability, we hypothesized that both boys and girls would favor girls because those beliefs are congruent with multiple factors, including societal stereotypes, actual performance, and social acceptability of endorsing the stereotype (i.e., the stereotype is less condemned).
Children’s implicit biases about academic abilities
The majority of studies examining children’s gender implicit biases regarding academic abilities have measured implicit bias using the Implicit Association Test (IAT). This test measures reaction times in stereotype-congruent versus stereotype-incongruent conditions, the theoretical principle being that children (and adults) respond more quickly to paired categories that are cognitively associated. More specifically, in the stereotype-congruent condition, children press one computer key if they see words associated with boy names or math concepts and another key if they see words associated with girl names or language concepts. Faster reaction times within this stereotype-congruent condition—compared to the stereotype-incongruent condition in which paired categories are boys/language and girls/math—are typically interpreted as indicating math-male implicit bias. Using this procedure, several studies have found that girls show stronger stereotypic implicit biases than boys. In a sample of Italian children in first grade, boys did not show an implicit bias whereas girls showed stereotype-consistent implicit bias [ 28 ]. Using a paper-and-pencil IAT in which participants had 30 seconds to classify words into categories, Italian girls in Grades 3, 5, and 8 showed an implicit bias, such that they categorized more words in the stereotype-congruent condition compared to the stereotype-incongruent condition [ 30 ]. Boys, on the other hand, showed stereotypic associations in eighth grade, but not in third or fifth grade. In a sample of German children in Grades 4, 7 and 9, boys did not show any stereotypic associations across any age group, whereas girls in Grades 4 and 9 showed significant stereotyping [ 31 ]. In a rare exception for this literature, girls in seventh grade did not show any bias. These are just a few examples, but other studies show similar patterns: Girls demonstrate a stereotypic implicit bias, whereas boys either demonstrate a stereotypic implicit bias or show no bias at all [ 27 , 29 , 35 , 38 , 44 , 45 ]. These findings cover age spans from 5- to 15-years-old, and use samples across multiple nations, including Canada, Chile, Italy, Singapore, and the United States. Based on the results of these IAT studies alone, one conclusion is that children (girls in particular) assimilate societal stereotypes about gender differences in math ability favoring boys from an early age [ 45 ]. This interpretation implies that research efforts and interventions ought to be focused on children’s math associations and beliefs. Yet, an outstanding question is whether these biases are truly about math rather than language, even though they are typically labeled as math-male biases.
Due to the paired nature of IAT categories, it is impossible to disambiguate whether children who show a “male-math” bias do so because they associate math with boys more strongly than they associate math with girls, or because they associate language with girls more strongly than they associate language with boys, or both. In some cases, studies have found that IAT scores predict math outcomes, but even then what is labeled as a “math outcome” is a relative difference between math and language outcomes. For instance, in a German sample of 4 th , 7 th , and 9 th graders, stereotype-consistent IAT scores were correlated positively for girls and negatively for boys with intentions to enroll in language versus math courses, grades in language versus math, and self-concepts in language versus math [ 31 ]. One experimental study did find that IAT scores were positively correlated with math performance on a test, explaining part of the relation between exposure to the math-male stereotype and math performance [ 28 ]. However, the relation to math outcomes is not always consistent, as some studies have found no relation [ 30 , 35 ].
A recent study further challenges the assumption that math associations drive previous findings. Critically, the study used an implicit bias measure that did not confound math and language bias [ 36 ]. Instead, participants pressed one key if they saw positive adjectives related to “doing good work in mathematics” and another key if they saw negative adjectives related to “doing poor work in mathematics.” In the stereotype-congruent condition, the key corresponding to doing good work in math was on the same side as a smiley face and a picture of a male doll on the screen. The key for doing poorly in math was paired with a sad face and a picture of a female doll. The pairings were reversed in the stereotype-incongruent condition. In a different set of blocks altogether, language implicit biases were assessed using an identical procedure, but the adjectives were described as being related to doing good or poor work in reading. Implicit biases were scored as the difference in reaction times between stereotype-congruent and stereotype-incongruent trials. Thus, the researchers were able to obtain implicit bias scores for math separately from those for language. Using this procedure in a sample of Canadian children in Grades 4–6, researchers found—in stark contrast to previous results using the IAT—that girls held a counter-stereotypical implicit bias favoring girls over boys in math. Boys demonstrated no math implicit bias. Language biases, on the other hand, were consistent with previous IAT findings; girls showed a stereotypical language-female bias, whereas boys demonstrated no language implicit bias.
In the only other study (to our knowledge) that used an implicit bias measure that disambiguated math and language implicit biases, girls also did not demonstrate stereotypical math-male bias [ 46 ]. In that study, German students in Grade 9 completed a go/no go association task as the measure of implicit bias. This task required participants in the stereotype-congruent condition to press the space bar if they saw words on the computer screen associated with math or boys and to ignore other words (i.e., words associated with girls and neutral stimuli). In the stereotype-incongruent condition they responded to words associated with math or girls and ignored all other words. The same procedure was applied to measure language bias. Scores were based on reaction time differences between the stereotype-congruent and stereotype-incongruent blocks. The results were that girls did not show a math-male bias, whereas boys did. On the other hand, girls showed an implicit language-female bias, whereas boys showed a counter-stereotypical bias favoring boys in language.
To summarize, all studies examining math/language implicit biases using the IAT have found that girls demonstrate stereotypical implicit biases (with the exception of one seventh grade subsample). Although these results might reflect math-male biases, language-female biases, or both biases, they have been labeled as “math-male” biases. In contrast, the only two published studies that have employed implicit bias measures that disambiguate math and language biases have not supported the notion that girls hold math-male biases. We base these statements on a literature search for peer-reviewed articles on Google Scholar and PsychINFO using different combinations of keywords: “IAT,” “implicit bias,” “implicit stereotype,” “math,” “language,” “children,” and “girls” (up-to-date as of March 2020). This pattern of findings suggests that measurement differences could explain the seemingly conflicting results. Further, when understood from the perspective of language gender biases, all the findings align.
In our study, we also used a measure that disambiguates math and language implicit biases. We tested two different predictions based on two theoretical accounts. The first was that girls would hold math-male implicit biases, consistent with the idea that they assimilate cultural stereotypes favoring boys in math (in line with the interpretation of IAT results). The alternatively hypothesis was that girls would show a math-female counter-stereotypical bias, consistent with national gender differences in overall academic performance. Because performance and stereotypes about language are consistent with each other, we expected girls to show implicit biases favoring girls in language. We were agnostic about the implicit biases of boys, as previous findings have been inconsistent and do not clearly favor one theoretical account over another. The dissociative processes by which girls and boys form automatic associations is in itself interesting, but not the subject of this report.
Affect misattribution procedure
In the current study, we used the Affect Misattribution Procedure (AMP) [ 47 ] to measure implicit biases about math and language ability among girls and boys. The AMP has several strengths for studying implicit biases [ 48 ]. First, it tests automatic associations to single domains, meaning it can assess implicit biases regarding math and language separately. Second, it has a simple structure, which is ideal for use with children. Participants follow simple instructions to make binary judgements about ambiguous stimuli across several trials. In the present study, the binary judgements were “good at math” versus “bad at math” (or language arts), and the stimuli were Chinese symbols. Each ambiguous stimulus (i.e., Chinese symbol) is preceded by a prime that participants are instructed to ignore. In the present study, the prime was a picture of a boy or a girl. The AMP measures participants’ unintended misattribution of affect or semantic content (e.g, [ 49 , 50 ]) from the prime to the ambiguous stimulus. For example, when asked to judge whether a Chinese symbol means “good at math” or “bad at math,” participants unintentionally use their judgements about the preceding prime (e.g., photo of a girl/boy) to make a response.
One potential concern about the AMP is that participants could ignore instructions and directly rate the primes, making the measure more akin to an explicit measure. To address this concern, one study tested two different AMP conditions assessing race implicit bias, one where adult participants were instructed to directly rate the primes and another one where they were instructed to ignore the primes and rate the target stimuli [ 51 ]. These two conditions yielded divergent results. The traditional AMP predicted racial bias in an impression formation task, whereas the “explicit” AMP did not, presumably because individuals were motivated to control expressions of prejudice in the explicit condition. Indeed, motivation to control prejudice was related to the explicit AMP but not the traditional one. These results suggest that, under normal conditions (i.e., when people are instructed to ignore the photo primes and evaluate the symbols), participants are not intentionally rating the photo primes. Though these findings were based on adult samples, they likely extend to children, as children tend to have less self-regulatory skills than adults to control their responses [ 52 ]. Another study addressing this concern found that adults are not able to accurately introspect on what influenced their response pattern [ 51 ], rendering self-reports of this information unreliable. Taken together, these findings suggest that any systematic influence of the photo primes on the ratings of the ambiguous stimuli reflects an unintended/automatic, and thus implicit, bias.
The potential weaknesses of the AMP have been tempered by empirical evidence, and its strengths have been documented extensively. Another strength of the AMP is its reliability. Meta-analytic procedures have shown the AMP to be more reliable than most reaction-time-based measures [ 48 ]. There is also evidence that it is a reliable measure for use with children, with good predictive validity [ 53 ].
Use of the AMP in this study allowed us to examine gender and age differences in implicit gender biases regarding math and language ability separately. Given the potential methodological specificity of previous findings regarding math implicit biases in children, it was important to select a measure that not only disambiguates biases by domain, but is also simple enough to use with children, reliable, and has proven to be valid.
Relations between implicit biases and explicit beliefs
In addition to measuring age and gender differences in reports, we also measured relations between implicit biases and explicit beliefs. Explicit reports are presumed to reflect personally endorsed attitudes, which may be shaped by motivated reasoning such as social desirability or the need to protect one’s social identity. Recent theoretical perspectives on implicit biases, in contrast, suggest that situational effects are a strong determinant of implicit biases [ 54 ]. Examples of these situational effects are cultural stereotypes cued by media or perceptible environmental inequalities, such as differences in classroom performance. Thus, for example, an adolescent who is aware of the math-male stereotype might show implicit biases favoring boys in math even if she does not personally endorse the stereotype, simply because it has been activated by something in the environment. Similarly, a child who observes differences in classroom performance might automatically associate girls with academic success, but still assert that boys are better than girls at a given subject in order to protect his gender self-esteem.
Thus, implicit and explicit measures might yield unrelated results because of motivational biases operating in explicit reports, or because implicit bias responses reflect activation of cultural knowledge or environmental cues not endorsed or acknowledged by the individual. Meta-analyses examining relations between the two have shown small, positive relations, with mean effect sizes often ranging between .20 and .24 [ 43 , 55 ]. Results of individual studies vary widely, however, with the strength of relations shaped by moderators such as conceptual correspondence between the two measures and other task characteristics. Findings from investigations focusing specifically on children’s academic biases and beliefs have also found small to no correlations. In their study of Singaporean children in first, third, and fifth grades, Cvencek et al. [ 27 ] found very low to no correlations between children’s implicit and explicit reports of gender differences in math ability. Implicit attitudes were unrelated to explicit math stereotypes in Passolunghi et al.’s [ 30 ] study of third, fifth, and eighth grade Italian children. Though these results could be indicative of a dissociation between implicit biases and explicit beliefs among children, they could also be indicative of the lack of correspondence between the IAT, which confounds math and language and was the implicit measure used in those studies, and their explicit measures, which focused on math.
Current study
The aim of this paper was to examine age and gender differences in implicit biases and explicit beliefs regarding gender differences in math and language abilities. By using the Affect Misattribution Procedure (AMP) [ 47 , 48 ] to measure implicit biases, we were able to obtain independent measures of gender biases regarding math as opposed to language abilities. We used a cross-sectional sample of youth in elementary school, middle school, and high school to test age differences.
With regard to explicit stereotypes, we expected age differences with the youngest age group most likely to favor their own gender in math. We did not have specific predictions about older children, as we could envision multiple factors influencing their beliefs, including the substantial efforts in recent decades to encourage the idea that girls can excel in math, increasing sensitivity to such social norms with age, cultural stereotypes, social status differences, and actual differences in performance. In contrast, given cultural stereotypes emphasizing girls’ success in language domains, combined with gender differences in academic performance, we expected that youth of both genders would favor girls in their explicit reports of language abilities.
With regard to youth’s implicit biases, we envisioned two potential outcomes based on two different theoretical accounts. We expected girls would show traditional math-male biases if they have assimilated cultural stereotypes that favor boys in math. In contrast, girls would favor girls in math if pervasive differences in academic performance are the primary factor shaping automatic associations about gender and math ability. We expected girls to show implicit biases favoring girls in language, regardless, as both cultural stereotypes and differences in academic performance favor girls in language domains. We were agnostic about trends for boys.
With regard to relations between implicit and explicit measures, given developmental differences across this age range and results of prior studies, we expected weak positive relations between explicit and implicit measures in each domain, with the possibility that the strength of relation might decrease with age. Whereas younger youth may be more transparent and explicitly report their automatic associations, older youth might control their responses so that their implicit biases are more dissociated from their explicit reports.
Participants
Participants were 270 youth (141 girls) ages 8 to 15. Youth were recruited from public libraries and schools in the southeastern region of the United States. A sensitivity analysis conducted in G*Power (Version 3.1.9.2) [ 56 ] indicates that this sample is sufficient to detect main effects as small as η 2 = .03 (f = .17) and interaction effects as small as η 2 = .04 (f = .19) at .80 power.
Youth ages 8–10 were grouped into an elementary school category ( n = 101, 53 girls and 48 boys, M age = 8.9, SD = 0.7). Those ages 11–13 were grouped into a middle school category ( n = 67, 25 girls and 42 boys, M age = 11.5, SD = 0.7), and youth ages 14–15 were grouped into a high school category ( n = 99, 63 girls and 36 boys, M age = 14.4, SD = 0.8). Our sample was 49.1% White, 29.9% Black, 12.0% Hispanic, 5.2% mixed race/ethnicity, 2.2% Asian, and 0.37% other (1.23% did not report their race).
All procedures were consistent with ethical standards of the American Psychological Association and were approved by the Institutional Review Board at the University of North Carolina at Chapel Hill. After parents provided informed consent, youth gave verbal and written assent to participate. Next, youth completed the Affect Misattribution Procedure (AMP), an implicit measure of academic stereotypes. A researcher read the initial instructions aloud and gave the participant intermittent reminders. The AMP is a computerized task that was administered on a laptop computer. Finally, participants completed a paper survey that included an explicit measure of academic stereotypes, along with other measures not included in the current report. If needed, a researcher assisted younger participants in reading the instructions and questions, but to prevent social desirability effects, the researcher read from a different survey, facing away from the child in order not to look at his or her responses. The research team included both men and women, African Americans, non-Hispanic Whites, and Hispanics. All stimulus materials reported here can be found in S1 File .
Children and adolescents were recruited from schools and from the community through announcements posted in public locations. Data collection was conducted in public libraries, at a local YMCA, and in four participating schools. In each of those settings, testing took place individually in a quiet and secluded area, facing away from other people.
Implicit bias.
We used the Affect Misattribution Procedure (AMP) [ 48 ] to measure implicit bias. The AMP has been validated as a measure of implicit biases in adults [ 47 ] and also in children ranging from 4 to 12 years old [ 57 – 59 ]. Each trial of the AMP began with a brief presentation (200 ms) of a photo on the computer screen. The photographs used as primes included 40 images of early adolescents—20 girls and 20 boys, balanced in race (Black and White). The photos were selected based on a pilot study to ensure that photos of the two genders did not differ on perceived attractiveness, age, or mood. Internal consistency for the measure was α = .40 (procedure outlined in [ 47 ], Experiment 1).
Following the randomly selected photograph, a black and white pattern (125 ms) and a Chinese symbol were presented (150 ms). A black and white pattern then appeared until the participant made a response. Participants were instructed to ignore the photograph and make a judgment about the meaning of the Chinese symbol. They made these judgements in two blocks of trials, one for math and one for language. Each block consisted of 40 trials. For example, in one block participants guessed whether each symbol was a word related to the ideas of “good at math” versus “bad at math.” In the next block, they guessed whether each symbol was related to being “good at language arts” versus “bad at language arts.” The keys on the keyboard were clearly labeled “good” or “bad” (in place of the “F” and “J” keys). Participants were told that each symbol was “a word from the Chinese alphabet,” and that we were interested in how people make guesses about the meaning of words. The instructions further specified that the participant should rate about half of the symbols as good at math (or language arts) and half as bad at math (or language arts). Two other school domains (sports and science) were included in separate blocks, but are not the focus of this report. Block presentation was counterbalanced across participants. We do not have information about whether any participants were familiar with the Chinese symbols in our study or on their thoughts about the cover story. However, we should note that the believability of the cover story is superfluous to the task’s objective, which was to rate ambiguous stimuli preceded by a prime. The mechanism by which the AMP functions (i.e., misattribution of affect/semantic content from prime to target) does not depend on the construal of the task. Familiarity with the Chinese symbols does present a problem, which is that the symbols would no longer be neutral, weakening priming effects. Thus, though unlikely given our sample’s demographics, we should note that the effects reported here might be underestimates.
Participants were reminded between blocks that they should ignore the photo of the person and to just focus on the symbol. The sequence of photographs was randomized within each block, and the sequence of domains (language, math) was randomized across participants.
Each participant had two implicit bias scores for each domain, one representing their implicit bias regarding girls (i.e., the proportion of times the student designated “good in math” [or language] after seeing a photo of a girl) and one representing their implicit bias regarding boys (i.e., the proportion of times the student selected “good in math” [or language] after seeing a photo of a boy). Because these scores were proportions of the total number of times they viewed stimuli of each gender, scores had the possible range of zero to 1.00, with higher scores indicating greater bias favoring the gender of the prime photograph, which we refer to as the prime gender.
Explicit beliefs.
Youth used a visual analog scale (VAS), consisting of a 100 mm horizontal line, to indicate with a vertical mark how well they thought boys or girls performed on a specific academic subject and how difficult they thought boys or girls found the subject. They could place a mark anywhere on the line, which allowed them to give very low or high ratings without having to choose the extreme option, as is the case with Likert scales. This attribute of VAS lines is important when measuring beliefs or attitudes that are sensitive to social desirability effects, such as stereotypes.
Participants answered two items regarding math and two items regarding language ability (e.g. I think that in MATH boys do this well… , and I think that boys find MATH… , with the extremes of the line labeled from “not well at all” to “very well” and from “very hard” to “very easy,” respectively). Each item was answered separately in regard to boys and girls, and each gender group was represented on a separate page. Youth rated the competence of boys and girls in other domains, both academic and non-academic, but those data are not the focus of this report. Items were scored by measuring the distance in millimeters from the left scale anchor to the line drawn by the respondent for each item. The two items corresponding to each subject and gender were averaged. Scores ranged from zero to 100, and the correlations between the two items in each measure (i.e., math and language) ranged from r = .53 to r = .61. Higher values indicate endorsement of greater competence in math/language.
All data for this study can be found in the online repository: https://osf.io/fv5h8/ . Our exclusion criteria for the implicit bias task (pressing the same key on all trials or alternating keys on all trials) did not apply to any participant.
Implicit gender bias
Tables 1 and 2 show the average proportion of prime photos that youth associated with “good at math" or “good at language,” respectively, split by prime gender and participant characteristics. To assess implicit biases regarding math and language ability in boys and girls, we conducted a 2(Participant Gender) x 3(Age Group) x 2(Academic Subject) x 2(Prime Gender) ANOVA, with Participant Gender and Age Group as between-subject factors, Academic Subject and Prime Gender as within-subjects factors, and implicit scores as the dependent variable.
- PPT PowerPoint slide
- PNG larger image
- TIFF original image
https://doi.org/10.1371/journal.pone.0238230.t001
https://doi.org/10.1371/journal.pone.0238230.t002
The main effect of Prime Gender was significant, F (1, 256) = 6.79, p = .010, η 2 = .03, and was qualified by a significant Participant Gender x Prime Gender interaction, F (1, 256) = 10.94, p = .001, η 2 = .04. Fig 1 displays that girls of all ages showed an implicit own-gender bias. Girls rated a greater proportion of symbols preceded by photos of girls as good at math and language arts compared to symbols preceded by photos of boys, and this difference was statistically significant (see Table 3 ). Boys, on the other hand, did not show implicit bias. The Participant Gender x Academic Subject x Prime Gender interaction was not significant, F (1, 256) = 0.62, p = .432, failing to provide evidence that boys and girls differed in the extent to which they showed consistent gender-domain associations. None of the other interactions were significant. These results show implicit biases as being invariant across age, with girls holding an implicit in-group bias for both math and language, and boys not associating either math or language with either gender preferentially.
Values indicate the proportion each prime gender associated with “good at math” or “good at language arts.” Bars represent standard errors.
https://doi.org/10.1371/journal.pone.0238230.g001
https://doi.org/10.1371/journal.pone.0238230.t003
An alternative interpretation of our results is that the AMP was measuring generalized gender biases (e.g., girls = good) rather than domain-specific biases (e.g., girls = good at math). To test this possibility, we examined girls’ and boys’ implicit biases regarding sports ability (one of the domains included in the study, but not the focus of this report). We report the results in our S1 File . In short, boys showed evidence of implicit bias favoring boys for the sports domain, but girls showed no implicit bias. Although these results might still reflect generalized biases (i.e., girls = good at academics / boys = good at athletics), they suggest that the AMP was sensitive to domain category and not just valance. In theory, if the instrument distinguishes between broad categories (e.g., sports versus academics), then it can distinguish between the categories specified in the actual task (sports v. math v. language), unless the effects of the specific academic subjects are so small as to be overwhelmed by the broader category. This latter point is in itself informative and contrary to the current understanding of girls’ implicit biases.
Overall, these results challenge the assumption that girls automatically associate math ability with boys rather than girls, and they suggest that measurement differences could explain ostensibly conflicting results from previous studies. Of course, more research is needed in this area to be conclusive, but the current study highlights the importance of measuring math and language implicit biases separately to better understand children’s automatic associations between gender and domain-specific abilities. Especially in light of persistent gender disparities in STEM, this issue deserves careful attention, as children’s perceptions of language domains may have implications for the interests they develop and the trajectories they pursue.
Explicit gender beliefs
Tables 4 and 5 show the average competence scores that children gave to boys and girls, split by participant characteristics. To assess gender differences in children’s explicit beliefs regarding math and language ability in boys and girls, we conducted a 2(Participant Gender) x 3(Age Group) x 2(Academic Subject) x 2(Target Gender) ANOVA, with Participant Gender and Age Group as between-subject factors, Academic Subject and Target Gender as within-subject factors, and explicit scores as the dependent variable. We found significant main effects of Academic Subject, F (1, 258) = 11.21, p = .001, η 2 = .05, Target Gender, F (1, 258) = 57.64, p < .001, η 2 = .18, and Age Group, F (2, 258) = 6.71, p = .001, η 2 = .05. These main effects were qualified by significant two-way and three-way interactions. We only describe the three-way interactions here, as they qualify the two-way interactions (see the S1 File for full results). The Age Group x Participant Gender x Target Gender interaction was significant, F (2, 258) = 6.82, p = .001, η 2 = .05. Adjusting for multiple comparisons using a Bonferroni adjustment, we found that with scores collapsed across the two academic domains, girls of all three age groups favored girls, whereas boys favored girls in middle school and high school, but not in elementary school (statistics appear in Table 6 ).
https://doi.org/10.1371/journal.pone.0238230.t004
https://doi.org/10.1371/journal.pone.0238230.t005
https://doi.org/10.1371/journal.pone.0238230.t006
The Age Group x Academic Subject x Target Gender interaction was also significant, F (2, 258) = 3.51, p = .031, η 2 = .03. Children favored girls in language across all three age groups. In contrast, in the case of math, only children in elementary school showed a bias, favoring girls over boys in math. Youth in middle school and high school did not show a gender bias in explicit reports of math ability. These results should be interpreted with caution, though, as the effect size is below the threshold calculated by our sensitivity analysis.
Though the four-way interaction was not significant, perhaps due to low power, we conducted pairwise comparisons to directly test our hypothesis that the youngest children would favor their own group in math. We report our results in the S1 File . Our hypothesis was partly supported; elementary-school girls reported girls as being better than boys at math. Elementary-school boys were neutral regarding gender differences, but they gave boys a significantly higher rating in math than elementary-school girls gave boys. Our hypothesis that children of all age groups would favor girls over boys in language ability was also partly supported; with the exception of elementary-school-aged boys (who were neutral), children favored girls over boys in language ability.
In summary, regardless of age group, girls explicitly endorsed an in-group preference in both math and language. Boys, on the other hand, explicitly favored girls over boys in math and language only later in development (i.e., middle school and high school, but not elementary school). These results are somewhat consistent with what we predicted. We hypothesized that younger children would show an in-group preference in math. Though that was not exactly the case for boys, younger boys were, on average, less prone than older boys to explicitly favor girls. Unexpectedly, older children of both genders rated girls’ ability in math and language as superior to that of boys. Their agreement on these gender differences could be due to their observations of academic performance within their classrooms and schools.
Correlations between explicit and implicit measures
Finally, we computed bivariate correlations between implicit bias and explicit stereotypes. Implicit math gender biases were not correlated with explicit math stereotypes, r (259) = -.03, 95% CI = [-.15, .09], p = .624. Neither were implicit language biases correlated with explicit language stereotypes, r (258) = .11, 95% CI = [-.01, .23], p = .076. Bivariate correlations of implicit and explicit stereotype scores for each domain, calculated separately for each participant gender and age group revealed a similar pattern after adjusting the alpha level to .008 for multiple tests. Implicit biases and explicit stereotypes were not correlated for math or language across any age group for either boys or girls (all p’ s > .008).
Correlations between pairs of implicit scores and pairs of gender group competence scores are presented in Table 7 (correlations split by participant gender are reported in the S1 Table in S1 File , but in general, they follow the same pattern). For these correlations, for implicit scores we used the proportion of items in which [girls; boys] were associated with the “good in” prompt; for explicit group competence, we used the average of the two explicit items for each gender. All explicit gender group competence ratings were positively associated. For example, youth who rated boys as highly competent in math also tended to rate boys as highly competent in language, and youth who rated boys as competent in language tended to also rate girls as capable in language, r’s = .672 and .328, respectively. In contrast, implicit scores were positively correlated only within gender.
https://doi.org/10.1371/journal.pone.0238230.t007
Because of the salience of gender identity for most children and adolescents, youths’ perceptions of gender differences in academic skills are posited to shape perceptions of the self, classroom motivation and behaviors, and long-term career goals [ 1 , 2 , 60 ]. In the current study, we examined explicit beliefs and implicit biases regarding perceptions of gender differences in math and language abilities in a cross-sectional sample of youth from elementary school, middle school, and high school. In addition to testing possible age and gender differences, we also measured correlations between implicit and explicit gender stereotypes. We found that girls showed in-group preferences in math and language across both implicit and explicit measures. These findings are consistent with gender differences in academic performance at the national level, and they contradict traditional math stereotypes. Boys, on the other hand, showed no implicit biases within any of the three age groups. Explicitly, boys in middle school and high school favored girls in math and language. Boys in elementary school reported egalitarian beliefs on the explicit measure.
The results of this study, at first glance, appear to be at odds with persistent gender disparities in STEM careers. They also contradict previous implicit bias findings that have shown math implicit biases among girls. In the next sections, we discuss potential explanations for these results and their implications.
Children and adolescents’ implicit biases regarding gender differences in abilities
An important contribution of the current study is that we used a measure of implicit gender biases in which math and language abilities were not confounded. Most prior research in this area has used the Implicit Association Task, in which the two pairings (e.g., boys-math; girls-language) are not measured independently. Of note, authors of those studies have often interpreted their results as indicating an implicit bias favoring boys in math, and have used their measures to predict math-related outcomes. However, our results suggest that girls hold implicit biases favoring girls in both math and language. In supplemental analyses, we examined whether these associations might have been an artifact of the measure’s sensitivity, which may not have been granular enough to test domain-specific associations, but only broader associations (e.g., “girls = good” rather than “girls = good at math). We examined implicit biases regarding sports ability and found a different pattern of results from academic biases. Girls were neutral whereas boys favored boys in sports ability. Although these biases may still reflect a general association between girls and good academic performance, they suggest the AMP was sensitive to domain-category and challenge prior assumptions that girls implicitly favor boys over girls in math. At a minimum, our study suggests that gender math associations do not override the positive associations that girls have about girls’ general academic performance compared to boys.’ Another interpretation is that girls’ associate girls more than boys with good math performance, in line with their explicit reports.
It is possible that a strong girls-language association—an association that may be more likely to emerge on both implicit and explicit measures (rather than just implicit) due to its relative social acceptability—may be a particularly powerful predictor of both better language outcomes and, perhaps, worse math outcomes. To our knowledge, our study is only the third to use an implicit bias measure that disambiguates math and language biases allowing us to better assess these nuanced research questions. In the two earlier studies, one study found a counter-stereotypical math-female bias among girls [ 36 ], and the other found no math bias among girls [ 46 ]. Measurement differences could account for these ostensibly conflicting findings in the literature, and future research should continue to disentangle gendered associations within different domains to clarify their relation to meaningful academic outcomes.
Using the AMP, we found that in contrast to explicit reports, which differed across age, gender, and academic domains, youths’ implicit biases differed primarily by gender of the respondent, with girls favoring girls in both domains and boys showing egalitarian responses. These responses to our implicit bias measure might reflect a combination of in-group preference as well as youths’ lived reality of gender differences in school performance. Beginning with school entry and continuing throughout primary and secondary education, girls receive better grades than boys, are rated by teachers and parents as more engaged in schoolwork, and have higher graduation rates [ 21 , 24 , 25 ]. In addition, some scholars have suggested there is a discord between traditional norms of masculinity and behaviors that promote academic success such as help-seeking and cooperation [ 61 , 62 ]. These factors have led scholars to posit that school is perceived as a feminine domain. Indeed, using an implicit measure, Heyder and Kessels [ 63 ] found that German ninth graders associated school with girls more strongly than with boys, and that boys’ tendencies to view school as feminine and to ascribe negative masculine traits to themselves were related to lower grades in German. The view of academic success as a feminine trait may have led girls in the present study to show implicit biases favoring girls in both domains, whereas for boys, those views may have been tempered by a tendency to show in-group preference, resulting in their egalitarian scores on the task.
Though, at first glance, our results might not appear consistent with gender disparities in STEM careers, they are revealing in that they support recent theoretical frameworks suggesting that girls opt out of math, not due to perceived deficit in math ability compared to boys, but due to perceived strength in language ability over math ability. For example, a large international study of 15-year-old students found that girls’ comparative advantage in reading as opposed to math can largely explain gender disparities in intentions to pursue math-related careers [ 64 ]. In that study, girls who were found to be good at math were more likely than boys to be even better at reading than at math. The gap between math and reading performance accounted completely for gender differences in math self-concept, interest in math, and attitudes towards math. Other studies have also found that intra-individual contrasts of math and language abilities predict STEM disparities. In a longitudinal study of twelfth grade students, those with high ability in both math and language (more girls than boys) were less likely to pursue STEM careers than those with high ability in math and moderate ability in language [ 6 ]. Although cultural stereotypes can still be detrimental to girls insofar as they elicit stereotype-threat effects [ 65 ] or signal lack of belonging [ 66 ], our results imply that girls hold positive associations about their gender group across both math and language ability, consistent with models that depict girls as having more choices in their pursuits, rather than being bound by real or perceived ability constraints. Research that distinguishes between math and language implicit beliefs, then, is important because it can lead to different conclusions about the type of interventions that might be effective for reducing STEM disparities.
Youths’ explicit reports of gender differences in language and math abilities
According to social identity theory [ 7 ], in-group preferences frequently emerge when individuals identify with a social category such as gender, and young children (as compared to older children and adolescents) are particularly likely to show in-group preferences [ 8 , 9 ]. We hypothesized that elementary school-aged youth would demonstrate such in-group preferences on explicit measures. On the other hand, because adolescents are more likely than younger children to be aware of conflicting information such as cultural stereotypes favoring boys in math, gender differences in school performance favoring girls, and campaigns in recent decades to promote girls’ math engagement, we did not have specific predictions about their explicit beliefs regarding math. In regard to language ability, we predicted that boys and girls would favor girls over boys because cultural stereotypes, gender differences in school performance, and the social acceptability of the language-female stereotype are all congruent. Youths’ explicit reports were generally consistent with those hypotheses: Elementary -school aged girls favored girls in both domains, whereas boys reported egalitarian beliefs regarding both language and math. Because of the consistently better average performance of girls in elementary school, these egalitarian reports of boys may represent a lack of calibration to actual achievement disparities and, thus, might be considered as a type of in-group preference. In contrast, for the two older age groups, both boys and girls reported explicit stereotypes favoring girls in math and language abilities.
These explicit reports of adolescents reflect to some extent youth’s performance on U.S. national standardized tests. On the National Assessment of Educational Progress (NAEP) exams, girls outperform boys in reading at every grade level, and gender differences in math disappeared in 1996 [ 67 ]. Adolescents’ beliefs about girls’ superiority in math ability might reflect both cultural impetus to encourage girls to take math courses and women to consider math-related careers as well as historical change in the number of women pursuing math, engineering, and computer science degrees. However, although the number of women in math-related fields has increased substantially in the last generation, some gender differences persist. In high school, boys are more likely than girls to take Advanced Placement exams in BC Calculus and Physics [ 68 ]. Although women are equally as likely as men to major in math in college, in 2017 approximately twice as many doctoral degrees were awarded to men as to women in the physical and earth sciences, mathematics, and computer sciences, and almost three times as many in engineering [ 69 ]. Our results are consistent with the view that although we have not yet reached gender parity in math-intensive fields, the historical landscape of gender differences in math may be changing.
Relations between implicit and explicit stereotypes
Important contributions of this study were the age comparisons and also the examination of relations between implicit biases and explicit beliefs. Though we had expected to find weak positive relations, especially for elementary-school youth, we did not find any significant correlations between implicit biases and explicit beliefs across any age or gender groups.
These results differ from the meta-analytic findings regarding implicit-explicit correlations in the adult literature [ 43 ]. Hofmann and colleagues [ 43 ] found that, although general implicit-explicit representations are associated about 0.24 on average, the correlation is lower for stereotypes. They also found that, in adults, a critical moderator of implicit-explicit correlations is the spontaneity of explicit reports. When people relied more on “gut” reactions to report their beliefs, there was a greater congruence between implicit and explicit scores. The lack of significant correlations between math and language implicit gender biases and explicit gender stereotypes in our sample suggests that children as young as 8 years old are not reporting their “gut reactions” or automatic associations to explicit questions about gender differences. Rather, they are controlling their explicit responses. It is also possible that the lack of correspondence in our study might be linked to our choice of measures, and that a different methodological approach might yield significant relations between children’s implicit associations and their explicit reports.
Limitations and recommendations for future research
A significant limitation of the current study was that we had insufficient sample size to explore our research questions within racial/ethnic subgroups. Prior research has shown that gender stereotypes about academic abilities and students’ responses to stereotype threat vary according to racial/ethnic identity [ 18 , 70 , 71 ]. However, we were unable to test whether explicit and implicit beliefs differed across groups due to the lack of racial/ethnic diversity within our sample. Because of the cultural specificity of many gender stereotypes, including academic stereotypes [ 72 ], research using either a sample that is homogeneous or samples in which racial, ethnic, or national groups are compared might further advance our understanding of children and adolescents’ academic stereotypes.
A second limitation of the study is that we did not evaluate the personal relevance of the math and verbal domains, and therefore an important caveat to our conclusions is that it is unclear to what extent responses indicate a personal connection to the academic domain versus perceptions of gender differences in ability. For example, although several studies have found that young children tend to show own-gender preferences in competence reports, Cvencek et al. [ 45 ] found that as early as second grade, boys showed stronger “me-math” associations than girls. Our measures involved judgements of the gender group in general rather than the individual child’s connection to that domain. Girls’ implicit bias favoring girls in math in the current study in spite of gender differences at the national level in selections of high school math course-taking would indicate that the implicit scores are a stronger reflection of perceptions of gender-group competence rather than individual identification with the domain. Future research should examine the degree to which gender group competence associations differ from gender differences in individual identification, and age differences in those effects.
A third limitation of the study is that we assessed students’ implicit associations and explicit beliefs regarding gender differences in the abilities of youth targets, but not adults. Some studies have shown that children apply cultural academic stereotypes to adults more readily than they do to children [e.g., 33]. Although the explicit reports of adolescents in our sample favored girls in math, youth may have favored men over women had we used adult targets. Such results would reflect gender differences in career choices that favor men in STEM domains and would also be consistent with stereotype threat effects that show performance decrements for women when gender identity is made salient in test situations.
Finally, we note that we did not ask participants whether they were familiar with the Chinese symbols used as neutral stimuli. Although familiarity was unlikely in our sample due to the demographics and location, the effects reported here may be underestimates due to weakened priming effects.
Despite these limitations, this study advances our understanding of youth’s implicit biases and explicit beliefs regarding gender differences in academic abilities. We found no correlations between implicit biases and explicit reports, suggesting that youth as young as 8 years old are controlling their responses on explicit measures instead of reporting their automatic associations. Analyses of youth’s explicit reports suggested that youth are using information about academic performance and gender stereotype knowledge to adjust their responses, especially as they age. A major goal of our study was to analyze youth’s implicit reports across several age groups. Youth’s implicit biases were consistent with national gender differences in academic performance, especially for girls. These findings suggest that girls across a wide age range automatically associate good math performance with girls, rather than boys. A key takeaway of both our explicit and implicit findings is that girls strongly favor their in-group in both math and language. These beliefs and positive associations regarding girls’ language abilities may, in part, contribute to STEM disparities by giving girls more choices than boys, who perceive their gender group as less-qualified in non-STEM domains.
Supporting information
https://doi.org/10.1371/journal.pone.0238230.s001
- View Article
- Google Scholar
- PubMed/NCBI
- 15. Ruble D, Martin CL, Berenbaum SA. Gender development. 6th ed. In: Eisenberg N, Damon W, Lerner RM, editors. Handbook of child psychology: Social, emotional, and personality development. 6th ed. New Jersey: Wiley; 2006. pp. 864–911.
- 42. Petty RE, Fazio RH, Brinol P. The new implicit measures: An overview. In: Petty RE, Fazio RH, Brinol P, editors. Attitudes: Insihgts from the New Implicit Measures. New York: Psychology; 2008. pp. 3–19.
- 52. Geldhof GJ, Fenn ML, Finders JK. A self-determination perspective on self-regulation across the life span. In: Wehmeyer ML, Shogren K, Little T, Lopez S, editors. Development of self-determination through the life-course. Dordrecht: Springer; 2017. pp. 221–235.
- 67. Coley RJ. Differences in the gender gap: Comparisons across racial/ethnic groups in education and work. In: Educational Testing Service [Internet]. 2001. Available: https://www.ets.org/Media/Research/pdf/PICGENDER.pdf
- 68. College Board. AP data: AP report to the nation 2014. 2019 [cited 29 Jan 2019]. Available: https://research.collegeboard.org/programs/ap/data/nation/2014
Thank you for visiting nature.com. You are using a browser version with limited support for CSS. To obtain the best experience, we recommend you use a more up to date browser (or turn off compatibility mode in Internet Explorer). In the meantime, to ensure continued support, we are displaying the site without styles and JavaScript.
- View all journals
- My Account Login
- Explore content
- About the journal
- Publish with us
- Sign up for alerts
- Open access
- Published: 06 July 2018
No intrinsic gender differences in children’s earliest numerical abilities
- Alyssa J. Kersey ORCID: orcid.org/0000-0001-6685-5982 1 , 2 ,
- Emily J. Braham 3 , 4 ,
- Kelsey D. Csumitta 1 ,
- Melissa E. Libertus ORCID: orcid.org/0000-0002-6461-1156 3 , 4 &
- Jessica F. Cantlon 1 , 2
npj Science of Learning volume 3 , Article number: 12 ( 2018 ) Cite this article
54k Accesses
46 Citations
2751 Altmetric
Metrics details
- Human behaviour
Recent public discussions have suggested that the under-representation of women in science and mathematics careers can be traced back to intrinsic differences in aptitude. However, true gender differences are difficult to assess because sociocultural influences enter at an early point in childhood. If these claims of intrinsic differences are true, then gender differences in quantitative and mathematical abilities should emerge early in human development. We examined cross-sectional gender differences in mathematical cognition from over 500 children aged 6 months to 8 years by compiling data from five published studies with unpublished data from longitudinal records. We targeted three key milestones of numerical development: numerosity perception, culturally trained counting, and formal and informal elementary mathematics concepts. In addition to testing for statistical differences between boys’ and girls’ mean performance and variability, we also tested for statistical equivalence between boys’ and girls’ performance. Across all stages of numerical development, analyses consistently revealed that boys and girls do not differ in early quantitative and mathematical ability. These findings indicate that boys and girls are equally equipped to reason about mathematics during early childhood.
Similar content being viewed by others
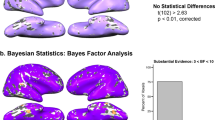
Gender similarities in the brain during mathematics development
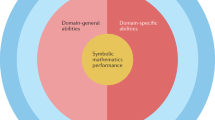
Environmental influences on mathematics performance in early childhood
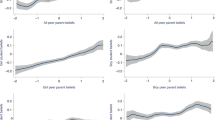
Gendered beliefs about mathematics ability transmit across generations through children’s peers
Introduction.
Adult gender differences in science, technology, engineering, and math (STEM) career representation sometimes are thought to originate from inborn differences between the sexes in aptitude for STEM fields. 1 , 2 , 3 , 4 , 5 Gender differences could be biological differences that are present at birth, or they might emerge over time with maturation. 4 In this study, we focus on gender differences in early childhood. Although adult STEM talent is derived from a large suite of cognitive abilities and unlikely to be traceable to a single domain or skill, if intrinsic differences between the sexes are indeed a root cause for the under-representation of women in STEM, one expectation is that gender differences in quantitative cognition will emerge early in human development.
Understanding the nature of gender differences in mathematics has been a focus of research for many years. However, differences in measurements, analyses, and participant samples have led to a variety of findings. For one, differences can emerge in mean performance on mathematical tasks, 6 , 7 , 8 and small differences in favor of boys have been reported in a range of numerical skills by the end of kindergarten. 9 Although most studies of school-aged children that find gender differences report higher performance in boys, some studies have only found advantages for boys when tasks involve more reasoning or are more spatial in nature. 2 , 10 In contrast, elementary school girls sometimes show an advantage on computational tasks and when performance is assessed using school grades. 11 Other studies find no differences, trivial differences, or differences in older children, but not younger children. 10 , 12 , 13 , 14 Group differences can sometimes be attributed to cohort effects. For instance, some studies show that differences between US and Chinese children in mathematics depend on generation or school, 15 , 16 and a recent study showed that the strength of any advantage in mathematics for boys vs. girls varies by country. 17 Gender differences may also emerge in the variability of mathematical performance across boys and girls. When these gender differences in cognition are observed, boys tend to show greater variability than girls, resulting in more boys than girls at the high-performing and low-performing ends of distributions. 6 , 7 , 8 , 17 , 18 This may cause gender differences in mean performance to be absent at the group level 12 , 14 but detectable at the high-performing and low-performing ends of the distributions. 18
Another major obstacle in assessing such gender differences on school-based mathematics metrics is that sociocultural influences, such as stereotype threat and the influence of teachers and parents, make it difficult to tease apart gender differences in experience from differences in intrinsic abilities. 19 , 20 , 21 , 22 , 23 , 24 , 25 , 26 , 27 , 28 , 29 For example, school-aged children could show gender differences in mathematics abilities because girls are given less or different exposure to mathematics than boys or are told that “math is not for girls.” Therefore, it is unclear whether differences in mathematics abilities are rooted in intrinsic differences in numerical reasoning in early childhood or whether gender differences emerge as a result of differences in cultural exposure to mathematical concepts. Understanding the sources of any gender differences is crucial for optimizing early childhood math and science curricula.
Previous research 30 described evidence against the existence of gender differences in visuospatial reasoning in early childhood. Across six tasks, boys and girls performed similarly on measures of object tracking (the ability to follow multiple, independent moving objects), early numerical processing, and core geometric abilities (Fig. 1 ). Those data revealed no gender differences in some basic cognitive abilities of children aged 3–10 years. However, that research leaves open key areas for investigating gender differences in core numerical processing, including patterns of looking at quantitative information during infancy, early discrimination acuity during quantity processing, and formal mathematics learning.
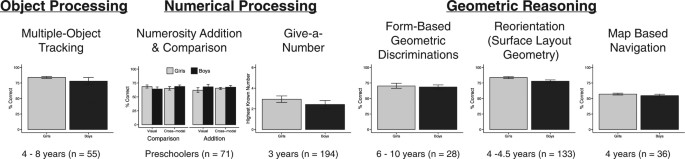
Previously described gender similarities. Redrawn data 30 showing no gender differences in early childhood on measures of object processing, numerical processing, and geometric reasoning
We examined children’s early mathematical cognition during infancy and early childhood to provide insight into whether gender differences are evident in early childhood. With the exception of the infant data, these data were collected as part of standard testing batteries measuring numerical processing skills. While we acknowledge that there are other ways to measure mathematical thinking in this age range, we combined published data 31 , 32 , 33 , 34 , 35 with unpublished data from our longitudinal records that measured children’s performance in three key areas of numerical processing from our standard testing battery of early childhood numerical cognition. First, we assessed numerosity perception and acuity in infancy and childhood. Numerosity perception allows us to estimate the quantity of a set without knowing exactly how many items are in the set—we measured children’s acuity to detect differences in numerosity. Next, we examined two aspects of verbal counting acquisition during preschool, which is the earliest emerging exact understanding of quantities. Finally, we evaluated school-based mathematics during the first few years of schooling when children learn to manipulate numbers. School-based mathematics refers to comprehensive, standardized testing of a variety of mathematical skills including counting proficiency, numeral knowledge, concrete set comparison and transformation, word problems with numerical comparisons and basic arithmetic transformations, and part-whole concepts. Because the tests are age-based, the tasks completed by each child varied. These data are largely unpublished but were combined with published data 31 , 32 , 33 , 34 , 35 in order to examine gender differences in over 500 children.
We conducted several analyses to test for statistical differences and statistical equivalence in performance, the emergence or disappearance of differences with age, and statistical differences in variability between groups. Similarities and differences between boys’ and girls’ performance were assessed using independent-samples t tests to identify statistical differences in mean performance and Schuirmann’s two one-sided tests of equivalence 36 to identify statistical equivalence in mean performance (similarity within ½ standard deviation (s.d.) of the group data; implementation of this test for SAT-Math scores. 37 ) Testing for both statistical differences and statistical equivalence is important. Non-significant t tests only allow us to conclude that there is not enough evidence to reject the assumption that performance is equivalent between groups. However, this does not necessarily mean that the groups are statistically equivalent. By including tests of equivalence, we can determine whether the lack of a significant difference between groups reflects statistically equivalent distributions of scores between groups. To date, tests of equivalence have not been conducted on data on mathematical abilities in early childhood, but these tests are especially important for informing the “Gender Similarities Hypothesis.” 38 , 39 To determine whether the results of the t test were consistent across age, we also conducted simultaneous linear regressions with age, gender, and their interaction entered as predictors. A main effect of gender would suggest that there is a difference between boys and girls when controlling for age and an interaction would suggest that differences may emerge only at one end of the age range. In addition to assessing children’s mean performance, we determined whether boys and girls showed equal variance in performance using Levene’s test. Testing for equality of variance is particularly important in light of previous work that suggests that there are more high-performing and low-performing males than females because males show greater variability in measures of quantitative processing. 4 For thoroughness, tests of statistical equivalence and differences in variability on scores controlled for age are reported in Supplement 1 (statistical differences in age-controlled scores should be evident in the regression analyses). Finally, for visualization purposes, we calculated growth curves at the group level following previous work. 40 Because these curves were calculated at the group level, we do not statistically test for differences between boys’ and girls’ growth rates and simply provide these curves as a way to visualize changes in performance with age.
Across all three aspects of early mathematical cognition assessed here, we would expect that if boys and girls truly differ in their capacities for numerical processing, we should find evidence of statistical differences in mean performance (independent-samples t tests), and we should see that this effect is consistent across age (main effect of gender in the linear regressions) or driven by one end of the age range (interaction between gender and age in the linear regression). However, the cross-sectional analysis indicates that there are no robust gender differences in early numerical processing including preverbal numerosity perception, counting acquisition, and school-based mathematics ability.
Core numerosity perception
Humans have the ability to perceptually estimate the numerical magnitude of a set of objects without counting. For example, without counting, people can rapidly determine that a set of 20 objects is numerically greater than a set of 10. Because numerosity representations are only noisy estimations of number, discrimination between quantities depends on the numerical ratio of the sets based on Weber’s law. 41 For example, using estimation it is equally easy for people to choose the larger quantity of 10 vs. 5 as 20 vs. 10, because they have the same ratio (2:1 ratio)—quantities with finer ratios like 7 vs. 5 or 15 vs. 10 will be more difficult to discriminate. Research has shown that this ability to represent and discriminate numerosities emerges within the first year of life 42 , 43 , 44 , 45 and that it is evident in nonhuman animals, 46 , 47 , 48 , 49 , 50 , 51 suggesting an evolutionarily primitive origin. At 6 months, human infants can discriminate quantities that differ by a ratio of 2:1 (e.g., 16 vs. 8 dots), 44 , 45 but by 9 months, infants can discriminate quantities at a 3:2 ratio. 45 Numerosity representations become more refined with age such that 4-year-old children can discriminate at a 4:3 ratio and adults can discriminate at a ratio of 10:9. 52 The visuospatial nature of numerosity perception makes it an important ability to investigate in children because gender differences in mathematics have sometimes been attributed to fundamental visuospatial skills, such as mental rotation. 53 Moreover, because the acuity of these representations has been shown to relate to math ability 54 , 55 , 56 (but note opposing views 57 ), understanding whether there are gender differences in early numerical processing is essential to understanding the fundamental nature of gender differences in math achievement. Here we examined data from infants, preschool children, and early school-aged children.
To test for gender differences in numerosity representations in infancy, we analyzed previously published data from 80 6-month-old infants 35 (range = 5 months 13 days–6 months 17 days, 38 girls, 42 boys). The precision of infants’ numerosity representations was assessed using a preferential looking paradigm in which infants were presented with two image streams: one in which numerosities alternated between images and one in which numerosity was constant (see Fig. 2a ). Infants preferred to look to the numerically alternating image stream if numerosities differed by at least a 2:1 ratio, and there were individual differences in infants’ preferences. 35 An independent-samples t test and Schuirmann’s test of equivalence revealed no gender differences ( t test: t (78) = 0.14, p = 0.89, difference = 0.41%, 95% confidence interval (CI) = −6 to 7; equivalence test: t 1 (78) = 2.36, p = 0.01; t 2 (78) = −2.08, p = 0.026), with boys showing a mean preference for the numerically alternating image stream of 7.5% (±14.6) and girls showing a mean preference of 7.09% (±14.3). Levene’s test of Equality of Variances revealed no significant differences in variance between girls and boys ( F (1, 78) < 0.01, p = .94, boys’ s.d. = 14.65, girls’ s.d. = 14.27). This is consistent with the previous work showing no overall differences between boys’ and girls’ sensitivity to numerosity in infancy. 58 , 59 , 60
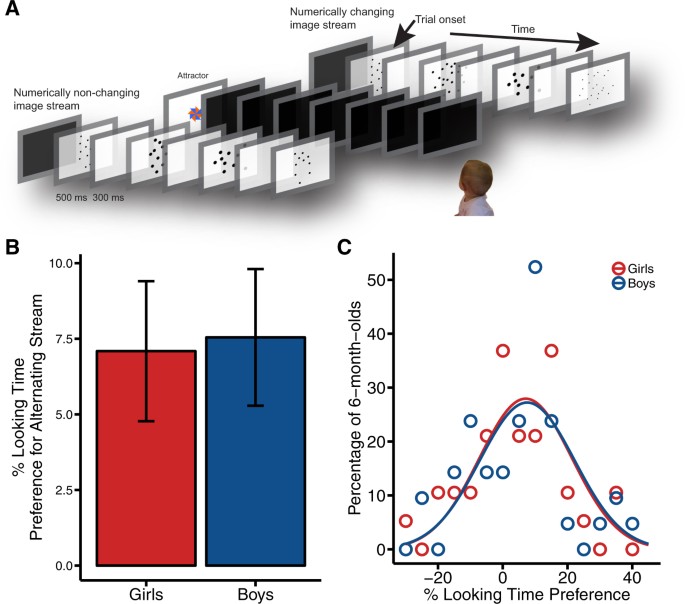
Infant numerosity. a Depiction of numerosity change detection task. b Average percentage of time looking at the numerically changing image stream for girls (red) and boys (blue). Error bars represent standard error of the mean. c Density distributions for percentage of girls (red) and boys (blue) at a given % looking time preference
We also tested for gender differences in numerosity perception in the earliest years of formal education. Two hundred forty-one scores were collected from 3- to 7-year-old children (mean age = 5.48 years, 125 girls, 116 boys; data from 68 children have been previously reported 31 , 32 ). All children completed a computerized numerical comparison task. In this task, children were shown two side-by-side dot arrays and were asked to choose the side that had more dots. The numerical ratio between dot arrays varied between 4:1 and 10:9. This type of numerical discrimination task permits a psychophysical evaluation of numerosity representation and is consistent with previous literature using this task in adults and children. 52 , 61 , 62 , 63 , 64 , 65 Furthermore, performance on this type of task has been shown to be similar to neural measures of numerosity encoding, 31 , 63 indicating that this is a fundamental aspect of numerical cognition. Although previous work found that women and girls performed better than men and boys, 52 sample sizes were small ( n = 16 per age group), so it is unclear whether these differences are representative of the general population.
To assess the acuity of boys’ and girls’ numerosity representations, Weber fractions ( w ) were calculated for each child. 66 The w score represents the acuity of numerosity representations such that a smaller w indicates greater acuity. An independent-samples t test and Schuirmann’s equivalence test revealed that boys and girls showed equal acuity of numerosity representations in early childhood (Fig. 3 ; t test: t (239) = 0.23, p = 0.82, boys’ mean = 0.56, girls’ mean = 0.58, difference = 0.02, 95% CI = −0.12 to 0.15; equivalence test: t 1 (239) = 4.10, p = 0.00003; t 2 (239) = −3.64, p = 0.0002). A simultaneous regression further revealed that while acuity improves with age, the effect of age on acuity of numerosity representations does not differ between boys and girls ( F (3, 237) = 32.03, p < 0.0001, R 2 = 0.29; Gender: b = 0.10, t (237) = 0.33, p = 0.74; Age: b = −0.27, t (237) = −7.33, p < 0.0001; Age × Gender: b = 0.02, t (237) = 0.30, p = 0.77). Finally, Levene’s test of Equality of Variances did not reveal a difference in variance between boys and girls ( F (1, 239) = 0.09, p = 0.76; boys’ s.d. = 0.57, girls’ s.d. = 0.49).
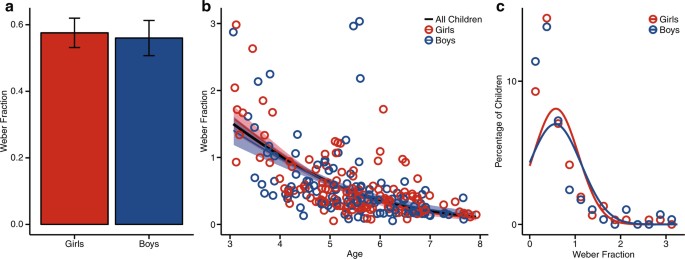
Early childhood numerosity. a Average Weber fraction for girls (red) and boys (blue). Error bars represent standard error of the mean. b Growth curves for Weber fractions calculated across girls (red), boys (blue), and all children (black). Lightly shaded areas around girls’ and boys’ growth curves indicate 1 standard deviation above and below the mean growth curve. c Density distributions for percentage of boys (blue) and girls (red) at a given Weber fraction
Taken together, we find that from infancy into early childhood, boys and girls do not differ in their earliest numerosity perceptions. Boys and girls are equally capable of discriminating numerosities.
Culturally trained counting
Verbal counting is the first culturally trained symbolic mathematics concept to develop in children. Knowledge of the verbal counting routine emerges gradually between the ages of 2 and 5 years. First, children learn to rote recite the count list (2–2.5 years). Over the next 6 to 12 months, children begin to acquire the meanings of the number words one at a time: they learn that the number word “one” corresponds to exactly one item, then that the word “two” corresponds to exactly two items, then “three,” and finally “four.” Around 3.5 years, children seemingly suddenly become cardinal-principle knowers in that they learn that each number word refers to a specific quantity and that a number word can be used to label the size of a set as determined by counting. 67 , 68 , 69 , 70 , 71 We tested children’s knowledge of the rote-memorized counting sequence with the “How High?” task, and we tested their cardinal knowledge of number and counting principle knowledge with the “Give-N” task. 69 , 70 Although there are other ways to assess counting skills and knowledge of the cardinal principles, 9 , 69 , 70 , 71 , 72 these tasks are commonly used and standardized across the literature. These two measures of culturally trained counting allowed us to determine whether boys or girls show a general advantage for early number word learning or whether there are different patterns of gender differences in memorizing the counting sequence (“How High” task) vs. learning the meanings of number words (“Give-N” task). A general advantage for early number word learning would be supported by differences in favor of one gender on both measures of early number word knowledge. An advantage on only one test would suggest the advantage is isolated to a specific skill.
For the “How High?” task, children were asked to count as high as they could until they reached 100. One hundred forty-three children aged 2–5.5 years old were tested (mean age = 4.10 years, 71 girls, 72 boys). An independent-samples t test revealed that boys and girls did not show a difference in their ability to memorize the verbal counting sequence ( t (141) = 1.48, p = 0.14, boys’ mean = 30 girls mean = 23, difference = 7, 95% CI = −2 to 16), and Schuirmann’s tests of equivalence found marginal statistical equivalence ( t 1 (141) = 4.48, p = 0.00001; t 2 (141) = −1.52, p = 0.06). A simultaneous regression confirmed that although children’s ability to recite the count list improves across age, differences do not emerge when controlling for age or at one end of the age range (Fig. 4a for scatterplot of data by age; F (3, 139) = 20.96, p < 0.0001, R 2 = 0.31; Gender: b = 12.75, t (139) = 0.58, p = 0.56; Age: b = 18.26, t (139) = 5.10, p < 0.0001; Age × Gender: b = 4.20, t (139) = 0.80, p = 0.43). Furthermore, Levene’s test of Equality of Variances revealed no difference in variability ( F (1, 141) = 1.41, p = 0.24; boys’ s.d. = 30, girls’ s.d. = 25). Taken together, this suggests that from 2 to 5.5 years of age, boys and girls show equal proficiency in memorizing and reciting the count list.
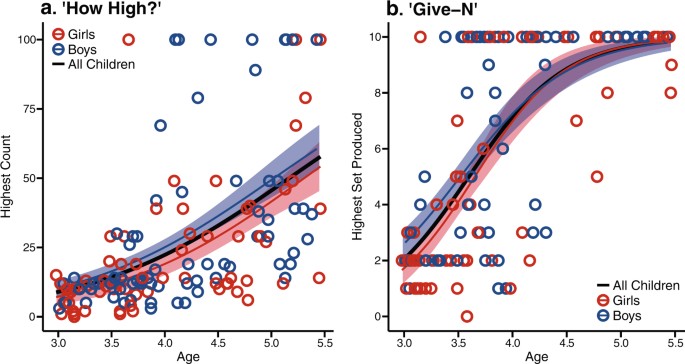
Early childhood counting. Growth curves for performance on the a “How High?” task and b “Give-N” task. Growth curves are calculated across girls (red), boys (blue), and all children (black). Lightly shaded areas around boys’ and girls’ growth curves indicate 1 standard deviation above and below the mean growth curve
Performance on the “How High?” task only represents verbal learning of the culturally trained, rote-memorized list of count terms and is not an index of children’s quantitative or logical reasoning during counting. To test children’s understanding of the counting procedure, we tested children on the “Give-N” task. In the “Give-N” task, 69 , 70 children were asked to count in order to produce sets of 1 to 10 objects. One hundred and twenty-three children aged 2.98–5.47 years completed the tasks (mean age = 3.87 years, 65 girls, 58 boys). Children were scored by the highest set size that they could correctly produce. An independent-samples t test revealed no statistical difference between boys and girls, but Schuirmann’s tests of equivalence test failed to find statistical equivalence ( t test: t (121) = 1.67, p = 0.097, boys’ mean = 6.38, girls’ mean = 5.26, difference = 1.12, 95% CI = −0.2 to 2.44; equivalence tests: t 1 (121) = 4.46, p = 0.00001; t 2 (121) = −1.12, p = 0.13). The simultaneous regression revealed a main effect of age, but no effect of gender or interaction between gender and age (Fig. 4b for scatterplot of data by age. F (3, 119) = 31.63, p < 0.0001, R 2 = 0.44; Gender: b = 1.67, t (119) = 0.57, p = 0.57; Age: b = 3.58, t (119) = 7.45, p < 0.0001; Age × Gender: b = 0.23, t (119) = 0.30, p = 0.76). In addition, we did not detect differences in variance between boys and girls ( F (1, 121) = 0, p = 0.99; boys’ s.d. = 3.61, girls’ s.d. = 3.78). Overall, there are no strong differences between boys and girls in their ability to use counting to produce sets.
Thus, boys and girls do not significantly differ in their cardinal and logical knowledge of the counting sequence during early childhood. The lack of a difference between boys and girls is consistent with the findings depicted in Fig. 1 that tested 194 3-year-old children on similar counting tasks. 30
In sum, we find that boys and girls show equal proficiency in memorizing and reciting the count list, and comparable abilities to learn the logic of the counting sequence. We conclude that there is no true gender difference in children’s early counting.
Formal and informal early elementary mathematics
Children begin to learn school-based numerical and mathematical concepts shortly after acquiring the counting principles. To test for early gender differences in the foundations of school-based mathematical concepts, we administered the Test of Early Mathematics Ability Third Edition (TEMA-3 73 ) to 275 children aged 3.07–7.92 years (mean age = 5.45 years, 133 boys, 142 girls; data from 77 children have been previously reported 32 , 33 , 34 ). The TEMA-3 is a comprehensive test of school-based mathematical knowledge for children aged 3–9 years. Items are categorized as “formal” and “informal”: Formal items tap into knowledge that is formally taught such as numeral names, numeral writing, and arithmetic facts. Informal items tap into children’s abilities to count and reason about quantitative relations and transformations that draw on acquired knowledge but are not explicitly trained or memorized. Although some test items overlap with the skills measured in the previous section on verbal counting acquisition, the TEMA-3 represents math achievement at a broader level. Importantly, the achievement scores that result from the TEMA-3 reflect knowledge on a wide range of mathematical skills including, but not limited to, counting ability. We compared boys’ and girls’ performance on the TEMA-3 overall and on items tapping into formal vs. informal math achievement separately.
Boys and girls did not differ in overall math achievement, suggesting that children show equal understanding of math concepts in early childhood (Fig. 5 ; t test: t (273) = 1.11, p = 0.27, boys’ mean = 32.32, girls’ mean = 30.04, difference = 2.28, 95% CI = −1.76 to 6.31; equivalence test: t 1 (273) = 5.25, p < 0.001; t 2 (212) = −3.04, p = 0.001; test of equality of variances: F (1, 273) = 0.002, p = 0.99; boys’ s.d. = 16.96 girls’ s.d. 17.02). This pattern was consistent across age suggesting that during early childhood boys and girls show equal competency for math concepts (regression: F (3, 271) = 224.3, p < 0.00001, R 2 = 0.71; Gender: b = 3.81, t (271) = 0.70, p = 0.49; Age: b = 12.72, t (271) = 19.00, p < 0.0001; Gender × Age: b = 0.19, t (271) = 0.19, p = 0.85).
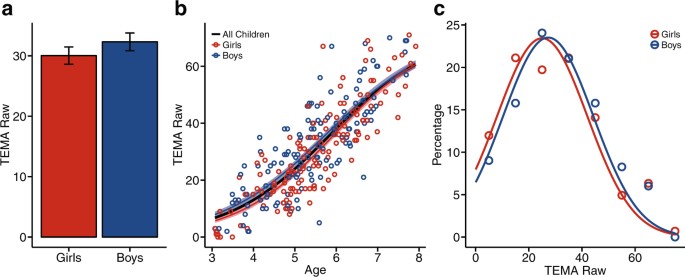
Early childhood mathematics. a Average raw TEMA score for girls (red) and boys (blue). Error bars represent standard error of the mean. b Growth curves for performance on TEMA calculated across girls (red), boys (blue), and all children (black). Lightly shaded areas around boys’ and girls’ growth curves indicate 1 standard deviation above and below the mean growth curve. c Density distributions for percentage of girls (red) and boys (blue) at a given raw TEMA score
To look at differences in boys’ and girls’ performance by question type, we compared formal vs. informal math scores. We conducted a 2 (Formal/Informal) × 2 (Boys/Girls) repeated-measures analysis of variance (ANOVA) on a subset of the data from children who answered at least four formal questions and at least four informal questions (for a similar approach 74 ). We found no interaction between gender and question type nor did we find a main effect of gender (Fig. 6 ; Gender: F (1, 207) = 0.56, p = 0.46; Question Type: F (1, 207) = 235.98, p < 0.0001; Gender × Question Type: F (1, 207) = 0.30, p = 0.58). Furthermore, we found statistical equivalence between boys’ scores and girls’ scores and no differences in variances for both formal and informal questions (Formal Questions: equivalence tests: t 1 (207) = 3.77, p = 0.0001; t 2 (107) = −3.44, p = 0.0003, boys’ mean = 0.46, girls’ mean = 0.46; variance test: F (1, 207) = 0.24, p = 0.63, boys’ s.d. = 0.17, girls’ s.d. = 0.17; Informal Questions: equivalence tests: t 1 (207) = 4.57, p < 0.00001; t 2 (207) = −2.65, p = 0.004, boys’ mean = 0.71, girls’ mean = 0.69; variance test: F (1, 207) = 0.32, p = 0.57; boys’ s.d. = 0.15, girls’ s.d. = 0.16). In addition, differences did not emerge for either question type when controlling for age or testing for interactions between gender and age (Formal Questions: F (3, 205) = 8.25, p = 0.00003, R 2 = 0.12; Gender: b = 0.05, t (205) = 0.14, p = 0.74; Age: b = 0.06, t (205) = 3.70, p = 0.0003; Gender × Age: b = −0.01, t (205) = −0.25, p = 0.81; Informal Questions: F (3, 205) = 19.62, p < 0.0001, R 2 = 0.22; Gender: b = 0.16, t (205) = 1.29, p = 0.20; Age: b = 0.09, t (205) = 6.09, p < 0.00001; Gender × Age: b = −0.02, t (205) = −1.05, p = 0.29).
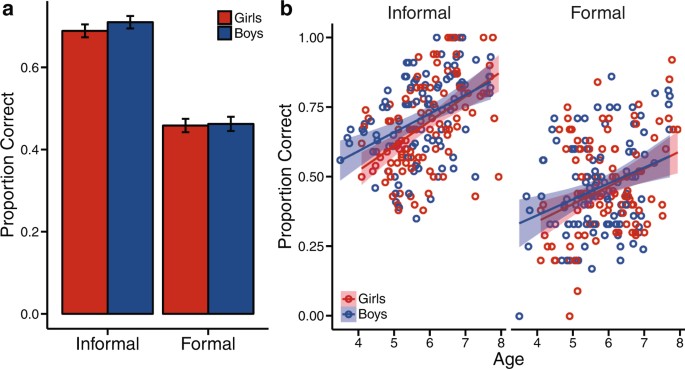
Early childhood formal and informal mathematics. a Average proportion correct (mean score) for administered TEMA informal and formal questions for girls (red) and boys (blue). Error bars represent standard error of the mean. b Proportion correct (mean score) for administered Informal and Formal Questions plotted by age. Lightly shaded areas around the regression lines indicate the 95% confidence interval
In sum, we did not find any robust performance differences in early childhood math ability between boys and girls. Differences did not emerge with age or by question type. This suggests that boys and girls show equal competency forming mathematics concepts in early childhood.
Recent public discussions surrounding the under-representation of women in STEM fields have suggested that differences in career choices between men and women could be due to intrinsic differences in aptitude in STEM domains. This claim would predict that gender differences should be evident from early on in childhood. Our data, compiled across studies from over 500 infants and children, provide a comprehensive analysis of the effect of gender on early mathematical cognition, and show that in fact, there are no substantive gender differences in mathematical thinking skills during infancy or early childhood. Boys and girls perform equivalently on numerosity perception, counting acquisition, and early school-based math concepts. Our results are consistent with those of a previous study of nearly 200 children who were tested on knowledge of the counting procedure using the “Give-N” task and found no evidence of a statistical difference between boys and girls. 30 Furthermore, early school-based mathematical concepts that build upon knowledge of the logical principles of counting did not show any gender-based differences, suggesting that boys and girls learn mathematics similarly even beyond counting acquisition, into early schooling. This interpretation is consistent with a prior analysis of three million elementary school children showing that school test performance differences in mathematics between boys and girls are non-existent or trivial during elementary school, but steadily increase through high school and college. 10 , 13 Thus, boys and girls begin education with equivalent early mathematical thinking skills.
Although these results are consistent with some previous work in this age range, 10 , 12 , 30 these results contrast with other work in this age range. For example, a small advantage for boys in a variety of numerical skills by the end of kindergarten has been previously reported. 9 However, the growth curve trajectories they fit for each test suggest that these differences were not consistent across every timepoint assessed during kindergarten. For some tests, such as numerical estimation and counting skills, boys and girls were indistinguishable at the initial timepoint. For other tests, such as patterns, number recognition, and number combinations, boys and girls had overlapping scores in the middle timepoints. This shows that even when gender differences are detected, they are inconsistent and highlights the importance of future work that measures gender differences using a longitudinal approach. In contrast, their work found consistent differences in math ability based on socioeconomic status. 9 Although gender differences between socioeconomic statuses could not be assessed in the present study, it is important to take this into consideration in future work. Comparing the present study to previous work also emphasizes the reality that there are many ways to measure mathematical thinking in early childhood and group differences could vary across tasks, cohorts, and age.
The absence of statistical differences across the major developmental milestones of early mathematical cognition are unlikely to be due to sample size. Power analyses suggest that given the sizes of the samples analyzed here, we should have been able to detect small to medium effect sizes ranging from Cohen’s d = 0.34 to 0.65 (80% power, p = 0.05; Infant Numerosity Comparison (looking time): d = 0.65; Early Childhood Numerosity Comparison ( w ): d = 0.37; Recitation of Count List (“How High?” task): d = 0.47; Counting Principles (“Give-N” task): d = 0.52; Math Concepts (TEMA): d = 0.34, (Formal/Informal Math Scores): d = 0.40). Importantly, even if smaller effects do exist, they are unlikely to reliably, meaningfully, or consistently manifest in children. Caution should be taken when interpreting any small effects in large sample to ensure that their importance is not over-exaggerated. 13 , 75
The origin of adult gender differences in science, technology, engineering, and mathematics likely has a complex sociological explanation 2 , 4 and cannot be easily reduced to intrinsic differences in aptitude in early childhood. Women have been discouraged from participating in mathematics and science, and there is a long legacy of sexism in academics. Stereotype threat has been shown to have deleterious effects on girls’ and women’s mathematics performance 19 , 20 (but see Ganley et al. 76 ), and the strength of implicit stereotypes associating men over women with science predicted gender differences in 8th grade math achievement. 77 Prior studies have found that science and mathematics teachers are more likely to encourage boys to ask and answer questions, explain concepts to boys, praise boys, and spend more time interacting with boys. 22 , 23 , 24 , 25 Another source for gender differences includes parental perceptions of children’s abilities. 26 Parents who believed that men are superior at math gave significantly higher math-ability estimates to their sons than to their daughters even when controlling for the children’s actual scores. 27 , 28 Several studies have also found that parental expectations for children’s abilities and success are correlated with their children’s self-concepts of their own abilities and later performance. 26 , 29 In fact, parental perceptions of children’s abilities may influence children’s beliefs in their abilities more than grades. 78 In addition, teachers’ perceptions of students’ math ability have been shown to predict later math achievement scores when earlier measures of ability are controlled. 21 Taken together, there is a strong cultural influence on math achievement throughout childhood. Expelling the stereotype that boys have an intrinsic advantage for mathematics in early childhood may lead to increased mathematics exposure and improved parental and societal perceptions, resulting in improved success in mathematics for girls.
The findings presented here provide strong evidence that boys and girls have comparable cognitive faculties for reasoning about mathematics during early childhood. Although it remains possible that gender differences in STEM involvement emerge later in development from maturation, 4 in other cognitive skills, 79 , 80 or from interactions between cultural stereotypes, training, and sexually dimorphic behaviors, 4 , 81 , 82 there is compelling evidence that males’ and females’ abilities are shaped by different cultural experiences that affect their self-image, treatment, and opportunities, and little evidence to support claims of intrinsic or biological gender differences in early mathematical cognition.
Participants
Five hundred and seven children (256 girls, 251 boys, range = 5 months 13 days to 7.92) contributed 868 measures of quantitative reasoning. Informed consent was obtained from children’s parents and assent was obtained from children aged 7 years and older. Children were rewarded with small toys and stickers, and their parents were compensated for travel expenses.
Children were tested across three testing sites: the University of Rochester in Rochester, NY, the University of Pittsburgh in Pittsburgh, PA, and Duke University in Durham, NC. Protocols were approved by the local Institutional Review Board at each location and parents of all children provided written consent.
Testing procedures
Preferential looking paradigm for numerosity perception.
Eighty children (38 girls, 42 boys, boys’ mean age = 6 months 1 day, girls’ mean age = 6 months and 2 days, range = 5 months 13 days to 6 months 17 days) participated in one of five conditions (16 infants per condition). For full details on additional infants who were tested but excluded from the analyses see previously reported work. 35
Infants were seated on a parent’s lap or in a high chair approximately 105 cm away from the middle of three 17-inch computer screens. The experimenter began the trials when the infant looked at the attractor on the middle screen (see Fig. 2 ). Infants completed four 60-s trials. During each trial, infants were simultaneously presented with image streams on each of the two outer computer screens. One stream continuously alternated between two different numbers of dots (numerosities), while the other stream contained images with a constant number of dots. The numerosities on the alternating stream differed by one of five ratios. Infants were randomly assigned to one of these five conditions: 24 vs. 6 (4:1 ratio), 18 vs. 6 (3:1 ratio), 20 vs. 10 (2:1 ratio), 16 vs. 8 (2:1 ratio), and 18 vs. 12 (3:2 ratio). Each image was presented for 500 ms followed by 300 ms of blank screen. Every other image was the same between the two streams, and identical images were interspersed with images that differed in numerosity. One-third of the images that differed between the two streams were matched on either total surface area, individual element size, or total perimeter of the dots. Because half of the images differing in numerosity were matched on density, the two streams could not be differentiated using element size, cumulative surface area, cumulative perimeter, or density. The side of the changing image stream alternated between trials, and the order was counterbalanced between participants. Half of the participants in each condition saw a non-changing image stream containing the larger numerosity, while the other half saw the smaller numerosity.
Looking behavior was recorded digitally and was later coded by an experienced observer using a custom-made coding program written in RealBasic. 83 A second observer coded more than one-fourth of all participants. Reliability between the two observers was very high ( r = 0.99). For each stream, we calculated the proportion of time each infant spent looking at the changing and non-changing image streams as a function of total looking behavior to both screens for each infant. The analyses presented here were then conducted on preference scores, which were calculated by subtracting the average percent looking time to the non-changing stream from the percent looking time to the changing stream across all four trials, such that a positive score indicates a preference for changing over non-changing streams.
Numerosity discrimination task
Two hundred and fifty children (129 girls, 121 boys, girls’ mean age = 5.43 years, boys’ mean age = 5.46 years, range = 3.07–7.92 years) completed a computerized numerical discrimination task to measure the acuity of their numerosity perception. During the task, children were shown two side-by-side dot arrays too brief to count and were asked to indicate which array contained more dots. Dots varied in location from trial to trial and correct answers (i.e., the larger quantities) were equally presented on the left and right sides of the screen. Children completed one of four versions of this task:
Dot arrays consisted of 1 to 30 dots. Comparisons were defined as having a small, medium, or large number of dots and were made across five different ratios (24 trials per ratio): 4.0 (e.g., 16 dots vs. 4 dots), 3.0, 2.0, 1.43, and 1.11. To ensure that participants used numerical information to make their decision, on half of the trials, the dots were the same size in both arrays, and on the other half of the trials. the dots varied in size between the arrays such that cumulative surface area was the same in both arrays. Dot densities (average interitem distance) varied equally between a large density and a small density.
Dot arrays contained between 12 and 36 dots, and dot size varied within single arrays (average dot diameter = 36 pixels; allowed variation = 20%). There were 18 trials for each of four ratio categories: 2.0, 1.5, 1.17, and 1.14. To ensure that participants used numerical information instead of other perceptual cues to determine the correct response, three trial types were included: Congruent (i.e., the array with the larger number had the larger cumulative area), Incongruent (i.e., the array with the smaller number had the larger cumulative area but both arrays had equal cumulative perimeter), and Neutral (i.e., the arrays had equal cumulative area).
Dot arrays contained 3 to 31 dots and dot size varied within single arrays. There were three ratio categories: 2.0 (easy trial), 1.2533 (medium trial), and 1.11 or less (difficult trial). Children who were successful on the first ten trials (accuracy >80%) completed more medium or difficult trials in the remaining trials to ensure children stayed motivated across the task. Children completed between 30 and 48 trials. In this version, after children made a decision, they placed a bet on how sure they were of their answers. Correct answers were reward with virtual tokens and incorrect answers resulted in taking away tokens.
Dot arrays contained between 3 and 20 dots. Comparisons were made across three ratios (24 trials per ratio): 4.0, 2.0, and 1.25. On ¼ of the trials, dot size was held constant between the two arrays. On another ¼ of the trials, cumulative surface area was constant between the arrays. On ½ the trials, the cumulative surface area varied at a ratio of 2.5 such that on half of those the larger cumulative surface area was congruent with the correct answer and on the other half the larger surface area was incongruent.
To assess the acuity of their numerosity perception, Weber fractions were calculated following Pica et al. 66 Children were excluded from analyses if their performance on the task was at or below chance ( w > 74, based on simulated 50% accuracy data; n = 1 girl, 2 boys) or if their weber fraction was >2 s.d. from the remaining mean ( w > 3.99, n = 3 girls, 3 boys). The final sample consisted of data from 241 children (125 girls, 116 boys, girls’ mean age = 5.47, boys’ mean age = 5.49). Of these children, data from 32 children who completed version A and 36 children who completed version C had been previously reported, respectively 31 , 32 (sample sizes in previous work differ due to differences in age ranges examined in previous vs. present analyses or because some children had also completed the task at an earlier date—we only report the earliest test date in the present work).
“How High?” Task
One hundred and forty-three children (71 girls, 72 boys, girls’ mean age = 4.04 years, boys’ mean age = 4.15 years, range = 2.98–5.46 years) completed a rote memorization counting task where they were asked to count out loud as high as they could up to 100. Performance was measured as the highest number counted with no errors.
Give-N Task
One hundred and twenty-three children (65 girls, 58 boys, girls’ mean age = 3.83 years, boys’ mean age = 3.92 years, range = 2.98–5.47 years) completed a test of knowledge of number word meanings. 69 , 70 In our version of this task, children were asked to remove a specified number of gold coins from a treasure chest, place them on the table, and count them out loud one at a time. Children were allowed to correct their mistakes until they verbally confirmed that the requested number of coins was on the table. The trial was scored as correct or incorrect based on the final number of coins produced. Children were tested on 1 to 10 items, starting with 1 item and continuing until they failed to correctly produced a requested quantity on 2 out of 3 trials of that quantity. If the correct amount was produced, on the next trial, the child was asked to produce a set size of one more than the previous set. If the amount produced was incorrect, then the child was asked for a set size of one less than the previous set. If the child reached 10 items, trials alternated between 9 and 10 until three trials of each set size were administered. Performance was measured as the highest number that was correctly produced on at least two out of three trials.
Test of Early Mathematics Ability Third Edition
Two hundred and seventy-five children (142 girls, 133 boys, girls’ mean age 5.47 years, boys’ mean age = 5.43 years, range = 3.07–7.92 years) were administered the TEMA-3, 73 a comprehensive test of mathematic ability for children aged 3 to 9 years. Questions on the TEMA are classified as formal or informal. Informal concepts draw on untrained, acquired knowledge (e.g., “Show me 3 fingers.”), whereas formal concepts are formally taught in school (e.g., showing an Arabic numeral to the child and asking “What number is this?”). Following standard administration rules, children began at different points on the TEMA depending on their age and continued with the test until five consecutive items were answered incorrectly. Items before the starting point were only administered if the child did not reach five consecutive items that were answered correctly prior to reaching the ceiling point. Overall performance was based on raw scores, which were calculated by first finding the farthest point in the test where five consecutive items were answered correctly. Children then received a point for every question up through that series of five items. This means that children automatically received credit for every question up to the point where correct answers became irregular (<5 consecutive correct items), even for questions that were before the first tested item. An additional point was added to children’s scores for each item answered correctly after that point. Formal and informal scores were the average score (proportion correct) in each category. Unlike the overall performance scores, children were not given credit for formal and informal items that were not administered (e.g., items before the first item tested). Data from 77 children have been previously reported n = 10, 33 n = 23, 32 n = 44. 34
Statistical tests were performed using R (version 3.3.1) and R-Studio (version 1.0.44). Independent-samples t tests were conducted using the “ttest” function assuming equal variance. Tests of equivalence were conducted using the “TOSTtwo.raw” function from the TOSTER package (upper and lower bounds set to ±0.5 × standard deviation of the entire group; α = 0.05). This function returns two t values ( t 1 and t 2 ). For statistical equivalence, both t values must be statistically significant. Statistical equivalence is rejected if either t 1 or t 2 does not reach significance. Regressions were conducted using the “lm” function. Levene’s test of equivalence was carried out using the “leveneTest” function in the car package. ANOVAs were conducted using the “ezANOVA” function (type = 3) from the ez package.
Growth curves were calculated at the group level. 40 Specifically, Eq. 1 was fit to the data using the nls function (“port” algorithm) in R. Equation 1 fits two free parameters, alpha ( α ), which indicates the rate of growth, and lambda ( λ ), which indicates the age at which children are halfway to the maximum possible score. In Eq. 1 β 0 and β 1 represent the lower and upper score limits and are set to the lowest and highest possible scores for a given measure before the free parameters are fit to the data.
The growth curves displayed in Figs. 3 – 5 were calculated across the group data, and the standard deviation (shaded areas) at each age was calculated across 10,000 growth curves fit by bootstrapping the data. Because these curves were fit at the group level rather than the individual level, no statistical tests of gender-specific growth rates were conducted. Instead, these growth curves are meant to simply provide a visualization of boys’ and girls’ performance within the age range of the children in our sample.
Data availability
The datasets analyzed during the current study are available from the corresponding author on reasonable request.
Ecuyer-Dab, I. & Robert, M. Have sex differences in spatial ability evolved from male competition for mating and female concern for survival? Cognition 91 , 221–257 (2004).
Article PubMed Google Scholar
Geary, D. C. Sexual selection and sex differences in mathematical abilities. Behav. Brain Sci. 19 , 229–284 (1996).
Article Google Scholar
Sherry, D. F. & Hampson, E. Evolution and the hormonal control of sexually-dimorphic spatial abilities in humans. Trends Cogn. Sci. 1 , 50–56 (1997).
Article PubMed CAS Google Scholar
Halpern, D. F. et al. The science of sex differences in science and mathematics. Psychol. Sci. Public Interest. 8 , 1–51 (2007).
Article PubMed PubMed Central Google Scholar
Pinker, S. & Spelke, E. S. The Science of Gender and Science. Pinker vs. Spelke: A Debate (2005). www.edge.org/3rd_culture/debate05/debate05_index.html
Hedges, L. & Nowell, A. Sex differences in mental test scores, variability, and numbers of high-scoring individuals. Science (80-.) 269 , 41–45 (1995).
Article CAS Google Scholar
Benbow, C. P. & Stanley, J. C. Sex differences in mathematical abilty: fact or artifact? Science (80-.) 210 , 1262–1264 (1980).
Benbow, C. P. & Stanley, J. C. Sex differences in mathematical reasoning ability: more facts. Science (80-.) 22 , 1029–1031 (1983).
Jordan, N. C., Kaplan, D., Olah, L. N. & Locuniak, M. N. Number sense growth in kindergarten: a longitudinal investigation of children at-risk for mathematics difficulities. Child Dev. 77 , 153–177 (2006).
Hyde, J. S., Fennema, E. & Lamon, S. J. Gender differences in mathematics performance—a metaanalysis. Psychol. Bull. 107 , 139–155 (1990).
Kimball, M. M. A new perspective on women’s math achievement. Psychol. Bull. 105 , 198–214 (1989).
Hyde, J. S., Lindberg, S. M., Linn, M. C., Ellis, A. B. & Williams, C. C. Gender similarities characterize math performance. Science (80-.) 321 , 494–495 (2008).
Hyde, J. S. & Linn, M. C. Gender similarities in mathematics and science. Science (80-.) 314 , 599–600 (2006).
Lindberg, S. M., Hyde, J. S., Petersen, J. L. & Linn, M. C. New trends in gender and mathematics performance: a meta-analysis. Psychol. Bull. 136 , 1123–1135 (2010).
Siegler, R. S. & Mu, Y. Chinese children excel on novel mathematics problems even before elementary school. Psychol. Sci. 19 , 759–763 (2008).
Stevenson, W., Lee, S. & Stigler, J. W. Mathematics achievement of Chinese, Japanese, and American children. Science (80-.) 231 , 693–699 (1986).
Reilly, D., Neumann, D. L. & Andrews, G. Investigating gender differences in mathematics and science: Results from the 2011 Trends in Mathematics and Science Survey. Res. Sci. Educ . 1–26 (2017)..
Stoet, G. & Geary, D. C. Sex differences in mathematics and reading achievement are inversely related: within- and across-nation assessment of 10 years of PISA data. PLoS ONE 8 , e57988 (2013).
Article PubMed PubMed Central CAS Google Scholar
Doyle, R. A. & Voyer, D. Stereotype manipulation effects on math and spatial test performance: a meta-analysis. Learn. Individ. Differ. 47 , 103–116 (2016).
Steele, C. M. A threat in the air. How stereotypes shape intellectual identity and performance. Am. Psychol. 52 , 613–629 (1997).
Jussim, L. & Eccles, J. S. Teacher expectations II: constructions and reflection of student achievement. J. Pers. Soc. Psychol. 63 , 947–961 (1992).
American Association of University Women Educational Foundation. How schools shortchange girls. AAUW Rep . 1–13 (1992).
Becker, J. R. Differential treatment of females and males in mathematics classes. J. Res. Math. Educ. 12 , 40–53 (1981).
Jones, M. G. & Wheatley, J. Gender differences in teacher-student interactions in science classrooms. J. Res. Sci. Teach. 27 , 861–874 (1990).
Kelly, S. N. Teachers’ useful skills. J. Res. Music Educ. 46 , 374–383 (1998).
Bleeker, M. M. & Jacobs, J. E. Achievement in math and science: Do mothers’ beliefs matter 12 years later? J. Educ. Psychol. 96 , 97–109 (2004).
Jacobs, J. E. Influence of gender stereotypes on parent and child mathematics attitudes. J. Educ. Psychol. 83 , 518–527 (1991).
Jacobs, J. E. & Eccles, J. S. The impact of mothers’ gender-role stereotypic beliefs on mothers’ and children’s ability perceptions. J. Pers. Soc. Psychol. 63 , 932–944 (1992).
Parsons, J. E., Adler, T. F. & Kaczala, C. M. Socialization of achievement attitudes and beliefs: parental Influences. Child Dev. 53 , 310–321 (1982).
Spelke, E. S. & Ellison, K. in The Science on Women and Science (ed. Sommers, C. H.) 24–53 (The AEI Press, Washington, DC, 2009).
Kersey, A. J. & Cantlon, J. F. Neural tuning to numerosity relates to perceptual tuning in 3- to 6-year-old children. J. Neurosci. 37 , 512–522 (2017).
Vo, V. A., Li, R., Kornell, N., Pouget, A. & Cantlon, J. F. Young children bet on their numerical skills: metacognition in the numerical domain. Psychol. Sci. 25 , 1712–1721 (2013).
Cantlon, J. F. & Li, R. Neural activity during natural viewing of Sesame Street statistically predicts test scores in early childhood. PLoS Biol. 11 , e1001462 (2013).
Elliott, L., Braham, E. J. & Libertus, M. E. Understanding sources of individual variability in parents’ number talk with young children. J. Exp. Child Psychol. 159 , 1–15 (2017).
Libertus, M. E. & Brannon, E. M. Stable individual differences in number discrimination in infancy. Dev. Sci. 13 , 900–906 (2010).
Schuirmann, D. J. A comparison of the Two One-Sided Tests Procedure and the Power Approach for assessing the equivalence of average bioavailability. J. Pharmacokinet. Biopharm. 15 , 657–680 (1987).
Ball, L. C., Cribbie, R. A. & Steele, J. R. Beyond gender differences: using tests of equivalence to evaluate gender similarities. Psychol. Women Q. 37 , 147–154 (2013).
Hyde, J. S. Gender similarities and differences. Annu. Rev. Psychol. 65 , 373–398 (2014).
Hyde, J. S. The gender similarities hypothesis. Am. Psychol. 60 , 581–592 (2005).
Marceau, K., Ram, N., Houts, R. M., Grimm, K. J. & Susman, E. J. Individual differences in boys’ and girls’ timing and tempo of puberty: Modeling development with nonlinear growth models. Dev. Psychol. 47 , 1389–1409 (2011).
Gallistel, C. R. & Gelman, R. Preverbal counting and computation. Cognition 44 , 43–74 (1992).
Jordan, K. E. & Brannon, E. M. The multisensory representation of number in infancy. Proc. Natl. Acad. Sci. USA 103 , 3486–3489 (2006).
Izard, V., Sann, C., Spelke, E. S. & Streri, A. Newborn infants perceive abstract numbers. Proc. Natl. Acad. Sci. USA 106 , 10382–10385 (2009).
Xu, F. & Spelke, E. S. Large number discrimination in 6-month-old infants. Cognition 74 , B1–B11 (2000).
Lipton, J. S. & Spelke, E. S. Origins of the number sense: large-number discrimination in human infants. Psychol. Sci. 14 , 396–401 (2003).
Beran, M. J. Rhesus monkeys ( Macaca mulatta ) enumerate large and small sequentially presented sets of items using analog numerical representations. J. Exp. Psychol. Anim. Behav. Process. 33 , 42–54 (2007).
Cantlon, J. F. & Brannon, E. M. Shared system for ordering small and large numbers in monkeys and humans. Psychol. Sci. 17 , 401–406 (2006).
Cantlon, J. F. & Brannon, E. M. Basic math in monkeys and college students. PLoS Biol. 5 , 2912–2919 (2007).
Beran, M. J. Chimpanzees ( Pan troglodytes ) respond to nonvisible sets after one-by-one addition and removal of items. J. Comp. Psychol. 118 , 25–36 (2004).
Beran, M. J. The evolutionary and developmental foundations of mathematics. PLoS Biol. 6 , 221–223 (2008).
Gallistel, C. R. Animal cognition: the representation of space, time and number. Annu. Rev. Psychol. 40 , 155–189 (1989).
Halberda, J. & Feigenson, L. Developmental change in the acuity of the ‘number sense’: The approximate number system in 3-, 4-, 5-, and 6-year-olds and adults. Dev. Psychol. 44 , 1457–1465 (2008).
Casey, M. B., Nuttall, R., Pezaris, E. & Benbow, C. P. The influence of spatial ability on gender differences in mathematics college entrance test scores across diverse samples. Dev. Psychol. 31 , 697–705 (1995).
Libertus, M. E., Feigenson, L. & Halberda, J. Preschool acuity of the approximate number system correlates with school math ability. Dev. Sci. 14 , 1292–1300 (2011).
Starr, A., Libertus, M. E. & Brannon, E. M. Number sense in infancy predicts mathematical abilities in childhood. Proc. Natl. Acad. Sci. USA 110 , 18116–18120 (2013).
Tibber, M. S. et al. Sensitivity to numerosity is not a unique visuospatial psychophysical predictor of mathematical ability. Vision Res. 89 , 1–9 (2013).
De Smedt, B., Noël, M.-P., Gilmore, C. & Ansari, D. How do symbolic and non-symbolic numerical magnitude processing skills relate to individual differences in children’s mathematical skills? A review of evidence from brain and behavior. Trends Neurosci. Educ. 2 , 48–55 (2013).
Antell, S. E. & Keating, D. P. Perception of numerical invariance in neonates. Child Dev. 54 , 695–701 (2016).
Strauss, M. S. & Curtis, L. E. Infant perception of numerosity. Child Dev. 52 , 1146–1152 (2016).
Starkey, P., Spelke, E. S. & Gelman, R. Numerical abstraction by human infants. Cognition 36 , 97–127 (1990).
Halberda, J., Mazzocco, M. M. M. & Feigenson, L. Individual differences in non-verbal number acuity correlate with maths achievement. Nature 455 , 665–668 (2008).
Feigenson, L., Libertus, M. E. & Halberda, J. Links between the intuitive sense of number and formal mathematics ability. Child Dev. Perspect. 7 , 74–79 (2013).
Piazza, M., Izard, V., Pinel, P., Le Bihan, D. & Dehaene, S. Tuning curves for approximate numerosity in the human intraparietal sulcus. Neuron 44 , 547–555 (2004).
Whalen, J., Gallistel, C. R. & Gelman, R. Nonverbal counting in humans: the psychophysics of number representation. Psychol. Sci. 10 , 130–137 (1999).
Cordes, S., Gelman, R., Gallistel, C. R. & Whalen, J. Variability signatures distinguish verbal from nonverbal counting for both large and small numbers. Psychon. Bull. Rev. 8 , 698–707 (2001).
Pica, P., Lemer, C., Izard, V. & Dehaene, S. Exact and approximate arithmetic in an Amazonian indigene group. Science (80-.) 306 , 499–503 (2004).
Gelman, R. & Gallistel, C. R. The Child’s Understanding of Number (Harvard University Press, Cambridge, MA, 1978).
Gallistel, C. R. & Gelman, R. Preverbal and verbal counting and computation. Cognition 44 , 43–74 (1992).
Wynn, K. Children’s understanding of counting. Cognition 36 , 155–193 (1990).
Wynn, K. Children’s acquisition of the number words and the counting system. Cogn. Psychol. 24 , 220–251 (1992).
Le Corre, M. & Carey, S. One, two, three, four, nothing more: An investigation of the conceptual sources of the verbal counting principles. Cognition 105 , 395–438 (2007).
Lipton, J. S. & Spelke, E. S. Preschool children’s mapping of number words to nonsymbolic numerosities. Child Dev. 76 , 978–988 (2005).
Ginsburg, H. & Baroody, A. J. Test of Early Mathematics Ability, 3rd Edition (TEMA-3) . (PRO-ED, Austin, TX, 2003).
Libertus, M. E., Feigenson, L. & Halberda, J. Numerical approximation abilities correlate with and predict informal but not formal mathematics abilities. J. Exp. Child Psychol. 116 , 829–838 (2013).
Wilkinson, L., Task Force on Statistical Inference American Psychological. Statistical methods in psychology journals: guidelines and explanations. Am. Psychol. 54 , 594–604 (1999).
Ganley, C. M. et al. An examination of stereotype threat effects on girls’ mathematics performance. Dev. Psychol. 49 , 1886–1897 (2013).
Nosek, B. A. et al. National differences in gender-science stereotypes predict national sex differences in science and math achievement. Proc. Natl. Acad. Sci. USA 106 , 10593–10597 (2009).
Frome, P. M. & Eccles, J. S. Parents’ influence on children’s achievement-related perceptions. J. Pers. Soc. Psychol. 74 , 435–452 (1998).
Moore, D. S. & Johnson, S. P. Mental rotation in human infants. Psychol. Sci. 19 , 1063–1066 (2008).
Levine, S. C., Huttenlocher, J., Taylor, A. & Langrock, A. Early sex differences in spatial skill. Dev. Psychol. 35 , 940–949 (1999).
Geary, D. C. A model for representing gender differences in the pattern of cognitive abilities. Am. Psychol. 44 , 1155–1156 (1989).
Arnold, A. P. et al. Minireview: sex chromosomes and brain sexual differentiation. Endocrinology 145 , 1057–1062 (2004).
Libertus, K. Preferential looking coder. Available at: http://www.duke.edu/~k141 (2008). Accessed 5 August 2011.
Download references
Acknowledgements
This work was supported by the National Science Foundation (DRL-1459625 to J.F.C. and DGE-1419118 to A.J.K.) and the National Institutes of Health (R01 HD064636 to J.F.C). E.J.B and M.E.L were supported by the National Science Foundation, grant number DUE-1534830.
Author information
Authors and affiliations.
Department of Brain and Cognitive Sciences, University of Rochester, Rochester, NY, 14627, USA
Alyssa J. Kersey, Kelsey D. Csumitta & Jessica F. Cantlon
Rochester Center for Brain Imaging, University of Rochester Medical Center, Rochester, NY, 14620, USA
Alyssa J. Kersey & Jessica F. Cantlon
Department of Psychology, University of Pittsburgh, Pittsburgh, PA, 15260, USA
Emily J. Braham & Melissa E. Libertus
Learning Research and Development Center, University of Pittsburgh, Pittsburgh, PA, 15260, USA
You can also search for this author in PubMed Google Scholar
Contributions
A.J.K., M.E.L., and J.F.C. designed the research, A.J.K, E.J.B, and K.D.C analyzed the data, A.J.K, K.D.C., M.E.L., and J.F.C. wrote the paper. All authors revised and approved the paper.
Corresponding author
Correspondence to Alyssa J. Kersey .

Ethics declarations
Competing interests.
The authors declare no competing interests.
Additional information
Publisher's note: Springer Nature remains neutral with regard to jurisdictional claims in published maps and institutional affiliations.
Electronic supplementary material
Supplementary notes, rights and permissions.
Open Access This article is licensed under a Creative Commons Attribution 4.0 International License, which permits use, sharing, adaptation, distribution and reproduction in any medium or format, as long as you give appropriate credit to the original author(s) and the source, provide a link to the Creative Commons license, and indicate if changes were made. The images or other third party material in this article are included in the article’s Creative Commons license, unless indicated otherwise in a credit line to the material. If material is not included in the article’s Creative Commons license and your intended use is not permitted by statutory regulation or exceeds the permitted use, you will need to obtain permission directly from the copyright holder. To view a copy of this license, visit http://creativecommons.org/licenses/by/4.0/ .
Reprints and permissions
About this article
Cite this article.
Kersey, A.J., Braham, E.J., Csumitta, K.D. et al. No intrinsic gender differences in children’s earliest numerical abilities. npj Science Learn 3 , 12 (2018). https://doi.org/10.1038/s41539-018-0028-7
Download citation
Received : 01 November 2017
Revised : 07 June 2018
Accepted : 20 June 2018
Published : 06 July 2018
DOI : https://doi.org/10.1038/s41539-018-0028-7
Share this article
Anyone you share the following link with will be able to read this content:
Sorry, a shareable link is not currently available for this article.
Provided by the Springer Nature SharedIt content-sharing initiative
This article is cited by
How do numerical and literacy stimulations in mother–child interactions relate to early gender differences in mathematical competencies empirical results from the neps newborn cohort study.
- Loreen Beier
- Hans-Peter Blossfeld
Large-scale Assessments in Education (2023)
Static and dynamic assessment of STEM gender stereotypes in secondary education using a novel cluster-based analysis
- Jose-Luis Diez
- Amparo Ramos
- Carlos Candela
International Journal of Technology and Design Education (2023)
Assessment of gender divide in scientific communities
- Antonio De Nicola
- Gregorio D’Agostino
Scientometrics (2021)
- Alyssa J. Kersey
- Kelsey D. Csumitta
- Jessica F. Cantlon
npj Science of Learning (2019)
Sex differences in brain correlates of STEM anxiety
- Ariel A. Gonzalez
- Katherine L. Bottenhorn
- Angela R. Laird
Quick links
- Explore articles by subject
- Guide to authors
- Editorial policies
Sign up for the Nature Briefing newsletter — what matters in science, free to your inbox daily.

ORIGINAL RESEARCH article
Gender differences in mathematics motivation: differential effects on performance in primary education.
- 1 Department of Psychology, University of A Coruña, A Coruña, Spain
- 2 Department of Pedagogy and Didactics, University of Santiago de Compostela, Santiago de Compostela, Spain
- 3 Department of Pedagogy and Didactics, University of A Coruña, A Coruña, Spain
In addition to attempting to verify gender differences, this study aims to examine the explanatory potential of boys’ and girls’ attitudes toward mathematics on their performance. The sample comprised 897 students in the 5th and 6th years of primary education (450 boys and 447 girls). The results confirm what previous research has suggested, that girls tended to exhibit less positive attitudes about mathematics than their male classmates, in particular lower motivation, worse perception of competence, and higher rates of anxiety, although in all cases the effect sizes were small. Even though there no significant gender differences in academic performance, as expected, the explanatory power of attitudes toward mathematics was clearly more significant in boys than in girls ( R 2 = 0.194 and R 2 = 0.103, respectively). The results of the regression analysis for each sample reinforce the well-known positive impact of perceived self-efficacy on mathematics performance and introduce the effect of achievement emotions of academic performance. Test anxiety in mathematics seems to only have a negative effect on boys’ grades, as this variable does not appear in the regression equation when explaining girls’ performance. In the light of control-value theory, we discuss the contingency of perceived competence and its involvement in anxiety and academic performance. Boys’ results could be affected by the levels of anxiety inasmuch as they tend to be confident in their abilities, motivated to stand out, and interested in mathematics. Whereas despite girls reporting high rates of anxiety, what may have a negative impact on their results might have more to do with a higher value placed on mathematics, as their perception of control may be low.
Introduction
Spanish students’ scores in the mathematics tests in Programme for International Student Assessment (PISA) 2015 ( Ministerio de Educación Cultura y Deporte [MECD], 2016 ) do not help us to be optimistic about the teaching and learning in this subject in our country. The score in the mathematics test from May 2015, which evaluated 37,205 of the 414,276 15-year-old students in Spain, was 486 points, significantly lower than the OECD average of 490 points. In a ranking of the 36 member countries, Spain is in 26th position, and only 7.2% of Spanish students reached high levels of achievement (5 and 6) in mathematics, which is 3.5 percentage points less than the OECD average (10.7%).
These results seem to reflect a problem that continually calls into question the effectiveness of teaching–learning processes for mathematics content. Motivational and emotional variables, which involve beliefs, emotions, and attitudes, seem to be important when it comes to fully understanding and explaining the results. In fact, everything points toward math achievement being related to variables such as perceived competence and self-efficacy ( Randhawa et al., 1993 ; Pajares and Graham, 1999 ; Fast et al., 2010 ; Williams and Williams, 2010 ; Parker et al., 2014 ), interest ( Köller et al., 2001 ; Lee et al., 2014 ; Jansen et al., 2016 ) and anxiety ( Ashcraft, 2002 ; Ashcraft and Ridley, 2005 ; Lyons and Beilock, 2012 ; Ramirez et al., 2016 ).
In this context, when we look at the results of PISA 2015 ( Ministerio de Educación Cultura y Deporte [MECD], 2016 ), the difference in mathematics performance between boys and girls aged 15 is an average of 8 points in OECD countries and 11 points in the European Union (EU) overall, with boys scoring higher than girls. In Spain, boys scored 16 points higher than girls, much higher than the OECD average. Various meta-analyses in the 1970s and 1980s demonstrated a slight male advantage in mathematics in secondary students ( Hyde et al., 1990 ; Hedges and Nowell, 1995 ), at least in complex problem-solving tasks. However, this varies by country ( Else-Quest et al., 2010 ; Mullis et al., 2012 ; Ministerio de Educación Cultura y Deporte [MECD], 2016 ), and more recent data suggest that this gender gap may be disappearing ( Hyde et al., 2008 ; Hyde and Mertz, 2009 ; Lindberg et al., 2010 ).
It is an established fact that beliefs and attitudes can have a significant impact on the decision to choose a professional career related to mathematics ( Colbeck et al., 2001 ; Ceci and Williams, 2011 ; Sadler et al., 2012 ; Kanny et al., 2014 ; Legewie and DiPrete, 2014 ; Wang and Degol, 2017 ). In addition, women are often underrepresented in STEM programs (science, technology, engineering, and mathematics programs), particularly in engineering, physical sciences, and computational sciences in western universities (e.g., Larivière et al., 2013 ; Hyde, 2014 ). With that in mind, it is essential to include this topic in the research agenda of educational psychology in order to thoroughly understand the interaction between motivational constructs and each group’s performance (girls and boys). In addition, research suggests that gender differences in mathematics ability are minimal during early childhood and do not consistently arise until middle to late adolescence (e.g., Lindberg et al., 2010 ; Robinson and Lubienski, 2011 ). The current study addresses the need to delve into the affective-motivational dimension of students in the final years of primary education, as girls may be losing their motivation for mathematics as they advance through this particular educational stage.
Academic Motivation and Performance in Mathematics
Motivational research in mathematics has addressed constructs such as self-efficacy, which indicates students’ judgments of their abilities to perform specific mathematical tasks, and interest in mathematics, as positive affective experiences in activities related to mathematics. As laid out in expectancy-value theory, both expectations of success and subjective values related to the task directly influence performance, attainment, effort, and persistence ( Wigfield and Eccles, 2000 ). Various studies have shown that perceived self-efficacy positively predicts academic achievement in mathematics ( Randhawa et al., 1993 ; Pajares and Graham, 1999 ; Stevens et al., 2006 ; Fast et al., 2010 ; Williams and Williams, 2010 ; Parker et al., 2014 ). Similarly, students’ interest in mathematics is associated with a strong preference for mathematics content, which translates to sustained commitment over time and better performance ( Köller et al., 2001 ; Hidi and Renninger, 2006 ; Lee et al., 2014 ; Jansen et al., 2016 ) in both childhood and adolescence ( Lepper et al., 2005 ; Aunola et al., 2006 ; Denissen et al., 2007 ; Viljaranta et al., 2009 ).
There is also evidence that math anxiety is negatively related to performance, leading to avoidance and diminishing working memory resources needed to deal with mathematical tasks ( Ashcraft, 2002 ; Ashcraft and Ridley, 2005 ; Lyons and Beilock, 2012 ; Ramirez et al., 2016 ). The nuances of the research must be understood when it comes to operationalizing the measure of math anxiety, as must the fact that the full spectrum of emotional reactions may be associated with it ( Goetz and Hall, 2013 ; Pekrun and Linnenbrink-Garcia, 2014 ) and affect academic performance ( Pekrun et al., 2014 , 2017 ). For that reason, in this study, we address negative emotions associated with mathematics and math anxiety separately. In accordance with the control-value theory of achievement emotions ( Pekrun, 2006 ), students who enjoy mathematics are assumed to focus their attention on the tasks, making better use of deep learning strategies and, therefore, getting better results. Students who are, for example, bored in mathematics classes pay less attention and make less use of learning strategies or use more superficial strategies, which leads to them having lower achievement ( Pekrun, 2006 ; Pekrun et al., 2017 ; Putwain et al., 2018 ).
Gender Differences in Mathematics Motivation
Research has confirmed gender differences, even in primary education, in mathematics self-concept, self-efficacy, and interest, suggesting that boys generally have better motivational profiles in mathematics than have girls ( Eccles et al., 1993 ; Kurtz-Costes et al., 2008 ). The study of gender differences in mathematics motivation in these first educational phases is of particular interest. In this way, the most could be made of female students’ potential, improving the gender balance of participation in future STEM courses, which have been recognized as a critical filter for highly qualified and highly paid jobs.
According to previous research ( Fredricks and Eccles, 2002 ; Jacobs et al., 2002 ; Preckel et al., 2008 ; Else-Quest et al., 2010 ; Frenzel et al., 2010 ; Guo et al., 2015 ; Ganley and Lubienski, 2016 ), girls report lower levels of individual interest and perceived mathematics competence. The most significant differences are in secondary school and university students rather than students in lower educational levels.
Demonstrating the need to explore gender differences in academic motivation, previous research has concluded that, for example, mathematics self-concept could be positively linked to achievement in boys but could even have a negative effect on girls’ achievement ( Yoon et al., 1996 ). It has also demonstrated that the impact of interest on mathematics achievement may be slightly more important for girls than for boys ( Ganley and Lubienski, 2016 ). On this point, although expectation-value theory does not develop a theoretical framework to address gender differences in particular, it may be used to facilitate the interpretation of the differential impact of self-beliefs and values ( Wigfield et al., 1991 , 1997 ; Eccles et al., 1993 ).
In terms of gender differences related to emotions provoked by mathematics, among which studies on math anxiety stand out, research indicates (albeit with small effect sizes) the existence of greater rates of anxiety in girls than boys during tasks involving mathematical reasoning ( Hyde et al., 1990 ; Else-Quest et al., 2010 ). Assuming the possibility that these differences start as early as primary school ( Yüksel-şahin, 2008 ; Griggs et al., 2013 ), research suggests the existence of a higher rate of math anxiety, and also more negative feelings and attitudes in boys than girls ( Hyde et al., 1990 ; Nagy et al., 2008 ; Goetz and Hall, 2013 ; Goetz et al., 2013 ; Bieg et al., 2015 ). According to the principles of control-value theory, studies addressing the different impact suggest that positive emotions associated with mathematics could have a more pronounced effect on girls’ dedication ( Pinxten et al., 2014 ) and that the rates of anxiety and negative emotions may not affect girls’ achievement as negatively as might have been expected ( Goetz et al., 2013 ).
Based on these considerations, and apart from attempting to verify gender differences, the primary purpose of this study is to analyze the possible differential impact of the variables used to explore mathematical motivation on the academic achievement of boys and girls in primary education. Firstly, we hypothesize that there will be statistically significant differences in mathematical motivation between boys and girls. We expect the boys’ motivational pattern, in terms of perceived competence and intrinsic motivation for mathematics, to be more positive than the girls’ ( Fredricks and Eccles, 2002 ; Jacobs et al., 2002 ; Preckel et al., 2008 ; Else-Quest et al., 2010 ; Frenzel et al., 2010 ; Guo et al., 2015 ; Ganley and Lubienski, 2016 ), and the girls to exhibit more negative feelings and greater anxiety toward mathematics than do the boys ( Hyde et al., 1990 ; Nagy et al., 2008 ; Goetz et al., 2013 ; Bieg et al., 2015 ). We will examine the explanatory potential and differential incidence of competency beliefs and intrinsic motivation, along with negative feelings and anxiety over mathematics performance in boys and girls, assuming that perceived competence will have an impact on academic achievement in both cases ( Randhawa et al., 1993 ; Pajares and Graham, 1999 ; Stevens et al., 2006 ; Fast et al., 2010 ; Williams and Williams, 2010 ; Parker et al., 2014 ). We will also explore the possibility that the impact of intrinsic motivation on performance will be more significant when it comes to explaining girls’ achievement rather than boys’ (e.g., Yoon et al., 1996 ; Ganley and Lubienski, 2016 ). Finally, we will also look at whether anxiety is more important when explaining boys,’ compared with girls,’ mathematics achievement (e.g., Goetz et al., 2013 ).
Materials and Methods
Participants.
The sample was composed of 897 students from 13 public primary schools in the Spanish province of A Coruña. Half (50.2%) were boys and half (49.8%) were girls. They were aged between 9 and 13 years ( M = 10.77; SD = 0.69). Out of the total, 437 were in the 5th year of primary education (223 boys and 213 girls) and 460 were in the 6th year of primary education (227 boys and 233 girls).
Instruments
To measure students’ attitudes toward mathematics, we used the IAM ( Inventario de Actitudes hacia las Matemáticas/Inventory of Attitudes Toward Mathematics ). This instrument is the result of expanding the Fennema–Sherman Mathematics Attitude Scale (FSS) by Fennema and Sherman (1976) . It is an extended version of the scale, with some modifications, adapted into Spanish and including new dimensions aimed at measuring more accurately students’ attitudes and motivation for mathematics ( Silva, 2005 ; González-Pienda et al., 2012 ; Cueli et al., 2014 ). In this study, we used the following IAM dimensions:
• Intrinsic motivation toward mathematics (four items; α = 0.72): this evaluates motivation toward learning and understanding mathematics content for the pleasure and personal satisfaction that comes from working with this type of content (example item: “I find mathematics enjoyable and stimulating”).
• Perceived competence in mathematics (four items; α = 0.75): this evaluates the level of confidence in oneself for learning and getting good mathematics results (example item: “I think I can do even more difficult math tasks”).
• Negative feelings caused by mathematics (three items; α = 0.71): this evaluates the presence and intensity of sadness and unease caused by studying, homework, or attending math classes (example item: “In math class I am sad and unhappy”).
• Math anxiety (three items; α = 0.77): this evaluates the level of students’ fear and nervousness with math tests and tasks (example item: “I feel uncomfortable and nervous about mathematics”).
The items in each dimension were in a Likert-type format with five response options from 1 (completely false) to 5 (completely true).
The evaluation of academic performance in mathematics was obtained via the final school grades that the participating students received in this subject. The following grades were used: 1 = poor, 2 = satisfactory, 3 = good, 4 = very good, and 5 = outstanding.
The data were collected during school hours by personnel external to the school with the prior consent of the school management and the students’ teachers. Prior to participating in the study, the teachers, students, and parents (depending on school regulations) were informed of the study content and procedure. Before data collection, which was done on a single occasion, the participants were reminded of the importance of answering the various questions honestly.
Data about the target variables were collected in accordance with the recommendations of the ethical standards established in the Research and Teaching Ethics Committee of the University of A Coruña and the Declaration of Helsinki. Confidentiality of data was ensured, and participation was voluntarily such that withdrawal from the study was possible at any time.
Data Analysis
In addition to descriptive and correlational analyses, analysis of variance (ANOVA) was also used to examine gender differences in mathematics motivation. A stepwise linear regression analysis was performed to examine the predictive capacity of the motivational variables on achievement in mathematics between boys and girls. In both cases, the predictor variables were intrinsic motivation , perceived competence , negative feelings , and math anxiety , with the criterion variable being the students’ final academic grade in mathematics. Firstly, the analysis aimed to understand the contribution of each of the variables added to the regression equation when it came to predicting mathematics performance. In addition, we wanted to ascertain the weight and specific significance of the predictor variables in each sample. Effect sizes were calculated according to the criteria in Cohen (1988) classic work: d < 0.20 = non-significant effect; d ≥ 0.20 and d < 0.50 = small effect; d ≥ 0.50 and d < 0.80 = moderate effect; and d ≥ 0.80 = large effect.
The analysis of results first looks at determining whether there are significant differences in intrinsic motivation , perceived competence , negative feelings , and math anxiety by gender . Following that, we examine the contribution of this set of affective-motivational variables on students’ academic performance.
Table 1 shows the correlation coefficients as well as descriptive statistics of central tendency, distribution, and dispersion for the variables in this study. Considering the correlations, academic achievement in mathematics had a positive, statistically significant relationship to intrinsic motivation toward mathematics ( r = 0.19 , p < 0.01) and perceived competence ( r = 0.28 , p < 0.01). Anxiety and negative feelings toward mathematics had a negative association with performance ( r = −0.29, p < 0.01, and r = −0.33, p < 0.01, respectively).
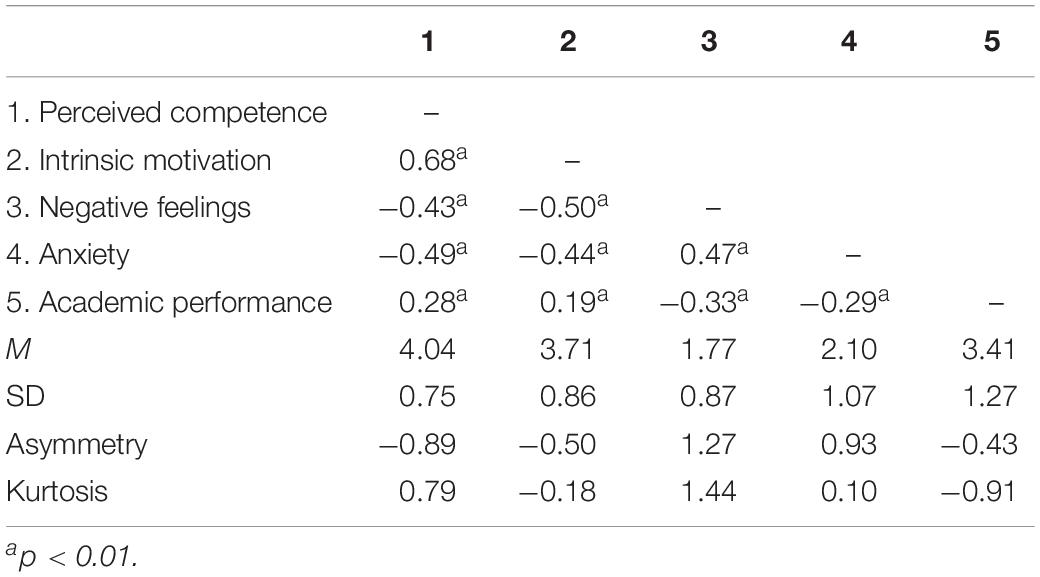
Table 1. Means, standard deviations, asymmetry, kurtosis, and correlation matrix.
The relationship between perceived competence and both negative emotions and math anxiety was negative and significant ( r = −0.43, p < 0.01, and r = −0.49, p < 0.01, respectively). Similarly, negative feelings toward mathematics and anxiety were both significantly, negatively correlated with intrinsic motivation toward mathematics ( r = −0.50, p < 0.01, and r = −0.49, p < 0.01, respectively). As one might expect, there was a significant, positive association between negative feelings toward mathematics and math anxiety ( r = 0.47, p < 0.01).
Differences in Mathematics Motivation Between Boys and Girls
We found statistically significant differences in mathematics motivation between boys and girls in primary education, with a moderate effect size, even though the differences in mathematics performance were not significant [ F ( 1 , 895 ) = 1.174, p = 0.279]. Girls reported lower levels of self-efficacy [ F ( 1 , 985 ) = 11.227; p < 0.01; η p 2 = 0.012; d = 0.71] and intrinsic motivation [ F ( 1 , 895 ) = 6.522; p < 0.05; η p 2 = 0.007; d = 0.61] for mathematics than did boys. On the other hand, whereas both boys and girls had similar levels of negative feelings toward mathematics [ F ( 1 , 895 ) = 1.272, p = 0.260], the girls exhibited higher rates of math anxiety than did their male classmates [ F ( 1 , 895 ) = 11.018; p < 0.01; η p 2 = 0.012; d = 0.70].
The Impact of Motivation on Mathematics Performance in Girls and Boys
We performed two identical stepwise regression analyses, one for the sample of boys ( n = 450; M age = 10.79; SD = 0.70) and one for the girls ( n = 447; M age = 10.75; SD = 0.68), with the aim of determining the predictive value of the motivational variables on mathematics performance in each sample.
As Table 2 shows, in the boys’ group, both negative feelings and anxiety associated with mathematics, along with perceived competence in the subject, contributed significantly to the prediction of academic performance [ F ( 3 , 446 ) = 36.914; p < 0.001], explaining almost 20% of the variance ( R 2 = 0.194) in the criterion variable.
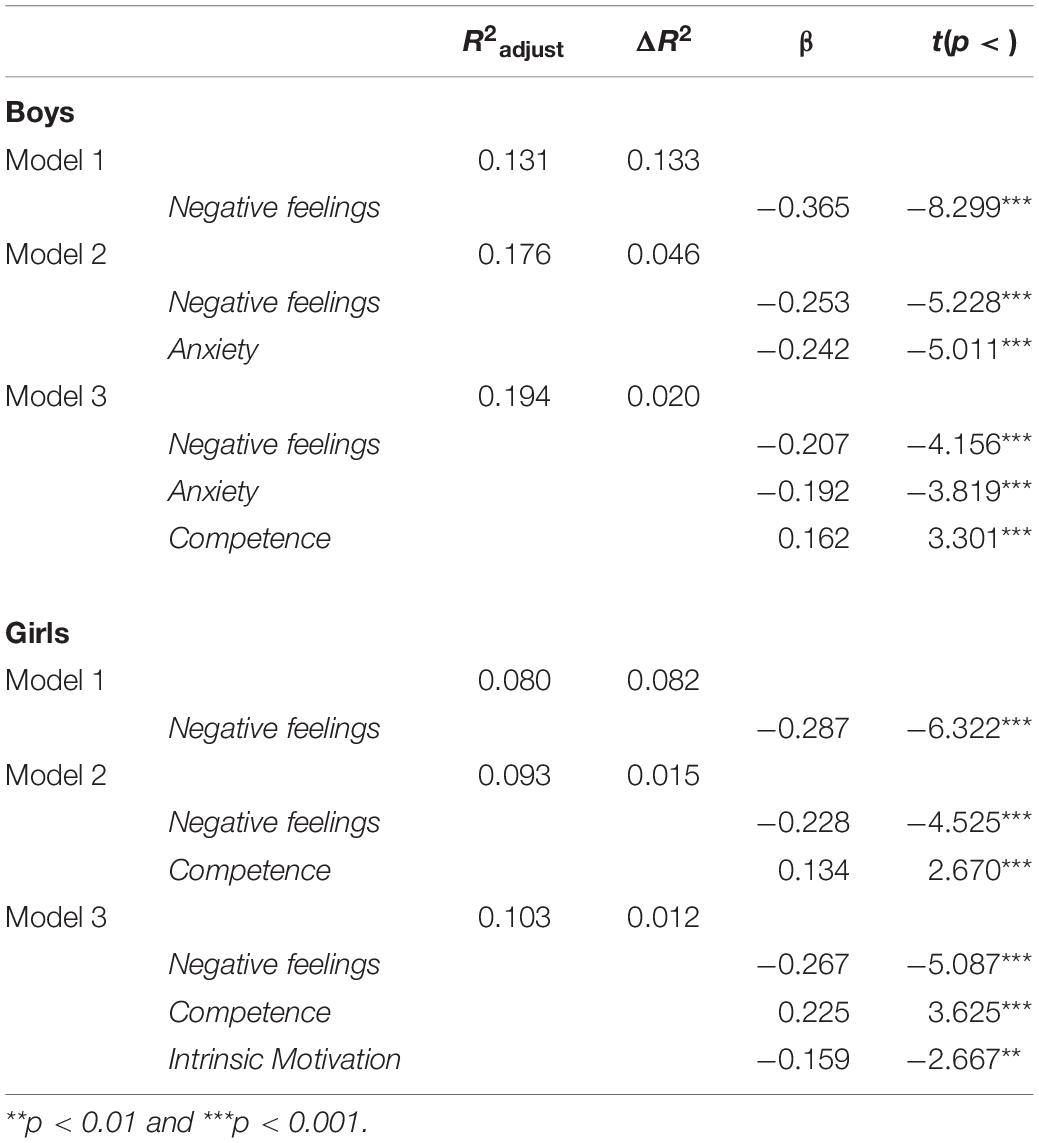
Table 2. Explained variance ( R 2 ), change in R 2 (Δ R 2 ), regression coefficients ( β ), and associated statistical significance [ t ( p <)] in the prediction of boys’ and girls’ mathematics performance.
In the girls’ group, intrinsic motivation, negative feelings toward mathematics, and perceived competence in the subject were also predictor variables of mathematics performance [ F ( 3 , 443 ) = 18.093; p < 0.001], although in this case the percentage of explained variance was half of that in the boys’ sample ( R 2 = 0.103).
The results of the analyses confirm the explanatory potential of both negative feelings toward mathematics (β = −0.207; t = −4.156; p < 0.001 for the boys, and β = −0.267; t = −5.087; p < 0.001 for the girls) and perceived competence (β = 0.162 ; t = 3.301; p < 0.001 for the boys, and β = 0.225; t = 3.625; p < 0.001 for the girls) in mathematics performance (see Table 2 ). In both samples, negative feelings were associated with poor mathematics performance, whereas the positive regression coefficient for perceived competence in the subject indicates, as previous research has noted, the positive link between these types of beliefs and performance.
The results also indicate some differential aspects that should be underscored. Firstly, the contribution of math anxiety was only an explanatory factor for the boys (β = −0.192; t = −3.819; p < 0.001), whereas intrinsic motivation appeared as a significant variable in the explanation of girls’ performance (β = −0.159; t = −2.667; p < 0.05). The negative regression coefficient of this variable indicates that, for girls, commitment to mathematics for intrinsic reasons may negatively affect their performance in this subject. We discuss this result in light of Pekrun’s (2006) control-value theory.
Discussion and Conclusion
In line with previous research ( Hyde et al., 1990 ; Else-Quest et al., 2010 ; Goetz et al., 2013 ; Bieg et al., 2015 ), we confirm that girls in the 5th and 6th years of primary education report higher levels of math anxiety than do boys, in this case, with moderate effect sizes. However, these gender differences in math anxiety may not be transferable to other negative feelings or emotions such as sadness or boredom, as previous studies have suggested ( Hyde et al., 1990 ; Nagy et al., 2008 ; Goetz and Hall, 2013 ; Goetz et al., 2013 ; Bieg et al., 2015 ). Our results also replicate prior studies, showing that boys report greater intrinsic motivation and perceived competence for mathematics than do girls ( Fredricks and Eccles, 2002 ; Jacobs et al., 2002 ; Preckel et al., 2008 ; Else-Quest et al., 2010 ; Frenzel et al., 2010 ; Louis and Mistele, 2012 ; Guo et al., 2015 ; Ganley and Lubienski, 2016 ), even though their performance in mathematics is similar ( Skaalvik and Skaalvik, 2004 ; Else-Quest et al., 2010 ; Lindberg et al., 2010 ).
In general terms, as expected, our results confirm the well-known positive impact of perceived self-efficacy on mathematics performance at school ( Zimmerman, 1995 ; Pajares and Graham, 1999 ; Valentine et al., 2004 ; Fast et al., 2010 ; Williams and Williams, 2010 ; Parker et al., 2014 ), found in both standardized tests and, as in our case, the grades awarded in class ( Randhawa et al., 1993 ; Fast et al., 2010 ; Grigg et al., 2018 ).
In addition, as various perspectives have suggested, our results highlight the importance and influence of students’ emotions on their learning and performance. The explanatory power of negative emotions associated with mathematics has been shown to be even more important than the oft-quoted math anxiety when it comes to explaining performance in this subject. Research on emotions in mathematics has repeatedly focused on math anxiety, very probably ignoring a good number of different emotions such as sadness and boredom, which as “hot cognition,” may mark the path of learning and success. Even though studies indicate that the relationships between emotions and performance generally tend to have small or moderate effect sizes, it is possible that academic emotions end up having a significant cumulative long-term impact on performance. For this reason, we should not lose sight of their role in health, subjective well-being, choice and continuation of study, or in lifelong learning ( Pekrun, 2006 ).
The differences in percentages of variance in academic performance explained by the motivational variables that we examined in boys and girls indicate the need to encourage research into gender differences in academic emotions and motivations in general and in mathematics in particular. Whereas negative feelings, anxiety, and perceived competence together explain almost 20% of the variance in boys’ performance in mathematics, the explanatory power of negative feelings, competence, and intrinsic motivation in girls is practically half of that. Producing a body of knowledge that would let us characterize differential motivational profiles, in contexts of achievement, would allow better adjustment of affective-motivational interventions. The gender gap in cognitive abilities could be reduced in the long term with the promotion of specific educational experiences in these first stages of formal education ( Ganley and Lubienski, 2016 ).
In this study, the performance of the boys, who were more confident in their abilities, more motivated, and more interested in mathematics, was seen to diminish in relation to the appearance of negative feelings about mathematics, and there were high rates of anxiety. Nevertheless, and in line with observations by Goetz et al. (2013) , our results seem to demonstrate that although girls reported higher levels of anxiety, their performance in mathematics tasks and tests was not affected as negatively as might have been expected. The state-trait discrepancy that research recognizes in these and other academic emotions (e.g., Porter et al., 2000 ; Frenzel et al., 2007 ; Bieg et al., 2013 , 2014 ) suggests that gender differences found in math anxiety may not be reflected in day-to-day school learning processes. Emotional valuations may be strongly influenced by subjective beliefs such as expectations or attributional tendencies. The commonly accepted idea that in mathematics girls believe themselves to be less competent may be behind an erroneous anticipatory evaluation of their math anxiety (e.g., Skaalvik and Skaalvik, 2004 ; Ganley and Lubienski, 2016 ). These girls’ beliefs about their competence in mathematics, together with gender stereotypes ( Wigfield and Eccles, 2000 ; Pekrun, 2006 ; Thoman et al., 2013 ; Bieg et al., 2015 ), contribute to an emotional evaluation that differs from the emotions really associated with the specific tasks or situations. This is why despite girls exhibiting higher levels of anxiety about mathematics than do boys, this variable is not an explanatory factor in girls’ performance.
With respect to the premises of the control-value theory, negative feelings such as sadness, unhappiness, and despair associated with mathematics tasks are surely the result of a pattern of low control with high value placed on success ( Frenzel et al., 2007 ; Bieg et al., 2015 ). The inclusion of intrinsic motivation as a negative predictor of girls’ mathematics performance must be understood in this context, characterized by low perceived competence for the subject. Just as Pekrun’s (2006) theory determines, if negative emotions are the result of a pattern of low control together with high value placed on success, involvement for intrinsic reasons, indicating high value placed on mathematics, could end up decreasing girls’ performance inasmuch as it could contribute to negative feelings in this area.
Educational Implications
In terms of the potential of emotions associated with the classroom, it is worth suggesting longitudinal, experimental, and interventional research to examine the assumed causal relationships between discrete emotions, both negative (as we have done in this study) and positive (enjoyment, hope, and gratitude among others), and performance. Apart from incorporating knowledge about moderating and mediating variables of academic emotions and their background into the teacher training syllabus, teachers should also consider the impact of educational styles on their students’ academic emotions, and their role in encouraging positive emotions and reducing negative emotions associated with the classroom.
In terms of our results, and in line with Ganley and Lubienski (2016) , mathematics interventions for girls should start early and deal specifically with perceptions of confidence and control. On the other hand, management of emotions, particularly anxiety, could be extremely important for mathematics interventions for boys. The identification and development of instruction strategies and intervention plans to improve the affective-emotional experiences associated with the learning process should be on the educational and research agenda.
Limitations
Apart from the inherent limitations of the research design, we must recognize the use of a self-report to measure emotions and the fact that boys and girls surely differ in their abilities and dispositions when reporting their emotions ( Bryant et al., 1996 ). Information about emotions may be especially vulnerable to social desirability or to stereotyping in a context such as mathematics, which is perceived as a male domain.
Data Availability Statement
The datasets generated for this study are available on request to the corresponding author.
Ethics Statement
The studies involving human participants were reviewed and approved by Ethics Committee of University of A Coruña. Written informed consent to participate in this study was provided by the participants’ legal guardian/next of kin.
Author Contributions
SR and IE contributed to collect the data, data analysis, and writing the manuscript. BR and AV contributed to data analysis and writing the manuscript. IP wrote the manuscript.
This work was carried out with financing from the research projects EDU2013-44062-P (MINECO) and EDU2017-82984-P (MEIC).
Conflict of Interest
The authors declare that the research was conducted in the absence of any commercial or financial relationships that could be construed as a potential conflict of interest.
Ashcraft, M. H. (2002). Math anxiety: personal, educational, and cognitive consequences. Curr. Dir. Psychol. Sci. 11, 181–185. doi: 10.1111/1467-8721.00196
CrossRef Full Text | Google Scholar
Ashcraft, M. H., and Ridley, K. S. (2005). “Math anxiety and its cognitive consequences,” in Handbook of Mathematical Cognition , ed. J. I. D. Campbell, (New York, NY: Psychology Press), 315–327.
Google Scholar
Aunola, K., Leskinen, E., and Nurmi, J. E. (2006). Developmental dynamics between mathematical performance, task motivation, and teachers’ goals during the transition to primary school. Br. J. Educ. Psychol. 76, 21–40. doi: 10.1348/000709905X51608
PubMed Abstract | CrossRef Full Text | Google Scholar
Bieg, M., Goetz, T., and Hubbard, K. (2013). Can I master it and does it matter? An intraindividual analysis on control–value antecedents of trait and state academic emotions. Learn. Individ. Differ. 28, 102–108. doi: 10.1016/j.lindif.2013.09.006
Bieg, M., Goetz, T., and Lipnevich, A. A. (2014). What students think they feel differs from what they really feel–academic self-concept moderates the discrepancy between students’ trait and state emotional self-reports. PLos One 9:e92563. doi: 10.1371/journal.pone.0092563
Bieg, M., Goetz, T., Wolter, I., and Hall, N. C. (2015). Gender stereotype endorsement differentially predicts girls’ and boys’ trait-state discrepancy in math anxiety. Front. Psychol. 6:1404. doi: 10.3389/fpsyg.2015.01404
Bryant, F. B., Yarnold, P. R., and Grimm, L. G. (1996). Toward a measurement model of the affect intensity measure: a three-factor structure. J. Res. Pers. 30, 223–247. doi: 10.1006/jrpe.1996.0015
Ceci, S. J., and Williams, W. M. (2011). Understanding current causes of women’s underrepresentation in science. Proc. Natl. Acad. Sci. 108, 3157–3162. doi: 10.1073/pnas.1014871108
Cohen, J. (1988). Statistical Power Analysis for the Behavioural Sciences. New Jersey: Lawrence Erlbaum.
Colbeck, C. L., Cabrera, A. F., and Terenzini, P. T. (2001). Learning professional confidence: linking teaching practices, students’ self-perceptions, and gender. Rev. High. Educ. 24, 173–191. doi: 10.1353/rhe.2000.0028
Cueli, M., González-Castro, P., Álvarez, L., García, T., and González-Pienda, J. A. (2014). Variables afectivo-motivacionales y rendimiento en matemáticas: un análisis bidireccional. Rev. Mex. Psicol. 31, 153–163.
Denissen, J. J. A., Zarrett, N. R., and Eccles, J. S. (2007). I like to do it, I’m able, and I know I am: longitudinal couplings between domain-specific achievement, selfconcept, and interest. Child Dev. 78, 430–447. doi: 10.1111/j.1467-8624.2007.01007.x
Eccles, J., Wigfield, A., Harold, R. D., and Blumenfeld, P. (1993). Age and gender differences in children’s self-and task perceptions during elementary school. Child Dev. 64, 830–847. doi: 10.1111/j.1467-8624.1993.tb02946.x
Else-Quest, N. M., Hyde, J. S., and Linn, M. C. (2010). Cross-national patterns of gender differences in mathematics: a meta-analysis. Psychol. Bull. 136, 103–127. doi: 10.1037/a0018053
Fast, L. A., Lewis, J. L., Bryant, M. J., Bocian, K. A., Cardullo, R. A., Rettig, M., et al. (2010). Does math self-efficacy mediate the effect of the perceived classroom environment on standardized math test performance? J. Educ. Psychol. 102, 729–740. doi: 10.1037/a0018863
Fennema, E., and Sherman, J. A. (1976). Fennema-sherman mathematics attitudes scales: instruments designed to measure attitudes toward the learning of mathematics by females and males. J. Res. Math. Educ. 7, 324–326. doi: 10.2307/748467
Fredricks, J. A., and Eccles, J. S. (2002). Children’s competence and value beliefs from childhood through adolescence: growth trajectories in two male-sex-typed domains. Dev. Psychol. 38, 519–533. doi: 10.1037/0012-1649.38.4.519
Frenzel, A. C., Goetz, T., Pekrun, R., and Watt, H. M. (2010). Development of mathematics interest in adolescence: influences of gender, family, and school context. J. Res. Adolesc 20, 507–537. doi: 10.1111/j.1532-7795
Frenzel, A. C., Pekrun, R., and Goetz, T. (2007). Perceived learning environment and students’ emotional experiences: a multilevel analysis of mathematics classrooms. Learn. Instr. 17, 478–493. doi: 10.1016/j.learninstruc.2007.09.001
Ganley, C. M., and Lubienski, S. T. (2016). Mathematics confidence, interest, and performance: examining gender patterns and reciprocal relations. Learn. Individ. Differ. 47, 182–193. doi: 10.1016/j.lindif.2016.01.002
Goetz, T., Bieg, M., Lüdtke, O., Pekrun, R., and Hall, N. C. (2013). Do girls really experience more anxiety in mathematics? Psychol. Sci. 24, 2079–2287. doi: 10.1177/0956797613486989
Goetz, T., and Hall, N. C. (2013). “Emotion and achievement in the classroom,” in International Guide to Student Achievement , eds J. Hattie and E. M. Anderman, (New York, NY: Routledge), 192–195.
González-Pienda, J. A., Fernández–Cueli, M., García, T., Suárez, N., Fernández, E., Tuero–Herrero, E., et al. (2012). Diferencias de género en actitudes hacia las matemáticas en la enseñanza obligatoria. Rev. Iberoam. Psicol. Salud. 3, 55–73.
Grigg, S., Perera, H. N., McIlveen, P., and Svetleff, Z. (2018). Relations among math self-efficacy, interest, intentions, and achievement: a social cognitive perspective. Contemp. Educ. Psychol. 53, 73–86. doi: 10.1016/j.cedpsych.2018.01.007
Griggs, M. S., Rimm-Kaufman, S. E., Merritt, E. G., and Patton, C. L. (2013). The responsive classroom approach and fifth grade students’ math and science anxiety and self-efficacy. Sch. Psychol. Q. 28, 360–373. doi: 10.1037/spq0000026
Guo, J., Parker, P. D., Marsh, H. W., and Morin, A. J. (2015). Achievement, motivation, and educational choices: a longitudinal study of expectancy and value using a multiplicative perspective. Dev. Psychol. 51, 1163–1176. doi: 10.1037/a0039440
Hedges, L. V., and Nowell, A. (1995). Sex differences in mental test scores, variability, and numbers of high-scoring individuals. Science 269, 41–45. doi: 10.1126/science.7604277
Hidi, S., and Renninger, K. A. (2006). The four-phase model of interest development. Educ. Psychol. 41, 111–127. doi: 10.1207/s15326985ep4102_4
Hyde, J. S. (2014). Gender similarities and differences. Annu. Rev. Psychol. 65, 373–398. doi: 10.1146/annurev-psych-010213-115057
Hyde, J. S., Fennema, E., Ryan, M., Frost, L. A., and Hopp, C. (1990). Gender comparisons of mathematics attitudes and affect: a meta-analysis. Psychol. Women Q. 14, 299–324. doi: 10.1111/j.1471-6402.1990.tb00022.x
Hyde, J. S., Lindberg, S. M., Linn, M. C., Ellis, A. B., and Williams, C. C. (2008). Gender similarities characterize math performance. Science 321, 494–495. doi: 10.1126/science.1160364
Hyde, J. S., and Mertz, J. E. (2009). Gender, culture, and mathematics performance. Proc. Natl. Acad. Sci. 106, 8801–8807. doi: 10.1073/pnas.0901265106
Jacobs, J. E., Lanza, S., Osgood, D. W., Eccles, J. S., and Wigfield, A. (2002). Changes in children’s self-competence and values: gender and domain differences across grades one through twelve. Child Dev. 73, 509–527. doi: 10.1111/1467-8624.00421
Jansen, M., Lüdtke, O., and Schroeders, U. (2016). Evidence for a positive relation between interest and achievement: examining between-person and within-person variation in five domains. Contemp. Educ. Psychol. 46, 116–127. doi: 10.1016/j.cedpsych.2016.05.004
Kanny, M. A., Sax, L. J., and Riggers-Piehl, T. A. (2014). Investigating forty years of STEM research: how explanations for the gender gap have evolved over time. J. Women Minor. Sci. Eng. 20, 127–148. doi: 10.1615/JWomenMinorScienEng.2014007246
Köller, O., Baumert, J., and Schnabel, K. (2001). Does interest matter? The relationship between academic interest and achievement in mathematics. J. Res. Math. Educ. 32, 448–470. doi: 10.2307/749801
Kurtz-Costes, B., Rowley, S. J., Harris-Britt, A., and Woods, T. A. (2008). Gender stereotypes about mathematics and science and self-perceptions of ability in late childhood and early adolescence. MerrillPalmer. Quarterl. 54, 386–409. doi: 10.1007/s10212-014-0216-7
Larivière, V., Ni, C., Gingras, Y., Cronin, B., and Sugimoto, C. R. (2013). Bibliometrics: global gender disparities in science. Nat. News 504, 211–213. doi: 10.1038/504211a
Lee, W., Lee, M. J., and Bong, M. (2014). Testing interest and self-efficacy as predictors of academic self-regulation and achievement. Contemp. Educ. Psychol. 39, 86–99. doi: 10.1016/j.cedpsych.2014.02.002
Legewie, J., and DiPrete, T. A. (2014). The high school environment and the gender gap in science and engineering. Soc. Educ. 87, 259–280. doi: 10.1177/0038040714547770
Lepper, M. R., Henderlong Corpus, J., and Iyengar, S. S. (2005). Intrinsic and extrinsic motivational orientations in the classroom: age differences and academic correlates. J. Educ. Psychol. 97, 184–196. doi: 10.1037/0022-0663.97.2.184
Lindberg, S. M., Hyde, J. S., Petersen, J. L., and Linn, M. C. (2010). New trends in gender and mathematics performance: a meta-analysis. Psychol. Bull. 136, 1123–1135. doi: 10.1037/a0021276
Louis, R. A., and Mistele, J. M. (2012). The differences in scores and self-efficacy by student gender in mathematics and science. Int. J. Sci. Math. Educ. 10, 1163–1190. doi: 10.1007/s10763-011-9325-9
Lyons, I. M., and Beilock, S. L. (2012). When math hurts: math anxiety predicts pain network activation in anticipation of doing math. PLos One 7:e48076. doi: 10.1371/journal.pone.0048076
Ministerio de Educación Cultura y Deporte [MECD] (2016). PISA 2015. Programa Para la Evaluación Internacional de los Alumnos. Informe Español. Madrid: Ministerio de Educación, Cultura y Deporte..
Mullis, I. V., Martin, M. O., Foy, P., and Arora, A. (2012). TIMSS 2011 International Results in Mathematics. Amsterdam: International Association for the Evaluation of Educational Achievement.
Nagy, G., Garrett, J., Trautwein, U., Cortina, K. S., Baumert, J., and Eccles, J. (2008). “Gendered high school course selection as a precursor of gendered occupational careers: The mediating role of self-concept and intrinsic value,” in Gendered Occupational Outcomes: Longitudinal Assessments of Individual, Social, and Cultural Influences , eds H. M. G. Watt and J. S. Eccles, (Washington, DC: American Psychological Association), 115–143. doi: 10.1037/11706-004
Pajares, F., and Graham, L. (1999). Self-efficacy, motivation constructs, and mathematics performance of entering middle school students. Contemp. Educ. Psychol. 24, 124–139. doi: 10.1006/ceps.1998.0991
Parker, P. D., Marsh, H. W., Ciarrochi, J., Marshall, S., and Abduljabbar, A. S. (2014). Juxtaposing math self-efficacy and self-concept as predictors of long-term achievement outcomes. Educ. Psychol. 34, 29–48. doi: 10.1080/01443410.2013.797339
Pekrun, R. (2006). The control-value theory of achievement emotions: assumptions, corollaries, and implications for educational research and practice. Educ. Psychol. Rev. 18, 315–341. doi: 10.1007/s10648-006-9029-9
Pekrun, R., Hall, N. C., Goetz, T., and Perry, R. P. (2014). Boredom and academic achievement: testing a model of reciprocal causation. J. Educ. Psychol. 106, 696–710. doi: 10.1037/a0036006
Pekrun, R., Lichtenfeld, S., Marsh, H. W., Murayama, K., and Goetz, T. (2017). Achievement emotions and academic performance: longitudinal models of reciprocal effects. Child. Dev. 88, 1653–1670. doi: 10.1111/cdev.12704
Pekrun, R., and Linnenbrink-Garcia, L. (eds) (2014). International Handbook of Emotions in Education. New York, NY: Taylor & Francis.
Pinxten, M., Marsh, H. W., De Fraine, B., Van Den Noortgate, W., and Van Damme, J. (2014). Enjoying mathematics or feeling competent in mathematics? Reciprocal effects on mathematics achievement and perceived math effort expenditure. Br. J. Educ. Psychol. 84, 152–174. doi: 10.1111/bjep.12028
Porter, L. S., Marco, C. A., Schwartz, J. E., Neale, J. M., Shiffman, S., and Stone, A. A. (2000). Gender differences in coping: a comparison of trait and momentary assessments. J. Soc. Clin. Psychol. 19, 480–498. doi: 10.1521/jscp.2000.19.4.480
Preckel, F., Goetz, T., Pekrun, R., and Kleine, M. (2008). Gender differences in gifted and average-ability students: comparing girls’ and boys’ achievement, self-concept, interest, and motivation in mathematics. Gift. Child. Q. 52, 146–159. doi: 10.1177/0016986208315834
Putwain, D. W., Becker, S., Symes, W., and Pekrun, R. (2018). Reciprocal relations between students’ academic enjoyment, boredom, and achievement over time. Learn. Instr. 54, 73–81. doi: 10.1016/j.learninstruc.2017.08.004
Ramirez, G., Chang, H., Maloney, E. A., Levine, S. C., and Beilock, S. L. (2016). On the relationship between math anxiety and math achievement in early elementary school: the role of problem solving strategies. J. Exp. Child. Psychol. 141, 83–100. doi: 10.1016/j.jecp.2015.07.014
Randhawa, B. S., Beamer, J. E., and Lundberg, I. (1993). Role of mathematics self-efficacy in the structural model of mathematics achievement. J. Educ. Psychol. 85, 41–48. doi: 10.1037/0022-0663.85.1.41
Robinson, J. P., and Lubienski, S. T. (2011). The development of gender achievement gaps in mathematics and reading during elementary and middle school: examining direct cognitive assessments and teacher ratings. Am. Educ. Res. J. 48, 268–302. doi: 10.3102/0002831210372249
Sadler, P. M., Sonnert, G., Hazari, Z., and Tai, R. (2012). Stability and volatility of STEM career interest in high school: a gender study. Sci. Educ. 96, 411–427. doi: 10.1002/sce.21007
Silva, E. H. (2005). Actitudes Hacia el Aprendizaje de las Matemáticas. Tesis Doctoral no publicada, Universidad de Oviedo, Oviedo.
Skaalvik, E. M., and Skaalvik, S. (2004). Self-concept and self-efficacy: A test of the internal/external frame of reference model and predictions of subsequent motivation and achievement. Psychol. Rep. 95, 1187–1202. doi: 10.2466/pr0.95.3f.1187-1202
Stevens, T., Olivarez, A., and Hamman, D. (2006). The role of cognition, motivation, and emotion in explaining the mathematics achievement gap between Hispanic and white students. Hispanic J. Behav. Sci. 28, 161–186. doi: 10.1177/0739986305286103
Thoman, D. B., Smith, J. L., Brown, E. R., Chase, J., and Lee, J. Y. K. (2013). Beyond performance: a motivational experiences model of stereotype threat. Educ. Psychol. Rev. 25, 211–243. doi: 10.1007/s10648-013-9219-1
Valentine, J., DuBois, D., and Cooper, H. (2004). The relation between self-beliefs and academic achievement: a meta-analytic review. Educ. Psychol. 39, 111–133. doi: 10.1207/s15326985ep3902_3
Viljaranta, J., Lerkkanen, M. K., Poikkeus, A. M., Aunola, K., and Nurmi, J. E. (2009). Cross-lagged relations between task motivation and performance in arithmetic and literacy in kindergarten. Learn. Instr. 19, 335–344. doi: 10.1016/j.learninstruc.2008.06.011
Wang, M. T., and Degol, J. L. (2017). Gender gap in science, technology, engineering, and mathematics (STEM): current knowledge, implications for practice, policy, and future directions. Educ. Psychol. Rev. 29, 119–140. doi: 10.1007/s10648-015-9355-x
Wigfield, A., Eccles, J., Mac Iver, D., Reuman, D., and Midgley, C. (1991). Transitions at early adolescence: changes in children’s domain-specific self-perceptions and general selfesteem across the transition to junior high school. Dev. Psychol. 27, 552–565. doi: 10.1037/0012-1649.27.4.552
Wigfield, A., and Eccles, J. S. (2000). Expectancy-value theory ofachievement motivation. Contemp. Educ. Psychol. 25, 68–81. doi: 10.1006/ceps.1999.1015
Wigfield, A., Eccles, J. S., Yoon, K. S., Harold, R. D., Arbreton, A., Freedman-Doan, K., et al. (1997). Changes in children’s competence beliefs and subjective task values across the elementary school years: a three-year study. J. Educ. Psychol. 89, 451–469. doi: 10.1037/0022-0663.89.3.451
Williams, T., and Williams, K. (2010). Self-efficacy and performance in mathematics: reciprocal determinism in 33 nations. J. Educ. Psychol. 102, 453–466. doi: 10.1037/a0017271
Yoon, K. S., Eccles, J. S., and Wigfield, A. (1996). “Self-concept of ability, value, and academic achievement: a test of causal relations,” in Annual Meeting of the American Educational Research Association , (New York, NY).
Yüksel-şahin, F. (2008). Mathematics anxiety among 4th and 5th grade Turkish elementary school students. Int. Electron. J. Math. Educ. 3, 179–192.
Zimmerman, B. J. (1995). “Self-efficacy and educational development,” in Self-Efficacy in Changing Societies , eds A. Bandura (New York, NY: Cambridge University Press), 202–231. doi: 10.1017/CBO9780511527692.009
Keywords : gender differences, mathematics, academic motivation, academic performance, primary education
Citation: Rodríguez S, Regueiro B, Piñeiro I, Estévez I and Valle A (2020) Gender Differences in Mathematics Motivation: Differential Effects on Performance in Primary Education. Front. Psychol. 10:3050. doi: 10.3389/fpsyg.2019.03050
Received: 18 October 2019; Accepted: 23 December 2019; Published: 29 January 2020.
Reviewed by:
Copyright © 2020 Rodríguez, Regueiro, Piñeiro, Estévez and Valle. This is an open-access article distributed under the terms of the Creative Commons Attribution License (CC BY) . The use, distribution or reproduction in other forums is permitted, provided the original author(s) and the copyright owner(s) are credited and that the original publication in this journal is cited, in accordance with accepted academic practice. No use, distribution or reproduction is permitted which does not comply with these terms.
*Correspondence: Susana Rodríguez, [email protected]
Disclaimer: All claims expressed in this article are solely those of the authors and do not necessarily represent those of their affiliated organizations, or those of the publisher, the editors and the reviewers. Any product that may be evaluated in this article or claim that may be made by its manufacturer is not guaranteed or endorsed by the publisher.
Advertisement
K-8 Teachers’ Overall and Gender-Specific Beliefs About Mathematical Aptitude
- Published: 11 July 2020
- Volume 19 , pages 1251–1269, ( 2021 )
Cite this article
- Yasemin Copur-Gencturk ORCID: orcid.org/0000-0001-8819-3035 1 ,
- Ian Thacker 2 &
- David Quinn 1
1303 Accesses
11 Citations
33 Altmetric
Explore all metrics
Teachers’ beliefs play a significant role in students’ academic attainment and career choices. Despite comparable attainment levels between genders, persistent stereotypes and beliefs that certain disciplines require innate ability and that men and women have different ability levels impede students’ academic career paths. In this study, we examined the prevalence of US mathematics teachers’ explicit general and gender-specific beliefs about mathematical ability and identified which teacher characteristics were associated with these beliefs. An analysis of data from 382 K-8 teachers in the USA indicated that overall, teachers disagreed with the idea that general and gender-specific mathematical ability is innate and agreed with the idea that hard work and dedication are required for success in mathematics. However, our findings indicate that those who believed mathematics requires brilliance also tended to think girls lacked this ability. We also found that teachers who were teaching mathematics to 11- to 14-year-old students seemed to believe that mathematics requires innate ability compared with teachers who were teaching mathematics to 5- to 10-year-old students. In addition, more experienced teachers and teachers who worked with special education students seemed to believe less in the role of hard work in success in mathematics, which could have serious consequences for shaping their students’ beliefs about their academic self-concept and future career-related decisions.
This is a preview of subscription content, log in via an institution to check access.
Access this article
Price includes VAT (Russian Federation)
Instant access to the full article PDF.
Rent this article via DeepDyve
Institutional subscriptions
Similar content being viewed by others
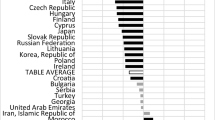
Gender Differences in Mathematics Self-concept Across the World: an Exploration of Student and Parent Data of TIMSS 2015
Investigating gender differences in mathematics and science: results from the 2011 trends in mathematics and science survey.
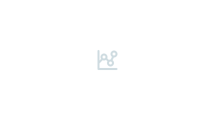
Math Self-efficacy and the Likelihood of Pursuing a STEM-Based Career: a Gender-Based Analysis
We use the term “ability” to be consistent with the literature on “field-specific ability beliefs” (see, e.g., Leslie et al., 2015 ) which uses the term “ability” synonymously with terms such as “natural gift,” “talent,” or “brilliance.”
The term attainment refers to education levels that are reached as set by given standard benchmarks (e.g., grades, standardized test scores, educational credentials), a concept that differs notably from the nebulous idea of innate mathematical ability.
This evidence is based on graduation data from the UNESCO website ( http://data.uis.unesco.org ) under “Distribution of Tertiary Graduates.”
In total, 664 teachers received an e-mail for the survey, and 434 completed our items.
Note that coefficients of internal consistency tend to increase as the number of items goes up; thus, our scales consisting of two items might be expected to have a lower value for alpha than what is conventionally accepted (e.g., Nunnally & Bernstein, 1994 ).
Acker, J. (2006). Inequality regimes. Gender, class, and race in organizations. Gender & Society, 20 (4), 441–464.
Article Google Scholar
Ambady, N., Shih, M., Kim, A., & Pittinsky, T. L. (2001). Stereotype susceptibility in children: Effects of identity activation on quantitative performance. Psychological Science, 12 , 385–390.
Bargh, J. A. (1994). The four horsemen of automaticity: Awareness, intention, efficiency, and control in social cognition. In R. S. Wyer & T. K. Srull (Eds.), Handbook of social cognition , Basic processes (Vol. 1, pp. 1–40). Hillsdale, NJ: Lawrence Erlbaum Associates.
Google Scholar
Bennett, C. (2011). Beyond the leaky pipeline: Consolidating understanding and incorporating new research about women’s science careers in the UK. Business and Economic Review, 54 , 149–176.
Bjerrum Nielsen, H. (2003). One of the boys? Doing gender in scouting . Génève, Sweden: World Organization of the Scout Movement.
Boaler, J. (1997). Reclaiming school mathematics: The girls fight back. Gender and Education, 9 (3), 285–305.
Boaler, J. (2013). Ability and mathematics: The mindset revolution that is reshaping education. Forum, 55 (1), 143–152.
Bolzendahl, C. I., & Myers, D. J. (2004). Feminist attitudes and support for gender equality: Opinion change in women and men, 1974–1998. Social Forces, 83 (2), 759–789.
Bong, M., & Skaalvik, E. M. (2003). Academic self-concept and self-efficacy: How different are they really? Educational Psychology Review, 15 (1), 1–40.
Brandell, G., Leder, G., & Nyström, P. (2007). Gender and mathematics: Recent development from a Swedish perspective. ZDM, 39 (3), 235–250.
Brophy, J. E., & Good, T. L. (1970). Teachers' communication of differential expectations for children's classroom performance: Some behavioral data. Journal of Educational Psychology, 61 (5), 365–374.
Ceci, S. J., Williams, W. M., & Barnett, S. M. (2009). Women’s underrepresentation in science: Sociocultural and biological considerations. Psychological Bulletin, 135 (2), 218–261.
Chrysostomou, M., & Philippou, G. N. (2010). Teachers’ epistemological beliefs and efficacy beliefs about mathematics. Procedia-Social and Behavioral Sciences, 9 , 1509–1515.
Cimpian, J. R., Lubienski, S. T., Timmer, J. D., Makowski, M. B., & Miller, E. K. (2016). Have gender gaps in math closed? Achievement, teacher perceptions, and learning behaviors across two ECLS-K cohorts. AERA Open, 2 (4), 2332858416673617.
Copur-Gencturk, Y., Cimpian, J. R., Lubienski, S. T., & Thacker, I. (2020). Teachers’ bias against the mathematical ability of female, black, and Hispanic Students. Educational Researcher, 49 (1), 30–43. https://doi.org/10.3102/0013189X19890577 .
Cooney, T. J. (1985). A beginning teacher’s view of problem solving. Journal for Research in Mathematics Education, 16 , 324–336.
Correll, S. J. (2001). Gender and the career choice process: The role of biased self-assessments. American Journal of Sociology, 106 (6), 1691–1730.
Debellis, V. A., & Goldin, G. A. (2006). Affect and meta-affect in mathematical problem solving: A representational perspective. Educational Studies in Mathematics, 63 (2), 131–147.
Depaepe, F., DeCorte, E., & Verschaffel, L. (2016). Mathematical epistemological beliefs. In J. A. Greene, W. A. Sandoval, & I. Bråten (Eds.), Handbook of epistemic cognition (pp. 147–164). New York, NY: Routledge.
Di Martino, P., & Zan, R. (2015). The construct of attitude in mathematics education. In B. Pepin & B. Roesken-Winter (Eds.), From beliefs to dynamic affect systems in mathematics education (pp. 51–72). New York, NY: Springer.
Dweck, C. (1986). Motivational processes affecting learning. American Psychologist, 41 , 1040–1048.
Dweck, C. S. (2006). Is math a gift? Beliefs that put females at risk. In S. J. Ceci & W. Williams (Eds.), Why Aren’t more women in science? Top researchers debate the evidence . Washington, DC: American Psychological Association.
Dweck, C. S. (2008). Mindsets and math/science achievement . New York, NY: Carnegie Corp. of New York, Institute for Advanced Study, Commission on Mathematics and Science Education.
Ercikan, K., McCreith, T., & Lapointe, V. (2005). Factors associated with mathematics achievement and participation in advanced mathematics courses: An examination of gender differences from an international perspective. School Science and Mathematics, 105 (1), 5–14.
Ernest, P. (1989). The impact of beliefs on the teaching of mathematics. In C. Keitel, P. Damerow, A. Bishop, & P. Gerdes (Eds.), Mathematics, education, and society (pp. 99–101). Paris, France: UNESCO.
Eynde, P. O., de Corte, E., & Verschaffel, L. (2003). Framing students’ mathematics-related beliefs. A quest for conceptual clarity and a comprehensive categorization. In G. C. Leder, E. Pehkonen, & G. Torner (Eds.), Beliefs: A hidden variable in mathematics education? (pp. 13–38). Dordrecht, The Netherlands: Kluwer Academic Publishers.
Fennema, E., Peterson, P. L., Carpenter, T. P., & Lubinski, C. A. (1990). Teachers' attributions and beliefs about girls, boys, and mathematics. Educational Studies in Mathematics, 21 (1), 55–69.
Furnham, A., Hosoe, T., & Tang, T. (2002). Male hubris and female humility a cross-cultural study of ratings of self, parental, and sibling multiple intelligence in America, Britain, and Japan. Intelligence, 30 , 101–115.
Geary, D. C. (1995). Sexual selection and sex differences in spatial cognition. Learning and Individual Differences, 7 (4), 289–301.
Geary, D. C., Saults, S. J., Liu, F., & Hoard, M. K. (2000). Sex differences in spatial cognition, computational fluency, and arithmetical reasoning. Journal of Experimental Child Psychology, 77 (4), 337–353.
Good, C., Rattan, A., & Dweck, C. S. (2012). Why do women opt out? Sense of belonging and women’s representation in mathematics. Journal of Personality and Social Psychology, 102 (4), 700–717.
Greenwald, A. G., & Banaji, M. R. (1995). Implicit social cognition: Attitudes, self-esteem, and stereotypes. Psychological Review, 102 (1), 4–27.
Greenwald, A. G., Rudman, L. A., Nosek, B. A., Banaji, M. R., Farnham, S. D., & Mellott, D. S. (2002). A unified theory of implicit attitudes, stereotypes, self-esteem, and self-concept. Psychological Review, 109 (1), 3–25.
Handal, B. (2003). Teachers' mathematical beliefs: A review. The Mathematics Educator, 13 (2), 47–57.
Hannula, M. S. (2012). Exploring new dimensions of mathematics-related affect: Embodied and social theories. Research in Mathematics Education, 14 (2), 137–161.
Husain, M., & Millimet, D. L. (2009). The mythical ‘boy crisis’? Economics of Education Review, 28 (1), 38–48.
Keller, C. (2001). Effect of teachers’ stereotyping on students’ stereotyping of mathematics as a male domain. Journal of Social Psychology, 141 (2), 165–173.
Kim, A. Y., Sinatra, G. M., & Seyranian, V. (2018). Developing a STEM identity among young women: A social identity perspective. Review of Educational Research, 88 (4), 589–625.
Lavy, V., & Sand, E. (2015). On the origins of gender human capital gaps: Short and long term consequences of teachers’ stereotypical biases (Working Paper No. w20909). Cambridge, MA: National Bureau of Economic Research.
Lecklider, A. S. (2013). Inventing the egghead: The paradoxes of brainpower in cold war American culture. Journal of American Studies, 45 (2), 245–265.
Leder, G. C., & Forgasz, J. F. (2003). Measuring mathematical beliefs and their impact on the learning of mathematics: A new approach. In G. C. Leder, E. Pehkonen, & G. Torner (Eds.), Beliefs: A hidden variable in mathematics education? (pp. 95–114). Dordrecht, The Netherlands: Kluwer Academic Publishers.
Leder, G. C., Pehkonen, E., & Torner, G. (2003). Beliefs: A hidden variable in mathematics education? Dordrecht, The Netherlands: Kluwer Academic Publishers.
Leedy, M. G., LaLonde, D., & Runk, K. (2003). Gender equity in mathematics: Beliefs of students, parents, and teachers. School Science and Mathematics, 103 (6), 285–292.
Leslie, S.-J., Cimpian, A., Meyer, M., & Freeland, E. (2015). Expectations of brilliance underlie gender distributions across academic disciplines. Science, 347 (6219), 23–34.
Li, Q. (1999). Teachers’ beliefs and gender differences in mathematics: A review. Educational Research, 41 (1), 63–76.
Liljedahl, P., Oesterle, S., & Bernèche, C. (2012). Stability of beliefs in mathematics education: A critical analysis. Nordic Studies in Mathematics Education, 17 (3–4), 101–118.
Lubienski, S. T., McGraw, R., & Strutchens, M. (2004). NAEP findings regarding gender: Mathematics achievement, student affect, and learning practices. In P. Kloosterman & F. K. Lester Jr. (Eds.), Results and interpretations of the 1990 through 2000 mathematics assessments of the National Assessment of educational Progress (pp. 305–336). Reston, VA: National Council of Teachers of Mathematics.
Mullis, I. V. S., Martin, M. O., Foy, P., & Hooper, M. (2016). TIMSS 2015 international results in mathematics . Boston, MA: Boston College International Study Center. Online at http://timssandpirls.bc.edu/timss2015/international-results/ .
National Science Foundation. (2015). Science and engineering degrees, by race/ethnicity of recipients: 2002–12 . Arlington, VA: Arlington. Retrieved from https://www.nsf.gov/statistics/2017/nsf17310/ .
Niepel, C., Stadler, M., & Greiff, S. (2019). Seeing is believing: Gender diversity in STEM is related to mathematics self-concept. Journal of Educational Psychology, 111 (6), 1119–1130.
Nosek, B. A., Smyth, F. L., Sriram, N., Lindner, N. M., Devos, T., Ayala, A., . . . Greenwald, A. G. (2009). National differences in gender-science stereotypes predict national sex differences in science and math achievement. Proceedings of the National Academy of Sciences, 106 (26), 10593–10597.
Nosek, B. A., & Smyth, F. L. (2011). Implicit social cognitions predict sex differences in math engagement and achievement. American Educational Research Journal, 48 (5), 1125–1156.
Nunnally, J. C., & Bernstein, I. H. (1994). Psychometric theory (3rd ed.). New York, NY: McGraw-Hill.
Nürnberger, M., Nerb, J., Schmitz, F., Keller, J., & Sütterlin, S. (2016). Implicit gender stereotypes and essentialist beliefs predict preservice teachers’ tracking recommendations. The Journal of Experimental Education, 84 (1), 152–174.
Organisation for Economic Co-operation and Development. (2015). The ABC of gender equality in education: Aptitude, behaviour, confidence. PISA . Paris, France: OECD Publishing. https://doi.org/10.1787/9789264229945-en
Organisation for Economic Co-operation and Development. (2019). PISA 2018 results (volume II): Where all students can succeed. PISA. Paris, France: OECD Publishing. https://doi.org/10.1787/b5fd1b8f-en .
Oyserman, D., Elmore, K., & Smith, G. (2012). Self, self-concept, and identity. In M. Leary & J. Tangney (Eds.), Handbook of self and identity (2nd ed., pp. 69–104). New York, NY: Guilford.
Philipp, R. A. (2007). Mathematics teachers’ beliefs and affect. In F. Lester (Ed.), Second handbook of research in mathematics teaching and learning (pp. 257–315). New York, NY: Information Age.
Robinson, J. P., & Lubienski, S. T. (2011). The development of gender achievement gaps in mathematics and reading during elementary and middle school: Examining direct cognitive assessments and teacher ratings. American Educational Research Journal, 48 (2), 268–302.
Robnett, R. D. (2016). Gender bias in STEM fields: Variation in prevalence and links to STEM self-concept. Psychology of Women Quarterly, 40 (1), 65–79.
Rosenthal, R., & Jacobson, L. (1968). Pygmalion in the classroom. The Urban Review, 3 (1), 16–20.
Rosenthal, R. (2002). Covert communication in classrooms, clinics, courtrooms, and cubicles. American Psychologist, 57 , 838–849.
Sax, L. J. (2008). The gender gap in college: Maximizing the developmental potential of women and men . San Francisco, CA: Jossey-Bass.
Schoon, I., & Eccles, J. S. (2014). Gender differences in aspirations and attainment: A life course perspective . Cambridge, UK: Cambridge University Press.
Book Google Scholar
Seals, C. (2018). Teacher beliefs: Effects of a teacher based mindset intervention on math student motivation and achievement . East Lansing, MI: Michigan State University. Retrieved from http://libproxy.usc.edu/login?url=https://search-proquest-com.libproxy2.usc.edu/docview/2155987278?accountid=14749 .
Snyder, T. D., de Brey, C., & Dillow, S. A. (2019). Digest of education statistics 2017 (NCES 2018–070) , National Center for Education Statistics, Institute of Education Sciences, U.S . Washington, DC: Department of Education. Retrieved from https://nces.ed.gov/programs/digest/d17/tables/dt17_209.22.asp?current=yes .
Steele, C. M., & Aronson, J. (1995). Stereotype threat and the intellectual test performance of African Americans. Journal of Personality and Social Psychology, 69 (5), 797–811.
Steele, C. M., Spencer, S. J., & Aronson, J. (2002). Contending with group image: The psychology of stereotype and social identity threat. In M. P. Zanna (Ed.), Advances in experimental social psychology (Vol. 34, pp. 379–440). San Diego, CA: Academic Press, Inc..
Stipek, D. J., Givvin, K. B., Salmon, J. M., & MacGyvers, V. L. (2001). Teachers’ beliefs and practices related to mathematics instruction. Teaching and Teacher Education, 17 (2), 213–226.
Strack, F., & Deutsch, R. (2004). Reflective and impulsive determinants of social behavior. Personality and Social Psychology Review, 8 , 220–247.
Sumpter, L. (2016). Investigating upper secondary school teachers’ conceptions: Is mathematical reasoning considered gendered? International Journal of Science and Mathematics Education, 14 , 347–362.
Thompson, A. (1984). The relationship of teachers' conceptions of mathematics and mathematics teaching to instructional practice. Educational Studies in Mathematics, 15 , 105–127.
Tiedemann, J. (2000). Gender-related belief of teachers in elementary school mathematics. Educational Studies in Mathematics, 41 (2), 191–207.
Tiedemann, J. (2002). Teachers’ gender stereotypes as determinants of teacher perceptions in elementary school mathematics. Educational Studies in Mathematics, 50 (1), 49–62.
United Nations Educational, Scientific, and Cultural Organization. (n.d.). Education: Distribution of tertiary graduates by field of study [Data file]. Retrieved April 20, 2020 from http://data.uis.unesco.org .
Wang, M. T., & Degol, J. L. (2017). Gender gap in science, technology, engineering, and mathematics (STEM): Current knowledge, implications for practice, policy, and future directions. Educational Psychology Review, 29 (1), 119–140.
Watt, H. M. G., & Eccles, J. S. (2008). Gender and occupational outcomes: Longitudinal assessments of individual, social, and cultural influences . Washington, DC: American Psychological Association.
Wigfield, A., Eccles, J. S., Yoon, K. S., Harold, R. D., Arbreton, A. J., Freedman-Doan, C., & Blumenfeld, P. C. (1997). Change in children's competence beliefs and subjective task values across the elementary school years: A 3-year study. Journal of Educational Psychology, 89 (3), 451–469.
Wilson, M., & Cooney, T. J. (2003). Mathematics teacher change and development. In G. C. Leder, E. Pehkonen, & G. Torner (Eds.), Beliefs: A hidden variable in mathematics education? (pp. 127–148). Dordrecht, The Netherlands: Kluwer Academic Publishers.
Zan, R., Brown, L., Evans, J., & Hannula, M. S. (2006). Affect in mathematics education: An introduction. Educational Studies in Mathematics, 63 (2), 113–121.
Download references
Acknowledgments
We thank Caroline Brayer Ebby and Jonathan A. Supovitz for their help conduct us this research.
Author information
Authors and affiliations.
Rossier School of Education, University of Southern California, 3470 Trousdale Pkwy, Los Angeles, CA, 90089, USA
Yasemin Copur-Gencturk & David Quinn
University of Texas at San Antonio, San Antonio, TX, USA
Ian Thacker
You can also search for this author in PubMed Google Scholar
Corresponding author
Correspondence to Yasemin Copur-Gencturk .
Electronic supplementary material
(DOCX 33 kb)
Rights and permissions
Reprints and permissions
About this article
Copur-Gencturk, Y., Thacker, I. & Quinn, D. K-8 Teachers’ Overall and Gender-Specific Beliefs About Mathematical Aptitude. Int J of Sci and Math Educ 19 , 1251–1269 (2021). https://doi.org/10.1007/s10763-020-10104-7
Download citation
Received : 19 October 2019
Accepted : 19 May 2020
Published : 11 July 2020
Issue Date : August 2021
DOI : https://doi.org/10.1007/s10763-020-10104-7
Share this article
Anyone you share the following link with will be able to read this content:
Sorry, a shareable link is not currently available for this article.
Provided by the Springer Nature SharedIt content-sharing initiative
- Gender-specific ability beliefs
- Mathematics-specific ability beliefs
- Teachers’ beliefs
- Find a journal
- Publish with us
- Track your research

IMAGES
VIDEO
COMMENTS
Research suggests that gender differences in math performance and self-perceived levels of math ability during childhood could be essential factors explaining this underrepresentation. Parental occupation type, a factor that seems less studied in the STEM gender gap literature, could also be an important factor in reducing women's ...
Gender differences in math self-concepts (i.e., how students perceive their own math ability) have often been considered in the literature as an explanation for the gender gap in math enrolment (e ...
Research suggests that gender differences in average math ability are minimal throughout childhood and do not emerge consistently until mid-to-late adolescence, although right-tail differences favoring males are present as early as kindergarten and girls do lose some ground as they progress through elementary school (Lindberg et al. 2010 ...
In terms of gender differences related to emotions provoked by mathematics, among which studies on math anxiety stand out, research indicates (albeit with small effect sizes) the existence of greater rates of anxiety in girls than boys during tasks involving mathematical reasoning (Hyde et al., 1990; Else-Quest et al., 2010).
Child-to-child neural saimilarity. Average neural similarity (r (500) ≥ 0.115, p ≤ 0.01) when calculated across children of the same gender (yellow, column 1) and children of different genders ...
Overall, most recent large-scale studies, such as the Programme for International Student Assessment (PISA) by the Organization for Economic Cooperation and Development (OECD), point out that gender-related disparities in mathematics attainments are small and that there is no consistent picture across countries (Guiso et al., 2008).For example, Stoet and Geary's (2013) analysis on within- and ...
Although mean effect sizes are small, boys consistently reported significantly more positive attitudes about mathematics (d 0.21-. 0.33). Similarly, girls reported greater mathematics anxiety than did boys (d 0.27). Ranges of gender differences in math attitudes and affect were larger than those in math achievement.
This narrative review of current research on gender and mathematics covers the years 2020 to 2022. The number of exemplary publications within these 3 years and the diversity of topics, theoretical frameworks, subjects, and authors are indications of gender and mathematics remaining a robust and evolving area of study. Of particular interest are studies of lived experiences of students in ...
We argue the importance of further research into gender differences in mathematics and science for two main reasons. Firstly, as noted across many developed nations, there is a shortage of science graduates to meet the needs of industry, which Handelsman et al. have argued can best be addressed by broadening the pool of applicants entering the science pipeline to include a greater ...
The role of gender in mathematical abilities has caught the interest of researchers for several decades; however, their findings are not conclusive yet. Recently the need to explore its influence on the development of some foundational mathematic skills has been highlighted. Thus, the current study examined whether gender differentially affects young children's performance in several basic ...
Using data from the Trends in International Mathematics and Science Study (TIMSS) 2015 assessment of fourth-grade students in 32 countries, a series of mean comparisons and regression analyses were conducted to determine (1) the gender gap in students' self-concept in mathematics; (2) to what extent student achievement, student gender, and parental characteristics (early numeracy activities ...
Although gender differences in cognitive abilities are frequently reported, the magnitude of these differences and whether they hold practical significance in the educational outcomes of boys and girls is highly debated. Furthermore, when gender gaps in reading, mathematics and science literacy are reported they are often attributed to innate, biological differences rather than social and ...
In a cross-sectional study of youth ages 8-15, we examined implicit and explicit gender stereotypes regarding math and language abilities. We investigated how implicit and explicit stereotypes differ across age and gender groups and whether they are consistent with cultural stereotypes. Participants (N = 270) completed the Affect Misattribution Procedure (AMP) and a survey of explicit ...
Levene's test of Equality of Variances revealed no significant differences in variance between girls and boys ( F (1, 78) < 0.01, p = .94, boys' s.d. = 14.65, girls' s.d. = 14.27). This is ...
The misconception items asked for all of the characterizing aspects of each hypothesized math-gender misconception, by also referring to research findings (Dweck, 1999; Tiedemann, 2002; Muenks et al., 2020; Sáinz et al., 2020), and academic as well as non-academic resources (Baron-Cohen, 2005; Escovar et al., 2016).For the empathizing-systemizing misconception, we constructed each of the ...
Objective. Previous research has shown that women have an advantage on verbal episodic memory and processing speed tasks, while men show an advantage on spatial ability measures. Previous work has also found differences in cognition across age. The current study examines gender differences in neurocognitive functioning across adulthood, whether ...
For ungraduated students, in the area of mathematics only four studies of the meta-analysis of Voyer and Voyer (2014) report higher grades in male students, two studies show results without differences by gender and fourteen articles report more favorable results for females (the mean estimated effect size of these samples measured by Cohen's d (female-male) is 0.12 and the 95% confidence ...
In terms of gender differences related to emotions provoked by mathematics, among which studies on math anxiety stand out, research indicates (albeit with small effect sizes) the existence of greater rates of anxiety in girls than boys during tasks involving mathematical reasoning (Hyde et al., 1990; Else-Quest et al., 2010).
Study with Quizlet and memorize flashcards containing terms like Research on gender differences in tested abilities indicates that there are _____., Studies on gender differences in abilities and achievements indicate that _____., According to meta-analyses of research on gender differences in mathematics performance, _____. and more.
A widespread belief exists in many societies that some people are born with mathematics ability, which is regarded as an innate aptitude or the potential to perform well in mathematics.Historical and psychological research supports the existence of prevalent stereotypes and beliefs associating men more than women with mathematical ability (e.g., Bennett, 2011; Furnham, Hosoe, & Tang, 2002 ...
Study with Quizlet and memorize flashcards containing terms like The boundaries between how the typical male and the typical female are expected to behave is becoming ____________, Research on females exposed prenatally to high levels of androgens suggests, Research on gender differences in mathematical ability indicates that __________ and more.
the gender difference in throwing distance in adolescence and beyond. Study with Quizlet and memorize flashcards containing terms like Research on gender differences in abilities indicates that, Research on spatial ability shows that, A woman gets an A on a statistics exam. She believes it was due to luck.
Research on gender differences in mathematical ability indicates that. a. girls are more precocious than boys in mathematics. b. boys generally do better than girls on mathematical analogies. c. girls outperform boys at the high end of the mathematical ability distribution. d.