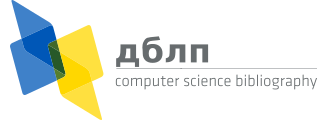
- solidarity - (ua) - (ru)
- news - (ua) - (ru)
- donate - donate - donate
for scientists:
- ERA4Ukraine
- Assistance in Germany
- Ukrainian Global University
- #ScienceForUkraine

default search action
- combined dblp search
- author search
- venue search
- publication search


Welcome to dblp
Search dblp, full-text search, please enter a search query.

- How to use the dblp search?
- Which technology does dblp use for searching the website?
- case-insensitive prefix search: default e.g., sig matches "SIGIR" as well as "signal"
- exact word search: append dollar sign ($) to word e.g., graph$ matches "graph", but not "graphics"
- boolean and: separate words by space e.g., codd model
- boolean or: connect words by pipe symbol (|) e.g., graph|network
Update May 7, 2017: Please note that we had to disable the phrase search operator (.) and the boolean not operator (-) due to technical problems. For the time being, phrase search queries will yield regular prefix search result, and search terms preceded by a minus will be interpreted as regular (positive) search terms.
Author search results
Venue search results, refine list.
refine by author
- temporarily not available
refine by venue
refine by type
refine by year
Publication search results
found 7,239,904 matches
skipping 7,239,904 more matches
failed to load more results, please try again later

- by publisher

- books & theses
- reference works
- edited collections
read as PDF
(read full post)
(updated 2023-06-28) A few days ago, we discussed the new dataset publications in dblp. As a preparation for more and more detailed datasets we slightly modify the DTD that defines the structure of our XML data export. A quick reminder: you can download the dblp dataset as a single XML […]
Datasets and other research artifacts are a major topic in the scientific community in the recent years. Many ongoing projects focus on improving the standardization, publication and citation of these artifacts. Currently, the dblp team is involved in three of them: NFDI4DataScience, NFDIxCS, and Unknown Data. As part of these […]
On November 4, 2022, the Joint Science Conference (GWK) selected Schloss Dagstuhl – Leibniz Center for Informatics and the consortium NFDIxCS for federal and state funding within the German National Research Data Infrastructure (NFDI). The consortium will be funded in the double-digit millions of Euros and over a duration of five […]
In the six months since the release of the dblp RDF dump and its persistent snapshot releases, the RDF dump has been downloaded a total of about a thousand times. We are pleased to see that the community is interested in using our semantic data in their research and beyond. […]
more blog posts
The dblp computer science bibliography provides open bibliographic information on major computer science journals and proceedings. Originally created at the University of Trier in 1993, dblp is now operated and further developed by Schloss Dagstuhl . For more information check out our F.A.Q.
dblp statistics
- # of publications : 7,239,904
- # of authors : 3,509,859
- # of conferences : 6,651
- # of journals : 1,872
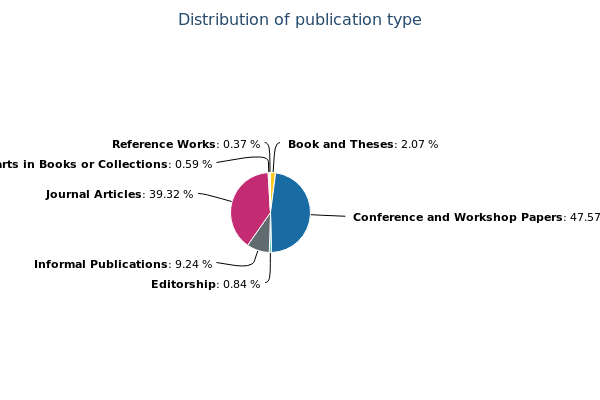
more statistics

Related resources
- ACM Digital Library
- IEEE Xplore | CSDL
- Semantic Scholar
- INSPIRE-HEP
more external links
Social media links

manage site settings
To protect your privacy, all features that rely on external API calls from your browser are turned off by default . You need to opt-in for them to become active. All settings here will be stored as cookies with your web browser. For more information see our F.A.Q.
Unpaywalled article links

load links from unpaywall.org
Privacy notice: By enabling the option above, your browser will contact the API of unpaywall.org to load hyperlinks to open access articles. Although we do not have any reason to believe that your call will be tracked, we do not have any control over how the remote server uses your data. So please proceed with care and consider checking the Unpaywall privacy policy .
Archived links via Wayback Machine

load content from archive.org
Privacy notice: By enabling the option above, your browser will contact the API of archive.org to check for archived content of web pages that are no longer available. Although we do not have any reason to believe that your call will be tracked, we do not have any control over how the remote server uses your data. So please proceed with care and consider checking the Internet Archive privacy policy .
Reference lists

load references from crossref.org and opencitations.net
Privacy notice: By enabling the option above, your browser will contact the APIs of crossref.org , opencitations.net , and semanticscholar.org to load article reference information. Although we do not have any reason to believe that your call will be tracked, we do not have any control over how the remote server uses your data. So please proceed with care and consider checking the Crossref privacy policy and the OpenCitations privacy policy , as well as the AI2 Privacy Policy covering Semantic Scholar.
Citation data
load citations from opencitations.net
Privacy notice: By enabling the option above, your browser will contact the API of opencitations.net and semanticscholar.org to load citation information. Although we do not have any reason to believe that your call will be tracked, we do not have any control over how the remote server uses your data. So please proceed with care and consider checking the OpenCitations privacy policy as well as the AI2 Privacy Policy covering Semantic Scholar.
OpenAlex data

load data from openalex.org
Privacy notice: By enabling the option above, your browser will contact the API of openalex.org to load additional information. Although we do not have any reason to believe that your call will be tracked, we do not have any control over how the remote server uses your data. So please proceed with care and consider checking the information given by OpenAlex .
retrieved on 2024-05-17 13:17 CEST from data curated by the dblp team

see also: Terms of Use | Privacy Policy | Imprint
dblp was originally created in 1993 at:
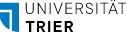
since 2018, dblp has been operated and maintained by:
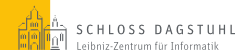
the dblp computer science bibliography is funded and supported by:
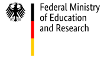
Reference management. Clean and simple.
The top list of academic research databases
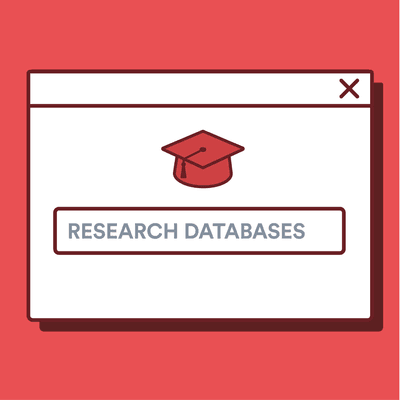
2. Web of Science
5. ieee xplore, 6. sciencedirect, 7. directory of open access journals (doaj), get the most out of your academic research database, frequently asked questions about academic research databases, related articles.
Whether you are writing a thesis , dissertation, or research paper it is a key task to survey prior literature and research findings. More likely than not, you will be looking for trusted resources, most likely peer-reviewed research articles.
Academic research databases make it easy to locate the literature you are looking for. We have compiled the top list of trusted academic resources to help you get started with your research:
Scopus is one of the two big commercial, bibliographic databases that cover scholarly literature from almost any discipline. Besides searching for research articles, Scopus also provides academic journal rankings, author profiles, and an h-index calculator .
- Coverage: 90.6 million core records
- References: N/A
- Discipline: Multidisciplinary
- Access options: Limited free preview, full access by institutional subscription only
- Provider: Elsevier
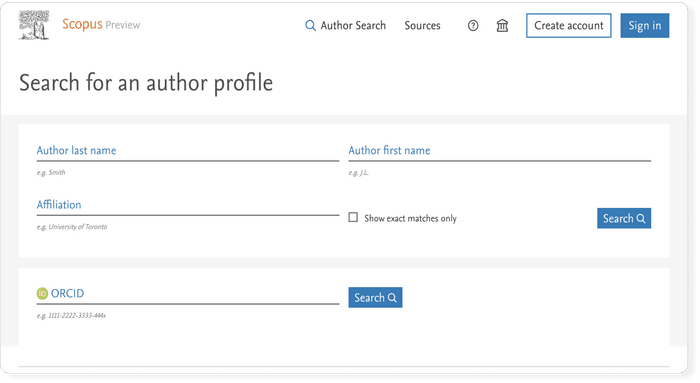
Web of Science also known as Web of Knowledge is the second big bibliographic database. Usually, academic institutions provide either access to Web of Science or Scopus on their campus network for free.
- Coverage: approx. 100 million items
- References: 1.4 billion
- Access options: institutional subscription only
- Provider: Clarivate (formerly Thomson Reuters)
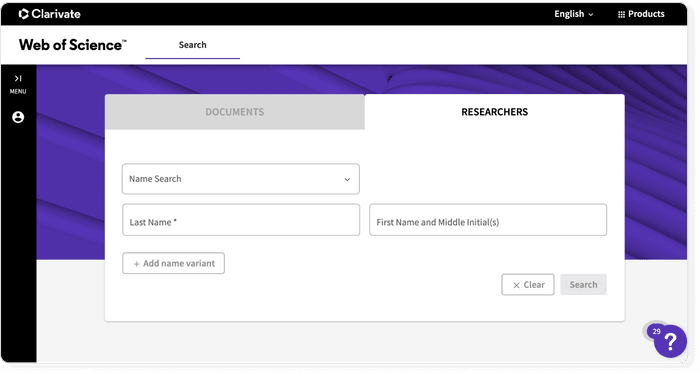
PubMed is the number one resource for anyone looking for literature in medicine or biological sciences. PubMed stores abstracts and bibliographic details of more than 30 million papers and provides full text links to the publisher sites or links to the free PDF on PubMed Central (PMC) .
- Coverage: approx. 35 million items
- Discipline: Medicine and Biological Sciences
- Access options: free
- Provider: NIH
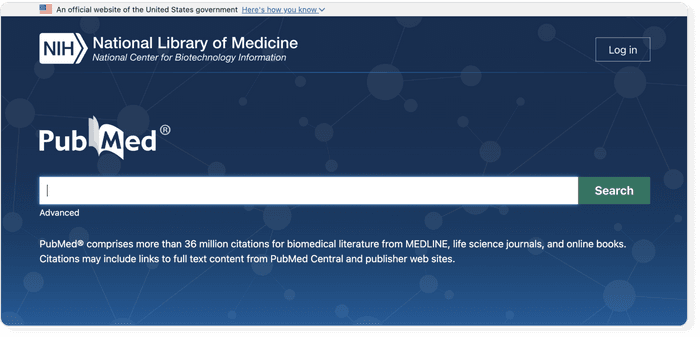
For education sciences, ERIC is the number one destination. ERIC stands for Education Resources Information Center, and is a database that specifically hosts education-related literature.
- Coverage: approx. 1.6 million items
- Discipline: Education
- Provider: U.S. Department of Education
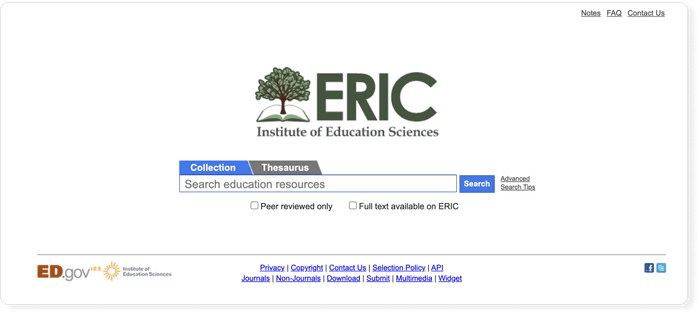
IEEE Xplore is the leading academic database in the field of engineering and computer science. It's not only journal articles, but also conference papers, standards and books that can be search for.
- Coverage: approx. 6 million items
- Discipline: Engineering
- Provider: IEEE (Institute of Electrical and Electronics Engineers)
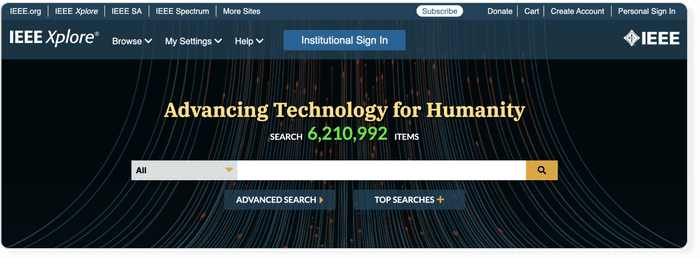
ScienceDirect is the gateway to the millions of academic articles published by Elsevier, 1.4 million of which are open access. Journals and books can be searched via a single interface.
- Coverage: approx. 19.5 million items
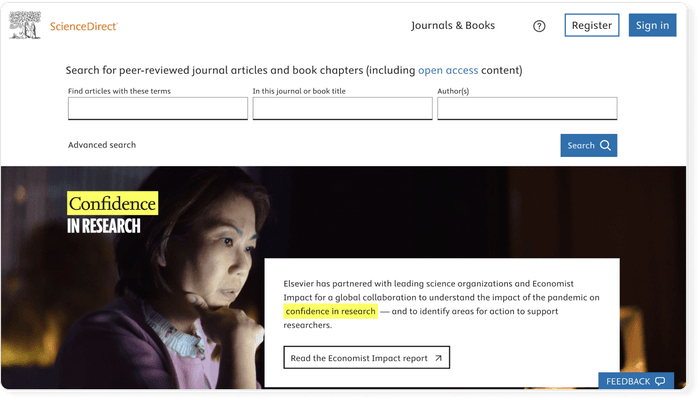
The DOAJ is an open-access academic database that can be accessed and searched for free.
- Coverage: over 8 million records
- Provider: DOAJ
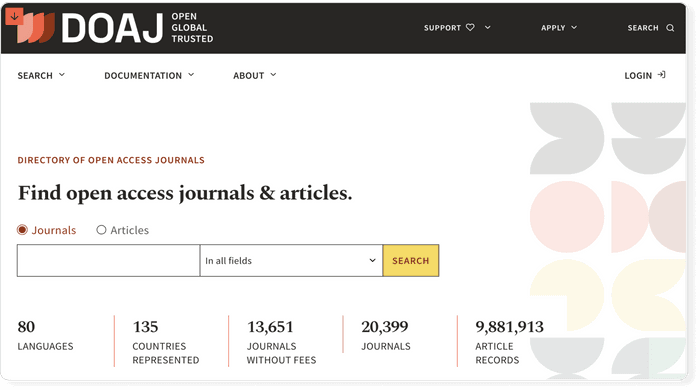
JSTOR is another great resource to find research papers. Any article published before 1924 in the United States is available for free and JSTOR also offers scholarships for independent researchers.
- Coverage: more than 12 million items
- Provider: ITHAKA
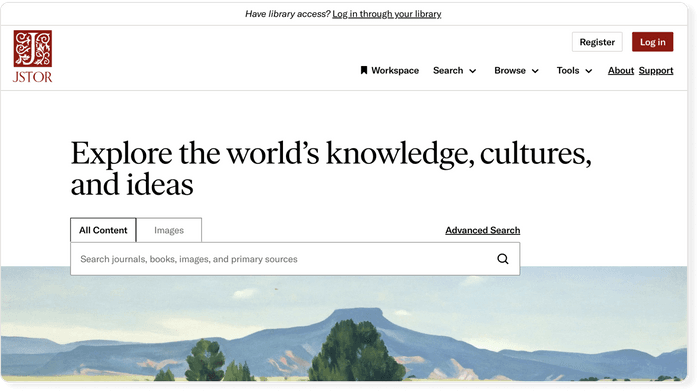
Start using a reference manager like Paperpile to save, organize, and cite your references. Paperpile integrates with PubMed and many popular databases, so you can save references and PDFs directly to your library using the Paperpile buttons:
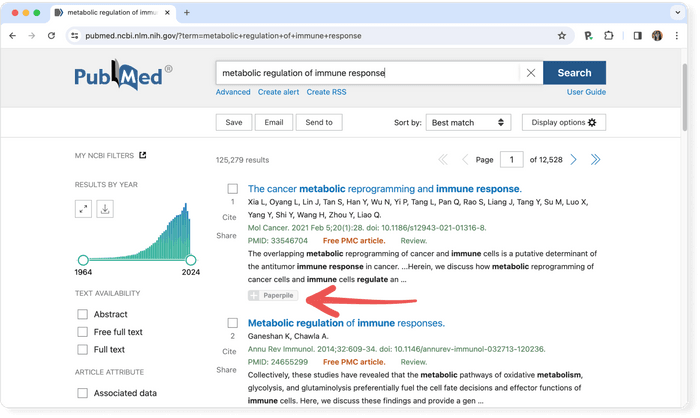
Scopus is one of the two big commercial, bibliographic databases that cover scholarly literature from almost any discipline. Beside searching for research articles, Scopus also provides academic journal rankings, author profiles, and an h-index calculator .
PubMed is the number one resource for anyone looking for literature in medicine or biological sciences. PubMed stores abstracts and bibliographic details of more than 30 million papers and provides full text links to the publisher sites or links to the free PDF on PubMed Central (PMC)
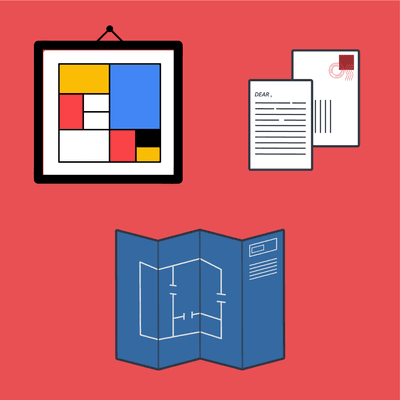
University Library, University of Illinois at Urbana-Champaign

Computer Science Research Resources: Find Articles & Papers
- Find Articles & Papers
- High-Impact Journals
- Standards & Technical Reports
- Patents & Government Documents
- E-Books & Reference
- Dissertations & Theses
- Additional Resources
Engineering Easy Search
University library search engines.
- Grainger Engineering Library Homepage With specialized searches for Engineering and the Physical Sciences.
- Easy Search The easiest way to locate University Library resources, materials, and more!
- Find Online Journals Search by title or by subject to view our subscription details, including date ranges and where you can access full text.
- Journal and Article Locator Finds electronic or print copy of articles by using a citation.
Engineering Article Databases
- Engineering Village This link opens in a new window Search for articles, conference paper, and report information in all areas of engineering. Full-text is often available through direct download.
- Scopus This link opens in a new window Search periodicals, conference proceedings, technical reports, trade literature, patents, books, and press releases in all engineering fields. Some full-text available as direct downloads.
- Web of Science (Core Collection) This link opens in a new window Search for articles in science and engineering. Also provides Science Citation Index that tracks citations in science and technical journals published since 1981. Journal Citation Reports are also available through ISI.
Computer Science Article Databases
- ACM Digital Library This link opens in a new window This site provides access to tables of contents, abstracts, reviews, and full text of every article ever published by ACM and bibliograhic citations from major publishers in computing.
- Compendex This link opens in a new window Compendex is the most comprehensive bibliographic database of scientific and technical engineering research available, covering all engineering disciplines. It includes millions of bibliographic citations and abstracts from thousands of engineering journals and conference proceedings. When combined with the Engineering Index Backfile (1884-1969), Compendex covers well over 120 years of core engineering literature.
- IEEE Xplore This link opens in a new window Provides full-text access to IEEE transactions, IEEE and IEE journals, magazines, and conference proceedings published since 1988, and all current IEEE standards; brings additional search and access features to IEEE/IEE digital library users. Browsable by books & e-books, conference publications, education and learning, journals and magazines, standards and by topic. Also provides links to IEEE standards, IEEE spectrum and other sites.
Subject Guide

Ask a Librarian
- Next: High-Impact Journals >>
- Last Updated: Jun 16, 2023 9:35 AM
- URL: https://guides.library.illinois.edu/cs
Navigation Menu
Search code, repositories, users, issues, pull requests..., provide feedback.
We read every piece of feedback, and take your input very seriously.
Saved searches
Use saved searches to filter your results more quickly.
To see all available qualifiers, see our documentation .
- Notifications
Papers from the computer science community to read and discuss.
papers-we-love/papers-we-love
Folders and files, repository files navigation.
Papers We Love ( PWL ) is a community built around reading, discussing and learning more about academic computer science papers. This repository serves as a directory of some of the best papers the community can find, bringing together documents scattered across the web. You can also visit the Papers We Love site for more info.
Due to licenses we cannot always host the papers themselves (when we do, you will see a 📜 emoji next to its title in the directory README) but we can provide links to their locations.
If you enjoy the papers, perhaps stop by a local chapter meetup and join in on the vibrant discussions around them. You can also discuss PWL events, the content in this repository, and/or anything related to PWL on our Discord server.
Let us know if you are interested in starting one in your city!
All of our meetups follow our Code of Conduct .
Past Presentations
Check out our YouTube channel for videos and video playlists.
We're looking for pull requests related to papers we should add, better organization of the papers we do have, and/or links to other paper-repos we should point to.
Other Good Places to Find Papers
- 2 Minute Papers
- Bell System Technical Journal, 1922-1983
- Best Paper Awards in Computer Science
- Google Scholar (choose a subcategory)
- Microsoft Research
- Functional Programming Books Review
- MIT's Artificial Intelligence Lab Publications
- MIT's Distributed System's Reading Group
- arXiv Paper Repository
- Services Engineering Reading List
- Readings in Distributed Systems
- Gradual Typing Bibliography
- Security Data Science Papers
- Research Papers from Robert Harper, Carnegie Mellon University
- Lobste.rs tagged as PDF
- The Morning Paper
Please check out our wiki-page for links to blogs, books, exchanges that are worth a good read.
How To Read a Paper
Reading a paper is not the same as reading a blogpost or a novel. Here are a few handy resources to help you get started.
- How to read an academic article
- Advice on reading academic papers
- How to read and understand a scientific paper
- Should I Read Papers?
- The Refreshingly Rewarding Realm of Research Papers
- How to read a paper
Applications/Ideas built around Papers We Love
- Love a Paper - @loveapaper
Download papers
Open your favourite terminal and run:
This will scrape markdown files for links to PDFs and download papers to their respective directories.
See README.md for more options.
Contributing Guidelines
Please take a look at our CONTRIBUTING.md file.
The name "Papers We Love" and the logos for the organization are copyrighted, and under the ownership of Papers We Love Ltd, all rights reserved. When starting a chapter, please review our guidelines and ask us about using the logo.
Code of conduct
Contributors 250.
- Shell 100.0%
University of Illinois Chicago
University library, search uic library collections.
Find items in UIC Library collections, including books, articles, databases and more.
Advanced Search
Search UIC Library Website
Find items on the UIC Library website, including research guides, help articles, events and website pages.
- Search Collections
- Search Website
- UIC Library
- Subject and Course Guides
- Computer Science
- Research Databases
Computer Science: Research Databases
- Managing Your Data
- How to Read a Scientific Paper
- Journal and Conference Rankings
- Writing Help

Computer Science Library Databases
This site provides access to tables of contents, abstracts, reviews, and full text of every article ever published by ACM and citations from major publishers in computing.
- IEEE Xplore IEEE journals and conference proceedings; Institution of Engineering and Technology (IET) journals and proceedings; all current IEEE Standards. Note: If you're unable to set up an IEEE account, go to https://ieeexplore.ieee.org/. After creating an account, go to IEEE in the library website and sign in.
- BrowZine Web BrowZine is a service that interacts with a library’s existing e-journal resources allowing users to browse journals in a normalized setting and create a personalized reading room of journals to follow. Originally developed for mobile devices, it is now being expanded to a web interface for laptop and desktop use. A BrowZine account is required to sync between the app and BrowZine Web.
General Sci/Tech Library Databases
- Google Scholar Searches for scholarly materials such as peer-reviewed papers, theses, books, preprints, abstracts and technical reports from broad areas of research. It includes a variety of academic publishers, professional societies, preprint repositories and universities, as well as scholarly articles available across the web.
- Scopus Scopus is the largest abstract and citation database including peer-reviewed titles from international publishers, Open Access journals, conference proceedings, trade publications and quality web sources. Subject coverage includes: Chemistry, Physics, Mathematics and Engineering; Life and Health Sciences; Social Sciences, Psychology and Economics; Biological, Agricultural and Environmental Sciences
- Web of Science Multidisciplinary coverage of over 10,000 journals in the sciences, social sciences, and arts and humanities, as well as published proceedings for over 12,000 conferences per year, and over 50,000 scholarly books. Allows searching for articles in the database that have cited a particular work, as well as perform other analytics.
Other Resources
arXiv is a pioneering open access e-print archive of physics, mathematics and computer science articles, hosted by the Cornell University Library.
- DBLP Another bibliographic database focused on CS research
- Semantic Scholar A new search that uses AI principles and semantic linking to try and create a better scholarly resource search engine. So far, has only been applied to CS materials, but they plan to use the search to crawl all scholarly resources in the future.
Subject Guide
- Next: Books >>
- Last Updated: Apr 15, 2024 2:56 PM
- URL: https://researchguides.uic.edu/computerscience
Open research in computer science
Spanning networks and communications to security and cryptology to big data, complexity, and analytics, SpringerOpen and BMC publish one of the leading open access portfolios in computer science. Learn about our journals and the research we publish here on this page.
Highly-cited recent articles
Spotlight on.
EPJ Data Science
See how EPJ Data Science brings attention to data science
Reasons to publish in Human-centric Computing and Information Sciences
Download this handy infographic to see all the reasons why Human-centric Computing and Information Sciences is a great place to publish.
We've asked a few of our authors about their experience of publishing with us.
What authors say about publishing in our journals:
Fast, transparent, and fair. - EPJ Data Science Easy submission process through online portal. - Journal of Cloud Computing Patient support and constant reminder at every phase. - Journal of Cloud Computing Quick and relevant. - Journal of Big Data
How to Submit Your Manuscript
Your browser needs to have JavaScript enabled to view this video
Computer science blog posts
Read the latest from the SpringerOpen blog
The SpringerOpen blog highlights recent noteworthy research of general interest published in our open access journals.
Failed to load RSS feed.

Computer Science
- Databases & Articles
- Web Resources
- Dictionaries/Encyclopedias
- Help with Research
- Citation Management
Why use Databases?
Databases are subscription resources that bring articles from a variety of magazines and/or journals into one place with a sophisticated search engine. Many of the databases allow you to read the entire article online. All databases that UM Libraries subscribes to can be accessed through Database Finder.
What if there is no full text?
Don't panic! You can:
- Request article from another library
Where can I find articles on my topic?
The UM Libraries subscribe to over 300 databases!!! The following databases are the primary electronic resources available for locating journal articles in computer science. All databases that UMCP subscribes to can be accessed through Database Finder
- ACM Digital Library Full-text repository of papers from publications that have been published, co-published, or co-marketed by ACM and other publishers. Publications include journals, magazines, transactions, special interest group (SIG) newsletters, proceedings, and publications by affiliated organizations. Contains citations and full text from ACM journal and newsletter articles and conference proceedings. 1985-present.
- IEEE Xplore Provides full-text access to IEEE transactions, journals, magazines and conference proceedings published since 1988 and all current IEEE Standards. Includes access to Bell Labs Technical journal Archive (BLTJA) 1922-2015
- Lecture Notes in Computer Science Established as a medium for the publication of new developments in computer science and information technology research and teaching. Selected volumes available from 1973-1996,2005-2011,2014
Multidisciplinary Resources
While not explicitly devoted to computer science topics, the following related, general, and/or multidisciplinary databases may prove useful in your research.
- MathSciNet: Mathematical Reviews on the Web Covering mathematical literature since 1940 this international database provides bibliographic data and reviews of mathematical research literature from 1700 current journals, books and other original documents. Books, journals, and proceedings in the field of mathematics. ISSN and ISBN searches are not available in this resource. Some of the citations are linked to the full text in online journals.
- ScienceDirect Peer-reviewed, full text database containing electronic book and journal titles covering the fields of science, technology and medicine. In addition to keyword searches, the image search and value added content associated with the publication can be found in the form of audio, video and datasets. Extensive coverage of the physical and biological sciences, significant numbers of journals in the social sciences, and some journals in the humanities. Dates of abstracting and indexing vary. Full text prior to 1995 often going back to vol. 1, iss. 1 Author searches are done on the last name only. Subject searches are supported, but subjects are not presented within the records. Truncation is not supported in phrases.
- Dissertations & Theses Global This database is the an authoritative source for information about doctoral dissertations and master's theses. The database represents the work of authors from over 1,000 graduate schools and universities. Full text is available from 1997 to the present. It also contains a significant amount of new international dissertations and theses both in citations and in full text. It offers access to more than 90 percent of the doctoral dissertations accepted each year in North America. The database also covers thousands of dissertations and theses from around the globe. Time Span: 1861 to present.
- EBSCO eBook Collection A collection of E-texts covering topics specifically chosen by Maryland Academic Libraries. Collection strengths include computer science, business, international relations, education, environmental science, psychology, and civil rights law and history.
- << Previous: Home
- Next: Web Resources >>
- Last Updated: Mar 11, 2024 2:38 PM
- URL: https://lib.guides.umd.edu/computerscience
University of Denver
University libraries, research guides, a guide to computer science research.
- Journal articles & conference papers
- Books & media
- Technical reports, stats, & other formats
- Standards & patents
- News articles
- Get full text of a specific article
- Request sources not at DU Libraries
- Search databases effectively
Identify the main concepts or keywords in your topic. Then, think of synonyms for your keywords.
Combine your search terms using and, or or not., use phrase searching., search for different word endings., broaden or narrow your search., re-sort your search results., use the database's thesaurus, if available., use analysis tools offered by the database..
- Evaluate your sources
- Confirm an article is peer-reviewed
- Cite sources properly
* An asterisk at the end of a word tells the database to find any possible ending to that word, so dens* will find dense, density, or densities .
Step 3: Combine your keywords using connectors like like "AND" and "OR" when you enter your search into a database -- the keyword table can help you understand how to connect your keywords.
Use AND , OR , or NOT to connect concepts together to broaden or narrow your search, or to eliminate concepts you don't want searched. These three words (AND, OR, and NOT) are called Boolean operators .
Make sure to capitalize AND , OR , and NOT -- this tells the database that the word is a " search operator " and not a keyword that it should be searching for.
You can use quotation marks to force the database to find an exact phrase.
Experiment with your searches to see which combination(s) of your keywords get the most useful results!
*Still getting zero results? Try another database or Ask Us ! We're here to help.
*If you've done a thorough literature search using a variety of different search queries in all appropriate databases and you still get zero results, celebrate! You've found a gap in the literature. Remember: gaps in the literature = opportunities for research = jobs!
Some databases have a controlled list of subject terms that you can use to build your search -- this list is usually called a thesaurus .
PubMed , an important biomedical database, has a thesaurus called MeSH (which stands for Medical Subject Headings). PubMed is such a complex database that it is usually more productive to build searches by selecting terms from MeSH . Below is an illustration of a table you could use to keep track of your search terms and the related thesaurus terms from each database:
If you'd like to learn about constructing a search using a database thesaurus, remember you can always contact a librarian .
- << Previous: Request sources not at DU Libraries
- Next: Evaluating & citing sources >>
- Last Updated: Mar 8, 2024 2:04 PM
- URL: https://libguides.du.edu/cs
- Harvard Library
- Research Guides
- Faculty of Arts & Sciences Libraries
Computer Science Library Research Guide
Find dissertations and theses.
- Get Started
- How to get the full-text
- What is Peer Review?
- Find Books in the SEC Library This link opens in a new window
- Find Conference Proceedings
- Find Patents This link opens in a new window
- Find Standards
- Find Technical Reports
- Find Videos
- Ask a Librarian This link opens in a new window
Engineering Librarian

How to search for Harvard dissertations
- DASH , Digital Access to Scholarship at Harvard, is the university's central, open-access repository for the scholarly output of faculty and the broader research community at Harvard. Most Ph.D. dissertations submitted from March 2012 forward are available online in DASH.
- Check HOLLIS, the Library Catalog, and refine your results by using the Advanced Search and limiting Resource Type to Dissertations
- Search the database ProQuest Dissertations & Theses Global Don't hesitate to Ask a Librarian for assistance.
How to search for Non-Harvard dissertations
Library Database:
- ProQuest Dissertations & Theses Global
Free Resources:
- Many universities provide full-text access to their dissertations via a digital repository. If you know the title of a particular dissertation or thesis, try doing a Google search.
Related Sites
- Formatting Your Dissertation - GSAS
- Ph.D. Dissertation Submission - FAS
- Empowering Students Before you Sign that Contract! - Copyright at Harvard Library
Select Library Titles
- << Previous: Find Conference Proceedings
- Next: Find Patents >>
- Last Updated: Feb 27, 2024 1:52 PM
- URL: https://guides.library.harvard.edu/cs
Harvard University Digital Accessibility Policy
computer science Recently Published Documents
Total documents.
- Latest Documents
- Most Cited Documents
- Contributed Authors
- Related Sources
- Related Keywords
Hiring CS Graduates: What We Learned from Employers
Computer science ( CS ) majors are in high demand and account for a large part of national computer and information technology job market applicants. Employment in this sector is projected to grow 12% between 2018 and 2028, which is faster than the average of all other occupations. Published data are available on traditional non-computer science-specific hiring processes. However, the hiring process for CS majors may be different. It is critical to have up-to-date information on questions such as “what positions are in high demand for CS majors?,” “what is a typical hiring process?,” and “what do employers say they look for when hiring CS graduates?” This article discusses the analysis of a survey of 218 recruiters hiring CS graduates in the United States. We used Atlas.ti to analyze qualitative survey data and report the results on what positions are in the highest demand, the hiring process, and the resume review process. Our study revealed that a software developer was the most common job the recruiters were looking to fill. We found that the hiring process steps for CS graduates are generally aligned with traditional hiring steps, with an additional emphasis on technical and coding tests. Recruiters reported that their hiring choices were based on reviewing resume’s experience, GPA, and projects sections. The results provide insights into the hiring process, decision making, resume analysis, and some discrepancies between current undergraduate CS program outcomes and employers’ expectations.
A Systematic Literature Review of Empiricism and Norms of Reporting in Computing Education Research Literature
Context. Computing Education Research (CER) is critical to help the computing education community and policy makers support the increasing population of students who need to learn computing skills for future careers. For a community to systematically advance knowledge about a topic, the members must be able to understand published work thoroughly enough to perform replications, conduct meta-analyses, and build theories. There is a need to understand whether published research allows the CER community to systematically advance knowledge and build theories. Objectives. The goal of this study is to characterize the reporting of empiricism in Computing Education Research literature by identifying whether publications include content necessary for researchers to perform replications, meta-analyses, and theory building. We answer three research questions related to this goal: (RQ1) What percentage of papers in CER venues have some form of empirical evaluation? (RQ2) Of the papers that have empirical evaluation, what are the characteristics of the empirical evaluation? (RQ3) Of the papers that have empirical evaluation, do they follow norms (both for inclusion and for labeling of information needed for replication, meta-analysis, and, eventually, theory-building) for reporting empirical work? Methods. We conducted a systematic literature review of the 2014 and 2015 proceedings or issues of five CER venues: Technical Symposium on Computer Science Education (SIGCSE TS), International Symposium on Computing Education Research (ICER), Conference on Innovation and Technology in Computer Science Education (ITiCSE), ACM Transactions on Computing Education (TOCE), and Computer Science Education (CSE). We developed and applied the CER Empiricism Assessment Rubric to the 427 papers accepted and published at these venues over 2014 and 2015. Two people evaluated each paper using the Base Rubric for characterizing the paper. An individual person applied the other rubrics to characterize the norms of reporting, as appropriate for the paper type. Any discrepancies or questions were discussed between multiple reviewers to resolve. Results. We found that over 80% of papers accepted across all five venues had some form of empirical evaluation. Quantitative evaluation methods were the most frequently reported. Papers most frequently reported results on interventions around pedagogical techniques, curriculum, community, or tools. There was a split in papers that had some type of comparison between an intervention and some other dataset or baseline. Most papers reported related work, following the expectations for doing so in the SIGCSE and CER community. However, many papers were lacking properly reported research objectives, goals, research questions, or hypotheses; description of participants; study design; data collection; and threats to validity. These results align with prior surveys of the CER literature. Conclusions. CER authors are contributing empirical results to the literature; however, not all norms for reporting are met. We encourage authors to provide clear, labeled details about their work so readers can use the study methodologies and results for replications and meta-analyses. As our community grows, our reporting of CER should mature to help establish computing education theory to support the next generation of computing learners.
Light Diacritic Restoration to Disambiguate Homographs in Modern Arabic Texts
Diacritic restoration (also known as diacritization or vowelization) is the process of inserting the correct diacritical markings into a text. Modern Arabic is typically written without diacritics, e.g., newspapers. This lack of diacritical markings often causes ambiguity, and though natives are adept at resolving, there are times they may fail. Diacritic restoration is a classical problem in computer science. Still, as most of the works tackle the full (heavy) diacritization of text, we, however, are interested in diacritizing the text using a fewer number of diacritics. Studies have shown that a fully diacritized text is visually displeasing and slows down the reading. This article proposes a system to diacritize homographs using the least number of diacritics, thus the name “light.” There is a large class of words that fall under the homograph category, and we will be dealing with the class of words that share the spelling but not the meaning. With fewer diacritics, we do not expect any effect on reading speed, while eye strain is reduced. The system contains morphological analyzer and context similarities. The morphological analyzer is used to generate all word candidates for diacritics. Then, through a statistical approach and context similarities, we resolve the homographs. Experimentally, the system shows very promising results, and our best accuracy is 85.6%.
A genre-based analysis of questions and comments in Q&A sessions after conference paper presentations in computer science
Gender diversity in computer science at a large public r1 research university: reporting on a self-study.
With the number of jobs in computer occupations on the rise, there is a greater need for computer science (CS) graduates than ever. At the same time, most CS departments across the country are only seeing 25–30% of women students in their classes, meaning that we are failing to draw interest from a large portion of the population. In this work, we explore the gender gap in CS at Rutgers University–New Brunswick, a large public R1 research university, using three data sets that span thousands of students across six academic years. Specifically, we combine these data sets to study the gender gaps in four core CS courses and explore the correlation of several factors with retention and the impact of these factors on changes to the gender gap as students proceed through the CS courses toward completing the CS major. For example, we find that a significant percentage of women students taking the introductory CS1 course for majors do not intend to major in CS, which may be a contributing factor to a large increase in the gender gap immediately after CS1. This finding implies that part of the retention task is attracting these women students to further explore the major. Results from our study include both novel findings and findings that are consistent with known challenges for increasing gender diversity in CS. In both cases, we provide extensive quantitative data in support of the findings.
Designing for Student-Directedness: How K–12 Teachers Utilize Peers to Support Projects
Student-directed projects—projects in which students have individual control over what they create and how to create it—are a promising practice for supporting the development of conceptual understanding and personal interest in K–12 computer science classrooms. In this article, we explore a central (and perhaps counterintuitive) design principle identified by a group of K–12 computer science teachers who support student-directed projects in their classrooms: in order for students to develop their own ideas and determine how to pursue them, students must have opportunities to engage with other students’ work. In this qualitative study, we investigated the instructional practices of 25 K–12 teachers using a series of in-depth, semi-structured interviews to develop understandings of how they used peer work to support student-directed projects in their classrooms. Teachers described supporting their students in navigating three stages of project development: generating ideas, pursuing ideas, and presenting ideas. For each of these three stages, teachers considered multiple factors to encourage engagement with peer work in their classrooms, including the quality and completeness of shared work and the modes of interaction with the work. We discuss how this pedagogical approach offers students new relationships to their own learning, to their peers, and to their teachers and communicates important messages to students about their own competence and agency, potentially contributing to aims within computer science for broadening participation.
Creativity in CS1: A Literature Review
Computer science is a fast-growing field in today’s digitized age, and working in this industry often requires creativity and innovative thought. An issue within computer science education, however, is that large introductory programming courses often involve little opportunity for creative thinking within coursework. The undergraduate introductory programming course (CS1) is notorious for its poor student performance and retention rates across multiple institutions. Integrating opportunities for creative thinking may help combat this issue by adding a personal touch to course content, which could allow beginner CS students to better relate to the abstract world of programming. Research on the role of creativity in computer science education (CSE) is an interesting area with a lot of room for exploration due to the complexity of the phenomenon of creativity as well as the CSE research field being fairly new compared to some other education fields where this topic has been more closely explored. To contribute to this area of research, this article provides a literature review exploring the concept of creativity as relevant to computer science education and CS1 in particular. Based on the review of the literature, we conclude creativity is an essential component to computer science, and the type of creativity that computer science requires is in fact, a teachable skill through the use of various tools and strategies. These strategies include the integration of open-ended assignments, large collaborative projects, learning by teaching, multimedia projects, small creative computational exercises, game development projects, digitally produced art, robotics, digital story-telling, music manipulation, and project-based learning. Research on each of these strategies and their effects on student experiences within CS1 is discussed in this review. Last, six main components of creativity-enhancing activities are identified based on the studies about incorporating creativity into CS1. These components are as follows: Collaboration, Relevance, Autonomy, Ownership, Hands-On Learning, and Visual Feedback. The purpose of this article is to contribute to computer science educators’ understanding of how creativity is best understood in the context of computer science education and explore practical applications of creativity theory in CS1 classrooms. This is an important collection of information for restructuring aspects of future introductory programming courses in creative, innovative ways that benefit student learning.
CATS: Customizable Abstractive Topic-based Summarization
Neural sequence-to-sequence models are the state-of-the-art approach used in abstractive summarization of textual documents, useful for producing condensed versions of source text narratives without being restricted to using only words from the original text. Despite the advances in abstractive summarization, custom generation of summaries (e.g., towards a user’s preference) remains unexplored. In this article, we present CATS, an abstractive neural summarization model that summarizes content in a sequence-to-sequence fashion while also introducing a new mechanism to control the underlying latent topic distribution of the produced summaries. We empirically illustrate the efficacy of our model in producing customized summaries and present findings that facilitate the design of such systems. We use the well-known CNN/DailyMail dataset to evaluate our model. Furthermore, we present a transfer-learning method and demonstrate the effectiveness of our approach in a low resource setting, i.e., abstractive summarization of meetings minutes, where combining the main available meetings’ transcripts datasets, AMI and International Computer Science Institute(ICSI) , results in merely a few hundred training documents.
Exploring students’ and lecturers’ views on collaboration and cooperation in computer science courses - a qualitative analysis
Factors affecting student educational choices regarding oer material in computer science, export citation format, share document.
We want to hear from you! Fill out the Library's User Survey and enter to win.
Computer Science: Find papers
- Find papers
- Access papers
- Evaluate Information
- Video tutorials and code repositories
- Cite and write
- Reading List
- Indigenous research & resources
Computer science research databases
Find journal articles using these research databases:.
- ACM Digital Library Conference proceedings, books, articles, magazines, newsletters, and multimedia titles relating to computing and information technology. Coverage: 1947 - present more... less... ACM Digital Library contains bibliographic information on ACM publications since 1947 and includes full-text of documents where available. Works published by affiliated organizations are included on a selected basis.
- IEEE Xplore Digital Library Resources for computer science, electrical engineering, electronics engineering, and mechanical engineering. Coverage: 1988 - present more... less... IEEE Xplore provides access to journals, annual conference proceedings, and IEEE technical standards.
- DBLP Computer Science Bibliography From the University of Trier, the "DBLP indexes more than 2.6 million articles and contains many links to home pages of computer scientists."
- ACL Anthology Browse through papers on the study of computational linguistics and natural language processing
- Eurographics Digital Library From the European Association for Computer Graphics.
- CiteSeerX A scientific literature digital library and search engine focusing on literature in computer and information science. Coverage: varies more... less... CiteSeerX attempts to provide algorithms, metadata, services, techniques, and software that can be used in other digital libraries. CiteSeer indexes PostScript and PDF research articles on the Web.
News and Business
- ABI/INFORM ProQuest access to articles on business and management from U.S. and international journals and trade magazines, including company histories, competitive intelligence, and product development. Coverage: 1971 - present more... less... Includes scholarly journals, trade magazines, working papers, market research, and company profiles.
- Factiva News, business magazines, trade journals, newsletters, and television and radio transcripts. Coverage: 1970s - present. Varies by publication more... less... Factiva forbids storing or using search results in research applications such as data mining or trend analysis. Note: If you are at a public workstation you will be asked for your WatIAM (Quest) User ID and Password.
- Nexis Uni News, legal, and business sources from around the world, Find news, legislation, case law, statutes, company profiles, market & industry reports. Coverage: 1970s - present more... less... Note: If you are at a public workstation you will be asked for your WatIAM (Quest) User ID and Password.
Multidisciplinary research databases
Not sure where to start? The databases below cover many disciplines including math, business, economics, health, life science, physical science, and technology.
- Scopus Peer-reviewed literature from scientific journals, books and conference proceedings, covering the fields of science, technology, medicine, social sciences, and arts and humanities. Coverage: 1966 - present
- Web of Science Articles and citations in the sciences, social sciences, arts, and humanities. Coverage: Varies more... less... Web of Science is comprised of several databases. The Science Citation Index Expanded (SCI) covers journals in the medical, physical and natural sciences, and engineering fields. The entire database extends back to 1899. The Social Sciences Citation Index (SSCI) covers journals in the social sciences. The entire database extends back to 1898. The Arts & Humanities Citation Index (AHCI) covers journals in the arts and humanities. It also selectively covers relevant items from science and technical journals. The entire database extends back to 1975.
- Google Scholar Google Scholar is a search engine finds scholarly information from many sources (however, not everything in Google Scholar is scholarly). To access materials paid for by your library, go to Google Scholar, then choose Settings and click "Library Links" to add the University of Waterloo. more... less... Google Scholar is a search engine that emphasizes scholarly information, particularly in the sciences and technology. It draws from academic publishers, professional societies, preprint repositories and universities. Note: Access To access materials paid for by your library, go to Google Scholar, then choose Settings and click "Library Links." The off-campus user will first need to login via "Get access from anywhere."
- arXiv A pre-print server which hosts papers (that have not been peer reviewed) relating to physics, mathematics, computer science, nonlinear sciences, qualitative biology and statistics Coverage: 1991 - present
- JSTOR Provides access to back issues of journals in the humanities, social sciences, and physical sciences, many of which date from the 1800s. Coverage: varies (excludes current 3 to 5 years)
- ProQuest A platform with many databases of journal indexes and abstracts, as well as some with full text Coverage: Varies more... less... This online platform hosts multiple resources.
- EBSCOhost A platform with many databases of journal indexes and abstracts, as well as some with full text Coverage: varies more... less... This online platform hosts multiple resources. Note: Offline digital lending: Requires Adobe Digital Editions.
Standards and Codes
- Standards & Codes by Ryan Ball Last Updated May 9, 2024 1225 views this year
Finding research databases for other disciplines
- Research guides at Waterloo Guides are created for each department on campus and list subject resources for each discipline. Find research in Computer Science, Engineering, Physics, Biology, Business, Psychology, Education, and more.
- << Previous: Home
- Next: Access papers >>
- Last Updated: Apr 19, 2024 8:08 AM
- URL: https://subjectguides.uwaterloo.ca/compsci
Research guides by subject
Course reserves
My library account
Book a study room
News and events
Work for the library
Support the library
We want to hear from you. You're viewing the newest version of the Library's website. Please send us your feedback !
- Contact Waterloo
- Maps & Directions
- Accessibility
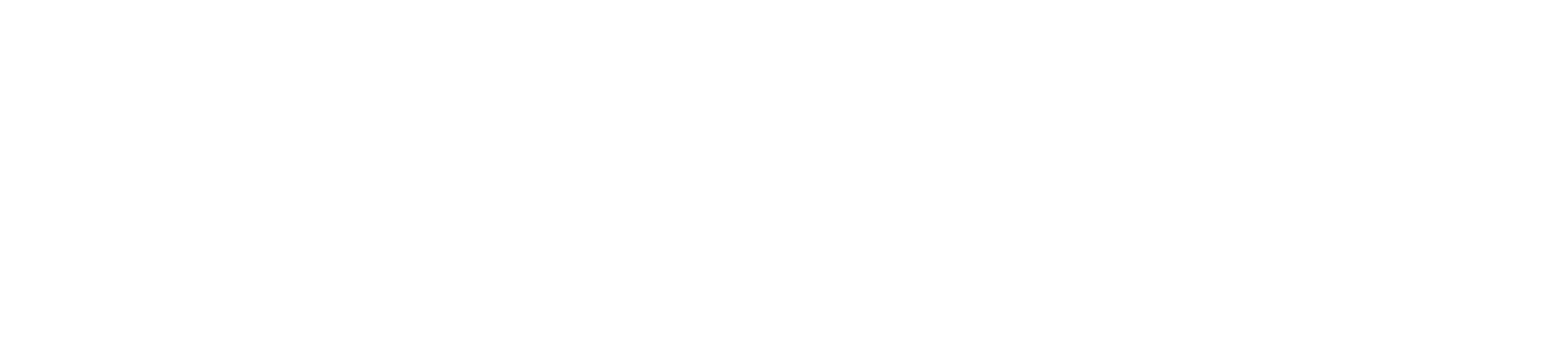
We gratefully acknowledge support from the Simons Foundation, member institutions, and all contributors.
- Accessibility
- Status Information
- Ancillary Files (data, code, images)
- Availability of submissions
- Category cross listing
- Endorsement
- Adding Journal Reference and DOI
- Text Overlap
- Metadata for Required and Optional Fields
- Submit a new version of a work
- Oversized Submissions
- Submit a Paper List for Conference Proceedings
- Creating tar and zip Files for Upload
- What is TeX
- Proxy / Third Party Submission
- Translations
- Version Availability
- Why Submit TeX?
- Withdraw / Retract a Submission
- Institutional Repository Interoperability
- Automated DOI and journal reference updates from publishers
- arXiv Usage Stats
Computer Science archive
Welcome to the Computing Research Repository (CoRR) in arXiv. The Computer Science section of arXiv was established in 1998 through a partnership of the Association for Computing Machinery, the Networked Computer Science Technical Reference Library, and arXiv.
You can view the subject category descriptions and browse papers from the main CS archive page . New readers and authors to arXiv should see our help pages for registration , submission and subscription .
The moderators for the computer science archive are listed here .
Computer Science Section Editorial Committee
The editorial committee members serve as consultants to Cornell University and to the arXiv Editorial Advisory Council . All arXiv policy decisions are ultimately made by Cornell University.
- Thomas Dietterich, University of Oregon (chair)
- Krzysztof Apt, Centrum Wiskunde & Informatica, and University of Amsterdam
- Ron Boisvert, National Institute of Standards and Technology
- Carol Hutchins, New York University
- Scott Delman, Association for Computing Machinery
- Jon Doyle, North Carolina State
- Ed Fox, Virginia Tech
- Lee Giles, The Pennsylvania State University
- Joseph Halpern, Cornell University
- Michael Lesk, Rutgers University
- Andrew McCallum, University of Massachusetts, Amherst
- Steve Minton, InferLink
- Andrew Odlyzko, University of Minnesota
- Michael O'Donnell, University of Chicago
- Erik Sandewall, Linköping University, Sweden
- Stuart Shieber, Harvard University
- Jeff Ullman, Stanford University
- Privacy Policy
- contact arXiv Click here to contact arXiv Contact
- subscribe to arXiv mailings Click here to subscribe Subscribe
- Report an issue Click here to report an issue with arXiv's documentation in github Report a documentation issue
- Web Accessibility Assistance
arXiv Operational Status Get status notifications via email or slack
Advertisement
Machine Learning: Algorithms, Real-World Applications and Research Directions
- Review Article
- Published: 22 March 2021
- Volume 2 , article number 160 , ( 2021 )
Cite this article
- Iqbal H. Sarker ORCID: orcid.org/0000-0003-1740-5517 1 , 2
506k Accesses
1443 Citations
23 Altmetric
Explore all metrics
In the current age of the Fourth Industrial Revolution (4 IR or Industry 4.0), the digital world has a wealth of data, such as Internet of Things (IoT) data, cybersecurity data, mobile data, business data, social media data, health data, etc. To intelligently analyze these data and develop the corresponding smart and automated applications, the knowledge of artificial intelligence (AI), particularly, machine learning (ML) is the key. Various types of machine learning algorithms such as supervised, unsupervised, semi-supervised, and reinforcement learning exist in the area. Besides, the deep learning , which is part of a broader family of machine learning methods, can intelligently analyze the data on a large scale. In this paper, we present a comprehensive view on these machine learning algorithms that can be applied to enhance the intelligence and the capabilities of an application. Thus, this study’s key contribution is explaining the principles of different machine learning techniques and their applicability in various real-world application domains, such as cybersecurity systems, smart cities, healthcare, e-commerce, agriculture, and many more. We also highlight the challenges and potential research directions based on our study. Overall, this paper aims to serve as a reference point for both academia and industry professionals as well as for decision-makers in various real-world situations and application areas, particularly from the technical point of view.
Similar content being viewed by others
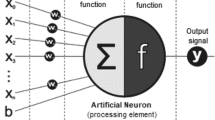
Deep Learning: A Comprehensive Overview on Techniques, Taxonomy, Applications and Research Directions
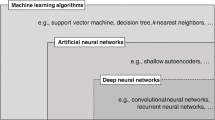
Machine learning and deep learning
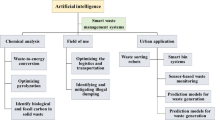

Artificial intelligence for waste management in smart cities: a review
Avoid common mistakes on your manuscript.
Introduction
We live in the age of data, where everything around us is connected to a data source, and everything in our lives is digitally recorded [ 21 , 103 ]. For instance, the current electronic world has a wealth of various kinds of data, such as the Internet of Things (IoT) data, cybersecurity data, smart city data, business data, smartphone data, social media data, health data, COVID-19 data, and many more. The data can be structured, semi-structured, or unstructured, discussed briefly in Sect. “ Types of Real-World Data and Machine Learning Techniques ”, which is increasing day-by-day. Extracting insights from these data can be used to build various intelligent applications in the relevant domains. For instance, to build a data-driven automated and intelligent cybersecurity system, the relevant cybersecurity data can be used [ 105 ]; to build personalized context-aware smart mobile applications, the relevant mobile data can be used [ 103 ], and so on. Thus, the data management tools and techniques having the capability of extracting insights or useful knowledge from the data in a timely and intelligent way is urgently needed, on which the real-world applications are based.
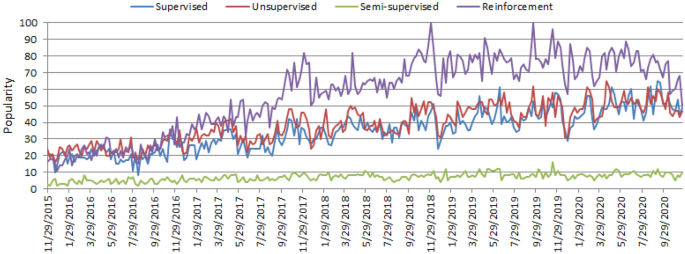
The worldwide popularity score of various types of ML algorithms (supervised, unsupervised, semi-supervised, and reinforcement) in a range of 0 (min) to 100 (max) over time where x-axis represents the timestamp information and y-axis represents the corresponding score
Artificial intelligence (AI), particularly, machine learning (ML) have grown rapidly in recent years in the context of data analysis and computing that typically allows the applications to function in an intelligent manner [ 95 ]. ML usually provides systems with the ability to learn and enhance from experience automatically without being specifically programmed and is generally referred to as the most popular latest technologies in the fourth industrial revolution (4 IR or Industry 4.0) [ 103 , 105 ]. “Industry 4.0” [ 114 ] is typically the ongoing automation of conventional manufacturing and industrial practices, including exploratory data processing, using new smart technologies such as machine learning automation. Thus, to intelligently analyze these data and to develop the corresponding real-world applications, machine learning algorithms is the key. The learning algorithms can be categorized into four major types, such as supervised, unsupervised, semi-supervised, and reinforcement learning in the area [ 75 ], discussed briefly in Sect. “ Types of Real-World Data and Machine Learning Techniques ”. The popularity of these approaches to learning is increasing day-by-day, which is shown in Fig. 1 , based on data collected from Google Trends [ 4 ] over the last five years. The x - axis of the figure indicates the specific dates and the corresponding popularity score within the range of \(0 \; (minimum)\) to \(100 \; (maximum)\) has been shown in y - axis . According to Fig. 1 , the popularity indication values for these learning types are low in 2015 and are increasing day by day. These statistics motivate us to study on machine learning in this paper, which can play an important role in the real-world through Industry 4.0 automation.
In general, the effectiveness and the efficiency of a machine learning solution depend on the nature and characteristics of data and the performance of the learning algorithms . In the area of machine learning algorithms, classification analysis, regression, data clustering, feature engineering and dimensionality reduction, association rule learning, or reinforcement learning techniques exist to effectively build data-driven systems [ 41 , 125 ]. Besides, deep learning originated from the artificial neural network that can be used to intelligently analyze data, which is known as part of a wider family of machine learning approaches [ 96 ]. Thus, selecting a proper learning algorithm that is suitable for the target application in a particular domain is challenging. The reason is that the purpose of different learning algorithms is different, even the outcome of different learning algorithms in a similar category may vary depending on the data characteristics [ 106 ]. Thus, it is important to understand the principles of various machine learning algorithms and their applicability to apply in various real-world application areas, such as IoT systems, cybersecurity services, business and recommendation systems, smart cities, healthcare and COVID-19, context-aware systems, sustainable agriculture, and many more that are explained briefly in Sect. “ Applications of Machine Learning ”.
Based on the importance and potentiality of “Machine Learning” to analyze the data mentioned above, in this paper, we provide a comprehensive view on various types of machine learning algorithms that can be applied to enhance the intelligence and the capabilities of an application. Thus, the key contribution of this study is explaining the principles and potentiality of different machine learning techniques, and their applicability in various real-world application areas mentioned earlier. The purpose of this paper is, therefore, to provide a basic guide for those academia and industry people who want to study, research, and develop data-driven automated and intelligent systems in the relevant areas based on machine learning techniques.
The key contributions of this paper are listed as follows:
To define the scope of our study by taking into account the nature and characteristics of various types of real-world data and the capabilities of various learning techniques.
To provide a comprehensive view on machine learning algorithms that can be applied to enhance the intelligence and capabilities of a data-driven application.
To discuss the applicability of machine learning-based solutions in various real-world application domains.
To highlight and summarize the potential research directions within the scope of our study for intelligent data analysis and services.
The rest of the paper is organized as follows. The next section presents the types of data and machine learning algorithms in a broader sense and defines the scope of our study. We briefly discuss and explain different machine learning algorithms in the subsequent section followed by which various real-world application areas based on machine learning algorithms are discussed and summarized. In the penultimate section, we highlight several research issues and potential future directions, and the final section concludes this paper.
Types of Real-World Data and Machine Learning Techniques
Machine learning algorithms typically consume and process data to learn the related patterns about individuals, business processes, transactions, events, and so on. In the following, we discuss various types of real-world data as well as categories of machine learning algorithms.
Types of Real-World Data
Usually, the availability of data is considered as the key to construct a machine learning model or data-driven real-world systems [ 103 , 105 ]. Data can be of various forms, such as structured, semi-structured, or unstructured [ 41 , 72 ]. Besides, the “metadata” is another type that typically represents data about the data. In the following, we briefly discuss these types of data.
Structured: It has a well-defined structure, conforms to a data model following a standard order, which is highly organized and easily accessed, and used by an entity or a computer program. In well-defined schemes, such as relational databases, structured data are typically stored, i.e., in a tabular format. For instance, names, dates, addresses, credit card numbers, stock information, geolocation, etc. are examples of structured data.
Unstructured: On the other hand, there is no pre-defined format or organization for unstructured data, making it much more difficult to capture, process, and analyze, mostly containing text and multimedia material. For example, sensor data, emails, blog entries, wikis, and word processing documents, PDF files, audio files, videos, images, presentations, web pages, and many other types of business documents can be considered as unstructured data.
Semi-structured: Semi-structured data are not stored in a relational database like the structured data mentioned above, but it does have certain organizational properties that make it easier to analyze. HTML, XML, JSON documents, NoSQL databases, etc., are some examples of semi-structured data.
Metadata: It is not the normal form of data, but “data about data”. The primary difference between “data” and “metadata” is that data are simply the material that can classify, measure, or even document something relative to an organization’s data properties. On the other hand, metadata describes the relevant data information, giving it more significance for data users. A basic example of a document’s metadata might be the author, file size, date generated by the document, keywords to define the document, etc.
In the area of machine learning and data science, researchers use various widely used datasets for different purposes. These are, for example, cybersecurity datasets such as NSL-KDD [ 119 ], UNSW-NB15 [ 76 ], ISCX’12 [ 1 ], CIC-DDoS2019 [ 2 ], Bot-IoT [ 59 ], etc., smartphone datasets such as phone call logs [ 84 , 101 ], SMS Log [ 29 ], mobile application usages logs [ 137 ] [ 117 ], mobile phone notification logs [ 73 ] etc., IoT data [ 16 , 57 , 62 ], agriculture and e-commerce data [ 120 , 138 ], health data such as heart disease [ 92 ], diabetes mellitus [ 83 , 134 ], COVID-19 [ 43 , 74 ], etc., and many more in various application domains. The data can be in different types discussed above, which may vary from application to application in the real world. To analyze such data in a particular problem domain, and to extract the insights or useful knowledge from the data for building the real-world intelligent applications, different types of machine learning techniques can be used according to their learning capabilities, which is discussed in the following.
Types of Machine Learning Techniques
Machine Learning algorithms are mainly divided into four categories: Supervised learning, Unsupervised learning, Semi-supervised learning, and Reinforcement learning [ 75 ], as shown in Fig. 2 . In the following, we briefly discuss each type of learning technique with the scope of their applicability to solve real-world problems.
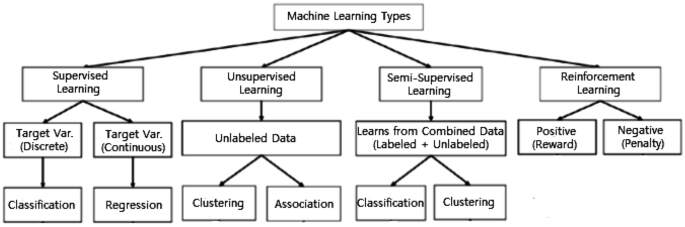
Various types of machine learning techniques
Supervised: Supervised learning is typically the task of machine learning to learn a function that maps an input to an output based on sample input-output pairs [ 41 ]. It uses labeled training data and a collection of training examples to infer a function. Supervised learning is carried out when certain goals are identified to be accomplished from a certain set of inputs [ 105 ], i.e., a task-driven approach . The most common supervised tasks are “classification” that separates the data, and “regression” that fits the data. For instance, predicting the class label or sentiment of a piece of text, like a tweet or a product review, i.e., text classification, is an example of supervised learning.
Unsupervised: Unsupervised learning analyzes unlabeled datasets without the need for human interference, i.e., a data-driven process [ 41 ]. This is widely used for extracting generative features, identifying meaningful trends and structures, groupings in results, and exploratory purposes. The most common unsupervised learning tasks are clustering, density estimation, feature learning, dimensionality reduction, finding association rules, anomaly detection, etc.
Semi-supervised: Semi-supervised learning can be defined as a hybridization of the above-mentioned supervised and unsupervised methods, as it operates on both labeled and unlabeled data [ 41 , 105 ]. Thus, it falls between learning “without supervision” and learning “with supervision”. In the real world, labeled data could be rare in several contexts, and unlabeled data are numerous, where semi-supervised learning is useful [ 75 ]. The ultimate goal of a semi-supervised learning model is to provide a better outcome for prediction than that produced using the labeled data alone from the model. Some application areas where semi-supervised learning is used include machine translation, fraud detection, labeling data and text classification.
Reinforcement: Reinforcement learning is a type of machine learning algorithm that enables software agents and machines to automatically evaluate the optimal behavior in a particular context or environment to improve its efficiency [ 52 ], i.e., an environment-driven approach . This type of learning is based on reward or penalty, and its ultimate goal is to use insights obtained from environmental activists to take action to increase the reward or minimize the risk [ 75 ]. It is a powerful tool for training AI models that can help increase automation or optimize the operational efficiency of sophisticated systems such as robotics, autonomous driving tasks, manufacturing and supply chain logistics, however, not preferable to use it for solving the basic or straightforward problems.
Thus, to build effective models in various application areas different types of machine learning techniques can play a significant role according to their learning capabilities, depending on the nature of the data discussed earlier, and the target outcome. In Table 1 , we summarize various types of machine learning techniques with examples. In the following, we provide a comprehensive view of machine learning algorithms that can be applied to enhance the intelligence and capabilities of a data-driven application.
Machine Learning Tasks and Algorithms
In this section, we discuss various machine learning algorithms that include classification analysis, regression analysis, data clustering, association rule learning, feature engineering for dimensionality reduction, as well as deep learning methods. A general structure of a machine learning-based predictive model has been shown in Fig. 3 , where the model is trained from historical data in phase 1 and the outcome is generated in phase 2 for the new test data.
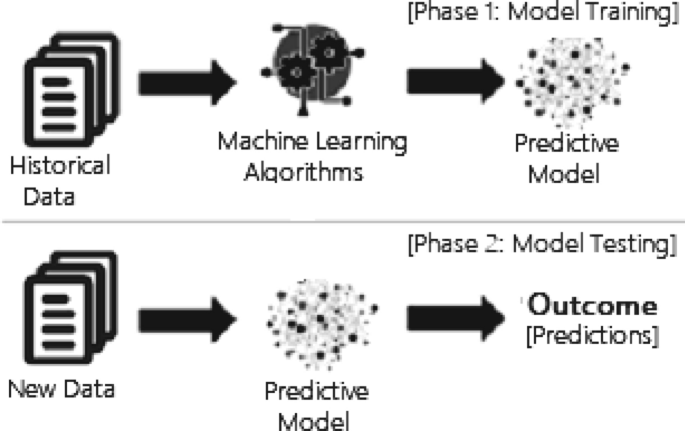
A general structure of a machine learning based predictive model considering both the training and testing phase
Classification Analysis
Classification is regarded as a supervised learning method in machine learning, referring to a problem of predictive modeling as well, where a class label is predicted for a given example [ 41 ]. Mathematically, it maps a function ( f ) from input variables ( X ) to output variables ( Y ) as target, label or categories. To predict the class of given data points, it can be carried out on structured or unstructured data. For example, spam detection such as “spam” and “not spam” in email service providers can be a classification problem. In the following, we summarize the common classification problems.
Binary classification: It refers to the classification tasks having two class labels such as “true and false” or “yes and no” [ 41 ]. In such binary classification tasks, one class could be the normal state, while the abnormal state could be another class. For instance, “cancer not detected” is the normal state of a task that involves a medical test, and “cancer detected” could be considered as the abnormal state. Similarly, “spam” and “not spam” in the above example of email service providers are considered as binary classification.
Multiclass classification: Traditionally, this refers to those classification tasks having more than two class labels [ 41 ]. The multiclass classification does not have the principle of normal and abnormal outcomes, unlike binary classification tasks. Instead, within a range of specified classes, examples are classified as belonging to one. For example, it can be a multiclass classification task to classify various types of network attacks in the NSL-KDD [ 119 ] dataset, where the attack categories are classified into four class labels, such as DoS (Denial of Service Attack), U2R (User to Root Attack), R2L (Root to Local Attack), and Probing Attack.
Multi-label classification: In machine learning, multi-label classification is an important consideration where an example is associated with several classes or labels. Thus, it is a generalization of multiclass classification, where the classes involved in the problem are hierarchically structured, and each example may simultaneously belong to more than one class in each hierarchical level, e.g., multi-level text classification. For instance, Google news can be presented under the categories of a “city name”, “technology”, or “latest news”, etc. Multi-label classification includes advanced machine learning algorithms that support predicting various mutually non-exclusive classes or labels, unlike traditional classification tasks where class labels are mutually exclusive [ 82 ].
Many classification algorithms have been proposed in the machine learning and data science literature [ 41 , 125 ]. In the following, we summarize the most common and popular methods that are used widely in various application areas.
Naive Bayes (NB): The naive Bayes algorithm is based on the Bayes’ theorem with the assumption of independence between each pair of features [ 51 ]. It works well and can be used for both binary and multi-class categories in many real-world situations, such as document or text classification, spam filtering, etc. To effectively classify the noisy instances in the data and to construct a robust prediction model, the NB classifier can be used [ 94 ]. The key benefit is that, compared to more sophisticated approaches, it needs a small amount of training data to estimate the necessary parameters and quickly [ 82 ]. However, its performance may affect due to its strong assumptions on features independence. Gaussian, Multinomial, Complement, Bernoulli, and Categorical are the common variants of NB classifier [ 82 ].
Linear Discriminant Analysis (LDA): Linear Discriminant Analysis (LDA) is a linear decision boundary classifier created by fitting class conditional densities to data and applying Bayes’ rule [ 51 , 82 ]. This method is also known as a generalization of Fisher’s linear discriminant, which projects a given dataset into a lower-dimensional space, i.e., a reduction of dimensionality that minimizes the complexity of the model or reduces the resulting model’s computational costs. The standard LDA model usually suits each class with a Gaussian density, assuming that all classes share the same covariance matrix [ 82 ]. LDA is closely related to ANOVA (analysis of variance) and regression analysis, which seek to express one dependent variable as a linear combination of other features or measurements.
Logistic regression (LR): Another common probabilistic based statistical model used to solve classification issues in machine learning is Logistic Regression (LR) [ 64 ]. Logistic regression typically uses a logistic function to estimate the probabilities, which is also referred to as the mathematically defined sigmoid function in Eq. 1 . It can overfit high-dimensional datasets and works well when the dataset can be separated linearly. The regularization (L1 and L2) techniques [ 82 ] can be used to avoid over-fitting in such scenarios. The assumption of linearity between the dependent and independent variables is considered as a major drawback of Logistic Regression. It can be used for both classification and regression problems, but it is more commonly used for classification.
K-nearest neighbors (KNN): K-Nearest Neighbors (KNN) [ 9 ] is an “instance-based learning” or non-generalizing learning, also known as a “lazy learning” algorithm. It does not focus on constructing a general internal model; instead, it stores all instances corresponding to training data in n -dimensional space. KNN uses data and classifies new data points based on similarity measures (e.g., Euclidean distance function) [ 82 ]. Classification is computed from a simple majority vote of the k nearest neighbors of each point. It is quite robust to noisy training data, and accuracy depends on the data quality. The biggest issue with KNN is to choose the optimal number of neighbors to be considered. KNN can be used both for classification as well as regression.
Support vector machine (SVM): In machine learning, another common technique that can be used for classification, regression, or other tasks is a support vector machine (SVM) [ 56 ]. In high- or infinite-dimensional space, a support vector machine constructs a hyper-plane or set of hyper-planes. Intuitively, the hyper-plane, which has the greatest distance from the nearest training data points in any class, achieves a strong separation since, in general, the greater the margin, the lower the classifier’s generalization error. It is effective in high-dimensional spaces and can behave differently based on different mathematical functions known as the kernel. Linear, polynomial, radial basis function (RBF), sigmoid, etc., are the popular kernel functions used in SVM classifier [ 82 ]. However, when the data set contains more noise, such as overlapping target classes, SVM does not perform well.
Decision tree (DT): Decision tree (DT) [ 88 ] is a well-known non-parametric supervised learning method. DT learning methods are used for both the classification and regression tasks [ 82 ]. ID3 [ 87 ], C4.5 [ 88 ], and CART [ 20 ] are well known for DT algorithms. Moreover, recently proposed BehavDT [ 100 ], and IntrudTree [ 97 ] by Sarker et al. are effective in the relevant application domains, such as user behavior analytics and cybersecurity analytics, respectively. By sorting down the tree from the root to some leaf nodes, as shown in Fig. 4 , DT classifies the instances. Instances are classified by checking the attribute defined by that node, starting at the root node of the tree, and then moving down the tree branch corresponding to the attribute value. For splitting, the most popular criteria are “gini” for the Gini impurity and “entropy” for the information gain that can be expressed mathematically as [ 82 ].
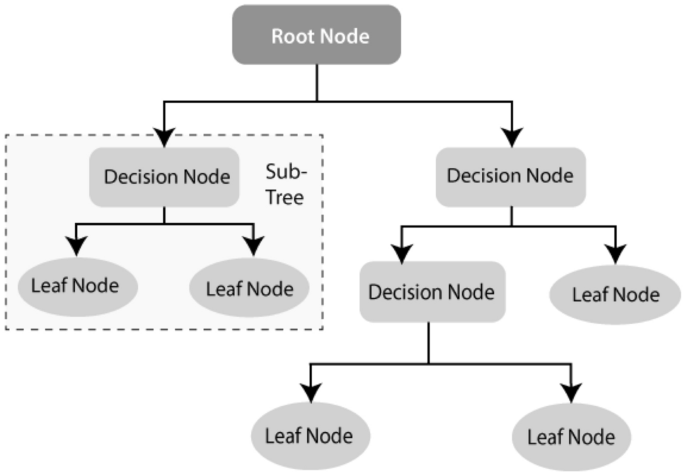
An example of a decision tree structure
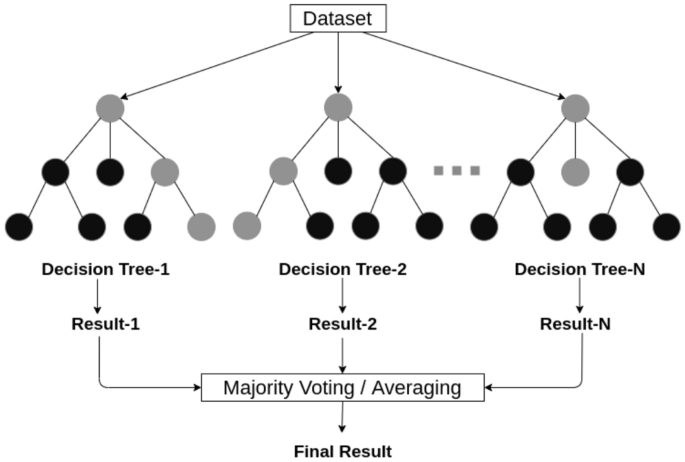
An example of a random forest structure considering multiple decision trees
Random forest (RF): A random forest classifier [ 19 ] is well known as an ensemble classification technique that is used in the field of machine learning and data science in various application areas. This method uses “parallel ensembling” which fits several decision tree classifiers in parallel, as shown in Fig. 5 , on different data set sub-samples and uses majority voting or averages for the outcome or final result. It thus minimizes the over-fitting problem and increases the prediction accuracy and control [ 82 ]. Therefore, the RF learning model with multiple decision trees is typically more accurate than a single decision tree based model [ 106 ]. To build a series of decision trees with controlled variation, it combines bootstrap aggregation (bagging) [ 18 ] and random feature selection [ 11 ]. It is adaptable to both classification and regression problems and fits well for both categorical and continuous values.
Adaptive Boosting (AdaBoost): Adaptive Boosting (AdaBoost) is an ensemble learning process that employs an iterative approach to improve poor classifiers by learning from their errors. This is developed by Yoav Freund et al. [ 35 ] and also known as “meta-learning”. Unlike the random forest that uses parallel ensembling, Adaboost uses “sequential ensembling”. It creates a powerful classifier by combining many poorly performing classifiers to obtain a good classifier of high accuracy. In that sense, AdaBoost is called an adaptive classifier by significantly improving the efficiency of the classifier, but in some instances, it can trigger overfits. AdaBoost is best used to boost the performance of decision trees, base estimator [ 82 ], on binary classification problems, however, is sensitive to noisy data and outliers.
Extreme gradient boosting (XGBoost): Gradient Boosting, like Random Forests [ 19 ] above, is an ensemble learning algorithm that generates a final model based on a series of individual models, typically decision trees. The gradient is used to minimize the loss function, similar to how neural networks [ 41 ] use gradient descent to optimize weights. Extreme Gradient Boosting (XGBoost) is a form of gradient boosting that takes more detailed approximations into account when determining the best model [ 82 ]. It computes second-order gradients of the loss function to minimize loss and advanced regularization (L1 and L2) [ 82 ], which reduces over-fitting, and improves model generalization and performance. XGBoost is fast to interpret and can handle large-sized datasets well.
Stochastic gradient descent (SGD): Stochastic gradient descent (SGD) [ 41 ] is an iterative method for optimizing an objective function with appropriate smoothness properties, where the word ‘stochastic’ refers to random probability. This reduces the computational burden, particularly in high-dimensional optimization problems, allowing for faster iterations in exchange for a lower convergence rate. A gradient is the slope of a function that calculates a variable’s degree of change in response to another variable’s changes. Mathematically, the Gradient Descent is a convex function whose output is a partial derivative of a set of its input parameters. Let, \(\alpha\) is the learning rate, and \(J_i\) is the training example cost of \(i \mathrm{th}\) , then Eq. ( 4 ) represents the stochastic gradient descent weight update method at the \(j^\mathrm{th}\) iteration. In large-scale and sparse machine learning, SGD has been successfully applied to problems often encountered in text classification and natural language processing [ 82 ]. However, SGD is sensitive to feature scaling and needs a range of hyperparameters, such as the regularization parameter and the number of iterations.
Rule-based classification : The term rule-based classification can be used to refer to any classification scheme that makes use of IF-THEN rules for class prediction. Several classification algorithms such as Zero-R [ 125 ], One-R [ 47 ], decision trees [ 87 , 88 ], DTNB [ 110 ], Ripple Down Rule learner (RIDOR) [ 125 ], Repeated Incremental Pruning to Produce Error Reduction (RIPPER) [ 126 ] exist with the ability of rule generation. The decision tree is one of the most common rule-based classification algorithms among these techniques because it has several advantages, such as being easier to interpret; the ability to handle high-dimensional data; simplicity and speed; good accuracy; and the capability to produce rules for human clear and understandable classification [ 127 ] [ 128 ]. The decision tree-based rules also provide significant accuracy in a prediction model for unseen test cases [ 106 ]. Since the rules are easily interpretable, these rule-based classifiers are often used to produce descriptive models that can describe a system including the entities and their relationships.
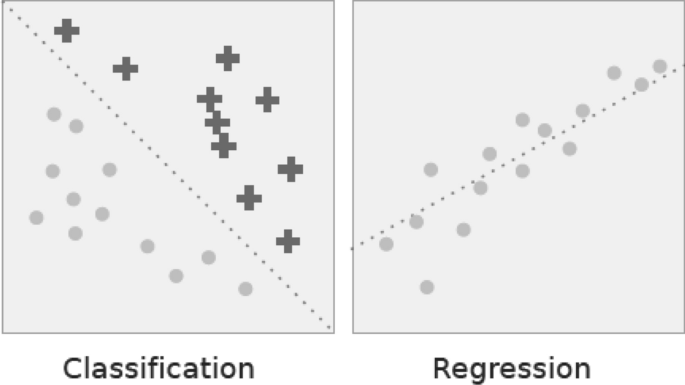
Classification vs. regression. In classification the dotted line represents a linear boundary that separates the two classes; in regression, the dotted line models the linear relationship between the two variables
Regression Analysis
Regression analysis includes several methods of machine learning that allow to predict a continuous ( y ) result variable based on the value of one or more ( x ) predictor variables [ 41 ]. The most significant distinction between classification and regression is that classification predicts distinct class labels, while regression facilitates the prediction of a continuous quantity. Figure 6 shows an example of how classification is different with regression models. Some overlaps are often found between the two types of machine learning algorithms. Regression models are now widely used in a variety of fields, including financial forecasting or prediction, cost estimation, trend analysis, marketing, time series estimation, drug response modeling, and many more. Some of the familiar types of regression algorithms are linear, polynomial, lasso and ridge regression, etc., which are explained briefly in the following.
Simple and multiple linear regression: This is one of the most popular ML modeling techniques as well as a well-known regression technique. In this technique, the dependent variable is continuous, the independent variable(s) can be continuous or discrete, and the form of the regression line is linear. Linear regression creates a relationship between the dependent variable ( Y ) and one or more independent variables ( X ) (also known as regression line) using the best fit straight line [ 41 ]. It is defined by the following equations:
where a is the intercept, b is the slope of the line, and e is the error term. This equation can be used to predict the value of the target variable based on the given predictor variable(s). Multiple linear regression is an extension of simple linear regression that allows two or more predictor variables to model a response variable, y, as a linear function [ 41 ] defined in Eq. 6 , whereas simple linear regression has only 1 independent variable, defined in Eq. 5 .
Polynomial regression: Polynomial regression is a form of regression analysis in which the relationship between the independent variable x and the dependent variable y is not linear, but is the polynomial degree of \(n^\mathrm{th}\) in x [ 82 ]. The equation for polynomial regression is also derived from linear regression (polynomial regression of degree 1) equation, which is defined as below:
Here, y is the predicted/target output, \(b_0, b_1,... b_n\) are the regression coefficients, x is an independent/ input variable. In simple words, we can say that if data are not distributed linearly, instead it is \(n^\mathrm{th}\) degree of polynomial then we use polynomial regression to get desired output.
LASSO and ridge regression: LASSO and Ridge regression are well known as powerful techniques which are typically used for building learning models in presence of a large number of features, due to their capability to preventing over-fitting and reducing the complexity of the model. The LASSO (least absolute shrinkage and selection operator) regression model uses L 1 regularization technique [ 82 ] that uses shrinkage, which penalizes “absolute value of magnitude of coefficients” ( L 1 penalty). As a result, LASSO appears to render coefficients to absolute zero. Thus, LASSO regression aims to find the subset of predictors that minimizes the prediction error for a quantitative response variable. On the other hand, ridge regression uses L 2 regularization [ 82 ], which is the “squared magnitude of coefficients” ( L 2 penalty). Thus, ridge regression forces the weights to be small but never sets the coefficient value to zero, and does a non-sparse solution. Overall, LASSO regression is useful to obtain a subset of predictors by eliminating less important features, and ridge regression is useful when a data set has “multicollinearity” which refers to the predictors that are correlated with other predictors.
Cluster Analysis
Cluster analysis, also known as clustering, is an unsupervised machine learning technique for identifying and grouping related data points in large datasets without concern for the specific outcome. It does grouping a collection of objects in such a way that objects in the same category, called a cluster, are in some sense more similar to each other than objects in other groups [ 41 ]. It is often used as a data analysis technique to discover interesting trends or patterns in data, e.g., groups of consumers based on their behavior. In a broad range of application areas, such as cybersecurity, e-commerce, mobile data processing, health analytics, user modeling and behavioral analytics, clustering can be used. In the following, we briefly discuss and summarize various types of clustering methods.
Partitioning methods: Based on the features and similarities in the data, this clustering approach categorizes the data into multiple groups or clusters. The data scientists or analysts typically determine the number of clusters either dynamically or statically depending on the nature of the target applications, to produce for the methods of clustering. The most common clustering algorithms based on partitioning methods are K-means [ 69 ], K-Mediods [ 80 ], CLARA [ 55 ] etc.
Density-based methods: To identify distinct groups or clusters, it uses the concept that a cluster in the data space is a contiguous region of high point density isolated from other such clusters by contiguous regions of low point density. Points that are not part of a cluster are considered as noise. The typical clustering algorithms based on density are DBSCAN [ 32 ], OPTICS [ 12 ] etc. The density-based methods typically struggle with clusters of similar density and high dimensionality data.
Hierarchical-based methods: Hierarchical clustering typically seeks to construct a hierarchy of clusters, i.e., the tree structure. Strategies for hierarchical clustering generally fall into two types: (i) Agglomerative—a “bottom-up” approach in which each observation begins in its cluster and pairs of clusters are combined as one, moves up the hierarchy, and (ii) Divisive—a “top-down” approach in which all observations begin in one cluster and splits are performed recursively, moves down the hierarchy, as shown in Fig 7 . Our earlier proposed BOTS technique, Sarker et al. [ 102 ] is an example of a hierarchical, particularly, bottom-up clustering algorithm.
Grid-based methods: To deal with massive datasets, grid-based clustering is especially suitable. To obtain clusters, the principle is first to summarize the dataset with a grid representation and then to combine grid cells. STING [ 122 ], CLIQUE [ 6 ], etc. are the standard algorithms of grid-based clustering.
Model-based methods: There are mainly two types of model-based clustering algorithms: one that uses statistical learning, and the other based on a method of neural network learning [ 130 ]. For instance, GMM [ 89 ] is an example of a statistical learning method, and SOM [ 22 ] [ 96 ] is an example of a neural network learning method.
Constraint-based methods: Constrained-based clustering is a semi-supervised approach to data clustering that uses constraints to incorporate domain knowledge. Application or user-oriented constraints are incorporated to perform the clustering. The typical algorithms of this kind of clustering are COP K-means [ 121 ], CMWK-Means [ 27 ], etc.
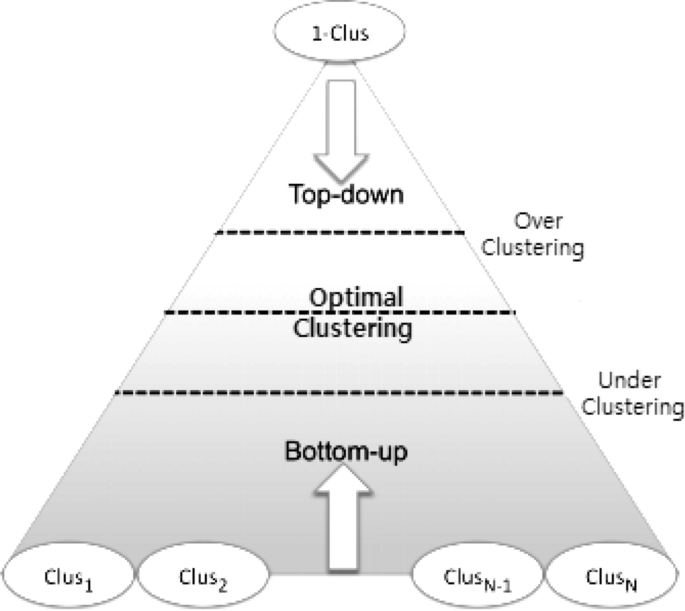
A graphical interpretation of the widely-used hierarchical clustering (Bottom-up and top-down) technique
Many clustering algorithms have been proposed with the ability to grouping data in machine learning and data science literature [ 41 , 125 ]. In the following, we summarize the popular methods that are used widely in various application areas.
K-means clustering: K-means clustering [ 69 ] is a fast, robust, and simple algorithm that provides reliable results when data sets are well-separated from each other. The data points are allocated to a cluster in this algorithm in such a way that the amount of the squared distance between the data points and the centroid is as small as possible. In other words, the K-means algorithm identifies the k number of centroids and then assigns each data point to the nearest cluster while keeping the centroids as small as possible. Since it begins with a random selection of cluster centers, the results can be inconsistent. Since extreme values can easily affect a mean, the K-means clustering algorithm is sensitive to outliers. K-medoids clustering [ 91 ] is a variant of K-means that is more robust to noises and outliers.
Mean-shift clustering: Mean-shift clustering [ 37 ] is a nonparametric clustering technique that does not require prior knowledge of the number of clusters or constraints on cluster shape. Mean-shift clustering aims to discover “blobs” in a smooth distribution or density of samples [ 82 ]. It is a centroid-based algorithm that works by updating centroid candidates to be the mean of the points in a given region. To form the final set of centroids, these candidates are filtered in a post-processing stage to remove near-duplicates. Cluster analysis in computer vision and image processing are examples of application domains. Mean Shift has the disadvantage of being computationally expensive. Moreover, in cases of high dimension, where the number of clusters shifts abruptly, the mean-shift algorithm does not work well.
DBSCAN: Density-based spatial clustering of applications with noise (DBSCAN) [ 32 ] is a base algorithm for density-based clustering which is widely used in data mining and machine learning. This is known as a non-parametric density-based clustering technique for separating high-density clusters from low-density clusters that are used in model building. DBSCAN’s main idea is that a point belongs to a cluster if it is close to many points from that cluster. It can find clusters of various shapes and sizes in a vast volume of data that is noisy and contains outliers. DBSCAN, unlike k-means, does not require a priori specification of the number of clusters in the data and can find arbitrarily shaped clusters. Although k-means is much faster than DBSCAN, it is efficient at finding high-density regions and outliers, i.e., is robust to outliers.
GMM clustering: Gaussian mixture models (GMMs) are often used for data clustering, which is a distribution-based clustering algorithm. A Gaussian mixture model is a probabilistic model in which all the data points are produced by a mixture of a finite number of Gaussian distributions with unknown parameters [ 82 ]. To find the Gaussian parameters for each cluster, an optimization algorithm called expectation-maximization (EM) [ 82 ] can be used. EM is an iterative method that uses a statistical model to estimate the parameters. In contrast to k-means, Gaussian mixture models account for uncertainty and return the likelihood that a data point belongs to one of the k clusters. GMM clustering is more robust than k-means and works well even with non-linear data distributions.
Agglomerative hierarchical clustering: The most common method of hierarchical clustering used to group objects in clusters based on their similarity is agglomerative clustering. This technique uses a bottom-up approach, where each object is first treated as a singleton cluster by the algorithm. Following that, pairs of clusters are merged one by one until all clusters have been merged into a single large cluster containing all objects. The result is a dendrogram, which is a tree-based representation of the elements. Single linkage [ 115 ], Complete linkage [ 116 ], BOTS [ 102 ] etc. are some examples of such techniques. The main advantage of agglomerative hierarchical clustering over k-means is that the tree-structure hierarchy generated by agglomerative clustering is more informative than the unstructured collection of flat clusters returned by k-means, which can help to make better decisions in the relevant application areas.
Dimensionality Reduction and Feature Learning
In machine learning and data science, high-dimensional data processing is a challenging task for both researchers and application developers. Thus, dimensionality reduction which is an unsupervised learning technique, is important because it leads to better human interpretations, lower computational costs, and avoids overfitting and redundancy by simplifying models. Both the process of feature selection and feature extraction can be used for dimensionality reduction. The primary distinction between the selection and extraction of features is that the “feature selection” keeps a subset of the original features [ 97 ], while “feature extraction” creates brand new ones [ 98 ]. In the following, we briefly discuss these techniques.
Feature selection: The selection of features, also known as the selection of variables or attributes in the data, is the process of choosing a subset of unique features (variables, predictors) to use in building machine learning and data science model. It decreases a model’s complexity by eliminating the irrelevant or less important features and allows for faster training of machine learning algorithms. A right and optimal subset of the selected features in a problem domain is capable to minimize the overfitting problem through simplifying and generalizing the model as well as increases the model’s accuracy [ 97 ]. Thus, “feature selection” [ 66 , 99 ] is considered as one of the primary concepts in machine learning that greatly affects the effectiveness and efficiency of the target machine learning model. Chi-squared test, Analysis of variance (ANOVA) test, Pearson’s correlation coefficient, recursive feature elimination, are some popular techniques that can be used for feature selection.
Feature extraction: In a machine learning-based model or system, feature extraction techniques usually provide a better understanding of the data, a way to improve prediction accuracy, and to reduce computational cost or training time. The aim of “feature extraction” [ 66 , 99 ] is to reduce the number of features in a dataset by generating new ones from the existing ones and then discarding the original features. The majority of the information found in the original set of features can then be summarized using this new reduced set of features. For instance, principal components analysis (PCA) is often used as a dimensionality-reduction technique to extract a lower-dimensional space creating new brand components from the existing features in a dataset [ 98 ].
Many algorithms have been proposed to reduce data dimensions in the machine learning and data science literature [ 41 , 125 ]. In the following, we summarize the popular methods that are used widely in various application areas.
Variance threshold: A simple basic approach to feature selection is the variance threshold [ 82 ]. This excludes all features of low variance, i.e., all features whose variance does not exceed the threshold. It eliminates all zero-variance characteristics by default, i.e., characteristics that have the same value in all samples. This feature selection algorithm looks only at the ( X ) features, not the ( y ) outputs needed, and can, therefore, be used for unsupervised learning.
Pearson correlation: Pearson’s correlation is another method to understand a feature’s relation to the response variable and can be used for feature selection [ 99 ]. This method is also used for finding the association between the features in a dataset. The resulting value is \([-1, 1]\) , where \(-1\) means perfect negative correlation, \(+1\) means perfect positive correlation, and 0 means that the two variables do not have a linear correlation. If two random variables represent X and Y , then the correlation coefficient between X and Y is defined as [ 41 ]
ANOVA: Analysis of variance (ANOVA) is a statistical tool used to verify the mean values of two or more groups that differ significantly from each other. ANOVA assumes a linear relationship between the variables and the target and the variables’ normal distribution. To statistically test the equality of means, the ANOVA method utilizes F tests. For feature selection, the results ‘ANOVA F value’ [ 82 ] of this test can be used where certain features independent of the goal variable can be omitted.
Chi square: The chi-square \({\chi }^2\) [ 82 ] statistic is an estimate of the difference between the effects of a series of events or variables observed and expected frequencies. The magnitude of the difference between the real and observed values, the degrees of freedom, and the sample size depends on \({\chi }^2\) . The chi-square \({\chi }^2\) is commonly used for testing relationships between categorical variables. If \(O_i\) represents observed value and \(E_i\) represents expected value, then
Recursive feature elimination (RFE): Recursive Feature Elimination (RFE) is a brute force approach to feature selection. RFE [ 82 ] fits the model and removes the weakest feature before it meets the specified number of features. Features are ranked by the coefficients or feature significance of the model. RFE aims to remove dependencies and collinearity in the model by recursively removing a small number of features per iteration.
Model-based selection: To reduce the dimensionality of the data, linear models penalized with the L 1 regularization can be used. Least absolute shrinkage and selection operator (Lasso) regression is a type of linear regression that has the property of shrinking some of the coefficients to zero [ 82 ]. Therefore, that feature can be removed from the model. Thus, the penalized lasso regression method, often used in machine learning to select the subset of variables. Extra Trees Classifier [ 82 ] is an example of a tree-based estimator that can be used to compute impurity-based function importance, which can then be used to discard irrelevant features.
Principal component analysis (PCA): Principal component analysis (PCA) is a well-known unsupervised learning approach in the field of machine learning and data science. PCA is a mathematical technique that transforms a set of correlated variables into a set of uncorrelated variables known as principal components [ 48 , 81 ]. Figure 8 shows an example of the effect of PCA on various dimensions space, where Fig. 8 a shows the original features in 3D space, and Fig. 8 b shows the created principal components PC1 and PC2 onto a 2D plane, and 1D line with the principal component PC1 respectively. Thus, PCA can be used as a feature extraction technique that reduces the dimensionality of the datasets, and to build an effective machine learning model [ 98 ]. Technically, PCA identifies the completely transformed with the highest eigenvalues of a covariance matrix and then uses those to project the data into a new subspace of equal or fewer dimensions [ 82 ].
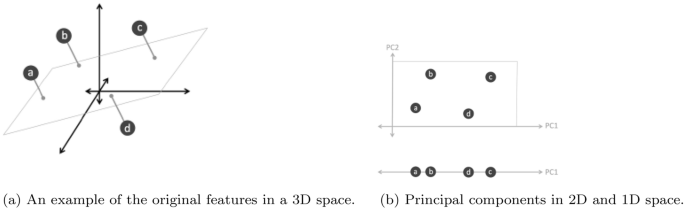
An example of a principal component analysis (PCA) and created principal components PC1 and PC2 in different dimension space
Association Rule Learning
Association rule learning is a rule-based machine learning approach to discover interesting relationships, “IF-THEN” statements, in large datasets between variables [ 7 ]. One example is that “if a customer buys a computer or laptop (an item), s/he is likely to also buy anti-virus software (another item) at the same time”. Association rules are employed today in many application areas, including IoT services, medical diagnosis, usage behavior analytics, web usage mining, smartphone applications, cybersecurity applications, and bioinformatics. In comparison to sequence mining, association rule learning does not usually take into account the order of things within or across transactions. A common way of measuring the usefulness of association rules is to use its parameter, the ‘support’ and ‘confidence’, which is introduced in [ 7 ].
In the data mining literature, many association rule learning methods have been proposed, such as logic dependent [ 34 ], frequent pattern based [ 8 , 49 , 68 ], and tree-based [ 42 ]. The most popular association rule learning algorithms are summarized below.
AIS and SETM: AIS is the first algorithm proposed by Agrawal et al. [ 7 ] for association rule mining. The AIS algorithm’s main downside is that too many candidate itemsets are generated, requiring more space and wasting a lot of effort. This algorithm calls for too many passes over the entire dataset to produce the rules. Another approach SETM [ 49 ] exhibits good performance and stable behavior with execution time; however, it suffers from the same flaw as the AIS algorithm.
Apriori: For generating association rules for a given dataset, Agrawal et al. [ 8 ] proposed the Apriori, Apriori-TID, and Apriori-Hybrid algorithms. These later algorithms outperform the AIS and SETM mentioned above due to the Apriori property of frequent itemset [ 8 ]. The term ‘Apriori’ usually refers to having prior knowledge of frequent itemset properties. Apriori uses a “bottom-up” approach, where it generates the candidate itemsets. To reduce the search space, Apriori uses the property “all subsets of a frequent itemset must be frequent; and if an itemset is infrequent, then all its supersets must also be infrequent”. Another approach predictive Apriori [ 108 ] can also generate rules; however, it receives unexpected results as it combines both the support and confidence. The Apriori [ 8 ] is the widely applicable techniques in mining association rules.
ECLAT: This technique was proposed by Zaki et al. [ 131 ] and stands for Equivalence Class Clustering and bottom-up Lattice Traversal. ECLAT uses a depth-first search to find frequent itemsets. In contrast to the Apriori [ 8 ] algorithm, which represents data in a horizontal pattern, it represents data vertically. Hence, the ECLAT algorithm is more efficient and scalable in the area of association rule learning. This algorithm is better suited for small and medium datasets whereas the Apriori algorithm is used for large datasets.
FP-Growth: Another common association rule learning technique based on the frequent-pattern tree (FP-tree) proposed by Han et al. [ 42 ] is Frequent Pattern Growth, known as FP-Growth. The key difference with Apriori is that while generating rules, the Apriori algorithm [ 8 ] generates frequent candidate itemsets; on the other hand, the FP-growth algorithm [ 42 ] prevents candidate generation and thus produces a tree by the successful strategy of ‘divide and conquer’ approach. Due to its sophistication, however, FP-Tree is challenging to use in an interactive mining environment [ 133 ]. Thus, the FP-Tree would not fit into memory for massive data sets, making it challenging to process big data as well. Another solution is RARM (Rapid Association Rule Mining) proposed by Das et al. [ 26 ] but faces a related FP-tree issue [ 133 ].
ABC-RuleMiner: A rule-based machine learning method, recently proposed in our earlier paper, by Sarker et al. [ 104 ], to discover the interesting non-redundant rules to provide real-world intelligent services. This algorithm effectively identifies the redundancy in associations by taking into account the impact or precedence of the related contextual features and discovers a set of non-redundant association rules. This algorithm first constructs an association generation tree (AGT), a top-down approach, and then extracts the association rules through traversing the tree. Thus, ABC-RuleMiner is more potent than traditional rule-based methods in terms of both non-redundant rule generation and intelligent decision-making, particularly in a context-aware smart computing environment, where human or user preferences are involved.
Among the association rule learning techniques discussed above, Apriori [ 8 ] is the most widely used algorithm for discovering association rules from a given dataset [ 133 ]. The main strength of the association learning technique is its comprehensiveness, as it generates all associations that satisfy the user-specified constraints, such as minimum support and confidence value. The ABC-RuleMiner approach [ 104 ] discussed earlier could give significant results in terms of non-redundant rule generation and intelligent decision-making for the relevant application areas in the real world.
Reinforcement Learning
Reinforcement learning (RL) is a machine learning technique that allows an agent to learn by trial and error in an interactive environment using input from its actions and experiences. Unlike supervised learning, which is based on given sample data or examples, the RL method is based on interacting with the environment. The problem to be solved in reinforcement learning (RL) is defined as a Markov Decision Process (MDP) [ 86 ], i.e., all about sequentially making decisions. An RL problem typically includes four elements such as Agent, Environment, Rewards, and Policy.
RL can be split roughly into Model-based and Model-free techniques. Model-based RL is the process of inferring optimal behavior from a model of the environment by performing actions and observing the results, which include the next state and the immediate reward [ 85 ]. AlphaZero, AlphaGo [ 113 ] are examples of the model-based approaches. On the other hand, a model-free approach does not use the distribution of the transition probability and the reward function associated with MDP. Q-learning, Deep Q Network, Monte Carlo Control, SARSA (State–Action–Reward–State–Action), etc. are some examples of model-free algorithms [ 52 ]. The policy network, which is required for model-based RL but not for model-free, is the key difference between model-free and model-based learning. In the following, we discuss the popular RL algorithms.
Monte Carlo methods: Monte Carlo techniques, or Monte Carlo experiments, are a wide category of computational algorithms that rely on repeated random sampling to obtain numerical results [ 52 ]. The underlying concept is to use randomness to solve problems that are deterministic in principle. Optimization, numerical integration, and making drawings from the probability distribution are the three problem classes where Monte Carlo techniques are most commonly used.
Q-learning: Q-learning is a model-free reinforcement learning algorithm for learning the quality of behaviors that tell an agent what action to take under what conditions [ 52 ]. It does not need a model of the environment (hence the term “model-free”), and it can deal with stochastic transitions and rewards without the need for adaptations. The ‘Q’ in Q-learning usually stands for quality, as the algorithm calculates the maximum expected rewards for a given behavior in a given state.
Deep Q-learning: The basic working step in Deep Q-Learning [ 52 ] is that the initial state is fed into the neural network, which returns the Q-value of all possible actions as an output. Still, when we have a reasonably simple setting to overcome, Q-learning works well. However, when the number of states and actions becomes more complicated, deep learning can be used as a function approximator.
Reinforcement learning, along with supervised and unsupervised learning, is one of the basic machine learning paradigms. RL can be used to solve numerous real-world problems in various fields, such as game theory, control theory, operations analysis, information theory, simulation-based optimization, manufacturing, supply chain logistics, multi-agent systems, swarm intelligence, aircraft control, robot motion control, and many more.
Artificial Neural Network and Deep Learning
Deep learning is part of a wider family of artificial neural networks (ANN)-based machine learning approaches with representation learning. Deep learning provides a computational architecture by combining several processing layers, such as input, hidden, and output layers, to learn from data [ 41 ]. The main advantage of deep learning over traditional machine learning methods is its better performance in several cases, particularly learning from large datasets [ 105 , 129 ]. Figure 9 shows a general performance of deep learning over machine learning considering the increasing amount of data. However, it may vary depending on the data characteristics and experimental set up.
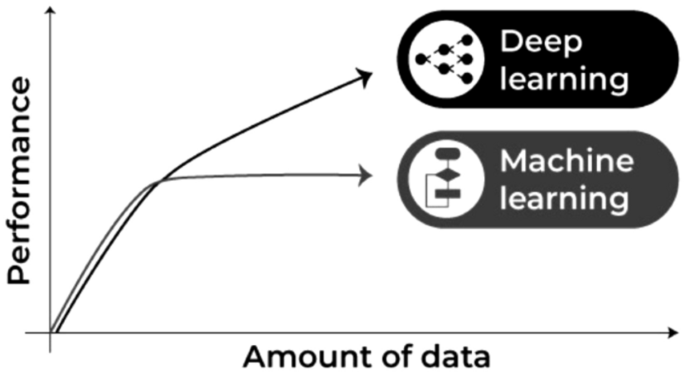
Machine learning and deep learning performance in general with the amount of data
The most common deep learning algorithms are: Multi-layer Perceptron (MLP), Convolutional Neural Network (CNN, or ConvNet), Long Short-Term Memory Recurrent Neural Network (LSTM-RNN) [ 96 ]. In the following, we discuss various types of deep learning methods that can be used to build effective data-driven models for various purposes.
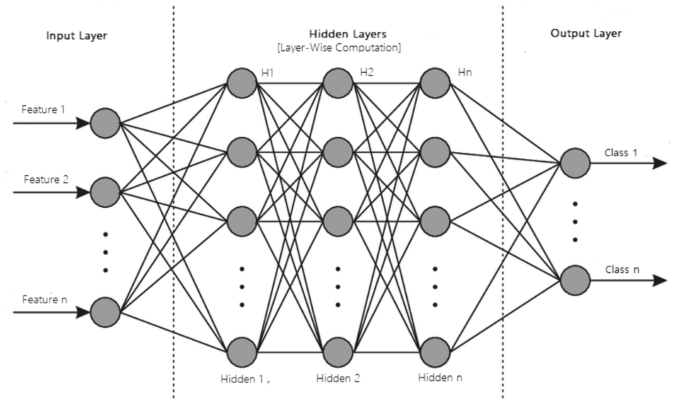
A structure of an artificial neural network modeling with multiple processing layers
MLP: The base architecture of deep learning, which is also known as the feed-forward artificial neural network, is called a multilayer perceptron (MLP) [ 82 ]. A typical MLP is a fully connected network consisting of an input layer, one or more hidden layers, and an output layer, as shown in Fig. 10 . Each node in one layer connects to each node in the following layer at a certain weight. MLP utilizes the “Backpropagation” technique [ 41 ], the most “fundamental building block” in a neural network, to adjust the weight values internally while building the model. MLP is sensitive to scaling features and allows a variety of hyperparameters to be tuned, such as the number of hidden layers, neurons, and iterations, which can result in a computationally costly model.
CNN or ConvNet: The convolution neural network (CNN) [ 65 ] enhances the design of the standard ANN, consisting of convolutional layers, pooling layers, as well as fully connected layers, as shown in Fig. 11 . As it takes the advantage of the two-dimensional (2D) structure of the input data, it is typically broadly used in several areas such as image and video recognition, image processing and classification, medical image analysis, natural language processing, etc. While CNN has a greater computational burden, without any manual intervention, it has the advantage of automatically detecting the important features, and hence CNN is considered to be more powerful than conventional ANN. A number of advanced deep learning models based on CNN can be used in the field, such as AlexNet [ 60 ], Xception [ 24 ], Inception [ 118 ], Visual Geometry Group (VGG) [ 44 ], ResNet [ 45 ], etc.
LSTM-RNN: Long short-term memory (LSTM) is an artificial recurrent neural network (RNN) architecture used in the area of deep learning [ 38 ]. LSTM has feedback links, unlike normal feed-forward neural networks. LSTM networks are well-suited for analyzing and learning sequential data, such as classifying, processing, and predicting data based on time series data, which differentiates it from other conventional networks. Thus, LSTM can be used when the data are in a sequential format, such as time, sentence, etc., and commonly applied in the area of time-series analysis, natural language processing, speech recognition, etc.
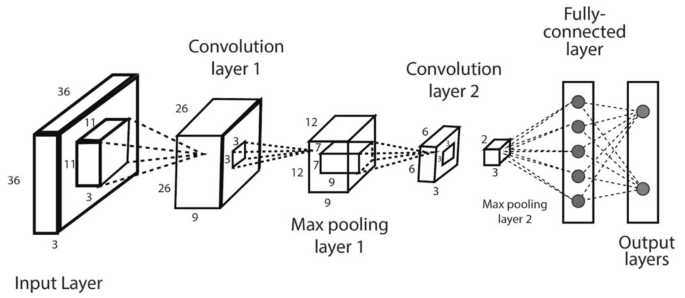
An example of a convolutional neural network (CNN or ConvNet) including multiple convolution and pooling layers
In addition to these most common deep learning methods discussed above, several other deep learning approaches [ 96 ] exist in the area for various purposes. For instance, the self-organizing map (SOM) [ 58 ] uses unsupervised learning to represent the high-dimensional data by a 2D grid map, thus achieving dimensionality reduction. The autoencoder (AE) [ 15 ] is another learning technique that is widely used for dimensionality reduction as well and feature extraction in unsupervised learning tasks. Restricted Boltzmann machines (RBM) [ 46 ] can be used for dimensionality reduction, classification, regression, collaborative filtering, feature learning, and topic modeling. A deep belief network (DBN) is typically composed of simple, unsupervised networks such as restricted Boltzmann machines (RBMs) or autoencoders, and a backpropagation neural network (BPNN) [ 123 ]. A generative adversarial network (GAN) [ 39 ] is a form of the network for deep learning that can generate data with characteristics close to the actual data input. Transfer learning is currently very common because it can train deep neural networks with comparatively low data, which is typically the re-use of a new problem with a pre-trained model [ 124 ]. A brief discussion of these artificial neural networks (ANN) and deep learning (DL) models are summarized in our earlier paper Sarker et al. [ 96 ].
Overall, based on the learning techniques discussed above, we can conclude that various types of machine learning techniques, such as classification analysis, regression, data clustering, feature selection and extraction, and dimensionality reduction, association rule learning, reinforcement learning, or deep learning techniques, can play a significant role for various purposes according to their capabilities. In the following section, we discuss several application areas based on machine learning algorithms.
Applications of Machine Learning
In the current age of the Fourth Industrial Revolution (4IR), machine learning becomes popular in various application areas, because of its learning capabilities from the past and making intelligent decisions. In the following, we summarize and discuss ten popular application areas of machine learning technology.
Predictive analytics and intelligent decision-making: A major application field of machine learning is intelligent decision-making by data-driven predictive analytics [ 21 , 70 ]. The basis of predictive analytics is capturing and exploiting relationships between explanatory variables and predicted variables from previous events to predict the unknown outcome [ 41 ]. For instance, identifying suspects or criminals after a crime has been committed, or detecting credit card fraud as it happens. Another application, where machine learning algorithms can assist retailers in better understanding consumer preferences and behavior, better manage inventory, avoiding out-of-stock situations, and optimizing logistics and warehousing in e-commerce. Various machine learning algorithms such as decision trees, support vector machines, artificial neural networks, etc. [ 106 , 125 ] are commonly used in the area. Since accurate predictions provide insight into the unknown, they can improve the decisions of industries, businesses, and almost any organization, including government agencies, e-commerce, telecommunications, banking and financial services, healthcare, sales and marketing, transportation, social networking, and many others.
Cybersecurity and threat intelligence: Cybersecurity is one of the most essential areas of Industry 4.0. [ 114 ], which is typically the practice of protecting networks, systems, hardware, and data from digital attacks [ 114 ]. Machine learning has become a crucial cybersecurity technology that constantly learns by analyzing data to identify patterns, better detect malware in encrypted traffic, find insider threats, predict where bad neighborhoods are online, keep people safe while browsing, or secure data in the cloud by uncovering suspicious activity. For instance, clustering techniques can be used to identify cyber-anomalies, policy violations, etc. To detect various types of cyber-attacks or intrusions machine learning classification models by taking into account the impact of security features are useful [ 97 ]. Various deep learning-based security models can also be used on the large scale of security datasets [ 96 , 129 ]. Moreover, security policy rules generated by association rule learning techniques can play a significant role to build a rule-based security system [ 105 ]. Thus, we can say that various learning techniques discussed in Sect. Machine Learning Tasks and Algorithms , can enable cybersecurity professionals to be more proactive inefficiently preventing threats and cyber-attacks.
Internet of things (IoT) and smart cities: Internet of Things (IoT) is another essential area of Industry 4.0. [ 114 ], which turns everyday objects into smart objects by allowing them to transmit data and automate tasks without the need for human interaction. IoT is, therefore, considered to be the big frontier that can enhance almost all activities in our lives, such as smart governance, smart home, education, communication, transportation, retail, agriculture, health care, business, and many more [ 70 ]. Smart city is one of IoT’s core fields of application, using technologies to enhance city services and residents’ living experiences [ 132 , 135 ]. As machine learning utilizes experience to recognize trends and create models that help predict future behavior and events, it has become a crucial technology for IoT applications [ 103 ]. For example, to predict traffic in smart cities, parking availability prediction, estimate the total usage of energy of the citizens for a particular period, make context-aware and timely decisions for the people, etc. are some tasks that can be solved using machine learning techniques according to the current needs of the people.
Traffic prediction and transportation: Transportation systems have become a crucial component of every country’s economic development. Nonetheless, several cities around the world are experiencing an excessive rise in traffic volume, resulting in serious issues such as delays, traffic congestion, higher fuel prices, increased CO \(_2\) pollution, accidents, emergencies, and a decline in modern society’s quality of life [ 40 ]. Thus, an intelligent transportation system through predicting future traffic is important, which is an indispensable part of a smart city. Accurate traffic prediction based on machine and deep learning modeling can help to minimize the issues [ 17 , 30 , 31 ]. For example, based on the travel history and trend of traveling through various routes, machine learning can assist transportation companies in predicting possible issues that may occur on specific routes and recommending their customers to take a different path. Ultimately, these learning-based data-driven models help improve traffic flow, increase the usage and efficiency of sustainable modes of transportation, and limit real-world disruption by modeling and visualizing future changes.
Healthcare and COVID-19 pandemic: Machine learning can help to solve diagnostic and prognostic problems in a variety of medical domains, such as disease prediction, medical knowledge extraction, detecting regularities in data, patient management, etc. [ 33 , 77 , 112 ]. Coronavirus disease (COVID-19) is an infectious disease caused by a newly discovered coronavirus, according to the World Health Organization (WHO) [ 3 ]. Recently, the learning techniques have become popular in the battle against COVID-19 [ 61 , 63 ]. For the COVID-19 pandemic, the learning techniques are used to classify patients at high risk, their mortality rate, and other anomalies [ 61 ]. It can also be used to better understand the virus’s origin, COVID-19 outbreak prediction, as well as for disease diagnosis and treatment [ 14 , 50 ]. With the help of machine learning, researchers can forecast where and when, the COVID-19 is likely to spread, and notify those regions to match the required arrangements. Deep learning also provides exciting solutions to the problems of medical image processing and is seen as a crucial technique for potential applications, particularly for COVID-19 pandemic [ 10 , 78 , 111 ]. Overall, machine and deep learning techniques can help to fight the COVID-19 virus and the pandemic as well as intelligent clinical decisions making in the domain of healthcare.
E-commerce and product recommendations: Product recommendation is one of the most well known and widely used applications of machine learning, and it is one of the most prominent features of almost any e-commerce website today. Machine learning technology can assist businesses in analyzing their consumers’ purchasing histories and making customized product suggestions for their next purchase based on their behavior and preferences. E-commerce companies, for example, can easily position product suggestions and offers by analyzing browsing trends and click-through rates of specific items. Using predictive modeling based on machine learning techniques, many online retailers, such as Amazon [ 71 ], can better manage inventory, prevent out-of-stock situations, and optimize logistics and warehousing. The future of sales and marketing is the ability to capture, evaluate, and use consumer data to provide a customized shopping experience. Furthermore, machine learning techniques enable companies to create packages and content that are tailored to the needs of their customers, allowing them to maintain existing customers while attracting new ones.
NLP and sentiment analysis: Natural language processing (NLP) involves the reading and understanding of spoken or written language through the medium of a computer [ 79 , 103 ]. Thus, NLP helps computers, for instance, to read a text, hear speech, interpret it, analyze sentiment, and decide which aspects are significant, where machine learning techniques can be used. Virtual personal assistant, chatbot, speech recognition, document description, language or machine translation, etc. are some examples of NLP-related tasks. Sentiment Analysis [ 90 ] (also referred to as opinion mining or emotion AI) is an NLP sub-field that seeks to identify and extract public mood and views within a given text through blogs, reviews, social media, forums, news, etc. For instance, businesses and brands use sentiment analysis to understand the social sentiment of their brand, product, or service through social media platforms or the web as a whole. Overall, sentiment analysis is considered as a machine learning task that analyzes texts for polarity, such as “positive”, “negative”, or “neutral” along with more intense emotions like very happy, happy, sad, very sad, angry, have interest, or not interested etc.
Image, speech and pattern recognition: Image recognition [ 36 ] is a well-known and widespread example of machine learning in the real world, which can identify an object as a digital image. For instance, to label an x-ray as cancerous or not, character recognition, or face detection in an image, tagging suggestions on social media, e.g., Facebook, are common examples of image recognition. Speech recognition [ 23 ] is also very popular that typically uses sound and linguistic models, e.g., Google Assistant, Cortana, Siri, Alexa, etc. [ 67 ], where machine learning methods are used. Pattern recognition [ 13 ] is defined as the automated recognition of patterns and regularities in data, e.g., image analysis. Several machine learning techniques such as classification, feature selection, clustering, or sequence labeling methods are used in the area.
Sustainable agriculture: Agriculture is essential to the survival of all human activities [ 109 ]. Sustainable agriculture practices help to improve agricultural productivity while also reducing negative impacts on the environment [ 5 , 25 , 109 ]. The sustainable agriculture supply chains are knowledge-intensive and based on information, skills, technologies, etc., where knowledge transfer encourages farmers to enhance their decisions to adopt sustainable agriculture practices utilizing the increasing amount of data captured by emerging technologies, e.g., the Internet of Things (IoT), mobile technologies and devices, etc. [ 5 , 53 , 54 ]. Machine learning can be applied in various phases of sustainable agriculture, such as in the pre-production phase - for the prediction of crop yield, soil properties, irrigation requirements, etc.; in the production phase—for weather prediction, disease detection, weed detection, soil nutrient management, livestock management, etc.; in processing phase—for demand estimation, production planning, etc. and in the distribution phase - the inventory management, consumer analysis, etc.
User behavior analytics and context-aware smartphone applications: Context-awareness is a system’s ability to capture knowledge about its surroundings at any moment and modify behaviors accordingly [ 28 , 93 ]. Context-aware computing uses software and hardware to automatically collect and interpret data for direct responses. The mobile app development environment has been changed greatly with the power of AI, particularly, machine learning techniques through their learning capabilities from contextual data [ 103 , 136 ]. Thus, the developers of mobile apps can rely on machine learning to create smart apps that can understand human behavior, support, and entertain users [ 107 , 137 , 140 ]. To build various personalized data-driven context-aware systems, such as smart interruption management, smart mobile recommendation, context-aware smart searching, decision-making that intelligently assist end mobile phone users in a pervasive computing environment, machine learning techniques are applicable. For example, context-aware association rules can be used to build an intelligent phone call application [ 104 ]. Clustering approaches are useful in capturing users’ diverse behavioral activities by taking into account data in time series [ 102 ]. To predict the future events in various contexts, the classification methods can be used [ 106 , 139 ]. Thus, various learning techniques discussed in Sect. “ Machine Learning Tasks and Algorithms ” can help to build context-aware adaptive and smart applications according to the preferences of the mobile phone users.
In addition to these application areas, machine learning-based models can also apply to several other domains such as bioinformatics, cheminformatics, computer networks, DNA sequence classification, economics and banking, robotics, advanced engineering, and many more.
Challenges and Research Directions
Our study on machine learning algorithms for intelligent data analysis and applications opens several research issues in the area. Thus, in this section, we summarize and discuss the challenges faced and the potential research opportunities and future directions.
In general, the effectiveness and the efficiency of a machine learning-based solution depend on the nature and characteristics of the data, and the performance of the learning algorithms. To collect the data in the relevant domain, such as cybersecurity, IoT, healthcare and agriculture discussed in Sect. “ Applications of Machine Learning ” is not straightforward, although the current cyberspace enables the production of a huge amount of data with very high frequency. Thus, collecting useful data for the target machine learning-based applications, e.g., smart city applications, and their management is important to further analysis. Therefore, a more in-depth investigation of data collection methods is needed while working on the real-world data. Moreover, the historical data may contain many ambiguous values, missing values, outliers, and meaningless data. The machine learning algorithms, discussed in Sect “ Machine Learning Tasks and Algorithms ” highly impact on data quality, and availability for training, and consequently on the resultant model. Thus, to accurately clean and pre-process the diverse data collected from diverse sources is a challenging task. Therefore, effectively modifying or enhance existing pre-processing methods, or proposing new data preparation techniques are required to effectively use the learning algorithms in the associated application domain.
To analyze the data and extract insights, there exist many machine learning algorithms, summarized in Sect. “ Machine Learning Tasks and Algorithms ”. Thus, selecting a proper learning algorithm that is suitable for the target application is challenging. The reason is that the outcome of different learning algorithms may vary depending on the data characteristics [ 106 ]. Selecting a wrong learning algorithm would result in producing unexpected outcomes that may lead to loss of effort, as well as the model’s effectiveness and accuracy. In terms of model building, the techniques discussed in Sect. “ Machine Learning Tasks and Algorithms ” can directly be used to solve many real-world issues in diverse domains, such as cybersecurity, smart cities and healthcare summarized in Sect. “ Applications of Machine Learning ”. However, the hybrid learning model, e.g., the ensemble of methods, modifying or enhancement of the existing learning techniques, or designing new learning methods, could be a potential future work in the area.
Thus, the ultimate success of a machine learning-based solution and corresponding applications mainly depends on both the data and the learning algorithms. If the data are bad to learn, such as non-representative, poor-quality, irrelevant features, or insufficient quantity for training, then the machine learning models may become useless or will produce lower accuracy. Therefore, effectively processing the data and handling the diverse learning algorithms are important, for a machine learning-based solution and eventually building intelligent applications.
In this paper, we have conducted a comprehensive overview of machine learning algorithms for intelligent data analysis and applications. According to our goal, we have briefly discussed how various types of machine learning methods can be used for making solutions to various real-world issues. A successful machine learning model depends on both the data and the performance of the learning algorithms. The sophisticated learning algorithms then need to be trained through the collected real-world data and knowledge related to the target application before the system can assist with intelligent decision-making. We also discussed several popular application areas based on machine learning techniques to highlight their applicability in various real-world issues. Finally, we have summarized and discussed the challenges faced and the potential research opportunities and future directions in the area. Therefore, the challenges that are identified create promising research opportunities in the field which must be addressed with effective solutions in various application areas. Overall, we believe that our study on machine learning-based solutions opens up a promising direction and can be used as a reference guide for potential research and applications for both academia and industry professionals as well as for decision-makers, from a technical point of view.
Canadian institute of cybersecurity, university of new brunswick, iscx dataset, http://www.unb.ca/cic/datasets/index.html/ (Accessed on 20 October 2019).
Cic-ddos2019 [online]. available: https://www.unb.ca/cic/datasets/ddos-2019.html/ (Accessed on 28 March 2020).
World health organization: WHO. http://www.who.int/ .
Google trends. In https://trends.google.com/trends/ , 2019.
Adnan N, Nordin Shahrina Md, Rahman I, Noor A. The effects of knowledge transfer on farmers decision making toward sustainable agriculture practices. World J Sci Technol Sustain Dev. 2018.
Agrawal R, Gehrke J, Gunopulos D, Raghavan P. Automatic subspace clustering of high dimensional data for data mining applications. In: Proceedings of the 1998 ACM SIGMOD international conference on Management of data. 1998; 94–105
Agrawal R, Imieliński T, Swami A. Mining association rules between sets of items in large databases. In: ACM SIGMOD Record. ACM. 1993;22: 207–216
Agrawal R, Gehrke J, Gunopulos D, Raghavan P. Fast algorithms for mining association rules. In: Proceedings of the International Joint Conference on Very Large Data Bases, Santiago Chile. 1994; 1215: 487–499.
Aha DW, Kibler D, Albert M. Instance-based learning algorithms. Mach Learn. 1991;6(1):37–66.
Article Google Scholar
Alakus TB, Turkoglu I. Comparison of deep learning approaches to predict covid-19 infection. Chaos Solit Fract. 2020;140:
Amit Y, Geman D. Shape quantization and recognition with randomized trees. Neural Comput. 1997;9(7):1545–88.
Ankerst M, Breunig MM, Kriegel H-P, Sander J. Optics: ordering points to identify the clustering structure. ACM Sigmod Record. 1999;28(2):49–60.
Anzai Y. Pattern recognition and machine learning. Elsevier; 2012.
MATH Google Scholar
Ardabili SF, Mosavi A, Ghamisi P, Ferdinand F, Varkonyi-Koczy AR, Reuter U, Rabczuk T, Atkinson PM. Covid-19 outbreak prediction with machine learning. Algorithms. 2020;13(10):249.
Article MathSciNet Google Scholar
Baldi P. Autoencoders, unsupervised learning, and deep architectures. In: Proceedings of ICML workshop on unsupervised and transfer learning, 2012; 37–49 .
Balducci F, Impedovo D, Pirlo G. Machine learning applications on agricultural datasets for smart farm enhancement. Machines. 2018;6(3):38.
Boukerche A, Wang J. Machine learning-based traffic prediction models for intelligent transportation systems. Comput Netw. 2020;181
Breiman L. Bagging predictors. Mach Learn. 1996;24(2):123–40.
Article MATH Google Scholar
Breiman L. Random forests. Mach Learn. 2001;45(1):5–32.
Breiman L, Friedman J, Stone CJ, Olshen RA. Classification and regression trees. CRC Press; 1984.
Cao L. Data science: a comprehensive overview. ACM Comput Surv (CSUR). 2017;50(3):43.
Google Scholar
Carpenter GA, Grossberg S. A massively parallel architecture for a self-organizing neural pattern recognition machine. Comput Vis Graph Image Process. 1987;37(1):54–115.
Chiu C-C, Sainath TN, Wu Y, Prabhavalkar R, Nguyen P, Chen Z, Kannan A, Weiss RJ, Rao K, Gonina E, et al. State-of-the-art speech recognition with sequence-to-sequence models. In: 2018 IEEE International Conference on Acoustics, Speech and Signal Processing (ICASSP), 2018 pages 4774–4778. IEEE .
Chollet F. Xception: deep learning with depthwise separable convolutions. In: Proceedings of the IEEE conference on computer vision and pattern recognition, pages 1251–1258, 2017.
Cobuloglu H, Büyüktahtakın IE. A stochastic multi-criteria decision analysis for sustainable biomass crop selection. Expert Syst Appl. 2015;42(15–16):6065–74.
Das A, Ng W-K, Woon Y-K. Rapid association rule mining. In: Proceedings of the tenth international conference on Information and knowledge management, pages 474–481. ACM, 2001.
de Amorim RC. Constrained clustering with minkowski weighted k-means. In: 2012 IEEE 13th International Symposium on Computational Intelligence and Informatics (CINTI), pages 13–17. IEEE, 2012.
Dey AK. Understanding and using context. Person Ubiquit Comput. 2001;5(1):4–7.
Eagle N, Pentland AS. Reality mining: sensing complex social systems. Person Ubiquit Comput. 2006;10(4):255–68.
Essien A, Petrounias I, Sampaio P, Sampaio S. Improving urban traffic speed prediction using data source fusion and deep learning. In: 2019 IEEE International Conference on Big Data and Smart Computing (BigComp). IEEE. 2019: 1–8. .
Essien A, Petrounias I, Sampaio P, Sampaio S. A deep-learning model for urban traffic flow prediction with traffic events mined from twitter. In: World Wide Web, 2020: 1–24 .
Ester M, Kriegel H-P, Sander J, Xiaowei X, et al. A density-based algorithm for discovering clusters in large spatial databases with noise. Kdd. 1996;96:226–31.
Fatima M, Pasha M, et al. Survey of machine learning algorithms for disease diagnostic. J Intell Learn Syst Appl. 2017;9(01):1.
Flach PA, Lachiche N. Confirmation-guided discovery of first-order rules with tertius. Mach Learn. 2001;42(1–2):61–95.
Freund Y, Schapire RE, et al. Experiments with a new boosting algorithm. In: Icml, Citeseer. 1996; 96: 148–156
Fujiyoshi H, Hirakawa T, Yamashita T. Deep learning-based image recognition for autonomous driving. IATSS Res. 2019;43(4):244–52.
Fukunaga K, Hostetler L. The estimation of the gradient of a density function, with applications in pattern recognition. IEEE Trans Inform Theory. 1975;21(1):32–40.
Article MathSciNet MATH Google Scholar
Goodfellow I, Bengio Y, Courville A, Bengio Y. Deep learning. Cambridge: MIT Press; 2016.
Goodfellow I, Pouget-Abadie J, Mirza M, Xu B, Warde-Farley D, Ozair S, Courville A, Bengio Y. Generative adversarial nets. In: Advances in neural information processing systems. 2014: 2672–2680.
Guerrero-Ibáñez J, Zeadally S, Contreras-Castillo J. Sensor technologies for intelligent transportation systems. Sensors. 2018;18(4):1212.
Han J, Pei J, Kamber M. Data mining: concepts and techniques. Amsterdam: Elsevier; 2011.
Han J, Pei J, Yin Y. Mining frequent patterns without candidate generation. In: ACM Sigmod Record, ACM. 2000;29: 1–12.
Harmon SA, Sanford TH, Sheng X, Turkbey EB, Roth H, Ziyue X, Yang D, Myronenko A, Anderson V, Amalou A, et al. Artificial intelligence for the detection of covid-19 pneumonia on chest ct using multinational datasets. Nat Commun. 2020;11(1):1–7.
He K, Zhang X, Ren S, Sun J. Spatial pyramid pooling in deep convolutional networks for visual recognition. IEEE Trans Pattern Anal Mach Intell. 2015;37(9):1904–16.
He K, Zhang X, Ren S, Sun J. Deep residual learning for image recognition. In: Proceedings of the IEEE conference on computer vision and pattern recognition, 2016: 770–778.
Hinton GE. A practical guide to training restricted boltzmann machines. In: Neural networks: Tricks of the trade. Springer. 2012; 599-619
Holte RC. Very simple classification rules perform well on most commonly used datasets. Mach Learn. 1993;11(1):63–90.
Hotelling H. Analysis of a complex of statistical variables into principal components. J Edu Psychol. 1933;24(6):417.
Houtsma M, Swami A. Set-oriented mining for association rules in relational databases. In: Data Engineering, 1995. Proceedings of the Eleventh International Conference on, IEEE.1995:25–33.
Jamshidi M, Lalbakhsh A, Talla J, Peroutka Z, Hadjilooei F, Lalbakhsh P, Jamshidi M, La Spada L, Mirmozafari M, Dehghani M, et al. Artificial intelligence and covid-19: deep learning approaches for diagnosis and treatment. IEEE Access. 2020;8:109581–95.
John GH, Langley P. Estimating continuous distributions in bayesian classifiers. In: Proceedings of the Eleventh conference on Uncertainty in artificial intelligence, Morgan Kaufmann Publishers Inc. 1995; 338–345
Kaelbling LP, Littman ML, Moore AW. Reinforcement learning: a survey. J Artif Intell Res. 1996;4:237–85.
Kamble SS, Gunasekaran A, Gawankar SA. Sustainable industry 4.0 framework: a systematic literature review identifying the current trends and future perspectives. Process Saf Environ Protect. 2018;117:408–25.
Kamble SS, Gunasekaran A, Gawankar SA. Achieving sustainable performance in a data-driven agriculture supply chain: a review for research and applications. Int J Prod Econ. 2020;219:179–94.
Kaufman L, Rousseeuw PJ. Finding groups in data: an introduction to cluster analysis, vol. 344. John Wiley & Sons; 2009.
Keerthi SS, Shevade SK, Bhattacharyya C, Radha Krishna MK. Improvements to platt’s smo algorithm for svm classifier design. Neural Comput. 2001;13(3):637–49.
Khadse V, Mahalle PN, Biraris SV. An empirical comparison of supervised machine learning algorithms for internet of things data. In: 2018 Fourth International Conference on Computing Communication Control and Automation (ICCUBEA), IEEE. 2018; 1–6
Kohonen T. The self-organizing map. Proc IEEE. 1990;78(9):1464–80.
Koroniotis N, Moustafa N, Sitnikova E, Turnbull B. Towards the development of realistic botnet dataset in the internet of things for network forensic analytics: bot-iot dataset. Fut Gen Comput Syst. 2019;100:779–96.
Krizhevsky A, Sutskever I, Hinton GE. Imagenet classification with deep convolutional neural networks. In: Advances in neural information processing systems, 2012: 1097–1105
Kushwaha S, Bahl S, Bagha AK, Parmar KS, Javaid M, Haleem A, Singh RP. Significant applications of machine learning for covid-19 pandemic. J Ind Integr Manag. 2020;5(4).
Lade P, Ghosh R, Srinivasan S. Manufacturing analytics and industrial internet of things. IEEE Intell Syst. 2017;32(3):74–9.
Lalmuanawma S, Hussain J, Chhakchhuak L. Applications of machine learning and artificial intelligence for covid-19 (sars-cov-2) pandemic: a review. Chaos Sol Fract. 2020:110059 .
LeCessie S, Van Houwelingen JC. Ridge estimators in logistic regression. J R Stat Soc Ser C (Appl Stat). 1992;41(1):191–201.
LeCun Y, Bottou L, Bengio Y, Haffner P. Gradient-based learning applied to document recognition. Proc IEEE. 1998;86(11):2278–324.
Liu H, Motoda H. Feature extraction, construction and selection: A data mining perspective, vol. 453. Springer Science & Business Media; 1998.
López G, Quesada L, Guerrero LA. Alexa vs. siri vs. cortana vs. google assistant: a comparison of speech-based natural user interfaces. In: International Conference on Applied Human Factors and Ergonomics, Springer. 2017; 241–250.
Liu B, HsuW, Ma Y. Integrating classification and association rule mining. In: Proceedings of the fourth international conference on knowledge discovery and data mining, 1998.
MacQueen J, et al. Some methods for classification and analysis of multivariate observations. In: Proceedings of the fifth Berkeley symposium on mathematical statistics and probability, 1967;volume 1, pages 281–297. Oakland, CA, USA.
Mahdavinejad MS, Rezvan M, Barekatain M, Adibi P, Barnaghi P, Sheth AP. Machine learning for internet of things data analysis: a survey. Digit Commun Netw. 2018;4(3):161–75.
Marchand A, Marx P. Automated product recommendations with preference-based explanations. J Retail. 2020;96(3):328–43.
McCallum A. Information extraction: distilling structured data from unstructured text. Queue. 2005;3(9):48–57.
Mehrotra A, Hendley R, Musolesi M. Prefminer: mining user’s preferences for intelligent mobile notification management. In: Proceedings of the International Joint Conference on Pervasive and Ubiquitous Computing, Heidelberg, Germany, 12–16 September, 2016; pp. 1223–1234. ACM, New York, USA. .
Mohamadou Y, Halidou A, Kapen PT. A review of mathematical modeling, artificial intelligence and datasets used in the study, prediction and management of covid-19. Appl Intell. 2020;50(11):3913–25.
Mohammed M, Khan MB, Bashier Mohammed BE. Machine learning: algorithms and applications. CRC Press; 2016.
Book Google Scholar
Moustafa N, Slay J. Unsw-nb15: a comprehensive data set for network intrusion detection systems (unsw-nb15 network data set). In: 2015 military communications and information systems conference (MilCIS), 2015;pages 1–6. IEEE .
Nilashi M, Ibrahim OB, Ahmadi H, Shahmoradi L. An analytical method for diseases prediction using machine learning techniques. Comput Chem Eng. 2017;106:212–23.
Yujin O, Park S, Ye JC. Deep learning covid-19 features on cxr using limited training data sets. IEEE Trans Med Imaging. 2020;39(8):2688–700.
Otter DW, Medina JR , Kalita JK. A survey of the usages of deep learning for natural language processing. IEEE Trans Neural Netw Learn Syst. 2020.
Park H-S, Jun C-H. A simple and fast algorithm for k-medoids clustering. Expert Syst Appl. 2009;36(2):3336–41.
Liii Pearson K. on lines and planes of closest fit to systems of points in space. Lond Edinb Dublin Philos Mag J Sci. 1901;2(11):559–72.
Pedregosa F, Varoquaux G, Gramfort A, Michel V, Thirion B, Grisel O, Blondel M, Prettenhofer P, Weiss R, Dubourg V, et al. Scikit-learn: machine learning in python. J Mach Learn Res. 2011;12:2825–30.
MathSciNet MATH Google Scholar
Perveen S, Shahbaz M, Keshavjee K, Guergachi A. Metabolic syndrome and development of diabetes mellitus: predictive modeling based on machine learning techniques. IEEE Access. 2018;7:1365–75.
Santi P, Ram D, Rob C, Nathan E. Behavior-based adaptive call predictor. ACM Trans Auton Adapt Syst. 2011;6(3):21:1–21:28.
Polydoros AS, Nalpantidis L. Survey of model-based reinforcement learning: applications on robotics. J Intell Robot Syst. 2017;86(2):153–73.
Puterman ML. Markov decision processes: discrete stochastic dynamic programming. John Wiley & Sons; 2014.
Quinlan JR. Induction of decision trees. Mach Learn. 1986;1:81–106.
Quinlan JR. C4.5: programs for machine learning. Mach Learn. 1993.
Rasmussen C. The infinite gaussian mixture model. Adv Neural Inform Process Syst. 1999;12:554–60.
Ravi K, Ravi V. A survey on opinion mining and sentiment analysis: tasks, approaches and applications. Knowl Syst. 2015;89:14–46.
Rokach L. A survey of clustering algorithms. In: Data mining and knowledge discovery handbook, pages 269–298. Springer, 2010.
Safdar S, Zafar S, Zafar N, Khan NF. Machine learning based decision support systems (dss) for heart disease diagnosis: a review. Artif Intell Rev. 2018;50(4):597–623.
Sarker IH. Context-aware rule learning from smartphone data: survey, challenges and future directions. J Big Data. 2019;6(1):1–25.
Sarker IH. A machine learning based robust prediction model for real-life mobile phone data. Internet Things. 2019;5:180–93.
Sarker IH. Ai-driven cybersecurity: an overview, security intelligence modeling and research directions. SN Comput Sci. 2021.
Sarker IH. Deep cybersecurity: a comprehensive overview from neural network and deep learning perspective. SN Comput Sci. 2021.
Sarker IH, Abushark YB, Alsolami F, Khan A. Intrudtree: a machine learning based cyber security intrusion detection model. Symmetry. 2020;12(5):754.
Sarker IH, Abushark YB, Khan A. Contextpca: predicting context-aware smartphone apps usage based on machine learning techniques. Symmetry. 2020;12(4):499.
Sarker IH, Alqahtani H, Alsolami F, Khan A, Abushark YB, Siddiqui MK. Context pre-modeling: an empirical analysis for classification based user-centric context-aware predictive modeling. J Big Data. 2020;7(1):1–23.
Sarker IH, Alan C, Jun H, Khan AI, Abushark YB, Khaled S. Behavdt: a behavioral decision tree learning to build user-centric context-aware predictive model. Mob Netw Appl. 2019; 1–11.
Sarker IH, Colman A, Kabir MA, Han J. Phone call log as a context source to modeling individual user behavior. In: Proceedings of the 2016 ACM International Joint Conference on Pervasive and Ubiquitous Computing (Ubicomp): Adjunct, Germany, pages 630–634. ACM, 2016.
Sarker IH, Colman A, Kabir MA, Han J. Individualized time-series segmentation for mining mobile phone user behavior. Comput J Oxf Univ UK. 2018;61(3):349–68.
Sarker IH, Hoque MM, MdK Uddin, Tawfeeq A. Mobile data science and intelligent apps: concepts, ai-based modeling and research directions. Mob Netw Appl, pages 1–19, 2020.
Sarker IH, Kayes ASM. Abc-ruleminer: user behavioral rule-based machine learning method for context-aware intelligent services. J Netw Comput Appl. 2020; page 102762
Sarker IH, Kayes ASM, Badsha S, Alqahtani H, Watters P, Ng A. Cybersecurity data science: an overview from machine learning perspective. J Big Data. 2020;7(1):1–29.
Sarker IH, Watters P, Kayes ASM. Effectiveness analysis of machine learning classification models for predicting personalized context-aware smartphone usage. J Big Data. 2019;6(1):1–28.
Sarker IH, Salah K. Appspred: predicting context-aware smartphone apps using random forest learning. Internet Things. 2019;8:
Scheffer T. Finding association rules that trade support optimally against confidence. Intell Data Anal. 2005;9(4):381–95.
Sharma R, Kamble SS, Gunasekaran A, Kumar V, Kumar A. A systematic literature review on machine learning applications for sustainable agriculture supply chain performance. Comput Oper Res. 2020;119:
Shengli S, Ling CX. Hybrid cost-sensitive decision tree, knowledge discovery in databases. In: PKDD 2005, Proceedings of 9th European Conference on Principles and Practice of Knowledge Discovery in Databases. Lecture Notes in Computer Science, volume 3721, 2005.
Shorten C, Khoshgoftaar TM, Furht B. Deep learning applications for covid-19. J Big Data. 2021;8(1):1–54.
Gökhan S, Nevin Y. Data analysis in health and big data: a machine learning medical diagnosis model based on patients’ complaints. Commun Stat Theory Methods. 2019;1–10
Silver D, Huang A, Maddison CJ, Guez A, Sifre L, Van Den Driessche G, Schrittwieser J, Antonoglou I, Panneershelvam V, Lanctot M, et al. Mastering the game of go with deep neural networks and tree search. nature. 2016;529(7587):484–9.
Ślusarczyk B. Industry 4.0: Are we ready? Polish J Manag Stud. 17, 2018.
Sneath Peter HA. The application of computers to taxonomy. J Gen Microbiol. 1957;17(1).
Sorensen T. Method of establishing groups of equal amplitude in plant sociology based on similarity of species. Biol Skr. 1948; 5.
Srinivasan V, Moghaddam S, Mukherji A. Mobileminer: mining your frequent patterns on your phone. In: Proceedings of the International Joint Conference on Pervasive and Ubiquitous Computing, Seattle, WA, USA, 13-17 September, pp. 389–400. ACM, New York, USA. 2014.
Szegedy C, Liu W, Jia Y, Sermanet P, Reed S, Anguelov D, Erhan D, Vanhoucke V, Rabinovich A. Going deeper with convolutions. In: Proceedings of the IEEE conference on computer vision and pattern recognition. 2015; pages 1–9.
Tavallaee M, Bagheri E, Lu W, Ghorbani AA. A detailed analysis of the kdd cup 99 data set. In. IEEE symposium on computational intelligence for security and defense applications. IEEE. 2009;2009:1–6.
Tsagkias M. Tracy HK, Surya K, Vanessa M, de Rijke M. Challenges and research opportunities in ecommerce search and recommendations. In: ACM SIGIR Forum. volume 54. NY, USA: ACM New York; 2021. p. 1–23.
Wagstaff K, Cardie C, Rogers S, Schrödl S, et al. Constrained k-means clustering with background knowledge. Icml. 2001;1:577–84.
Wang W, Yang J, Muntz R, et al. Sting: a statistical information grid approach to spatial data mining. VLDB. 1997;97:186–95.
Wei P, Li Y, Zhang Z, Tao H, Li Z, Liu D. An optimization method for intrusion detection classification model based on deep belief network. IEEE Access. 2019;7:87593–605.
Weiss K, Khoshgoftaar TM, Wang DD. A survey of transfer learning. J Big data. 2016;3(1):9.
Witten IH, Frank E. Data Mining: Practical machine learning tools and techniques. Morgan Kaufmann; 2005.
Witten IH, Frank E, Trigg LE, Hall MA, Holmes G, Cunningham SJ. Weka: practical machine learning tools and techniques with java implementations. 1999.
Wu C-C, Yen-Liang C, Yi-Hung L, Xiang-Yu Y. Decision tree induction with a constrained number of leaf nodes. Appl Intell. 2016;45(3):673–85.
Wu X, Kumar V, Quinlan JR, Ghosh J, Yang Q, Motoda H, McLachlan GJ, Ng A, Liu B, Philip SY, et al. Top 10 algorithms in data mining. Knowl Inform Syst. 2008;14(1):1–37.
Xin Y, Kong L, Liu Z, Chen Y, Li Y, Zhu H, Gao M, Hou H, Wang C. Machine learning and deep learning methods for cybersecurity. IEEE Access. 2018;6:35365–81.
Xu D, Yingjie T. A comprehensive survey of clustering algorithms. Ann Data Sci. 2015;2(2):165–93.
Zaki MJ. Scalable algorithms for association mining. IEEE Trans Knowl Data Eng. 2000;12(3):372–90.
Zanella A, Bui N, Castellani A, Vangelista L, Zorzi M. Internet of things for smart cities. IEEE Internet Things J. 2014;1(1):22–32.
Zhao Q, Bhowmick SS. Association rule mining: a survey. Singapore: Nanyang Technological University; 2003.
Zheng T, Xie W, Xu L, He X, Zhang Y, You M, Yang G, Chen Y. A machine learning-based framework to identify type 2 diabetes through electronic health records. Int J Med Inform. 2017;97:120–7.
Zheng Y, Rajasegarar S, Leckie C. Parking availability prediction for sensor-enabled car parks in smart cities. In: Intelligent Sensors, Sensor Networks and Information Processing (ISSNIP), 2015 IEEE Tenth International Conference on. IEEE, 2015; pages 1–6.
Zhu H, Cao H, Chen E, Xiong H, Tian J. Exploiting enriched contextual information for mobile app classification. In: Proceedings of the 21st ACM international conference on Information and knowledge management. ACM, 2012; pages 1617–1621
Zhu H, Chen E, Xiong H, Kuifei Y, Cao H, Tian J. Mining mobile user preferences for personalized context-aware recommendation. ACM Trans Intell Syst Technol (TIST). 2014;5(4):58.
Zikang H, Yong Y, Guofeng Y, Xinyu Z. Sentiment analysis of agricultural product ecommerce review data based on deep learning. In: 2020 International Conference on Internet of Things and Intelligent Applications (ITIA), IEEE, 2020; pages 1–7
Zulkernain S, Madiraju P, Ahamed SI. A context aware interruption management system for mobile devices. In: Mobile Wireless Middleware, Operating Systems, and Applications. Springer. 2010; pages 221–234
Zulkernain S, Madiraju P, Ahamed S, Stamm K. A mobile intelligent interruption management system. J UCS. 2010;16(15):2060–80.
Download references
Author information
Authors and affiliations.
Swinburne University of Technology, Melbourne, VIC, 3122, Australia
Iqbal H. Sarker
Department of Computer Science and Engineering, Chittagong University of Engineering & Technology, 4349, Chattogram, Bangladesh
You can also search for this author in PubMed Google Scholar
Corresponding author
Correspondence to Iqbal H. Sarker .
Ethics declarations
Conflict of interest.
The author declares no conflict of interest.
Additional information
Publisher's note.
Springer Nature remains neutral with regard to jurisdictional claims in published maps and institutional affiliations.
This article is part of the topical collection “Advances in Computational Approaches for Artificial Intelligence, Image Processing, IoT and Cloud Applications” guest edited by Bhanu Prakash K N and M. Shivakumar.
Rights and permissions
Reprints and permissions
About this article
Sarker, I.H. Machine Learning: Algorithms, Real-World Applications and Research Directions. SN COMPUT. SCI. 2 , 160 (2021). https://doi.org/10.1007/s42979-021-00592-x
Download citation
Received : 27 January 2021
Accepted : 12 March 2021
Published : 22 March 2021
DOI : https://doi.org/10.1007/s42979-021-00592-x
Share this article
Anyone you share the following link with will be able to read this content:
Sorry, a shareable link is not currently available for this article.
Provided by the Springer Nature SharedIt content-sharing initiative
- Machine learning
- Deep learning
- Artificial intelligence
- Data science
- Data-driven decision-making
- Predictive analytics
- Intelligent applications
- Find a journal
- Publish with us
- Track your research
Database Management Systems (DBMS)
Database group website: db.cs.berkeley.edu
Declarative languages and runtime systems
Design and implementation of declarative programming languages with applications to distributed systems, networking, machine learning, metadata management, and interactive visualization; design of query interface for applications.
Scalable data analysis and query processing
Scalable data processing in new settings, including interactive exploration, metadata management, cloud and serverless environments, and machine learning; query processing on compressed, semi-structured, and streaming data; query processing with additional constraints, including fairness, resource utilization, and cost.
Consistency, concurrency, coordination and reliability
Coordination avoidance, consistency and monotonicity analysis; transaction isolation levels and protocols; distributed analytics and data management, geo-replication; fault tolerance and fault injection.
Data storage and physical design
Hot and cold storage; immutable data structures; indexing and data skipping; versioning; new data types; implications of hardware evolution.
Metadata management
Data lineage and versioning; usage tracking and collective intelligence; scalability of metadata management services; metadata representations; reproducibility and debugging of data pipelines.
Systems for machine learning and model management
Distributed machine learning and graph analytics; physical and logical optimization of machine learning pipelines; online model management and maintenance; prediction serving; real-time personalization; latency-accuracy tradeoffs and edge computing for large-scale models; machine learning lifecycle management.
Data cleaning, data transformation, and crowdsourcing
Human-data interaction including interactive transformation, query authoring, and crowdsourcing; machine learning for data cleaning; statistical properties of data cleaning pipelines; end-to-end systems for crowdsourcing.
Interactive data exploration and visualization
Interactive querying and direct manipulation; scalable spreadsheets and data visualization; languages and interfaces for interactive exploration; progressive query visualization; predictive interaction.
Secure data processing
Data processing under homomorphic encryption; data compression and encryption; differential privacy; oblivious data processing; databases in secure hardware enclaves.
Foundations of data management
Optimal trade-offs between storage, quality, latency, and cost, with applications to crowdsourcing, distributed data management, stream data processing, version management; expressiveness, complexity, and completeness of data representations, query languages, and query processing; query processing with fairness constraints.
Research Centers
- EPIC Data lab
- Sky Computing Lab
- Alvin Cheung
- Natacha Crooks
- Joseph Gonzalez
- Joseph M. Hellerstein (coordinator)
- Jiantao Jiao
- Aditya Parameswaran
- Matei Zaharia
- Eric Brewer
- Michael Lustig
- Jelani Nelson
Faculty Awards
- ACM Prize in Computing: Eric Brewer, 2009.
- National Academy of Engineering (NAE) Member: Ion Stoica, 2024. Eric Brewer, 2007.
- American Academy of Arts and Sciences Member: Eric Brewer, 2018.
- Sloan Research Fellow: Aditya Parameswaran, 2020. Alvin Cheung, 2019. Jelani Nelson, 2017. Michael Lustig, 2013. Ion Stoica, 2003. Joseph M. Hellerstein, 1998. Eric Brewer, 1997.
Related Courses
- CS 186. Introduction to Database Systems
- CS 262A. Advanced Topics in Computer Systems
Computer Science
Overview - library research for computer science, quick start, need more help, tutorial: understanding journals, literature searches, what is libkey nomad, library article databases, browse journals using browzine, find computer science books, computer science books you can check out or read online, e-book collections, e-reference sources, find web sources, associations, organizations, & societies, interesting websites, find journals, finding a specific journal, browsing journals using browzine, interlibrary loan, library research for computer science.
This guide will help you get started searching the computer science "literature" for research papers on your topic and finding other resources such as books, journals, government websites, and published theses and dissertations.
Be sure to visit the different pages of this guide using the tabs on the left.
Library Search connects you to books, articles, and a variety of other library sources.
Library Search at Rowan University Libraries
Schedule an appointment with me:
- Request a Research Consultation
- Understanding Journals tutorial A short online tutorial for students on how to understand and use library journals.
Computer Science Articles
We recommend that you use the specialized subscription databases on the library website to search for engineering "literature" (papers) because you will see only content that the library subscribes to.
However if you prefer to use Google Scholar, you can create a Google Scholar profile with your Rowan University credentials , which will give you access to the library's subscription content.
Then when you search in Google Scholar, you will see links to the right of some results labeled Full Text @Rowan University . Selecting one of these links will take you to the subscribed content.

LibKey Nomad is a browser extension for the Chrome, Firefox, and Edge web browsers.
Once you install it, you can use it to download full text articles to which we subscribe, from any publisher website where you find them. (Otherwise you would need to go to the library website to access the full text if you are off campus.)

When the full text PDF is available to you, you will see a LibKey Download PDF icon in the bottom left corner of the page.
If it is not, you will see a button labeled Access Options which will direct you to our Interlibrary Loan system.
Watch the short video shown below to learn how to download the extension for the browser you use.
- LibKey Nomad Installation Video showing you how to install LibKey Nomad
The following subscription databases available through the library website contain scholarly articles in Computer Science.
- ACM Digital Library This link opens in a new window Academic journal articles in computer science. more... less... Full text of every article ever published by ACM (the professional organization for computer scientists) and bibliographic citations from major publishers in computing. (WALDO consortial access.)
- IEEE Xplore This link opens in a new window Journal and conference papers in electrical engineering. more... less... Full text access to journals and major conference proceedings published by the Institute of Electrical and Electronic Engineers (IEEE).
- MathSciNet This link opens in a new window The American Mathematical Society’s index to mathematical literature. more... less... Contains references, abstracts, and reviews from mathematical journals, conference proceedings, books, and dissertations.
- Web of Science This link opens in a new window Full text database of scientific journal articles with data on who has cited them. more... less... Web of Science® provides immediate data on who has cited research papers, covering over 12,000 of the highest impact journals worldwide, including Open Access journals and over 150,000 conference proceedings. You'll find current and retrospective coverage in the sciences, social sciences, arts, and humanities, with coverage to 1900.
- Scopus This link opens in a new window SciVerse Scopus is the world’s largest abstract and citation database of peer-reviewed literature and quality web sources.
- SIAM Journals Online Collection of journals published by the Society for Industrial and Applied Mathematics. more... less... Rowan account required.
Computer Science Books
This page explains ways to find computer science books through the library.
Computer science books are located in the QA76 section on the 4th floor of Campbell Library. Most programming books are in QA76 but some are in the TK (computer engineering) section.
- LibrarySearch Use Library Search to look up books by topic or title. Use the Books facet.
- Ebook Central ebook collection This link opens in a new window Collection of scholarly e-books in many academic disciplines. more... less... Multidisciplinary collection of scholarly ebooks, offering a strong collection of academic titles from leading scholarly publishers. Includes subscribed and purchased content. It is not a permanent acquisition of e-books, and is subject to change.
These subscription sources are available through the library website.
- Credo Reference - Science An online database which includes many science dictionaries and encyclopedias.
Computer Science Websites
This page highlights good websites for computer science research.
- American Association for Artificial Intelligence more... less... The AAAI, founded in 1979, is a scientific society devoted to advancing the scientific understanding of the mechanisms underlying thought and intelligent behavior and their embodiment in machines. Their site contains information on artificial intelligence, AAAI publications (book, journals, conference proceedings, and technical papers); conference, workshop, and symposia information; and membership benefits.
- ANSI standards search
- Association for Computing Machinery more... less... Billing itself as "the first society in computing," the ACM is the world's first educational and scientific computing society. Founded in 1947, its membership currently totals over 80,000 computing professionals and students world wide. The site includes information about ACM activities, services, conferences, publications, and policies. The ACM Digital Library contains full text of articles and papers from all of the their journals, magazines, and proceedings.
- IEEE Computer Society more... less... Founded in 1947, the IEEE Computer Society is the world's oldest and largest (98,000 members) professional association of people in computing. The site contains a full range of information about conferences, standards, publications, activities, education, certification, and employment. The Digital Library provides access to the Computer Society's magazines, transactions, and a growing body of conference proceedings.
- Society for Industrial and Applied Mathematics more... less... A group of professionals formed SIAM in 1952 to advance the application of mathematics to science and industry. SIAM members are computer scientists, mathematicians, engineers, statisticians, and engineers. This site includes information about their publications, conferences, meetings.
- Special Interest Group on Human-Computer Interaction
- ArXiv Pre-print repository of articles in Physics, Mathematics, and Computer Science maintained by Cornell University.
- Software Engineering Institute SEI is a federally-funded research and development center headquartered on the campus of Carnegie Mellon University.
- HCI Bibliography A site offering a bibliography of human-computer interaction resources.
- How to Get a Computer Science Job Commercial website with advice on getting your first professional job in computer science.
Computer Science Journals
This page offers several options for finding scientific journals containing research on computer science.
To discover whether the library subscribes to a specific journal, and see content in that journal, go to the Search Tools section of the Campbell Library home page. Click on the Journal Finder option.
Enter the complete name of the journal (no abbreviations) - for example, "Environmental & Engineering Geoscience."
If the journal is listed in the results, click on Available Online . Then scroll down to View Online and choose a database link. In this example there should be a link for the database GeoScienceWorld .
If you are looking for a specific issue of the journal, there is usually a way to browse volumes and issues to drill down to the desired issue.
Or, search within the journal using the Search box in the top right corner to find articles on a topic.
Journal articles to which we do not have full text access may be requested through our online Interlibrary Loan request system, Illiad. Articles are usually sent to your email address within a few days.
- Campbell Library Interlibrary Loan logon
- Last Updated: May 16, 2024 1:45 PM
- URL: https://libguides.rowan.edu/ComputerScience
Thank you for visiting nature.com. You are using a browser version with limited support for CSS. To obtain the best experience, we recommend you use a more up to date browser (or turn off compatibility mode in Internet Explorer). In the meantime, to ensure continued support, we are displaying the site without styles and JavaScript.
- View all journals
- My Account Login
- Explore content
- About the journal
- Publish with us
- Sign up for alerts
- Data Descriptor
- Open access
- Published: 03 May 2024
A dataset for measuring the impact of research data and their curation
- Libby Hemphill ORCID: orcid.org/0000-0002-3793-7281 1 , 2 ,
- Andrea Thomer 3 ,
- Sara Lafia 1 ,
- Lizhou Fan 2 ,
- David Bleckley ORCID: orcid.org/0000-0001-7715-4348 1 &
- Elizabeth Moss 1
Scientific Data volume 11 , Article number: 442 ( 2024 ) Cite this article
686 Accesses
8 Altmetric
Metrics details
- Research data
- Social sciences
Science funders, publishers, and data archives make decisions about how to responsibly allocate resources to maximize the reuse potential of research data. This paper introduces a dataset developed to measure the impact of archival and data curation decisions on data reuse. The dataset describes 10,605 social science research datasets, their curation histories, and reuse contexts in 94,755 publications that cover 59 years from 1963 to 2022. The dataset was constructed from study-level metadata, citing publications, and curation records available through the Inter-university Consortium for Political and Social Research (ICPSR) at the University of Michigan. The dataset includes information about study-level attributes (e.g., PIs, funders, subject terms); usage statistics (e.g., downloads, citations); archiving decisions (e.g., curation activities, data transformations); and bibliometric attributes (e.g., journals, authors) for citing publications. This dataset provides information on factors that contribute to long-term data reuse, which can inform the design of effective evidence-based recommendations to support high-impact research data curation decisions.
Similar content being viewed by others
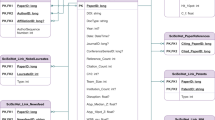
SciSciNet: A large-scale open data lake for the science of science research
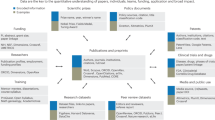
Data, measurement and empirical methods in the science of science
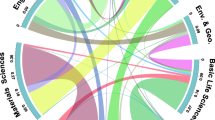
Interdisciplinarity revisited: evidence for research impact and dynamism
Background & summary.
Recent policy changes in funding agencies and academic journals have increased data sharing among researchers and between researchers and the public. Data sharing advances science and provides the transparency necessary for evaluating, replicating, and verifying results. However, many data-sharing policies do not explain what constitutes an appropriate dataset for archiving or how to determine the value of datasets to secondary users 1 , 2 , 3 . Questions about how to allocate data-sharing resources efficiently and responsibly have gone unanswered 4 , 5 , 6 . For instance, data-sharing policies recognize that not all data should be curated and preserved, but they do not articulate metrics or guidelines for determining what data are most worthy of investment.
Despite the potential for innovation and advancement that data sharing holds, the best strategies to prioritize datasets for preparation and archiving are often unclear. Some datasets are likely to have more downstream potential than others, and data curation policies and workflows should prioritize high-value data instead of being one-size-fits-all. Though prior research in library and information science has shown that the “analytic potential” of a dataset is key to its reuse value 7 , work is needed to implement conceptual data reuse frameworks 8 , 9 , 10 , 11 , 12 , 13 , 14 . In addition, publishers and data archives need guidance to develop metrics and evaluation strategies to assess the impact of datasets.
Several existing resources have been compiled to study the relationship between the reuse of scholarly products, such as datasets (Table 1 ); however, none of these resources include explicit information on how curation processes are applied to data to increase their value, maximize their accessibility, and ensure their long-term preservation. The CCex (Curation Costs Exchange) provides models of curation services along with cost-related datasets shared by contributors but does not make explicit connections between them or include reuse information 15 . Analyses on platforms such as DataCite 16 have focused on metadata completeness and record usage, but have not included related curation-level information. Analyses of GenBank 17 and FigShare 18 , 19 citation networks do not include curation information. Related studies of Github repository reuse 20 and Softcite software citation 21 reveal significant factors that impact the reuse of secondary research products but do not focus on research data. RD-Switchboard 22 and DSKG 23 are scholarly knowledge graphs linking research data to articles, patents, and grants, but largely omit social science research data and do not include curation-level factors. To our knowledge, other studies of curation work in organizations similar to ICPSR – such as GESIS 24 , Dataverse 25 , and DANS 26 – have not made their underlying data available for analysis.
This paper describes a dataset 27 compiled for the MICA project (Measuring the Impact of Curation Actions) led by investigators at ICPSR, a large social science data archive at the University of Michigan. The dataset was originally developed to study the impacts of data curation and archiving on data reuse. The MICA dataset has supported several previous publications investigating the intensity of data curation actions 28 , the relationship between data curation actions and data reuse 29 , and the structures of research communities in a data citation network 30 . Collectively, these studies help explain the return on various types of curatorial investments. The dataset that we introduce in this paper, which we refer to as the MICA dataset, has the potential to address research questions in the areas of science (e.g., knowledge production), library and information science (e.g., scholarly communication), and data archiving (e.g., reproducible workflows).
We constructed the MICA dataset 27 using records available at ICPSR, a large social science data archive at the University of Michigan. Data set creation involved: collecting and enriching metadata for articles indexed in the ICPSR Bibliography of Data-related Literature against the Dimensions AI bibliometric database; gathering usage statistics for studies from ICPSR’s administrative database; processing data curation work logs from ICPSR’s project tracking platform, Jira; and linking data in social science studies and series to citing analysis papers (Fig. 1 ).
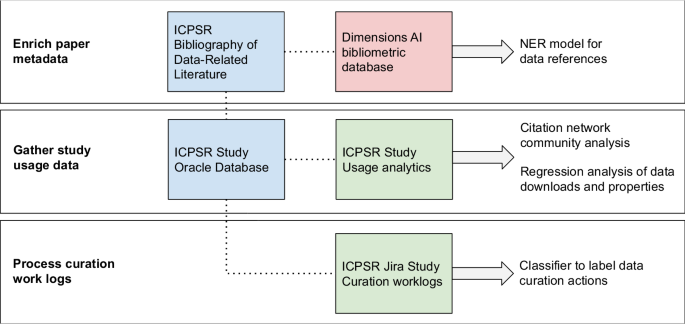
Steps to prepare MICA dataset for analysis - external sources are red, primary internal sources are blue, and internal linked sources are green.
Enrich paper metadata
The ICPSR Bibliography of Data-related Literature is a growing database of literature in which data from ICPSR studies have been used. Its creation was funded by the National Science Foundation (Award 9977984), and for the past 20 years it has been supported by ICPSR membership and multiple US federally-funded and foundation-funded topical archives at ICPSR. The Bibliography was originally launched in the year 2000 to aid in data discovery by providing a searchable database linking publications to the study data used in them. The Bibliography collects the universe of output based on the data shared in each study through, which is made available through each ICPSR study’s webpage. The Bibliography contains both peer-reviewed and grey literature, which provides evidence for measuring the impact of research data. For an item to be included in the ICPSR Bibliography, it must contain an analysis of data archived by ICPSR or contain a discussion or critique of the data collection process, study design, or methodology 31 . The Bibliography is manually curated by a team of librarians and information specialists at ICPSR who enter and validate entries. Some publications are supplied to the Bibliography by data depositors, and some citations are submitted to the Bibliography by authors who abide by ICPSR’s terms of use requiring them to submit citations to works in which they analyzed data retrieved from ICPSR. Most of the Bibliography is populated by Bibliography team members, who create custom queries for ICPSR studies performed across numerous sources, including Google Scholar, ProQuest, SSRN, and others. Each record in the Bibliography is one publication that has used one or more ICPSR studies. The version we used was captured on 2021-11-16 and included 94,755 publications.
To expand the coverage of the ICPSR Bibliography, we searched exhaustively for all ICPSR study names, unique numbers assigned to ICPSR studies, and DOIs 32 using a full-text index available through the Dimensions AI database 33 . We accessed Dimensions through a license agreement with the University of Michigan. ICPSR Bibliography librarians and information specialists manually reviewed and validated new entries that matched one or more search criteria. We then used Dimensions to gather enriched metadata and full-text links for items in the Bibliography with DOIs. We matched 43% of the items in the Bibliography to enriched Dimensions metadata including abstracts, field of research codes, concepts, and authors’ institutional information; we also obtained links to full text for 16% of Bibliography items. Based on licensing agreements, we included Dimensions identifiers and links to full text so that users with valid publisher and database access can construct an enriched publication dataset.
Gather study usage data
ICPSR maintains a relational administrative database, DBInfo, that organizes study-level metadata and information on data reuse across separate tables. Studies at ICPSR consist of one or more files collected at a single time or for a single purpose; studies in which the same variables are observed over time are grouped into series. Each study at ICPSR is assigned a DOI, and its metadata are stored in DBInfo. Study metadata follows the Data Documentation Initiative (DDI) Codebook 2.5 standard. DDI elements included in our dataset are title, ICPSR study identification number, DOI, authoring entities, description (abstract), funding agencies, subject terms assigned to the study during curation, and geographic coverage. We also created variables based on DDI elements: total variable count, the presence of survey question text in the metadata, the number of author entities, and whether an author entity was an institution. We gathered metadata for ICPSR’s 10,605 unrestricted public-use studies available as of 2021-11-16 ( https://www.icpsr.umich.edu/web/pages/membership/or/metadata/oai.html ).
To link study usage data with study-level metadata records, we joined study metadata from DBinfo on study usage information, which included total study downloads (data and documentation), individual data file downloads, and cumulative citations from the ICPSR Bibliography. We also gathered descriptive metadata for each study and its variables, which allowed us to summarize and append recoded fields onto the study-level metadata such as curation level, number and type of principle investigators, total variable count, and binary variables indicating whether the study data were made available for online analysis, whether survey question text was made searchable online, and whether the study variables were indexed for search. These characteristics describe aspects of the discoverability of the data to compare with other characteristics of the study. We used the study and series numbers included in the ICPSR Bibliography as unique identifiers to link papers to metadata and analyze the community structure of dataset co-citations in the ICPSR Bibliography 32 .
Process curation work logs
Researchers deposit data at ICPSR for curation and long-term preservation. Between 2016 and 2020, more than 3,000 research studies were deposited with ICPSR. Since 2017, ICPSR has organized curation work into a central unit that provides varied levels of curation that vary in the intensity and complexity of data enhancement that they provide. While the levels of curation are standardized as to effort (level one = less effort, level three = most effort), the specific curatorial actions undertaken for each dataset vary. The specific curation actions are captured in Jira, a work tracking program, which data curators at ICPSR use to collaborate and communicate their progress through tickets. We obtained access to a corpus of 669 completed Jira tickets corresponding to the curation of 566 unique studies between February 2017 and December 2019 28 .
To process the tickets, we focused only on their work log portions, which contained free text descriptions of work that data curators had performed on a deposited study, along with the curators’ identifiers, and timestamps. To protect the confidentiality of the data curators and the processing steps they performed, we collaborated with ICPSR’s curation unit to propose a classification scheme, which we used to train a Naive Bayes classifier and label curation actions in each work log sentence. The eight curation action labels we proposed 28 were: (1) initial review and planning, (2) data transformation, (3) metadata, (4) documentation, (5) quality checks, (6) communication, (7) other, and (8) non-curation work. We note that these categories of curation work are very specific to the curatorial processes and types of data stored at ICPSR, and may not match the curation activities at other repositories. After applying the classifier to the work log sentences, we obtained summary-level curation actions for a subset of all ICPSR studies (5%), along with the total number of hours spent on data curation for each study, and the proportion of time associated with each action during curation.
Data Records
The MICA dataset 27 connects records for each of ICPSR’s archived research studies to the research publications that use them and related curation activities available for a subset of studies (Fig. 2 ). Each of the three tables published in the dataset is available as a study archived at ICPSR. The data tables are distributed as statistical files available for use in SAS, SPSS, Stata, and R as well as delimited and ASCII text files. The dataset is organized around studies and papers as primary entities. The studies table lists ICPSR studies, their metadata attributes, and usage information; the papers table was constructed using the ICPSR Bibliography and Dimensions database; and the curation logs table summarizes the data curation steps performed on a subset of ICPSR studies.
Studies (“ICPSR_STUDIES”): 10,605 social science research datasets available through ICPSR up to 2021-11-16 with variables for ICPSR study number, digital object identifier, study name, series number, series title, authoring entities, full-text description, release date, funding agency, geographic coverage, subject terms, topical archive, curation level, single principal investigator (PI), institutional PI, the total number of PIs, total variables in data files, question text availability, study variable indexing, level of restriction, total unique users downloading study data files and codebooks, total unique users downloading data only, and total unique papers citing data through November 2021. Studies map to the papers and curation logs table through ICPSR study numbers as “STUDY”. However, not every study in this table will have records in the papers and curation logs tables.
Papers (“ICPSR_PAPERS”): 94,755 publications collected from 2000-08-11 to 2021-11-16 in the ICPSR Bibliography and enriched with metadata from the Dimensions database with variables for paper number, identifier, title, authors, publication venue, item type, publication date, input date, ICPSR series numbers used in the paper, ICPSR study numbers used in the paper, the Dimension identifier, and the Dimensions link to the publication’s full text. Papers map to the studies table through ICPSR study numbers in the “STUDY_NUMS” field. Each record represents a single publication, and because a researcher can use multiple datasets when creating a publication, each record may list multiple studies or series.
Curation logs (“ICPSR_CURATION_LOGS”): 649 curation logs for 563 ICPSR studies (although most studies in the subset had one curation log, some studies were associated with multiple logs, with a maximum of 10) curated between February 2017 and December 2019 with variables for study number, action labels assigned to work description sentences using a classifier trained on ICPSR curation logs, hours of work associated with a single log entry, and total hours of work logged for the curation ticket. Curation logs map to the study and paper tables through ICPSR study numbers as “STUDY”. Each record represents a single logged action, and future users may wish to aggregate actions to the study level before joining tables.
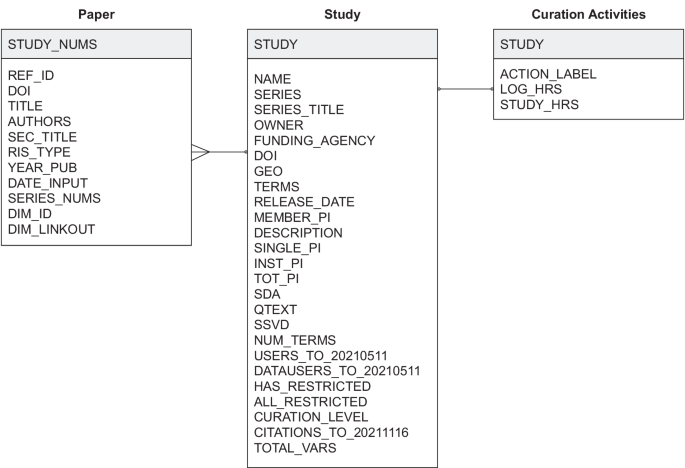
Entity-relation diagram.
Technical Validation
We report on the reliability of the dataset’s metadata in the following subsections. To support future reuse of the dataset, curation services provided through ICPSR improved data quality by checking for missing values, adding variable labels, and creating a codebook.
All 10,605 studies available through ICPSR have a DOI and a full-text description summarizing what the study is about, the purpose of the study, the main topics covered, and the questions the PIs attempted to answer when they conducted the study. Personal names (i.e., principal investigators) and organizational names (i.e., funding agencies) are standardized against an authority list maintained by ICPSR; geographic names and subject terms are also standardized and hierarchically indexed in the ICPSR Thesaurus 34 . Many of ICPSR’s studies (63%) are in a series and are distributed through the ICPSR General Archive (56%), a non-topical archive that accepts any social or behavioral science data. While study data have been available through ICPSR since 1962, the earliest digital release date recorded for a study was 1984-03-18, when ICPSR’s database was first employed, and the most recent date is 2021-10-28 when the dataset was collected.
Curation level information was recorded starting in 2017 and is available for 1,125 studies (11%); approximately 80% of studies with assigned curation levels received curation services, equally distributed between Levels 1 (least intensive), 2 (moderately intensive), and 3 (most intensive) (Fig. 3 ). Detailed descriptions of ICPSR’s curation levels are available online 35 . Additional metadata are available for a subset of 421 studies (4%), including information about whether the study has a single PI, an institutional PI, the total number of PIs involved, total variables recorded is available for online analysis, has searchable question text, has variables that are indexed for search, contains one or more restricted files, and whether the study is completely restricted. We provided additional metadata for this subset of ICPSR studies because they were released within the past five years and detailed curation and usage information were available for them. Usage statistics including total downloads and data file downloads are available for this subset of studies as well; citation statistics are available for 8,030 studies (76%). Most ICPSR studies have fewer than 500 users, as indicated by total downloads, or citations (Fig. 4 ).
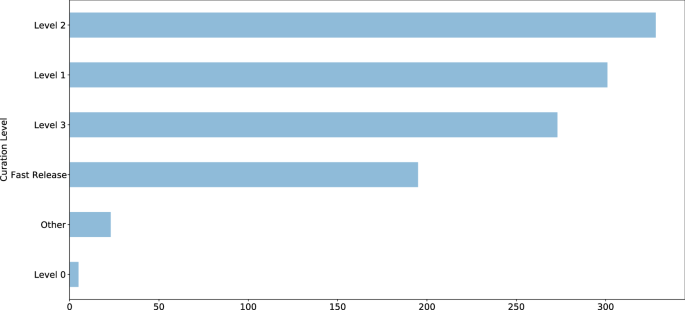
ICPSR study curation levels.
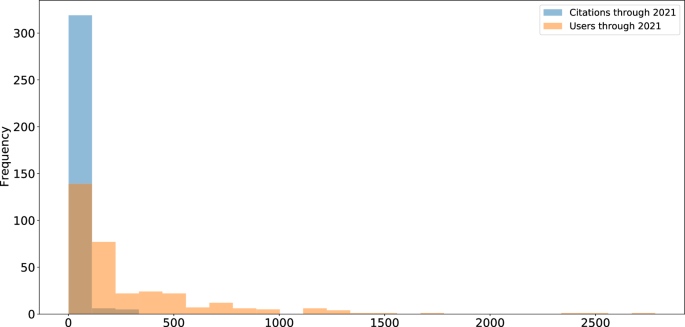
ICPSR study usage.
A subset of 43,102 publications (45%) available in the ICPSR Bibliography had a DOI. Author metadata were entered as free text, meaning that variations may exist and require additional normalization and pre-processing prior to analysis. While author information is standardized for each publication, individual names may appear in different sort orders (e.g., “Earls, Felton J.” and “Stephen W. Raudenbush”). Most of the items in the ICPSR Bibliography as of 2021-11-16 were journal articles (59%), reports (14%), conference presentations (9%), or theses (8%) (Fig. 5 ). The number of publications collected in the Bibliography has increased each decade since the inception of ICPSR in 1962 (Fig. 6 ). Most ICPSR studies (76%) have one or more citations in a publication.
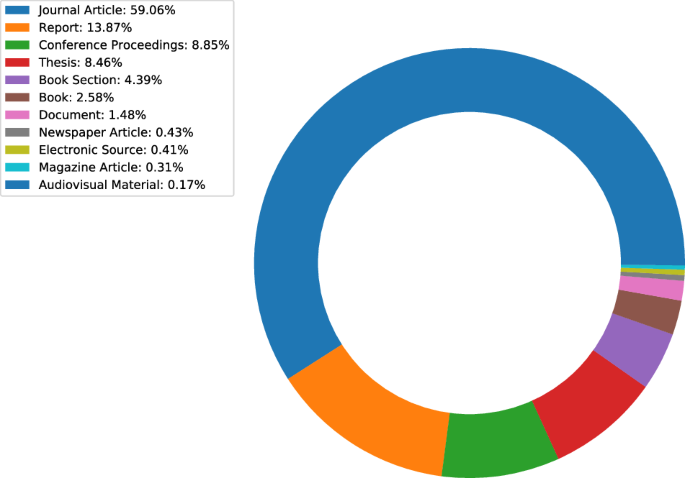
ICPSR Bibliography citation types.
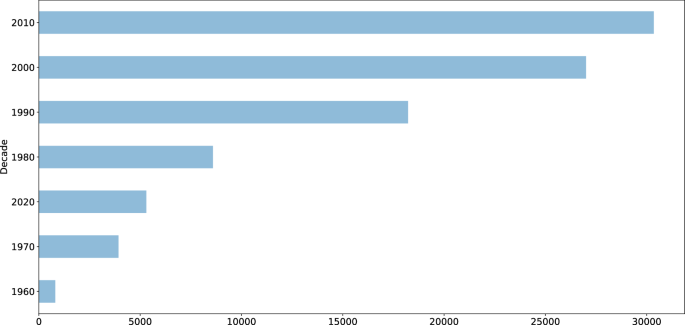
ICPSR citations by decade.
Usage Notes
The dataset consists of three tables that can be joined using the “STUDY” key as shown in Fig. 2 . The “ICPSR_PAPERS” table contains one row per paper with one or more cited studies in the “STUDY_NUMS” column. We manipulated and analyzed the tables as CSV files with the Pandas library 36 in Python and the Tidyverse packages 37 in R.
The present MICA dataset can be used independently to study the relationship between curation decisions and data reuse. Evidence of reuse for specific studies is available in several forms: usage information, including downloads and citation counts; and citation contexts within papers that cite data. Analysis may also be performed on the citation network formed between datasets and papers that use them. Finally, curation actions can be associated with properties of studies and usage histories.
This dataset has several limitations of which users should be aware. First, Jira tickets can only be used to represent the intensiveness of curation for activities undertaken since 2017, when ICPSR started using both Curation Levels and Jira. Studies published before 2017 were all curated, but documentation of the extent of that curation was not standardized and therefore could not be included in these analyses. Second, the measure of publications relies upon the authors’ clarity of data citation and the ICPSR Bibliography staff’s ability to discover citations with varying formality and clarity. Thus, there is always a chance that some secondary-data-citing publications have been left out of the bibliography. Finally, there may be some cases in which a paper in the ICSPSR bibliography did not actually obtain data from ICPSR. For example, PIs have often written about or even distributed their data prior to their archival in ICSPR. Therefore, those publications would not have cited ICPSR but they are still collected in the Bibliography as being directly related to the data that were eventually deposited at ICPSR.
In summary, the MICA dataset contains relationships between two main types of entities – papers and studies – which can be mined. The tables in the MICA dataset have supported network analysis (community structure and clique detection) 30 ; natural language processing (NER for dataset reference detection) 32 ; visualizing citation networks (to search for datasets) 38 ; and regression analysis (on curation decisions and data downloads) 29 . The data are currently being used to develop research metrics and recommendation systems for research data. Given that DOIs are provided for ICPSR studies and articles in the ICPSR Bibliography, the MICA dataset can also be used with other bibliometric databases, including DataCite, Crossref, OpenAlex, and related indexes. Subscription-based services, such as Dimensions AI, are also compatible with the MICA dataset. In some cases, these services provide abstracts or full text for papers from which data citation contexts can be extracted for semantic content analysis.
Code availability
The code 27 used to produce the MICA project dataset is available on GitHub at https://github.com/ICPSR/mica-data-descriptor and through Zenodo with the identifier https://doi.org/10.5281/zenodo.8432666 . Data manipulation and pre-processing were performed in Python. Data curation for distribution was performed in SPSS.
He, L. & Han, Z. Do usage counts of scientific data make sense? An investigation of the Dryad repository. Library Hi Tech 35 , 332–342 (2017).
Article Google Scholar
Brickley, D., Burgess, M. & Noy, N. Google dataset search: Building a search engine for datasets in an open web ecosystem. In The World Wide Web Conference - WWW ‘19 , 1365–1375 (ACM Press, San Francisco, CA, USA, 2019).
Buneman, P., Dosso, D., Lissandrini, M. & Silvello, G. Data citation and the citation graph. Quantitative Science Studies 2 , 1399–1422 (2022).
Chao, T. C. Disciplinary reach: Investigating the impact of dataset reuse in the earth sciences. Proceedings of the American Society for Information Science and Technology 48 , 1–8 (2011).
Article ADS Google Scholar
Parr, C. et al . A discussion of value metrics for data repositories in earth and environmental sciences. Data Science Journal 18 , 58 (2019).
Eschenfelder, K. R., Shankar, K. & Downey, G. The financial maintenance of social science data archives: Four case studies of long–term infrastructure work. J. Assoc. Inf. Sci. Technol. 73 , 1723–1740 (2022).
Palmer, C. L., Weber, N. M. & Cragin, M. H. The analytic potential of scientific data: Understanding re-use value. Proceedings of the American Society for Information Science and Technology 48 , 1–10 (2011).
Zimmerman, A. S. New knowledge from old data: The role of standards in the sharing and reuse of ecological data. Sci. Technol. Human Values 33 , 631–652 (2008).
Cragin, M. H., Palmer, C. L., Carlson, J. R. & Witt, M. Data sharing, small science and institutional repositories. Philosophical Transactions of the Royal Society A: Mathematical, Physical and Engineering Sciences 368 , 4023–4038 (2010).
Article ADS CAS Google Scholar
Fear, K. M. Measuring and Anticipating the Impact of Data Reuse . Ph.D. thesis, University of Michigan (2013).
Borgman, C. L., Van de Sompel, H., Scharnhorst, A., van den Berg, H. & Treloar, A. Who uses the digital data archive? An exploratory study of DANS. Proceedings of the Association for Information Science and Technology 52 , 1–4 (2015).
Pasquetto, I. V., Borgman, C. L. & Wofford, M. F. Uses and reuses of scientific data: The data creators’ advantage. Harvard Data Science Review 1 (2019).
Gregory, K., Groth, P., Scharnhorst, A. & Wyatt, S. Lost or found? Discovering data needed for research. Harvard Data Science Review (2020).
York, J. Seeking equilibrium in data reuse: A study of knowledge satisficing . Ph.D. thesis, University of Michigan (2022).
Kilbride, W. & Norris, S. Collaborating to clarify the cost of curation. New Review of Information Networking 19 , 44–48 (2014).
Robinson-Garcia, N., Mongeon, P., Jeng, W. & Costas, R. DataCite as a novel bibliometric source: Coverage, strengths and limitations. Journal of Informetrics 11 , 841–854 (2017).
Qin, J., Hemsley, J. & Bratt, S. E. The structural shift and collaboration capacity in GenBank networks: A longitudinal study. Quantitative Science Studies 3 , 174–193 (2022).
Article PubMed PubMed Central Google Scholar
Acuna, D. E., Yi, Z., Liang, L. & Zhuang, H. Predicting the usage of scientific datasets based on article, author, institution, and journal bibliometrics. In Smits, M. (ed.) Information for a Better World: Shaping the Global Future. iConference 2022 ., 42–52 (Springer International Publishing, Cham, 2022).
Zeng, T., Wu, L., Bratt, S. & Acuna, D. E. Assigning credit to scientific datasets using article citation networks. Journal of Informetrics 14 , 101013 (2020).
Koesten, L., Vougiouklis, P., Simperl, E. & Groth, P. Dataset reuse: Toward translating principles to practice. Patterns 1 , 100136 (2020).
Du, C., Cohoon, J., Lopez, P. & Howison, J. Softcite dataset: A dataset of software mentions in biomedical and economic research publications. J. Assoc. Inf. Sci. Technol. 72 , 870–884 (2021).
Aryani, A. et al . A research graph dataset for connecting research data repositories using RD-Switchboard. Sci Data 5 , 180099 (2018).
Färber, M. & Lamprecht, D. The data set knowledge graph: Creating a linked open data source for data sets. Quantitative Science Studies 2 , 1324–1355 (2021).
Perry, A. & Netscher, S. Measuring the time spent on data curation. Journal of Documentation 78 , 282–304 (2022).
Trisovic, A. et al . Advancing computational reproducibility in the Dataverse data repository platform. In Proceedings of the 3rd International Workshop on Practical Reproducible Evaluation of Computer Systems , P-RECS ‘20, 15–20, https://doi.org/10.1145/3391800.3398173 (Association for Computing Machinery, New York, NY, USA, 2020).
Borgman, C. L., Scharnhorst, A. & Golshan, M. S. Digital data archives as knowledge infrastructures: Mediating data sharing and reuse. Journal of the Association for Information Science and Technology 70 , 888–904, https://doi.org/10.1002/asi.24172 (2019).
Lafia, S. et al . MICA Data Descriptor. Zenodo https://doi.org/10.5281/zenodo.8432666 (2023).
Lafia, S., Thomer, A., Bleckley, D., Akmon, D. & Hemphill, L. Leveraging machine learning to detect data curation activities. In 2021 IEEE 17th International Conference on eScience (eScience) , 149–158, https://doi.org/10.1109/eScience51609.2021.00025 (2021).
Hemphill, L., Pienta, A., Lafia, S., Akmon, D. & Bleckley, D. How do properties of data, their curation, and their funding relate to reuse? J. Assoc. Inf. Sci. Technol. 73 , 1432–44, https://doi.org/10.1002/asi.24646 (2021).
Lafia, S., Fan, L., Thomer, A. & Hemphill, L. Subdivisions and crossroads: Identifying hidden community structures in a data archive’s citation network. Quantitative Science Studies 3 , 694–714, https://doi.org/10.1162/qss_a_00209 (2022).
ICPSR. ICPSR Bibliography of Data-related Literature: Collection Criteria. https://www.icpsr.umich.edu/web/pages/ICPSR/citations/collection-criteria.html (2023).
Lafia, S., Fan, L. & Hemphill, L. A natural language processing pipeline for detecting informal data references in academic literature. Proc. Assoc. Inf. Sci. Technol. 59 , 169–178, https://doi.org/10.1002/pra2.614 (2022).
Hook, D. W., Porter, S. J. & Herzog, C. Dimensions: Building context for search and evaluation. Frontiers in Research Metrics and Analytics 3 , 23, https://doi.org/10.3389/frma.2018.00023 (2018).
https://www.icpsr.umich.edu/web/ICPSR/thesaurus (2002). ICPSR. ICPSR Thesaurus.
https://www.icpsr.umich.edu/files/datamanagement/icpsr-curation-levels.pdf (2020). ICPSR. ICPSR Curation Levels.
McKinney, W. Data Structures for Statistical Computing in Python. In van der Walt, S. & Millman, J. (eds.) Proceedings of the 9th Python in Science Conference , 56–61 (2010).
Wickham, H. et al . Welcome to the Tidyverse. Journal of Open Source Software 4 , 1686 (2019).
Fan, L., Lafia, S., Li, L., Yang, F. & Hemphill, L. DataChat: Prototyping a conversational agent for dataset search and visualization. Proc. Assoc. Inf. Sci. Technol. 60 , 586–591 (2023).
Download references
Acknowledgements
We thank the ICPSR Bibliography staff, the ICPSR Data Curation Unit, and the ICPSR Data Stewardship Committee for their support of this research. This material is based upon work supported by the National Science Foundation under grant 1930645. This project was made possible in part by the Institute of Museum and Library Services LG-37-19-0134-19.
Author information
Authors and affiliations.
Inter-university Consortium for Political and Social Research, University of Michigan, Ann Arbor, MI, 48104, USA
Libby Hemphill, Sara Lafia, David Bleckley & Elizabeth Moss
School of Information, University of Michigan, Ann Arbor, MI, 48104, USA
Libby Hemphill & Lizhou Fan
School of Information, University of Arizona, Tucson, AZ, 85721, USA
Andrea Thomer
You can also search for this author in PubMed Google Scholar
Contributions
L.H. and A.T. conceptualized the study design, D.B., E.M., and S.L. prepared the data, S.L., L.F., and L.H. analyzed the data, and D.B. validated the data. All authors reviewed and edited the manuscript.
Corresponding author
Correspondence to Libby Hemphill .
Ethics declarations
Competing interests.
The authors declare no competing interests.
Additional information
Publisher’s note Springer Nature remains neutral with regard to jurisdictional claims in published maps and institutional affiliations.
Rights and permissions
Open Access This article is licensed under a Creative Commons Attribution 4.0 International License, which permits use, sharing, adaptation, distribution and reproduction in any medium or format, as long as you give appropriate credit to the original author(s) and the source, provide a link to the Creative Commons licence, and indicate if changes were made. The images or other third party material in this article are included in the article’s Creative Commons licence, unless indicated otherwise in a credit line to the material. If material is not included in the article’s Creative Commons licence and your intended use is not permitted by statutory regulation or exceeds the permitted use, you will need to obtain permission directly from the copyright holder. To view a copy of this licence, visit http://creativecommons.org/licenses/by/4.0/ .
Reprints and permissions
About this article
Cite this article.
Hemphill, L., Thomer, A., Lafia, S. et al. A dataset for measuring the impact of research data and their curation. Sci Data 11 , 442 (2024). https://doi.org/10.1038/s41597-024-03303-2
Download citation
Received : 16 November 2023
Accepted : 24 April 2024
Published : 03 May 2024
DOI : https://doi.org/10.1038/s41597-024-03303-2
Share this article
Anyone you share the following link with will be able to read this content:
Sorry, a shareable link is not currently available for this article.
Provided by the Springer Nature SharedIt content-sharing initiative
Quick links
- Explore articles by subject
- Guide to authors
- Editorial policies
Sign up for the Nature Briefing newsletter — what matters in science, free to your inbox daily.

Haifeng Xu won Best Paper Award at leading AI conference for pioneering research on mechanism design for LLMs
As this year’s Web Conference is under way, pioneering research work by Assistant Professor of Computer Science and Data Science Haifeng Xu and his collaborators has been announced as the winner for their prestigious Best Paper Award.
The Web Conference is a premier international conference on AI, Information Retrieval, and Web Technology. Since 1989, the Web Conference has focused on the future direction of the World Wide Web, and serves as a venue to present and discuss progress in research, development, standards, and applications of the topics related to the Web.
Xu’s paper, entitled “ Mechanism Design for Large Language Models ,” was selected from amongst 2008 submissions.
This paper lays out a newly developed method to aggregate language generations from multiple self-interested LLM agents into a single text generation. It does so by accounting for these LLM agents’ self-interests in an incentive-compatible way. As summarized in the meta review, “the review team unanimously finds the paper novel, well-executed, and … has potential to be a landmark paper sparking a new line of research linking LLMs and mechanism design.”
This paper is a joint work with Google Researchers. The technology Xu and his team developed has been tested on Google’s LLM model Bard and Xu reports that it performs very well. According to Xu, the nice (and often very rare) combination of both strong theoretical development and real-world implementation on Bard is probably a key reason for the paper to be named the Best Paper.
Congratulations, Haifeng!
Haifeng Xu named a AI2050 Early Career Fellow
Neurips 2023 award-winning paper by dsi faculty bo li, decodingtrust, provides a comprehensive framework for assessing trustworthiness of gpt models, more on this topic, transform cohort 3 participant healee uses ai to improve healthcare, rebecca willett awarded the siag data career prize, chicago data nights bring together academics and industry professionals in downtown chicago.
Star USC scientist faces scrutiny — retracted papers and a paused drug trial
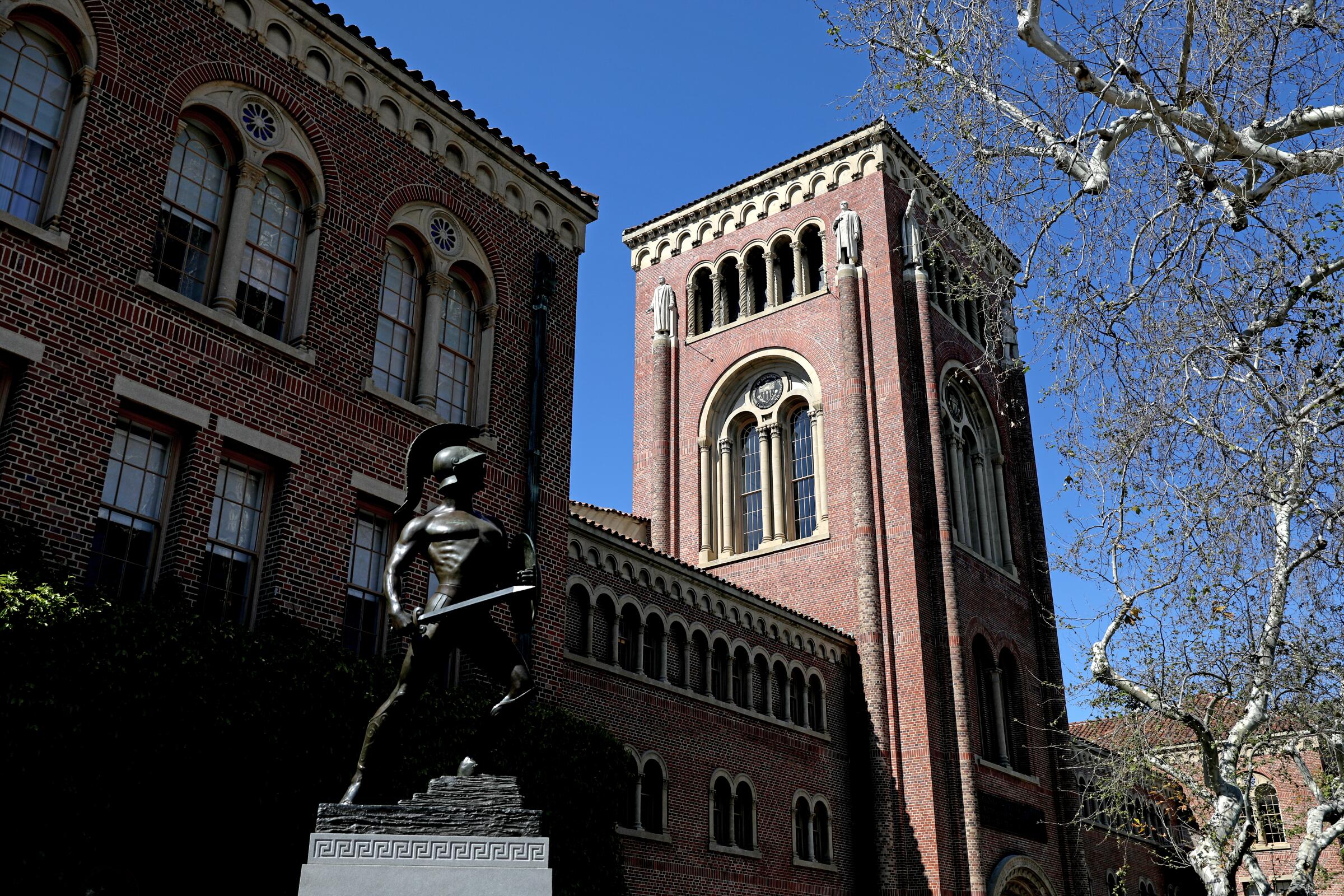
- Show more sharing options
- Copy Link URL Copied!
Late last year, a group of whistleblowers submitted a report to the National Institutes of Health that questioned the integrity of a celebrated USC neuroscientist’s research and the safety of an experimental stroke treatment his company was developing.
NIH has since paused clinical trials for 3K3A-APC, a stroke drug sponsored by ZZ Biotech, a Houston-based company co-founded by Berislav V. Zlokovic , professor and chair of the department of physiology and neuroscience at the Keck School of Medicine of USC.
Three of Zlokovic’s research papers have been retracted by the journal that published them because of problems with their data or images. Journals have issued corrections for seven more papers in which Zlokovic is the only common author, with one receiving a second correction after the new supplied data were found to have problems as well.
For an 11th paper co-authored by Zlokovic the journal Nature Medicine issued an expression of concern , a note journals append to articles when they have reason to believe there may be a problem with the paper but have not conclusively proven so. Since Zlokovic and his co-authors no longer had the original data for one of the questioned figures, the editors wrote, “[r]eaders are therefore alerted to interpret these results with caution.”
“It’s quite unusual to see this volume of retractions, corrections and expressions of concern, especially in high-tier influential papers,” said Dr. Matthew Schrag, an assistant professor of neurology at Vanderbilt who co-authored the whistleblower report independently of his work at the university.
Both Zlokovic and representatives for USC declined to comment, citing an ongoing review initiated in the wake of the allegations, which were first reported in the journal Science.
“USC takes any allegations of research integrity very seriously,” the university said in a statement. “Consistent with federal regulations and USC policies, this review must be kept confidential.”
Science & Medicine
USC neuroscientist faces scrutiny following allegations of data manipulation
Accusations against USC’s Berislav Zlokovic were made by a small group of independent researchers and reported in the journal Science.
Nov. 24, 2023
Zlokovic “remains committed to cooperating with and respecting that process, although it is unfortunately required due to allegations that are based on incorrect information and faulty premises,” his attorney Alfredo X. Jarrin wrote in an email.
Regarding the articles, “corrections and retractions are a normal and necessary part of the scientific post-publication process,” Jarrin wrote.
Authors of the whistleblower report and academic integrity experts challenged that assertion.
“If these are honest errors, then the authors should be able to show the actual original data,” said Elisabeth Bik , a microbiologist and scientific integrity consultant who co-wrote the whistleblower report. “It is totally human to make errors, but there are a lot of errors found in these papers. And some of the findings are suggestive of image manipulation.”
Given the staid pace of academic publishing, publishing this many corrections and retractions only a few months after the initial concerns were raised “is, bizarrely, pretty quick,” said Ivan Oransky, co-founder of Retraction Watch .
The whistleblower report submitted to NIH identified allegedly doctored images and data in 35 research papers in which Zlokovic was the sole common author.
“There had been rumblings about things not being reproducible [in Zlokovic’s research] for quite some time,” Schrag said. “The real motivation to speak publicly is that some of his work reached a stage where it was being used to justify clinical trials. And I think that when you have data that may be unreliable as the foundation for that kind of an experiment, the stakes are just so much higher. You’re talking about patients who are often at the most vulnerable medical moment of their life.”
World & Nation
Countries struggle to draft ‘pandemic treaty’ to avoid mistakes made during COVID
After the devastation of the COVID-19 pandemic, the World Health Organization and leaders worldwide vowed to do better next time but are still struggling to finalize a global plan.
May 11, 2024
Over the years, Zlokovic has created several biotech companies aimed at commercializing his scientific work. In 2007, he co-founded ZZ Biotech , which has been working to gain federal approval of 3K3A-APC.
The drug is intended to minimize the bleeding and subsequent brain damage that can occur after an ischemic stroke, in which a blood clot forms in an artery leading to the brain.
In 2022, USC’s Keck School of Medicine received from NIH the first $4 million of a planned $30-million grant to conduct Phase III trials of the experimental stroke treatment on 1,400 people.
In Phase II of the trial, which was published in 2018 and called Rhapsody, six of the 66 patients who received 3K3A-APC died in the first week after their stroke, compared to one person among the 44 patients who got a placebo. Patients who received the drug also tended to report more disability 90 days after their stroke than those who got the placebo. The differences between the two groups were not statistically significant and could have been due to chance, and the death rate for patients in both groups evened out one month after the initial stroke.
“The statements that there is a risk in this trial is false,” said Patrick Lyden, a USC neurologist and stroke expert who was employed by Cedars-Sinai at the time of the trial. Zlokovic worked with Lyden as a co-investigator on the study.
One correction has been issued to the paper describing the Phase II results, fixing an extra line in a data table that shifted some numbers to the wrong columns. “This mistake is mine. It’s not anybody else’s. I didn’t catch it in multiple readings,” Lyden said, adding that he noticed the error and was already working on the correction when the journal contacted him about it.
He disputed that the trial represented any undue risk to patients.
“I believe it’s safe, especially when you consider that the purpose of Rhapsody was to find a dose — the maximum dose — that was tolerated by the patients without risk, and the Rhapsody trial succeeded in doing that. We did not find any dose that was too high to limit proceeding to Phase III. It’s time to proceed with Phase III.”
Schrag stressed that the whistleblowers did not find evidence of manipulated data in the report from the Phase II trial. But given the errors and alleged data manipulation in Zlokovic’s earlier work, he said, it’s appropriate to scrutinize a clinical trial that would administer the product of his research to people in life-threatening situations.
In the Phase II data, “there’s a coherent pattern of [patient] outcomes trending in the wrong direction. There’s a signal in early mortality … there’s a trend toward worse disability numbers” for patients who received the drug instead of a placebo, he said.
None are “conclusive proof of harm,” he said. But “when you’re seeing a red flag or a trend in the clinical trial, I would tend to give that more weight in the setting of serious ethical concerns around the pre-clinical data.”
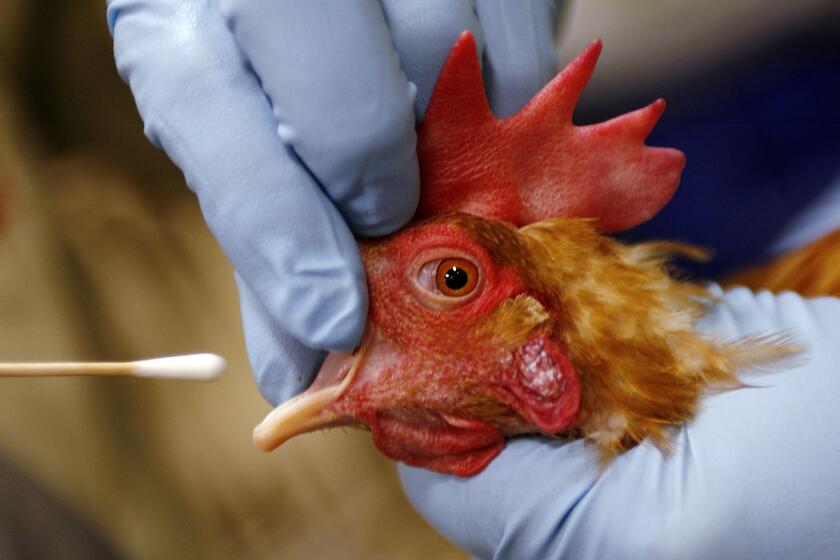
Climate & Environment
What you need to know about the bird flu outbreak, concerns about raw milk, and more
Answering the basics on Bird Flu 2024
May 15, 2024
The NIH paused the clinical trial in November, and it remains on hold, said Dr. Pooja Khatr, principal investigator of the NIH StrokeNet National Coordinating Center. Khatr declined to comment on the pause or the trial’s future, referring further questions to USC and NIH.
The NIH Office of Extramural Research declined to discuss Rhapsody or Zlokovic, citing confidentiality regarding grant deliberations.
ZZ Biotech Chief Executive Kent Pryor, who in 2022 called the drug “a potential game-changer,” said he had no comment or information on the halted trial.
Zlokovic is a leading researcher on the blood-brain barrier, with particular interest in its role in stroke and dementia. He received his medical degree and doctorate in physiology at the University of Belgrade and joined the faculty at USC’s Keck School of Medicine after several fellowships in London. A polyglot and amateur opera singer , Zlokovic left USC and spent 11 years at the University of Rochester before returning in 2011 . He was appointed director of USC’s Zilkha Neurogenetic Institute the following year.
A USC spokesperson confirmed that Zlokovic has retained his titles as department chair and director of the Zilkha institute.
About this article
Corinne Purtill is a science and medicine reporter for the Los Angeles Times. Her writing on science and human behavior has appeared in the New Yorker, the New York Times, Time Magazine, the BBC, Quartz and elsewhere. Before joining The Times, she worked as the senior London correspondent for GlobalPost (now PRI) and as a reporter and assignment editor at the Cambodia Daily in Phnom Penh. She is a native of Southern California and a graduate of Stanford University.
More From the Los Angeles Times
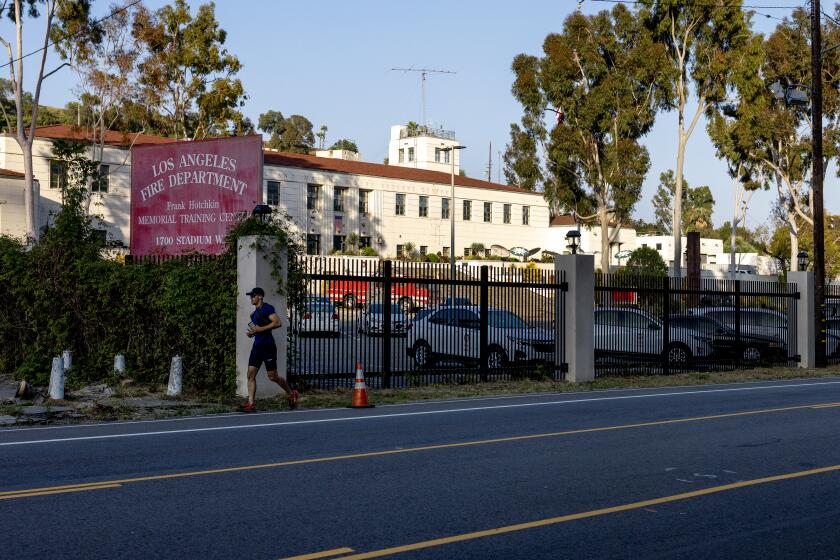
Cloudy with a chance of rage: Climatologists fume over relocation of L.A. weather station
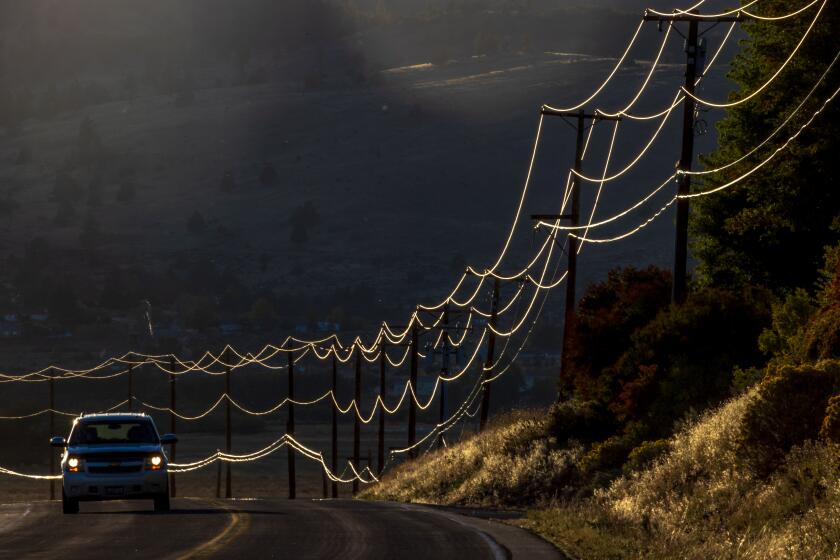
California is changing how big power companies charge for electricity. What to expect on your bill
May 16, 2024
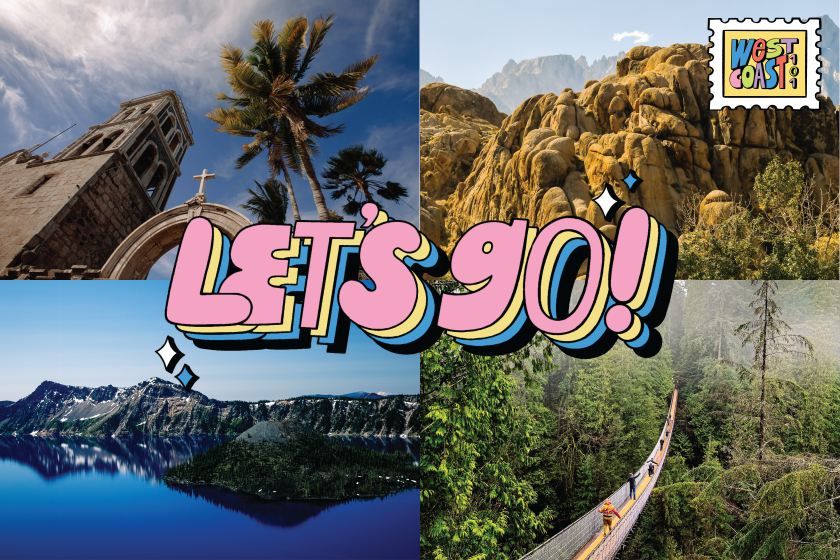
Travel & Experiences
The 101 best West Coast experiences

This anesthesiologist is L.A. County’s highest paid employee. He works 94 hours a week

COMMENTS
Covers all theoretical and applied aspects at the intersection of computer science and game theory, including work in mechanism design, learning in games (which may overlap with Learning), foundations of agent modeling in games (which may overlap with Multiagent systems), coordination, specification and formal methods for non-cooperative computational environments.
arXiv is a free distribution service and an open-access archive for nearly 2.4 million scholarly articles in the fields of physics, mathematics, computer science, quantitative biology, quantitative finance, statistics, electrical engineering and systems science, and economics. Materials on this site are not peer-reviewed by arXiv.
The dblp computer science bibliography is the online reference for open bibliographic information on major computer science journals and proceedings. ... Leibniz Center for Informatics and the consortium NFDIxCS for federal and state funding within the German National Research Data Infrastructure (NFDI). ... The paper DBLP - Some Lessons ...
Get 30 days free. 1. ACM Digital Library. ACM Digital Library is the clear number one when it comes to academic databases for computer science. The ACM Full-Text Collection currently has 540,000+ articles, while the ACM Guide to Computing Literature holds more than 2.8+ million bibliographic entries. Coverage: 2.8+ million articles. Abstracts: .
Digital Science & Research Solutions Ltd: Directory of Open Access Journals (DOAJ) Multidisciplinary: 8,265,735 Articles from 18,652 open access journals (Dec 2022) Free Infrastructure Services for Open Access (IS4OA) DBLP: Computer science: 5,387,041 Comprehensive list of papers from major computer science conferences and journals Free
Organize your papers in one place. Try Paperpile. 1. Scopus. Scopus is one of the two big commercial, bibliographic databases that cover scholarly literature from almost any discipline. Besides searching for research articles, Scopus also provides academic journal rankings, author profiles, and an h-index calculator. 2.
Computer science is the study and development of the protocols required for automated processing and manipulation of data. This includes, for example, creating algorithms for efficiently searching ...
A guide to finding articles and reference materials for students in the field of Computer Science. Use these databases to find articles, papers from conference proceedings, and dissertations and theses ... Computer Science Research Resources: Find Articles & Papers ... conference paper, and report information in all areas of engineering. ...
Several bibliographic databases index computer science papers, allowing users to find information on publications. Bibliographic databases structure information in ways that allow users to retrieve it, by means of keywords, identifiers [for example, the Digital Object Identifier (DOI)], authors' affiliations and links to other records (like references and citation tracking).
Papers We Love (PWL) is a community built around reading, discussing and learning more about academic computer science papers. This repository serves as a directory of some of the best papers the community can find, bringing together documents scattered across the web. You can also visit the Papers We Love site for more info.
Computer Science: Research Databases. This is a guide to UIC library databases and other resources in computer science. Research Databases; Books; Managing Your Data; ... Searches for scholarly materials such as peer-reviewed papers, theses, books, preprints, abstracts and technical reports from broad areas of research. It includes a variety of ...
Open research in computer science. Spanning networks and communications to security and cryptology to big data, complexity, and analytics, SpringerOpen and BMC publish one of the leading open access portfolios in computer science. Learn about our journals and the research we publish here on this page.
An e-print service which presents papers in physics, mathematics, nonlinear science, computer science, quantitative biology, quantitative finance, and statistics. arXiv.org is a fully automated electronic archive and distribution server for research papers which functions as a means of communicating ongoing research information in these subject ...
An on-line, open-access reference for bibliographic information on major computer science publications. DBLP indexes over 6 million publications covering over 1800 journals and more than 6000 conferences and workshops. All important journals on computer science are tracked. DBLP is a joint service of the University of Trier and Schloss Dagstuhl.
The following databases are the primary electronic resources available for locating journal articles in computer science. All databases that UMCP subscribes to can be accessed through Database Finder. ACM Digital Library. Full-text repository of papers from publications that have been published, co-published, or co-marketed by ACM and other ...
Most databases have a link to a help page that will show you what search features are supported. For example, in Web of Science, you can: Show which authors have written the most papers on a topic. Show what years have the most number of papers on a topic. <<
How to search for Harvard dissertations. DASH, Digital Access to Scholarship at Harvard, is the university's central, open-access repository for the scholarly output of faculty and the broader research community at Harvard.Most Ph.D. dissertations submitted from March 2012 forward are available online in DASH.; Check HOLLIS, the Library Catalog, and refine your results by using the Advanced ...
Computer science ( CS ) majors are in high demand and account for a large part of national computer and information technology job market applicants. Employment in this sector is projected to grow 12% between 2018 and 2028, which is faster than the average of all other occupations. Published data are available on traditional non-computer ...
Although databases have been well-defined and thoroughly discussed in the computer science ... Several scholars have even argued for the existence of the "database before the computer," such as the paper ... Renear A, et al. (2013) Foundations of data curation: The pedagogy and practice of 'purposeful work' with research data. ...
Finding research databases for other disciplines. Guides are created for each department on campus and list subject resources for each discipline. Find research in Computer Science, Engineering, Physics, Biology, Business, Psychology, Education, and more. Report a problem.
Welcome to the Computing Research Repository (CoRR) in arXiv. The Computer Science section of arXiv was established in 1998 through a partnership of the Association for Computing Machinery, the Networked Computer Science Technical Reference Library, and arXiv. You can view the subject category descriptions and browse papers from the main CS ...
In the current age of the Fourth Industrial Revolution (4IR or Industry 4.0), the digital world has a wealth of data, such as Internet of Things (IoT) data, cybersecurity data, mobile data, business data, social media data, health data, etc. To intelligently analyze these data and develop the corresponding smart and automated applications, the knowledge of artificial intelligence (AI ...
Faculty and students at Berkeley have repeatedly defined and redefined the broad field of data management, combining deep intellectual impact with the birth of multi-billion dollar industries, including relational databases, RAID storage, scalable Internet search, and big data analytics. Berkeley also gave birth to many of the most widely-used ...
Mamba, an architecture with RNN-like token mixer of state space model (SSM), was recently introduced to address the quadratic complexity of the attention mechanism and subsequently applied to vision tasks. Nevertheless, the performance of Mamba for vision is often underwhelming when compared with convolutional and attention-based models. In this paper, we delve into the essence of Mamba, and ...
Overview - Library Research for Computer Science. This guide will help you get started searching the computer science "literature" for research papers on your topic and finding other resources such as books, journals, government websites, and published theses and dissertations. Be sure to visit the different pages of this guide using the tabs ...
Crystal-specific geochemical and petrological data reveal contrasting depths and timescales for magma accumulation and storage. Pre-eruption storage is consistently interpreted at ~3 to 8 km depth (4-6).Accumulation timescales in these shallow chambers are interpreted to span 10s of a to 10s of ka (6-9).The chambers represent only the shallowest portions of vertically extensive magmatic ...
This paper introduces a dataset developed to measure the impact of archival and data curation decisions on data reuse. The dataset describes 10,605 social science research datasets, their curation ...
This year's Web Conference announced Assistant Professor of Computer Science and Data Science Haifeng Xu as the winner for the conference's Best Paper Award.. The Web Conference is a yearly international conference on AI, Information Retrieval, and Web Technology. Since 1989, the Web Conference has focused on the future direction of the World Wide Web, and serves as a venue to present and ...
QServe: W4A8KV4 Quantization and System Co-design for Efficient LLM Serving. Quantization can accelerate large language model (LLM) inference. Going beyond INT8 quantization, the research community is actively exploring even lower precision, such as INT4. Nonetheless, state-of-the-art INT4 quantization techniques only accelerate low-batch, edge ...
USC neuroscientist faces scrutiny following allegations of data manipulation. Nov. 24, 2023. Zlokovic "remains committed to cooperating with and respecting that process, although it is ...