Have a language expert improve your writing
Run a free plagiarism check in 10 minutes, generate accurate citations for free.
- Knowledge Base
- Research paper

Writing a Research Paper Introduction | Step-by-Step Guide
Published on September 24, 2022 by Jack Caulfield . Revised on March 27, 2023.
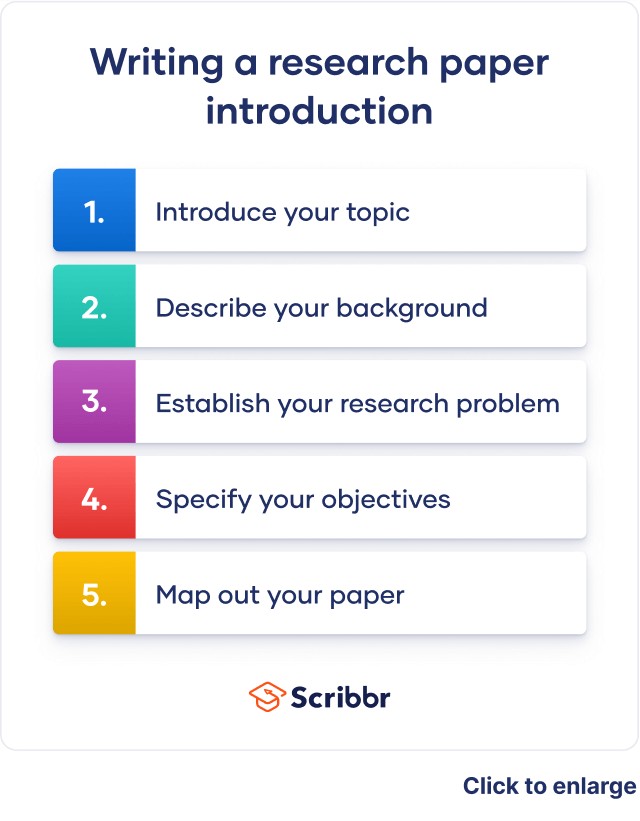
The introduction to a research paper is where you set up your topic and approach for the reader. It has several key goals:
- Present your topic and get the reader interested
- Provide background or summarize existing research
- Position your own approach
- Detail your specific research problem and problem statement
- Give an overview of the paper’s structure
The introduction looks slightly different depending on whether your paper presents the results of original empirical research or constructs an argument by engaging with a variety of sources.
Instantly correct all language mistakes in your text
Upload your document to correct all your mistakes in minutes
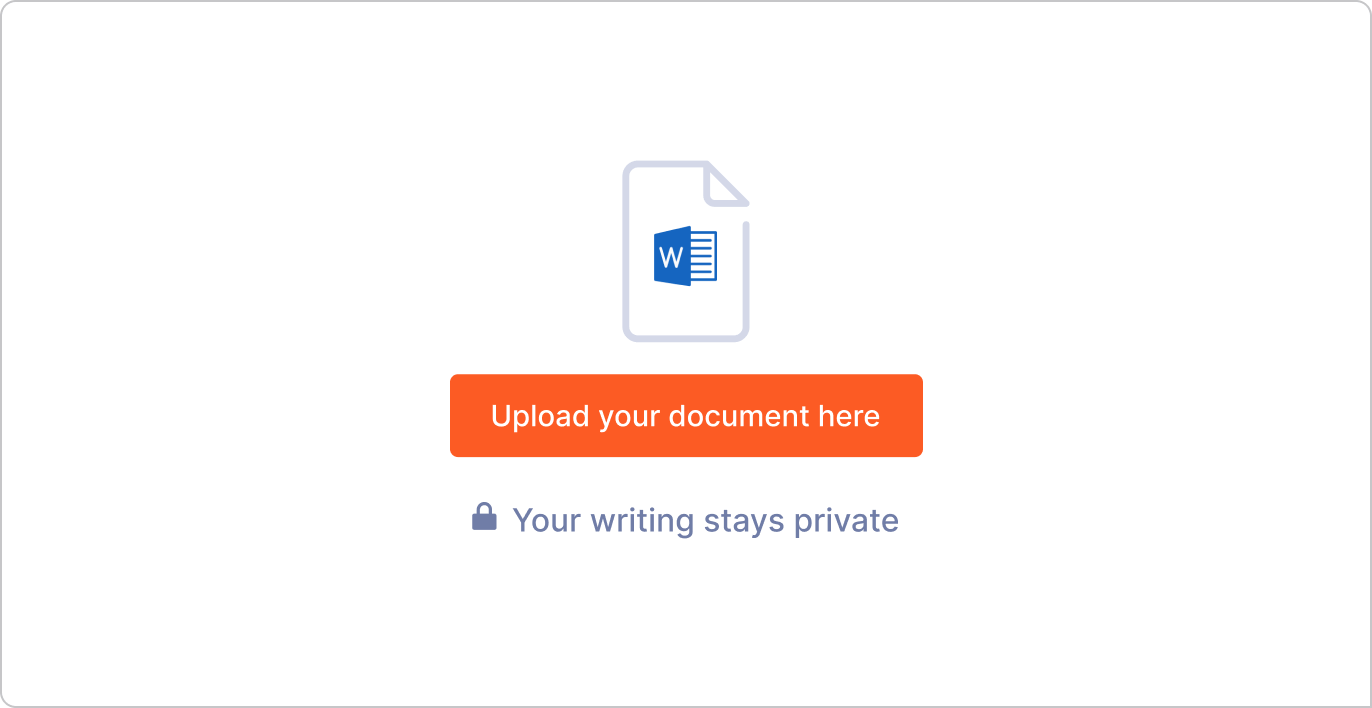
Table of contents
Step 1: introduce your topic, step 2: describe the background, step 3: establish your research problem, step 4: specify your objective(s), step 5: map out your paper, research paper introduction examples, frequently asked questions about the research paper introduction.
The first job of the introduction is to tell the reader what your topic is and why it’s interesting or important. This is generally accomplished with a strong opening hook.
The hook is a striking opening sentence that clearly conveys the relevance of your topic. Think of an interesting fact or statistic, a strong statement, a question, or a brief anecdote that will get the reader wondering about your topic.
For example, the following could be an effective hook for an argumentative paper about the environmental impact of cattle farming:
A more empirical paper investigating the relationship of Instagram use with body image issues in adolescent girls might use the following hook:
Don’t feel that your hook necessarily has to be deeply impressive or creative. Clarity and relevance are still more important than catchiness. The key thing is to guide the reader into your topic and situate your ideas.
The only proofreading tool specialized in correcting academic writing - try for free!
The academic proofreading tool has been trained on 1000s of academic texts and by native English editors. Making it the most accurate and reliable proofreading tool for students.
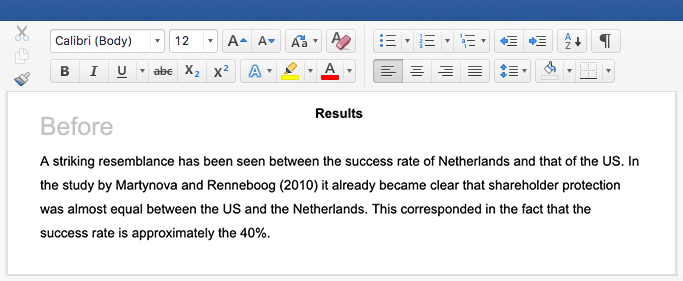
Try for free
This part of the introduction differs depending on what approach your paper is taking.
In a more argumentative paper, you’ll explore some general background here. In a more empirical paper, this is the place to review previous research and establish how yours fits in.
Argumentative paper: Background information
After you’ve caught your reader’s attention, specify a bit more, providing context and narrowing down your topic.
Provide only the most relevant background information. The introduction isn’t the place to get too in-depth; if more background is essential to your paper, it can appear in the body .
Empirical paper: Describing previous research
For a paper describing original research, you’ll instead provide an overview of the most relevant research that has already been conducted. This is a sort of miniature literature review —a sketch of the current state of research into your topic, boiled down to a few sentences.
This should be informed by genuine engagement with the literature. Your search can be less extensive than in a full literature review, but a clear sense of the relevant research is crucial to inform your own work.
Begin by establishing the kinds of research that have been done, and end with limitations or gaps in the research that you intend to respond to.
The next step is to clarify how your own research fits in and what problem it addresses.
Argumentative paper: Emphasize importance
In an argumentative research paper, you can simply state the problem you intend to discuss, and what is original or important about your argument.
Empirical paper: Relate to the literature
In an empirical research paper, try to lead into the problem on the basis of your discussion of the literature. Think in terms of these questions:
- What research gap is your work intended to fill?
- What limitations in previous work does it address?
- What contribution to knowledge does it make?
You can make the connection between your problem and the existing research using phrases like the following.
Now you’ll get into the specifics of what you intend to find out or express in your research paper.
The way you frame your research objectives varies. An argumentative paper presents a thesis statement, while an empirical paper generally poses a research question (sometimes with a hypothesis as to the answer).
Argumentative paper: Thesis statement
The thesis statement expresses the position that the rest of the paper will present evidence and arguments for. It can be presented in one or two sentences, and should state your position clearly and directly, without providing specific arguments for it at this point.
Empirical paper: Research question and hypothesis
The research question is the question you want to answer in an empirical research paper.
Present your research question clearly and directly, with a minimum of discussion at this point. The rest of the paper will be taken up with discussing and investigating this question; here you just need to express it.
A research question can be framed either directly or indirectly.
- This study set out to answer the following question: What effects does daily use of Instagram have on the prevalence of body image issues among adolescent girls?
- We investigated the effects of daily Instagram use on the prevalence of body image issues among adolescent girls.
If your research involved testing hypotheses , these should be stated along with your research question. They are usually presented in the past tense, since the hypothesis will already have been tested by the time you are writing up your paper.
For example, the following hypothesis might respond to the research question above:
Here's why students love Scribbr's proofreading services
Discover proofreading & editing
The final part of the introduction is often dedicated to a brief overview of the rest of the paper.
In a paper structured using the standard scientific “introduction, methods, results, discussion” format, this isn’t always necessary. But if your paper is structured in a less predictable way, it’s important to describe the shape of it for the reader.
If included, the overview should be concise, direct, and written in the present tense.
- This paper will first discuss several examples of survey-based research into adolescent social media use, then will go on to …
- This paper first discusses several examples of survey-based research into adolescent social media use, then goes on to …
Full examples of research paper introductions are shown in the tabs below: one for an argumentative paper, the other for an empirical paper.
- Argumentative paper
- Empirical paper
Are cows responsible for climate change? A recent study (RIVM, 2019) shows that cattle farmers account for two thirds of agricultural nitrogen emissions in the Netherlands. These emissions result from nitrogen in manure, which can degrade into ammonia and enter the atmosphere. The study’s calculations show that agriculture is the main source of nitrogen pollution, accounting for 46% of the country’s total emissions. By comparison, road traffic and households are responsible for 6.1% each, the industrial sector for 1%. While efforts are being made to mitigate these emissions, policymakers are reluctant to reckon with the scale of the problem. The approach presented here is a radical one, but commensurate with the issue. This paper argues that the Dutch government must stimulate and subsidize livestock farmers, especially cattle farmers, to transition to sustainable vegetable farming. It first establishes the inadequacy of current mitigation measures, then discusses the various advantages of the results proposed, and finally addresses potential objections to the plan on economic grounds.
The rise of social media has been accompanied by a sharp increase in the prevalence of body image issues among women and girls. This correlation has received significant academic attention: Various empirical studies have been conducted into Facebook usage among adolescent girls (Tiggermann & Slater, 2013; Meier & Gray, 2014). These studies have consistently found that the visual and interactive aspects of the platform have the greatest influence on body image issues. Despite this, highly visual social media (HVSM) such as Instagram have yet to be robustly researched. This paper sets out to address this research gap. We investigated the effects of daily Instagram use on the prevalence of body image issues among adolescent girls. It was hypothesized that daily Instagram use would be associated with an increase in body image concerns and a decrease in self-esteem ratings.
The introduction of a research paper includes several key elements:
- A hook to catch the reader’s interest
- Relevant background on the topic
- Details of your research problem
and your problem statement
- A thesis statement or research question
- Sometimes an overview of the paper
Don’t feel that you have to write the introduction first. The introduction is often one of the last parts of the research paper you’ll write, along with the conclusion.
This is because it can be easier to introduce your paper once you’ve already written the body ; you may not have the clearest idea of your arguments until you’ve written them, and things can change during the writing process .
The way you present your research problem in your introduction varies depending on the nature of your research paper . A research paper that presents a sustained argument will usually encapsulate this argument in a thesis statement .
A research paper designed to present the results of empirical research tends to present a research question that it seeks to answer. It may also include a hypothesis —a prediction that will be confirmed or disproved by your research.
Cite this Scribbr article
If you want to cite this source, you can copy and paste the citation or click the “Cite this Scribbr article” button to automatically add the citation to our free Citation Generator.
Caulfield, J. (2023, March 27). Writing a Research Paper Introduction | Step-by-Step Guide. Scribbr. Retrieved April 17, 2024, from https://www.scribbr.com/research-paper/research-paper-introduction/
Is this article helpful?
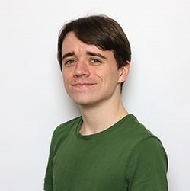
Jack Caulfield
Other students also liked, writing strong research questions | criteria & examples, writing a research paper conclusion | step-by-step guide, research paper format | apa, mla, & chicago templates, "i thought ai proofreading was useless but..".
I've been using Scribbr for years now and I know it's a service that won't disappoint. It does a good job spotting mistakes”
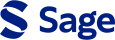
- Request new password
- Create a new account
An Introduction to Qualitative Research
Student resources, part 1 (chapters 1 – 5): foundations of qualitative research.
Graduate Research Hub
- Preparing my thesis
- Incorporating your published work in your thesis
- Examples of thesis and chapter formats when including publications
The following examples are acceptable ways of formatting your thesis and chapters when including one or more publications.
Essential requirements
All theses with publications must have the following:
- Declaration
- Preface – noting collaborations, and contributions to authorship
- Acknowledgements
- Table of contents
- List of tables, figures & illustrations
- Main text/chapters
- Bibliography or list of references
Main text examples
- Chapter 1: Introduction
- Chapter 2: Literature review
- Chapter 3: Methods
- Chapter 4: Paper 1 & general discussion
- Chapter 5: Paper 2
- Chapter 6: Regular thesis chapter – results
- Chapter 7 : Regular thesis chapter/general discussion tying in published and unpublished work
- Chapter 8: Conclusion
- Appendices - May include CD, DVD or other material, also reviews & methods papers
- Chapter 2: Methods
- Chapter 3: Paper 1
- Chapter 4: Regular thesis chapter
- Chapter 6: Regular thesis chapter, final preliminary study
- Chapter 7: General discussion
- Chapter 5: Regular thesis chapter
- Chapter 6: Regular thesis chapter
- Chapter 7: Regular thesis chapter, final preliminary study
- Chapter 8: General discussion
- Chapter 4: Paper 2 - e.g. data paper, including meta analyses
- Chapter 5: Paper 3
- Chapter 6: Paper 4
- Chapter 7: Paper 5
- Chapter 3: Major paper
- Chapter 4: Normal thesis chapter, final preliminary study
- Chapter 5: General discussion
Chapter examples
- Introduction – including specific aims and hypotheses
- Introduction – including specific aims, hypotheses
- Methods – results (including validation, preliminary) not included in the paper
- Results (including validation, preliminary) not included in paper
- Discussion – expansion of paper discussion, further method development
- Resources for candidates
- Orientation and induction
- Mapping my degree
- Principles for infrastructure support
- Peer activities
- Change my commencement date
- Meeting expectations
- Working with my supervisors
- Responsible Research & Research Integrity
- Guidelines for external supervisors
- Pre-confirmation
- Confirmation
- At risk of unsatisfactory progress
- Unsatisfactory progress
- Add or drop coursework subjects
- Apply for leave
- Return from leave
- Apply for Study Away
- Return from Study Away
- Change my study rate
- Check my candidature status
- Change my current supervisors
- Request an evidence of enrolment or evidence of qualification statement
- Change my project details
- Change department
- Transfer to another graduate research degree
- Late submission
- Withdraw from my research degree
- Check the status of a request
- Re-enrolment
- Advice on requesting changes
- Extension of candidature
- Lapse candidature
- How to cancel a form in my.unimelb
- Resolving issues
- Taking leave
- About Study Away
- Finishing on time
- Accepting an offer for a joint PhD online
- Tenured Study Spaces (TSS) Usage Guidelines
- Tenured Study Spaces Procedures
- Research skills
- Academic writing and communication skills
- Building professional and academic networks
- Research internships
- Commercialising my research
- Supplementary PhD Programs
- Writing my thesis
- Thesis with creative works
- Research Integrity in my Thesis
- Graduate researchers and digital assistance tools
- TES Statuses
- Submitting my thesis
- Depositing multiple components for your final thesis record
- The Chancellor's Prize
- TES Graduate Researcher FAQs
- Career planning
- Publishing my research
- Getting support
- Key graduate research contacts
- Melbourne Research Experience Survey
- Quality Indicators for Learning and Teaching (QILT)
- Current Students
- Privacy Policy
Buy Me a Coffee

Home » Research Paper Introduction – Writing Guide and Examples
Research Paper Introduction – Writing Guide and Examples
Table of Contents

Research Paper Introduction
Research paper introduction is the first section of a research paper that provides an overview of the study, its purpose, and the research question (s) or hypothesis (es) being investigated. It typically includes background information about the topic, a review of previous research in the field, and a statement of the research objectives. The introduction is intended to provide the reader with a clear understanding of the research problem, why it is important, and how the study will contribute to existing knowledge in the field. It also sets the tone for the rest of the paper and helps to establish the author’s credibility and expertise on the subject.
How to Write Research Paper Introduction
Writing an introduction for a research paper can be challenging because it sets the tone for the entire paper. Here are some steps to follow to help you write an effective research paper introduction:
- Start with a hook : Begin your introduction with an attention-grabbing statement, a question, or a surprising fact that will make the reader interested in reading further.
- Provide background information: After the hook, provide background information on the topic. This information should give the reader a general idea of what the topic is about and why it is important.
- State the research problem: Clearly state the research problem or question that the paper addresses. This should be done in a concise and straightforward manner.
- State the research objectives: After stating the research problem, clearly state the research objectives. This will give the reader an idea of what the paper aims to achieve.
- Provide a brief overview of the paper: At the end of the introduction, provide a brief overview of the paper. This should include a summary of the main points that will be discussed in the paper.
- Revise and refine: Finally, revise and refine your introduction to ensure that it is clear, concise, and engaging.
Structure of Research Paper Introduction
The following is a typical structure for a research paper introduction:
- Background Information: This section provides an overview of the topic of the research paper, including relevant background information and any previous research that has been done on the topic. It helps to give the reader a sense of the context for the study.
- Problem Statement: This section identifies the specific problem or issue that the research paper is addressing. It should be clear and concise, and it should articulate the gap in knowledge that the study aims to fill.
- Research Question/Hypothesis : This section states the research question or hypothesis that the study aims to answer. It should be specific and focused, and it should clearly connect to the problem statement.
- Significance of the Study: This section explains why the research is important and what the potential implications of the study are. It should highlight the contribution that the research makes to the field.
- Methodology: This section describes the research methods that were used to conduct the study. It should be detailed enough to allow the reader to understand how the study was conducted and to evaluate the validity of the results.
- Organization of the Paper : This section provides a brief overview of the structure of the research paper. It should give the reader a sense of what to expect in each section of the paper.
Research Paper Introduction Examples
Research Paper Introduction Examples could be:
Example 1: In recent years, the use of artificial intelligence (AI) has become increasingly prevalent in various industries, including healthcare. AI algorithms are being developed to assist with medical diagnoses, treatment recommendations, and patient monitoring. However, as the use of AI in healthcare grows, ethical concerns regarding privacy, bias, and accountability have emerged. This paper aims to explore the ethical implications of AI in healthcare and propose recommendations for addressing these concerns.
Example 2: Climate change is one of the most pressing issues facing our planet today. The increasing concentration of greenhouse gases in the atmosphere has resulted in rising temperatures, changing weather patterns, and other environmental impacts. In this paper, we will review the scientific evidence on climate change, discuss the potential consequences of inaction, and propose solutions for mitigating its effects.
Example 3: The rise of social media has transformed the way we communicate and interact with each other. While social media platforms offer many benefits, including increased connectivity and access to information, they also present numerous challenges. In this paper, we will examine the impact of social media on mental health, privacy, and democracy, and propose solutions for addressing these issues.
Example 4: The use of renewable energy sources has become increasingly important in the face of climate change and environmental degradation. While renewable energy technologies offer many benefits, including reduced greenhouse gas emissions and energy independence, they also present numerous challenges. In this paper, we will assess the current state of renewable energy technology, discuss the economic and political barriers to its adoption, and propose solutions for promoting the widespread use of renewable energy.
Purpose of Research Paper Introduction
The introduction section of a research paper serves several important purposes, including:
- Providing context: The introduction should give readers a general understanding of the topic, including its background, significance, and relevance to the field.
- Presenting the research question or problem: The introduction should clearly state the research question or problem that the paper aims to address. This helps readers understand the purpose of the study and what the author hopes to accomplish.
- Reviewing the literature: The introduction should summarize the current state of knowledge on the topic, highlighting the gaps and limitations in existing research. This shows readers why the study is important and necessary.
- Outlining the scope and objectives of the study: The introduction should describe the scope and objectives of the study, including what aspects of the topic will be covered, what data will be collected, and what methods will be used.
- Previewing the main findings and conclusions : The introduction should provide a brief overview of the main findings and conclusions that the study will present. This helps readers anticipate what they can expect to learn from the paper.
When to Write Research Paper Introduction
The introduction of a research paper is typically written after the research has been conducted and the data has been analyzed. This is because the introduction should provide an overview of the research problem, the purpose of the study, and the research questions or hypotheses that will be investigated.
Once you have a clear understanding of the research problem and the questions that you want to explore, you can begin to write the introduction. It’s important to keep in mind that the introduction should be written in a way that engages the reader and provides a clear rationale for the study. It should also provide context for the research by reviewing relevant literature and explaining how the study fits into the larger field of research.
Advantages of Research Paper Introduction
The introduction of a research paper has several advantages, including:
- Establishing the purpose of the research: The introduction provides an overview of the research problem, question, or hypothesis, and the objectives of the study. This helps to clarify the purpose of the research and provide a roadmap for the reader to follow.
- Providing background information: The introduction also provides background information on the topic, including a review of relevant literature and research. This helps the reader understand the context of the study and how it fits into the broader field of research.
- Demonstrating the significance of the research: The introduction also explains why the research is important and relevant. This helps the reader understand the value of the study and why it is worth reading.
- Setting expectations: The introduction sets the tone for the rest of the paper and prepares the reader for what is to come. This helps the reader understand what to expect and how to approach the paper.
- Grabbing the reader’s attention: A well-written introduction can grab the reader’s attention and make them interested in reading further. This is important because it can help to keep the reader engaged and motivated to read the rest of the paper.
- Creating a strong first impression: The introduction is the first part of the research paper that the reader will see, and it can create a strong first impression. A well-written introduction can make the reader more likely to take the research seriously and view it as credible.
- Establishing the author’s credibility: The introduction can also establish the author’s credibility as a researcher. By providing a clear and thorough overview of the research problem and relevant literature, the author can demonstrate their expertise and knowledge in the field.
- Providing a structure for the paper: The introduction can also provide a structure for the rest of the paper. By outlining the main sections and sub-sections of the paper, the introduction can help the reader navigate the paper and find the information they are looking for.
About the author
Muhammad Hassan
Researcher, Academic Writer, Web developer
You may also like

How to Cite Research Paper – All Formats and...

Delimitations in Research – Types, Examples and...

Research Paper Format – Types, Examples and...

Research Design – Types, Methods and Examples

Research Paper Title – Writing Guide and Example

Research Paper Conclusion – Writing Guide and...
- U.S. Locations
- UMGC Europe
- Learn Online
- Find Answers
- 855-655-8682
- Current Students
Online Guide to Writing and Research
The research process, explore more of umgc.
- Online Guide to Writing
Structuring the Research Paper
Formal research structure.
These are the primary purposes for formal research:
enter the discourse, or conversation, of other writers and scholars in your field
learn how others in your field use primary and secondary resources
find and understand raw data and information
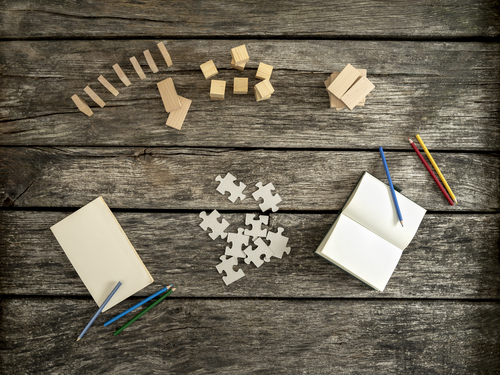
For the formal academic research assignment, consider an organizational pattern typically used for primary academic research. The pattern includes the following: introduction, methods, results, discussion, and conclusions/recommendations.
Usually, research papers flow from the general to the specific and back to the general in their organization. The introduction uses a general-to-specific movement in its organization, establishing the thesis and setting the context for the conversation. The methods and results sections are more detailed and specific, providing support for the generalizations made in the introduction. The discussion section moves toward an increasingly more general discussion of the subject, leading to the conclusions and recommendations, which then generalize the conversation again.
Sections of a Formal Structure
The introduction section.
Many students will find that writing a structured introduction gets them started and gives them the focus needed to significantly improve their entire paper.
Introductions usually have three parts:
presentation of the problem statement, the topic, or the research inquiry
purpose and focus of your paper
summary or overview of the writer’s position or arguments
In the first part of the introduction—the presentation of the problem or the research inquiry—state the problem or express it so that the question is implied. Then, sketch the background on the problem and review the literature on it to give your readers a context that shows them how your research inquiry fits into the conversation currently ongoing in your subject area.
In the second part of the introduction, state your purpose and focus. Here, you may even present your actual thesis. Sometimes your purpose statement can take the place of the thesis by letting your reader know your intentions.
The third part of the introduction, the summary or overview of the paper, briefly leads readers through the discussion, forecasting the main ideas and giving readers a blueprint for the paper.
The following example provides a blueprint for a well-organized introduction.
Example of an Introduction
Entrepreneurial Marketing: The Critical Difference
In an article in the Harvard Business Review, John A. Welsh and Jerry F. White remind us that “a small business is not a little big business.” An entrepreneur is not a multinational conglomerate but a profit-seeking individual. To survive, he must have a different outlook and must apply different principles to his endeavors than does the president of a large or even medium-sized corporation. Not only does the scale of small and big businesses differ, but small businesses also suffer from what the Harvard Business Review article calls “resource poverty.” This is a problem and opportunity that requires an entirely different approach to marketing. Where large ad budgets are not necessary or feasible, where expensive ad production squanders limited capital, where every marketing dollar must do the work of two dollars, if not five dollars or even ten, where a person’s company, capital, and material well-being are all on the line—that is, where guerrilla marketing can save the day and secure the bottom line (Levinson, 1984, p. 9).
By reviewing the introductions to research articles in the discipline in which you are writing your research paper, you can get an idea of what is considered the norm for that discipline. Study several of these before you begin your paper so that you know what may be expected. If you are unsure of the kind of introduction your paper needs, ask your professor for more information. The introduction is normally written in present tense.
THE METHODS SECTION
The methods section of your research paper should describe in detail what methodology and special materials if any, you used to think through or perform your research. You should include any materials you used or designed for yourself, such as questionnaires or interview questions, to generate data or information for your research paper. You want to include any methodologies that are specific to your particular field of study, such as lab procedures for a lab experiment or data-gathering instruments for field research. The methods section is usually written in the past tense.
THE RESULTS SECTION
How you present the results of your research depends on what kind of research you did, your subject matter, and your readers’ expectations.
Quantitative information —data that can be measured—can be presented systematically and economically in tables, charts, and graphs. Quantitative information includes quantities and comparisons of sets of data.
Qualitative information , which includes brief descriptions, explanations, or instructions, can also be presented in prose tables. This kind of descriptive or explanatory information, however, is often presented in essay-like prose or even lists.
There are specific conventions for creating tables, charts, and graphs and organizing the information they contain. In general, you should use them only when you are sure they will enlighten your readers rather than confuse them. In the accompanying explanation and discussion, always refer to the graphic by number and explain specifically what you are referring to; you can also provide a caption for the graphic. The rule of thumb for presenting a graphic is first to introduce it by name, show it, and then interpret it. The results section is usually written in the past tense.
THE DISCUSSION SECTION
Your discussion section should generalize what you have learned from your research. One way to generalize is to explain the consequences or meaning of your results and then make your points that support and refer back to the statements you made in your introduction. Your discussion should be organized so that it relates directly to your thesis. You want to avoid introducing new ideas here or discussing tangential issues not directly related to the exploration and discovery of your thesis. The discussion section, along with the introduction, is usually written in the present tense.
THE CONCLUSIONS AND RECOMMENDATIONS SECTION
Your conclusion ties your research to your thesis, binding together all the main ideas in your thinking and writing. By presenting the logical outcome of your research and thinking, your conclusion answers your research inquiry for your reader. Your conclusions should relate directly to the ideas presented in your introduction section and should not present any new ideas.
You may be asked to present your recommendations separately in your research assignment. If so, you will want to add some elements to your conclusion section. For example, you may be asked to recommend a course of action, make a prediction, propose a solution to a problem, offer a judgment, or speculate on the implications and consequences of your ideas. The conclusions and recommendations section is usually written in the present tense.
Key Takeaways
- For the formal academic research assignment, consider an organizational pattern typically used for primary academic research.
- The pattern includes the following: introduction, methods, results, discussion, and conclusions/recommendations.
Mailing Address: 3501 University Blvd. East, Adelphi, MD 20783 This work is licensed under a Creative Commons Attribution-NonCommercial-ShareAlike 4.0 International License . © 2022 UMGC. All links to external sites were verified at the time of publication. UMGC is not responsible for the validity or integrity of information located at external sites.
Table of Contents: Online Guide to Writing
Chapter 1: College Writing
How Does College Writing Differ from Workplace Writing?
What Is College Writing?
Why So Much Emphasis on Writing?
Chapter 2: The Writing Process
Doing Exploratory Research
Getting from Notes to Your Draft
Introduction
Prewriting - Techniques to Get Started - Mining Your Intuition
Prewriting: Targeting Your Audience
Prewriting: Techniques to Get Started
Prewriting: Understanding Your Assignment
Rewriting: Being Your Own Critic
Rewriting: Creating a Revision Strategy
Rewriting: Getting Feedback
Rewriting: The Final Draft
Techniques to Get Started - Outlining
Techniques to Get Started - Using Systematic Techniques
Thesis Statement and Controlling Idea
Writing: Getting from Notes to Your Draft - Freewriting
Writing: Getting from Notes to Your Draft - Summarizing Your Ideas
Writing: Outlining What You Will Write
Chapter 3: Thinking Strategies
A Word About Style, Voice, and Tone
A Word About Style, Voice, and Tone: Style Through Vocabulary and Diction
Critical Strategies and Writing
Critical Strategies and Writing: Analysis
Critical Strategies and Writing: Evaluation
Critical Strategies and Writing: Persuasion
Critical Strategies and Writing: Synthesis
Developing a Paper Using Strategies
Kinds of Assignments You Will Write
Patterns for Presenting Information
Patterns for Presenting Information: Critiques
Patterns for Presenting Information: Discussing Raw Data
Patterns for Presenting Information: General-to-Specific Pattern
Patterns for Presenting Information: Problem-Cause-Solution Pattern
Patterns for Presenting Information: Specific-to-General Pattern
Patterns for Presenting Information: Summaries and Abstracts
Supporting with Research and Examples
Writing Essay Examinations
Writing Essay Examinations: Make Your Answer Relevant and Complete
Writing Essay Examinations: Organize Thinking Before Writing
Writing Essay Examinations: Read and Understand the Question
Chapter 4: The Research Process
Planning and Writing a Research Paper
Planning and Writing a Research Paper: Ask a Research Question
Planning and Writing a Research Paper: Cite Sources
Planning and Writing a Research Paper: Collect Evidence
Planning and Writing a Research Paper: Decide Your Point of View, or Role, for Your Research
Planning and Writing a Research Paper: Draw Conclusions
Planning and Writing a Research Paper: Find a Topic and Get an Overview
Planning and Writing a Research Paper: Manage Your Resources
Planning and Writing a Research Paper: Outline
Planning and Writing a Research Paper: Survey the Literature
Planning and Writing a Research Paper: Work Your Sources into Your Research Writing
Research Resources: Where Are Research Resources Found? - Human Resources
Research Resources: What Are Research Resources?
Research Resources: Where Are Research Resources Found?
Research Resources: Where Are Research Resources Found? - Electronic Resources
Research Resources: Where Are Research Resources Found? - Print Resources
Structuring the Research Paper: Formal Research Structure
Structuring the Research Paper: Informal Research Structure
The Nature of Research
The Research Assignment: How Should Research Sources Be Evaluated?
The Research Assignment: When Is Research Needed?
The Research Assignment: Why Perform Research?
Chapter 5: Academic Integrity
Academic Integrity
Giving Credit to Sources
Giving Credit to Sources: Copyright Laws
Giving Credit to Sources: Documentation
Giving Credit to Sources: Style Guides
Integrating Sources
Practicing Academic Integrity
Practicing Academic Integrity: Keeping Accurate Records
Practicing Academic Integrity: Managing Source Material
Practicing Academic Integrity: Managing Source Material - Paraphrasing Your Source
Practicing Academic Integrity: Managing Source Material - Quoting Your Source
Practicing Academic Integrity: Managing Source Material - Summarizing Your Sources
Types of Documentation
Types of Documentation: Bibliographies and Source Lists
Types of Documentation: Citing World Wide Web Sources
Types of Documentation: In-Text or Parenthetical Citations
Types of Documentation: In-Text or Parenthetical Citations - APA Style
Types of Documentation: In-Text or Parenthetical Citations - CSE/CBE Style
Types of Documentation: In-Text or Parenthetical Citations - Chicago Style
Types of Documentation: In-Text or Parenthetical Citations - MLA Style
Types of Documentation: Note Citations
Chapter 6: Using Library Resources
Finding Library Resources
Chapter 7: Assessing Your Writing
How Is Writing Graded?
How Is Writing Graded?: A General Assessment Tool
The Draft Stage
The Draft Stage: The First Draft
The Draft Stage: The Revision Process and the Final Draft
The Draft Stage: Using Feedback
The Research Stage
Using Assessment to Improve Your Writing
Chapter 8: Other Frequently Assigned Papers
Reviews and Reaction Papers: Article and Book Reviews
Reviews and Reaction Papers: Reaction Papers
Writing Arguments
Writing Arguments: Adapting the Argument Structure
Writing Arguments: Purposes of Argument
Writing Arguments: References to Consult for Writing Arguments
Writing Arguments: Steps to Writing an Argument - Anticipate Active Opposition
Writing Arguments: Steps to Writing an Argument - Determine Your Organization
Writing Arguments: Steps to Writing an Argument - Develop Your Argument
Writing Arguments: Steps to Writing an Argument - Introduce Your Argument
Writing Arguments: Steps to Writing an Argument - State Your Thesis or Proposition
Writing Arguments: Steps to Writing an Argument - Write Your Conclusion
Writing Arguments: Types of Argument
Appendix A: Books to Help Improve Your Writing
Dictionaries
General Style Manuals
Researching on the Internet
Special Style Manuals
Writing Handbooks
Appendix B: Collaborative Writing and Peer Reviewing
Collaborative Writing: Assignments to Accompany the Group Project
Collaborative Writing: Informal Progress Report
Collaborative Writing: Issues to Resolve
Collaborative Writing: Methodology
Collaborative Writing: Peer Evaluation
Collaborative Writing: Tasks of Collaborative Writing Group Members
Collaborative Writing: Writing Plan
General Introduction
Peer Reviewing
Appendix C: Developing an Improvement Plan
Working with Your Instructor’s Comments and Grades
Appendix D: Writing Plan and Project Schedule
Devising a Writing Project Plan and Schedule
Reviewing Your Plan with Others
By using our website you agree to our use of cookies. Learn more about how we use cookies by reading our Privacy Policy .
- 66 Ogoja Road, Abakaliki, Ebonyi State 23480 NG.
- Sun - Fri 24Hours Saturday CLOSED
- support [@] writersking.com
- +23480-6075-5653 Hot Line
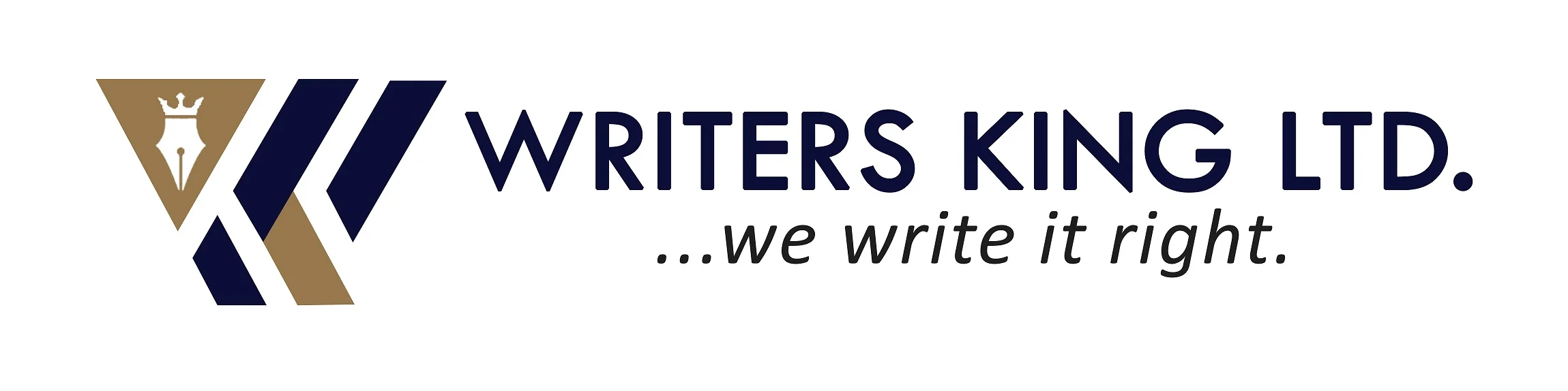
- Data Collection/Analysis
- Hire Proposal Writers
- Hire Essay Writers
- Hire Paper Writers
- Proofreading Services
- Thesis/Dissertation Writers
- Virtual Supervisor
- Turnitin Checker
- Book Chapter Writer
- Hire Business Writing Services
- Hire Blog Writers
- Writers King TV
- Proposal Sample
- Chapter 1-3 Sample
- Term Paper Sample
- Report Assignment Sample
- Course work Sample
- Payment Options
- Privacy Policy
- Terms of Service/Use
- Business Guide
- Academic Writing Guide
- General Writing Guide
- Research News
- Writing Paper Samples
Introducing Research project chapters – How to write chapters 1, 2, 3, 4 and 5 introductions in a thesis and dissertation
- October 28, 2022
- Posted by: IGBAJI U.C.
- Category: Academic Writing Guide
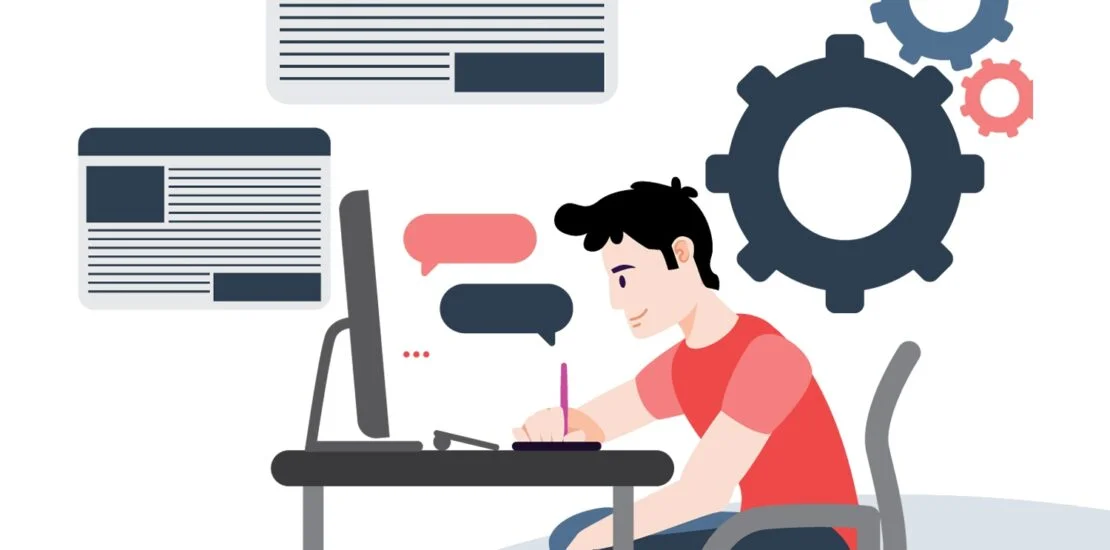
Every research project, thesis or dissertation is organised in chapters. Research project chapters range from 1, 2, 3, 4 and 5, 6 or 7 depending on the school, department and study level.
Content Outline
An introduction is the first section of a research project , an essay, or a book. It is a section that set the tone for the entire project as it gives a reader an insight into the essence of the project. Every research paper requires context, which is the foundation on which the research is based so that readers can comprehend why it was created.
Thus, it introduces the reader to what the research is about. A research project generically consists of five chapters. Thus, here you will discover how to compose an introduction of the various chapters that make up a research project.
A chapter is a separate section of a research report or thesis that must be read as such. Chapter introductions serve a similar orienting purpose as they expose the reader to the chapter’s foci, goals, technique, and argument, as well as any other pertinent reader information.
You will need to compose an introduction chapter while writing a thesis . The introduction of every research thesis or dissertation is crucial since it is the first part the supervisor or examiner will read, thus making a strong first impression is crucial.
How to write chapters 1, 2, 3, 4 and 5 introductions in a thesis and dissertation
Introducing chapter one (1) research project, thesis or dissertation.
Chapter one is the first chapter of a research project . It is often titled “Introduction” because it introduces the entire project and the scope of the project. In addition to providing a foundation for other chapters, it provides a framework for their construction.
When writing the introduction of your research project, you should ensure giving a broad overview of the topic you are writing on and then narrow it down to a particular context or aspects of the topic that your research will focus on.
You should also make effort at providing a brief clarification of the key terms of the project while eliciting an understanding of why your research is worthwhile. However, the following tips can help you introduce your chapter appropriately:
- Provide background information on the title of your project
- Make reference to vital findings of previous studies
- Specify your study objectives and questions
- State the rationale for your study
- State the scope of your study
Although the introduction of your chapter does not have a word limit unless specified, it must be written in a clear and concise manner. However, it is quite tricky writing the introduction of your project, thus, it is mostly recommended that you write your introduction last to ensure that all the necessary information is captured.
Introducing Chapter Two (2) Research Project, Thesis or Dissertation
Chapter two of a research project is often tagged literature review”. The chapter basically provides a review of scholarly studies related to the topic you are researching on. The essence of this chapter is to identify a gap in the literature on your topic of research .
However, introducing this chapter in research projects has not been a common practice among scholars. But this does not take away the necessity of doing so. Generically, chapter two of a research project contains three major sections. These are conceptual literature, theoretical literature, and empirical literature. Thus, when introducing this chapter, is necessary you highlight the various sections as contained in the main body of the review.
Chapter two (2) Introduction Sample:
This is the second chapter of this study. The goal of this chapter is to provide of review of conceptual, theoretical, and empirical literature in the topic area in order to identify gaps in the existing literature. The chapter concludes by providing a summary of the literature review and with a clear statement of the study gap and how the present study intends to occupy the gap .
Introducing Chapter three (3) Research Project, Thesis or Dissertation
The Third chapter of a research project, thesis, or dissertation is often tagged research methodology . It is a chapter where a researcher offers a description of the various methods he intends to adopt in ensuring that the research questions are addressed and the research objectives met.
Although there is no generic way of writing this chapter, there are some sections which are very important to include in your research methodology. These are sections that describe your research design, the population of the study, your sample and sample selection technique, the method of data collection , and the method of data analysis .
Thus, when introducing this chapter you have to give the reader a rundown of the content of the chapter and why it is necessary. A typical example of how to do this is presented below.
Chapter three (3) Introduction Sample:
This is the third chapter of this study. It contains a description of the different methods adopted by the researcher in order to address the research question and achieve the research objectives. The chapter provides a detailed description of the research design, research population, sample and sampling technique, method of data collection, and method of data analysis.
By doing this, a reader will be able to know what the chapter is all about and the scope of the chapter.
Introducing Chapter Four (4) Research Project, Thesis or Dissertation
This is the fourth chapter of a research project. Although the title of this chapter varies greatly, is a chapter that is generically used to present the study analysis. Thus, it is often tagged as “data presentation, analysis, and discussions” or “analysis and result”.
Whichever way, the goal of this chapter is to present and discuss the result of the various analyses carried out in the study. Thus, when introducing this chapter you should try to ensure that you tell the reader what the chapter is meant for.
Chapter four (4) Introduction Sample:
This is the fourth chapter of this study. The chapter presents the results and findings of the various methods of analysis adopted in ensuring that the study data are properly analyzed in order to ensure that the objectives of the study are achieved. The presentation of the study findings is done according to the study objectives stated in the first chapter of the study. The chapter also presents a discussion of the study findings in relation to similar past studies in the topic area.
By doing this you have the research and insight into the content of the chapter. Thus, whetting his/her appetite to delve in fully to see what you have done and how they have been able to address the research question and objectives.
Introducing Chapter Five (5) Research Project, Thesis or Dissertation
This is the final chapter of your research project. It is often tagged “summary of findings or study, conclusion and recommendation”. This is the chapter where you summarize your study by giving s rundown of what you did in prior chapters.
Based on this, you draw the conclusion (s) regarding what you discovered in the study and then make recommendations. Thus, when introducing this chapter you should try and ensure that you give the reader an insight into what the chapter is all about.
Chapter five (5) Introduction Sample
This is the final chapter of the study. The chapter provides a summary of the study, a conclusion, and a recommendation based on the study findings presented in the fourth chapter of the study.
However, while summarizing the study, you have to clearly restate the title of your research, your objective, the method you adopted, and your findings. Also, your conclusion and recommendation should be strictly based on your study findings.
Things to note while introducing chapters in a Research Project, thesis and dissertation
Capture the reader’s interest -introducing research project chapters.
When writing a chapter opening, you must first grab the reader’s interest with a discussion of a larger subject related to your study. Use research, statistics, and quotations from worldwide or national professional groups, governmental organizations, or prominent writers on the study’s issue to enhance impact.
The employment of a hook is another approach to pique the reader’s attention. A hook is a sentence or combination of lines that grab the reader’s attention and piques their interest in the essay’s substance. A fascinating hook may be used in any type of writing.
Furthermore, there are a number of techniques to pique a reader’s interest, ranging from making a bold, aggressive declaration to offering a provocative inquiry.
Give an overview of your research topic -Introducing Research project chapters
Your talk should then begin by delving further into the issue’s larger features before focusing on your research’s specific topic. When doing this, it’s a good idea to pretend the reader has no prior knowledge of the subject. As a result, terminology must be defined and explained, based on significant studies.
Alternatively, if you are dissatisfied with current definitions after reading relevant material for the literature review chapter, draw on these to create your own (but make sure this has been done).
Detail how your research is going to make a contribution -Introducing Research project chapters
You must sell your study subject suggestion by outlining the major reasons why the research will contribute significantly to the present body of knowledge. This may be done by presenting a gap or restriction in existing research and then illustrating how your study will fill that gap or constraint.
Explain what your interest is in the topic -Introducing Research project chapters
After that, you must explain why you choose the issue for yourself. These might be related to prior studies, employment, or experiences. Make a list of the broad research questions and issues that pique your attention.
Make a list of your passions that you may use as a starting point. Following that, you should be able to summarize your interests in a sentence, or at most a paragraph. What contribution will your study make to the field?
List your research objectives -Introducing Research project chapters
In each chapter of your academic writing , you must state the goal you want to attain. What do you hope to accomplish at the conclusion of each chapter? This will let readers get a head start on the chapter’s topic. The precise definition of each chapter’s aims and objectives is one of the most crucial components of a thesis, dissertation, or research paper .
This is because the breadth, depth, and direction of the chapter will ultimately be determined by your goals and objectives. With your aims stating what is to be accomplished and your objectives suggesting how it will be accomplished, a successful set of aims and objectives will provide your study emphasis and clarity to your reader.
Give a forthcoming chapter overview -Introducing Research project chapters
The introduction concludes with a summary of the remaining chapters of the thesis. The remaining sections can be placed in any order as long as they are in a logical order. Discuss the other chapters briefly. Make your writing enjoyable to read.
Make connections between the current chapter and the next chapters you will be working on. This will give the readers a foreknowledge of what your research intends to achieve.
Chapter writing discusses many sorts of hooks and how the writer should choose the one that best meets the paper’s aim. The chapter illustrates how a background section may be a beneficial supplement to the introduction, but it also warns that its content, focus, and length are all dependent on the writer’s assessment of its contribution to the paper’s persuasiveness.
It illustrates how the Reader’s Introduction should have all of the parts in the Writer’s Introduction, as well as a hook and a background section. The chapter discusses many sorts of hooks and how the writer should choose the one that best meets the paper’s aim.
Conclusion -Introducing Research project chapters
Introducing the various chapters of a research project is similar to the generic way of writing an introduction because they all perform the same function which is to give a reader an insight into what the chapter is all about. Although, in some cases, the content structure of research projects of most institutions or as prescribed by the project supervisor may or not permit the inclusion of an introduction of each chapter of the project.
But when not clearly specified by the content structure or project guideline you can make effort at introducing each chapter of your research project as you proceed. Also, try ensuring that you introduce each chapter in a precise and brief manner as a long or bulky introduction may discourage readers from reading your project.
Drop your comment, question or suggestion for the post improvement Cancel reply
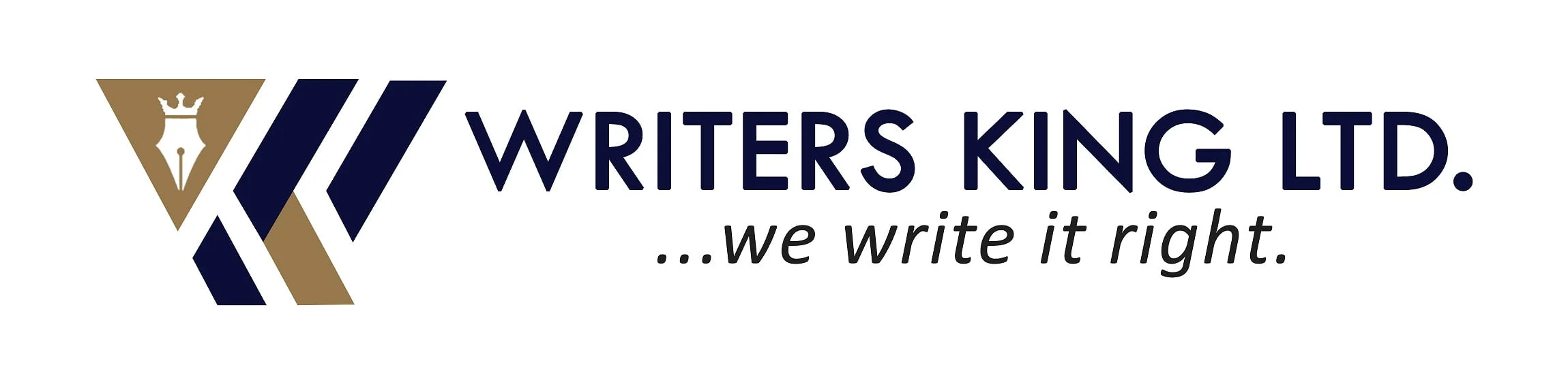
Find Us Today
Writers King LTD, Akachukwu Plaza,
66 Ogoja Road Abakaliki, Ebonyi State,
480101 Nigeria
Phone: 0806-075-5653
- Website: https://writersking.com/
- Email: support {@} writersking.com
- +2348060755653
Quick Links
Writing guide.

What’s Included: Research Paper Template
If you’re preparing to write an academic research paper, our free research paper template is the perfect starting point. In the template, we cover every section step by step, with clear, straightforward explanations and examples .
The template’s structure is based on the tried and trusted best-practice format for formal academic research papers. The template structure reflects the overall research process, ensuring your paper will have a smooth, logical flow from chapter to chapter.
The research paper template covers the following core sections:
- The title page/cover page
- Abstract (sometimes also called the executive summary)
- Section 1: Introduction
- Section 2: Literature review
- Section 3: Methodology
- Section 4: Findings /results
- Section 5: Discussion
- Section 6: Conclusion
- Reference list
Each section is explained in plain, straightforward language , followed by an overview of the key elements that you need to cover within each section. We’ve also included links to free resources to help you understand how to write each section.
The cleanly formatted Google Doc can be downloaded as a fully editable MS Word Document (DOCX format), so you can use it as-is or convert it to LaTeX.
FAQs: Research Paper Template
What format is the template (doc, pdf, ppt, etc.).
The research paper template is provided as a Google Doc. You can download it in MS Word format or make a copy to your Google Drive. You’re also welcome to convert it to whatever format works best for you, such as LaTeX or PDF.
What types of research papers can this template be used for?
The template follows the standard best-practice structure for formal academic research papers, so it is suitable for the vast majority of degrees, particularly those within the sciences.
Some universities may have some additional requirements, but these are typically minor, with the core structure remaining the same. Therefore, it’s always a good idea to double-check your university’s requirements before you finalise your structure.
Is this template for an undergrad, Masters or PhD-level research paper?
This template can be used for a research paper at any level of study. It may be slight overkill for an undergraduate-level study, but it certainly won’t be missing anything.
How long should my research paper be?
This depends entirely on your university’s specific requirements, so it’s best to check with them. We include generic word count ranges for each section within the template, but these are purely indicative.
What about the research proposal?
If you’re still working on your research proposal, we’ve got a template for that here .
We’ve also got loads of proposal-related guides and videos over on the Grad Coach blog .
How do I write a literature review?
We have a wealth of free resources on the Grad Coach Blog that unpack how to write a literature review from scratch. You can check out the literature review section of the blog here.
How do I create a research methodology?
We have a wealth of free resources on the Grad Coach Blog that unpack research methodology, both qualitative and quantitative. You can check out the methodology section of the blog here.
Can I share this research paper template with my friends/colleagues?
Yes, you’re welcome to share this template. If you want to post about it on your blog or social media, all we ask is that you reference this page as your source.
Can Grad Coach help me with my research paper?
Within the template, you’ll find plain-language explanations of each section, which should give you a fair amount of guidance. However, you’re also welcome to consider our private coaching services .
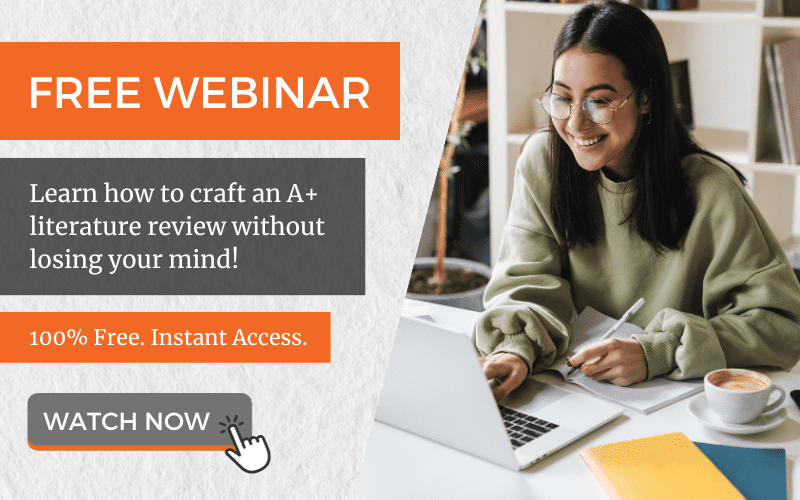

Want to create or adapt books like this? Learn more about how Pressbooks supports open publishing practices.
13.1 Formatting a Research Paper
Learning objectives.
- Identify the major components of a research paper written using American Psychological Association (APA) style.
- Apply general APA style and formatting conventions in a research paper.
In this chapter, you will learn how to use APA style , the documentation and formatting style followed by the American Psychological Association, as well as MLA style , from the Modern Language Association. There are a few major formatting styles used in academic texts, including AMA, Chicago, and Turabian:
- AMA (American Medical Association) for medicine, health, and biological sciences
- APA (American Psychological Association) for education, psychology, and the social sciences
- Chicago—a common style used in everyday publications like magazines, newspapers, and books
- MLA (Modern Language Association) for English, literature, arts, and humanities
- Turabian—another common style designed for its universal application across all subjects and disciplines
While all the formatting and citation styles have their own use and applications, in this chapter we focus our attention on the two styles you are most likely to use in your academic studies: APA and MLA.
If you find that the rules of proper source documentation are difficult to keep straight, you are not alone. Writing a good research paper is, in and of itself, a major intellectual challenge. Having to follow detailed citation and formatting guidelines as well may seem like just one more task to add to an already-too-long list of requirements.
Following these guidelines, however, serves several important purposes. First, it signals to your readers that your paper should be taken seriously as a student’s contribution to a given academic or professional field; it is the literary equivalent of wearing a tailored suit to a job interview. Second, it shows that you respect other people’s work enough to give them proper credit for it. Finally, it helps your reader find additional materials if he or she wishes to learn more about your topic.
Furthermore, producing a letter-perfect APA-style paper need not be burdensome. Yes, it requires careful attention to detail. However, you can simplify the process if you keep these broad guidelines in mind:
- Work ahead whenever you can. Chapter 11 “Writing from Research: What Will I Learn?” includes tips for keeping track of your sources early in the research process, which will save time later on.
- Get it right the first time. Apply APA guidelines as you write, so you will not have much to correct during the editing stage. Again, putting in a little extra time early on can save time later.
- Use the resources available to you. In addition to the guidelines provided in this chapter, you may wish to consult the APA website at http://www.apa.org or the Purdue University Online Writing lab at http://owl.english.purdue.edu , which regularly updates its online style guidelines.
General Formatting Guidelines
This chapter provides detailed guidelines for using the citation and formatting conventions developed by the American Psychological Association, or APA. Writers in disciplines as diverse as astrophysics, biology, psychology, and education follow APA style. The major components of a paper written in APA style are listed in the following box.
These are the major components of an APA-style paper:
Body, which includes the following:
- Headings and, if necessary, subheadings to organize the content
- In-text citations of research sources
- References page
All these components must be saved in one document, not as separate documents.
The title page of your paper includes the following information:
- Title of the paper
- Author’s name
- Name of the institution with which the author is affiliated
- Header at the top of the page with the paper title (in capital letters) and the page number (If the title is lengthy, you may use a shortened form of it in the header.)
List the first three elements in the order given in the previous list, centered about one third of the way down from the top of the page. Use the headers and footers tool of your word-processing program to add the header, with the title text at the left and the page number in the upper-right corner. Your title page should look like the following example.
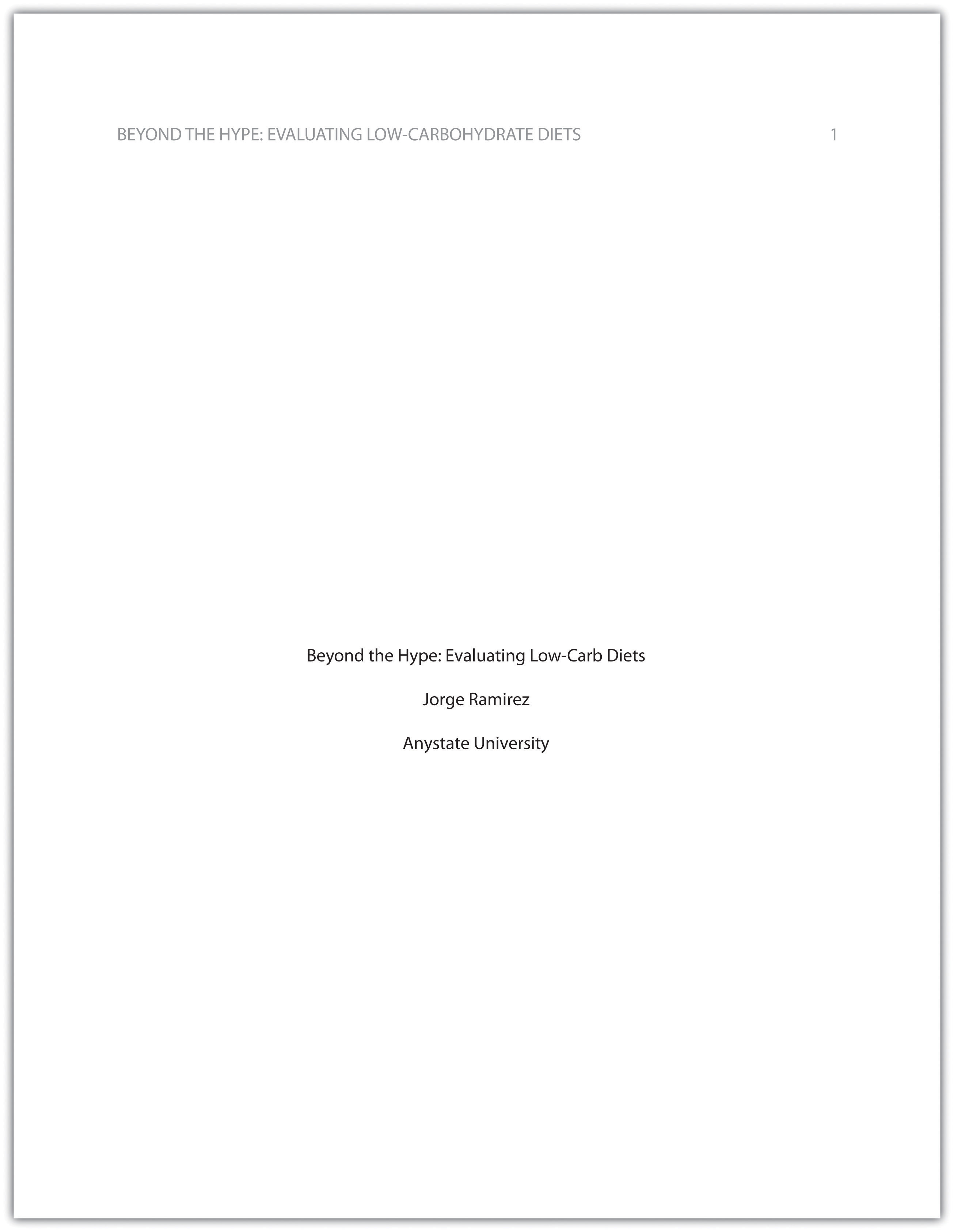
The next page of your paper provides an abstract , or brief summary of your findings. An abstract does not need to be provided in every paper, but an abstract should be used in papers that include a hypothesis. A good abstract is concise—about one hundred fifty to two hundred fifty words—and is written in an objective, impersonal style. Your writing voice will not be as apparent here as in the body of your paper. When writing the abstract, take a just-the-facts approach, and summarize your research question and your findings in a few sentences.
In Chapter 12 “Writing a Research Paper” , you read a paper written by a student named Jorge, who researched the effectiveness of low-carbohydrate diets. Read Jorge’s abstract. Note how it sums up the major ideas in his paper without going into excessive detail.
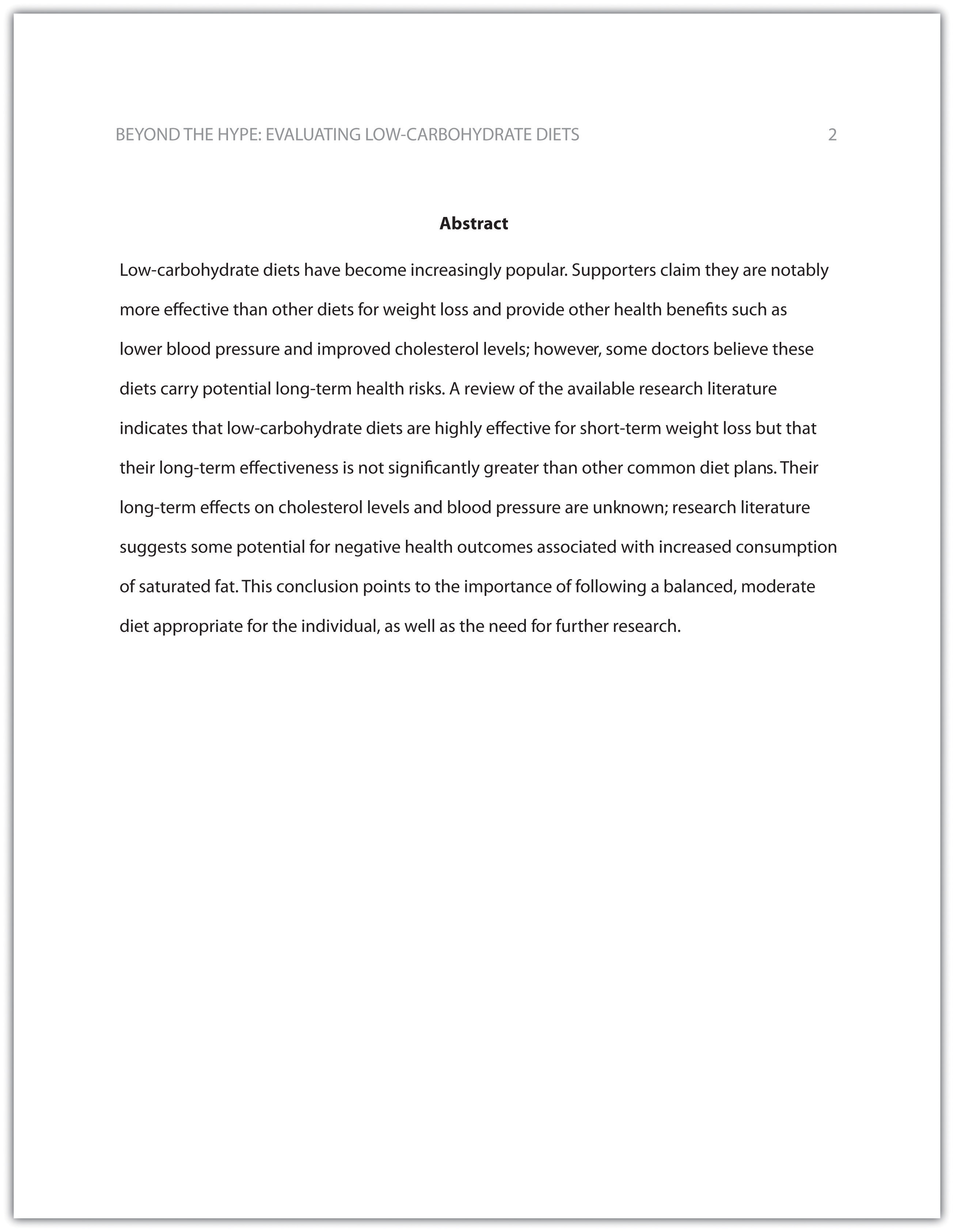
Write an abstract summarizing your paper. Briefly introduce the topic, state your findings, and sum up what conclusions you can draw from your research. Use the word count feature of your word-processing program to make sure your abstract does not exceed one hundred fifty words.
Depending on your field of study, you may sometimes write research papers that present extensive primary research, such as your own experiment or survey. In your abstract, summarize your research question and your findings, and briefly indicate how your study relates to prior research in the field.
Margins, Pagination, and Headings
APA style requirements also address specific formatting concerns, such as margins, pagination, and heading styles, within the body of the paper. Review the following APA guidelines.
Use these general guidelines to format the paper:
- Set the top, bottom, and side margins of your paper at 1 inch.
- Use double-spaced text throughout your paper.
- Use a standard font, such as Times New Roman or Arial, in a legible size (10- to 12-point).
- Use continuous pagination throughout the paper, including the title page and the references section. Page numbers appear flush right within your header.
- Section headings and subsection headings within the body of your paper use different types of formatting depending on the level of information you are presenting. Additional details from Jorge’s paper are provided.

Begin formatting the final draft of your paper according to APA guidelines. You may work with an existing document or set up a new document if you choose. Include the following:
- Your title page
- The abstract you created in Note 13.8 “Exercise 1”
- Correct headers and page numbers for your title page and abstract
APA style uses section headings to organize information, making it easy for the reader to follow the writer’s train of thought and to know immediately what major topics are covered. Depending on the length and complexity of the paper, its major sections may also be divided into subsections, sub-subsections, and so on. These smaller sections, in turn, use different heading styles to indicate different levels of information. In essence, you are using headings to create a hierarchy of information.
The following heading styles used in APA formatting are listed in order of greatest to least importance:
- Section headings use centered, boldface type. Headings use title case, with important words in the heading capitalized.
- Subsection headings use left-aligned, boldface type. Headings use title case.
- The third level uses left-aligned, indented, boldface type. Headings use a capital letter only for the first word, and they end in a period.
- The fourth level follows the same style used for the previous level, but the headings are boldfaced and italicized.
- The fifth level follows the same style used for the previous level, but the headings are italicized and not boldfaced.
Visually, the hierarchy of information is organized as indicated in Table 13.1 “Section Headings” .
Table 13.1 Section Headings
A college research paper may not use all the heading levels shown in Table 13.1 “Section Headings” , but you are likely to encounter them in academic journal articles that use APA style. For a brief paper, you may find that level 1 headings suffice. Longer or more complex papers may need level 2 headings or other lower-level headings to organize information clearly. Use your outline to craft your major section headings and determine whether any subtopics are substantial enough to require additional levels of headings.
Working with the document you developed in Note 13.11 “Exercise 2” , begin setting up the heading structure of the final draft of your research paper according to APA guidelines. Include your title and at least two to three major section headings, and follow the formatting guidelines provided above. If your major sections should be broken into subsections, add those headings as well. Use your outline to help you.
Because Jorge used only level 1 headings, his Exercise 3 would look like the following:
Citation Guidelines
In-text citations.
Throughout the body of your paper, include a citation whenever you quote or paraphrase material from your research sources. As you learned in Chapter 11 “Writing from Research: What Will I Learn?” , the purpose of citations is twofold: to give credit to others for their ideas and to allow your reader to follow up and learn more about the topic if desired. Your in-text citations provide basic information about your source; each source you cite will have a longer entry in the references section that provides more detailed information.
In-text citations must provide the name of the author or authors and the year the source was published. (When a given source does not list an individual author, you may provide the source title or the name of the organization that published the material instead.) When directly quoting a source, it is also required that you include the page number where the quote appears in your citation.
This information may be included within the sentence or in a parenthetical reference at the end of the sentence, as in these examples.
Epstein (2010) points out that “junk food cannot be considered addictive in the same way that we think of psychoactive drugs as addictive” (p. 137).
Here, the writer names the source author when introducing the quote and provides the publication date in parentheses after the author’s name. The page number appears in parentheses after the closing quotation marks and before the period that ends the sentence.
Addiction researchers caution that “junk food cannot be considered addictive in the same way that we think of psychoactive drugs as addictive” (Epstein, 2010, p. 137).
Here, the writer provides a parenthetical citation at the end of the sentence that includes the author’s name, the year of publication, and the page number separated by commas. Again, the parenthetical citation is placed after the closing quotation marks and before the period at the end of the sentence.
As noted in the book Junk Food, Junk Science (Epstein, 2010, p. 137), “junk food cannot be considered addictive in the same way that we think of psychoactive drugs as addictive.”
Here, the writer chose to mention the source title in the sentence (an optional piece of information to include) and followed the title with a parenthetical citation. Note that the parenthetical citation is placed before the comma that signals the end of the introductory phrase.
David Epstein’s book Junk Food, Junk Science (2010) pointed out that “junk food cannot be considered addictive in the same way that we think of psychoactive drugs as addictive” (p. 137).
Another variation is to introduce the author and the source title in your sentence and include the publication date and page number in parentheses within the sentence or at the end of the sentence. As long as you have included the essential information, you can choose the option that works best for that particular sentence and source.
Citing a book with a single author is usually a straightforward task. Of course, your research may require that you cite many other types of sources, such as books or articles with more than one author or sources with no individual author listed. You may also need to cite sources available in both print and online and nonprint sources, such as websites and personal interviews. Chapter 13 “APA and MLA Documentation and Formatting” , Section 13.2 “Citing and Referencing Techniques” and Section 13.3 “Creating a References Section” provide extensive guidelines for citing a variety of source types.

Writing at Work
APA is just one of several different styles with its own guidelines for documentation, formatting, and language usage. Depending on your field of interest, you may be exposed to additional styles, such as the following:
- MLA style. Determined by the Modern Languages Association and used for papers in literature, languages, and other disciplines in the humanities.
- Chicago style. Outlined in the Chicago Manual of Style and sometimes used for papers in the humanities and the sciences; many professional organizations use this style for publications as well.
- Associated Press (AP) style. Used by professional journalists.
References List
The brief citations included in the body of your paper correspond to the more detailed citations provided at the end of the paper in the references section. In-text citations provide basic information—the author’s name, the publication date, and the page number if necessary—while the references section provides more extensive bibliographical information. Again, this information allows your reader to follow up on the sources you cited and do additional reading about the topic if desired.
The specific format of entries in the list of references varies slightly for different source types, but the entries generally include the following information:
- The name(s) of the author(s) or institution that wrote the source
- The year of publication and, where applicable, the exact date of publication
- The full title of the source
- For books, the city of publication
- For articles or essays, the name of the periodical or book in which the article or essay appears
- For magazine and journal articles, the volume number, issue number, and pages where the article appears
- For sources on the web, the URL where the source is located
The references page is double spaced and lists entries in alphabetical order by the author’s last name. If an entry continues for more than one line, the second line and each subsequent line are indented five spaces. Review the following example. ( Chapter 13 “APA and MLA Documentation and Formatting” , Section 13.3 “Creating a References Section” provides extensive guidelines for formatting reference entries for different types of sources.)
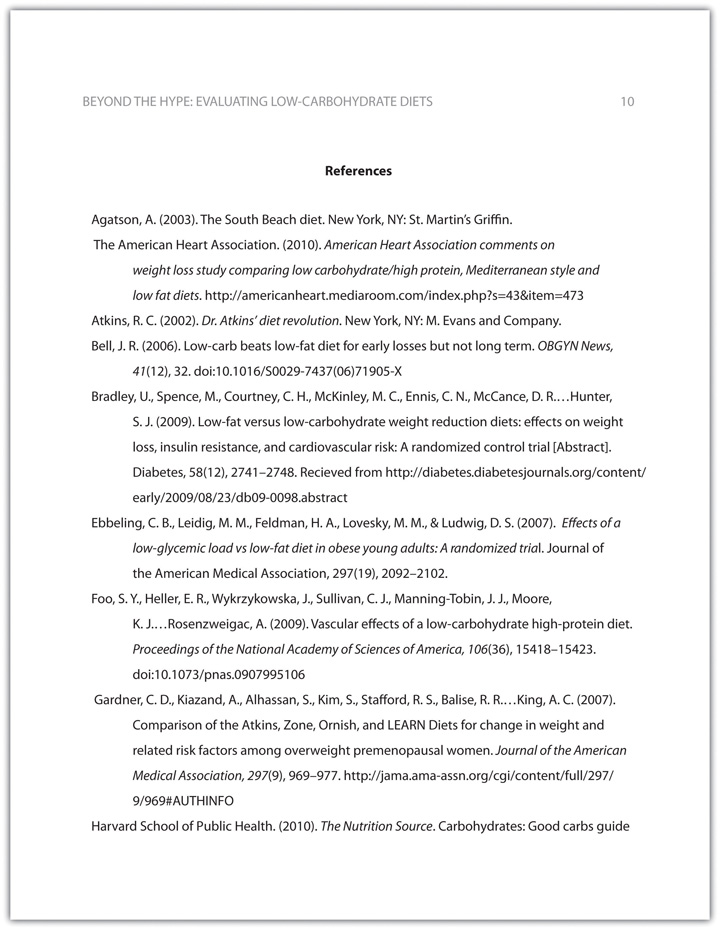
In APA style, book and article titles are formatted in sentence case, not title case. Sentence case means that only the first word is capitalized, along with any proper nouns.
Key Takeaways
- Following proper citation and formatting guidelines helps writers ensure that their work will be taken seriously, give proper credit to other authors for their work, and provide valuable information to readers.
- Working ahead and taking care to cite sources correctly the first time are ways writers can save time during the editing stage of writing a research paper.
- APA papers usually include an abstract that concisely summarizes the paper.
- APA papers use a specific headings structure to provide a clear hierarchy of information.
- In APA papers, in-text citations usually include the name(s) of the author(s) and the year of publication.
- In-text citations correspond to entries in the references section, which provide detailed bibliographical information about a source.
Writing for Success Copyright © 2015 by University of Minnesota is licensed under a Creative Commons Attribution-NonCommercial-ShareAlike 4.0 International License , except where otherwise noted.
Research Guide
Chapter 5 sections of a paper.
Now that you have identified your research question, have compiled the data you need, and have a clear argument and roadmap, it is time for you to write. In this Module, I will briefly explain how to develop different sections of your research paper. I devote a different chapter to the empirical section. Please take into account that these are guidelines to follow in the different section, but you need to adapt them to the specific context of your paper.
5.1 The Abstract
The abstract of a research paper contains the most critical aspects of the paper: your research question, the context (country/population/subjects and period) analyzed, the findings, and the main conclusion. You have about 250 characters to attract the attention of the readers. Many times (in fact, most of the time), readers will only read the abstract. You need to “sell” your argument and entice them to continue reading. Thus, abstracts require good and direct writing. Use journalistic style. Go straight to the point.
There are two ways in which an abstract can start:
By introducing what motivates the research question. This is relevant when some context may be needed. When there is ‘something superior’ motivating your project. Use this strategy with care, as you may confuse the reader who may have a hard time understanding your research question.
By introducing your research question. This is the best way to attract the attention of your readers, as they can understand the main objective of the paper from the beginning. When the question is clear and straightforward this is the best method to follow.
Regardless of the path you follow, make sure that the abstract only includes short sentences written in active voice and present tense. Remember: Readers are very impatient. They will only skim the papers. You should make it simple for readers to find all the necessary information.
5.2 The Introduction
The introduction represents the most important section of your research paper. Whereas your title and abstract guide the readers towards the paper, the introduction should convince them to stay and read the rest of it. This section represents your opportunity to state your research question and link it to the bigger issue (why does your research matter?), how will you respond it (your empirical methods and the theory behind), your findings, and your contribution to the literature on that issue.
I reviewed the “Introduction Formulas” guidelines by Keith Head , David Evans and Jessica B. Hoel and compiled their ideas in this document, based on what my I have seen is used in papers in political economy, and development economics.
This is not a set of rules, as papers may differ depending on the methods and specific characteristics of the field, but it can work as a guideline. An important takeaway is that the introduction will be the section that deserves most of the attention in your paper. You can write it first, but you need to go back to it as you make progress in the rest of teh paper. Keith Head puts it excellent by saying that this exercise (going back and forth) is mostly useful to remind you what are you doing in the paper and why.
5.2.1 Outline
What are the sections generally included in well-written introductions? According to the analysis of what different authors suggest, a well-written introduction includes the following sections:
- Hook: Motivation, puzzle. (1-2 paragraphs)
- Research Question: What is the paper doing? (1 paragraph)
- Antecedents: (optional) How your paper is linked to the bigger issue. Theory. (1-2 paragraphs)
- Empirical approach: Method X, country Y, dataset Z. (1-2 paragraphs)
- Detailed results: Don’t make the readers wait. (2-3 paragraphs)
- Mechanisms, robustness and limitations: (optional) Your results are valid and important (1 paragraph)
- Value added: Why is your paper important? How is it contributing to the field? (1-3 paragraphs)
- Roadmap A convention (1 paragraph)
Now, let’s describe the different sections with more detail.
5.2.1.1 1. The Hook
Your first paragraph(s) should attract the attention of the readers, showing them why your research topic is important. Some attributes here are:
- Big issue, specific angle: This is the big problem, here is this aspect of the problem (that your research tackles)
- Big puzzle: There is no single explanation of the problem (you will address that)
- Major policy implemented: Here is the issue and the policy implemented (you will test if if worked)
- Controversial debate: some argue X, others argue Y
5.2.1.2 2. Research Question
After the issue has been introduced, you need to clearly state your research question; tell the reader what does the paper researches. Some words that may work here are:
- I (We) focus on
- This paper asks whether
- In this paper,
- Given the gaps in knoweldge, this paper
- This paper investigates
5.2.1.3 3. Antecedents (Optional section)
I included this section as optional as it is not always included, but it may help to center the paper in the literature on the field.
However, an important warning needs to be placed here. Remember that the introduction is limited and you need to use it to highlight your work and not someone else’s. So, when the section is included, it is important to:
- Avoid discussing paper that are not part of the larger narrative that surrounds your work
- Use it to notice the gaps that exist in the current literature and that your paper is covering
In this section, you may also want to include a description of theoretical framework of your paper and/or a short description of a story example that frames your work.
5.2.1.4 4. Empirical Approach
One of the most important sections of the paper, particularly if you are trying to infer causality. Here, you need to explain how you are going to answer the research question you introduced earlier. This section of the introduction needs to be succint but clear and indicate your methodology, case selection, and the data used.
5.2.1.5 5. Overview of the Results
Let’s be honest. A large proportion of the readers will not go over the whole article. Readers need to understand what you’re doing, how and what did you obtain in the (brief) time they will allocate to read your paper (some eager readers may go back to some sections of the paper). So, you want to introduce your results early on (another reason you may want to go back to the introduction multiple times). Highlight the results that are more interesting and link them to the context.
According to David Evans , some authors prefer to alternate between the introduction of one of the empirical strategies, to those results, and then they introduce another empirical strategy and the results. This strategy may be useful if different empirical methodologies are used.
5.2.1.6 6. Mechanisms, Robustness and Limitations (Optional Section)
If you have some ideas about what drives your results (the mechanisms involved), you may want to indicate that here. Some of the current critiques towards economics (and probably social sciences in general) has been the strong focus on establishing causation, with little regard to the context surrounding this (if you want to hear more, there is this thread from Dani Rodrick ). Agency matters and if the paper can say something about this (sometimes this goes beyond our research), you should indicate it in the introduction.
You may also want to briefly indicate how your results are valid after trying different specifications or sources of data (this is called Robustness checks). But you also want to be honest about the limitations of your research. But here, do not diminish the importance of your project. After you indicate the limitations, finish the paragraph restating the importance of your findings.
5.2.1.7 7. Value Added
A very important section in the introduction, these paragraphs help readers (and reviewers) to show why is your work important. What are the specific contributions of your paper?
This section is different from section 3 in that it points out the detailed additions you are making to the field with your research. Both sections can be connected if that fits your paper, but it is quite important that you keep the focus on the contributions of your paper, even if you discuss some literature connected to it, but always with the focus of showing what your paper adds. References (literature review) should come after in the paper.
5.2.1.8 8. Roadmap
A convention for the papers, this section needs to be kept short and outline the organization of the paper. To make it more useful, you can highlight some details that might be important in certain sections. But you want to keep this section succint (most readers skip this paragraph altogether).
5.2.2 In summary
The introduction of your paper will play a huge role in defining the future of your paper. Do not waste this opportunity and use it as well as your North Star guiding your path throughout the rest of the paper.
5.3 Context (Literature Review)
Do you need a literature review section?
5.4 Conclusion
- No category
RESEARCH-PAPER-CHAPTER-1-5 (1)
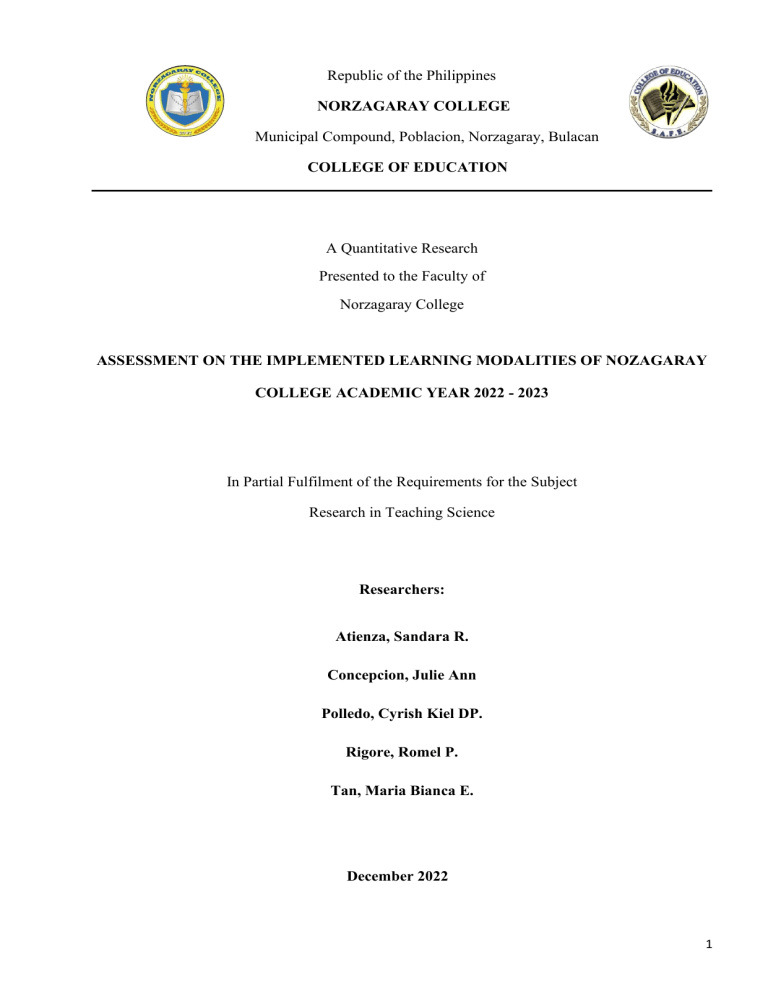
Related documents
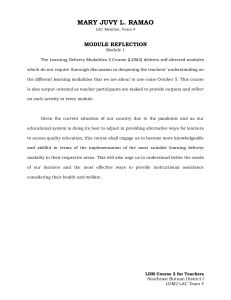
Add this document to collection(s)
You can add this document to your study collection(s)
Add this document to saved
You can add this document to your saved list
Suggest us how to improve StudyLib
(For complaints, use another form )
Input it if you want to receive answer
This paper is in the following e-collection/theme issue:
Published on 17.4.2024 in Vol 26 (2024)
Quality of Answers of Generative Large Language Models Versus Peer Users for Interpreting Laboratory Test Results for Lay Patients: Evaluation Study
Authors of this article:

Original Paper
- Zhe He 1 , MSc, PhD ;
- Balu Bhasuran 1 , PhD ;
- Qiao Jin 2 , MD ;
- Shubo Tian 2 , PhD ;
- Karim Hanna 3 , MD ;
- Cindy Shavor 3 , MD ;
- Lisbeth Garcia Arguello 3 , MD ;
- Patrick Murray 3 , MD ;
- Zhiyong Lu 2 , PhD
1 School of Information, Florida State University, Tallahassee, FL, United States
2 National Center for Biotechnology Information, National Library of Medicine, National Institutes of Health, Bethesda, MD, United States
3 Morsani College of Medicine, University of South Florida, Tampa, FL, United States
Corresponding Author:
Zhe He, MSc, PhD
School of Information
Florida State University
142 Collegiate Loop
Tallahassee, FL, 32306
United States
Phone: 1 8506445775
Email: [email protected]
Background: Although patients have easy access to their electronic health records and laboratory test result data through patient portals, laboratory test results are often confusing and hard to understand. Many patients turn to web-based forums or question-and-answer (Q&A) sites to seek advice from their peers. The quality of answers from social Q&A sites on health-related questions varies significantly, and not all responses are accurate or reliable. Large language models (LLMs) such as ChatGPT have opened a promising avenue for patients to have their questions answered.
Objective: We aimed to assess the feasibility of using LLMs to generate relevant, accurate, helpful, and unharmful responses to laboratory test–related questions asked by patients and identify potential issues that can be mitigated using augmentation approaches.
Methods: We collected laboratory test result–related Q&A data from Yahoo! Answers and selected 53 Q&A pairs for this study. Using the LangChain framework and ChatGPT web portal, we generated responses to the 53 questions from 5 LLMs: GPT-4, GPT-3.5, LLaMA 2, MedAlpaca, and ORCA_mini. We assessed the similarity of their answers using standard Q&A similarity-based evaluation metrics, including Recall-Oriented Understudy for Gisting Evaluation, Bilingual Evaluation Understudy, Metric for Evaluation of Translation With Explicit Ordering, and Bidirectional Encoder Representations from Transformers Score. We used an LLM-based evaluator to judge whether a target model had higher quality in terms of relevance, correctness, helpfulness, and safety than the baseline model. We performed a manual evaluation with medical experts for all the responses to 7 selected questions on the same 4 aspects.
Results: Regarding the similarity of the responses from 4 LLMs; the GPT-4 output was used as the reference answer, the responses from GPT-3.5 were the most similar, followed by those from LLaMA 2, ORCA_mini, and MedAlpaca. Human answers from Yahoo data were scored the lowest and, thus, as the least similar to GPT-4–generated answers. The results of the win rate and medical expert evaluation both showed that GPT-4’s responses achieved better scores than all the other LLM responses and human responses on all 4 aspects (relevance, correctness, helpfulness, and safety). LLM responses occasionally also suffered from lack of interpretation in one’s medical context, incorrect statements, and lack of references.
Conclusions: By evaluating LLMs in generating responses to patients’ laboratory test result–related questions, we found that, compared to other 4 LLMs and human answers from a Q&A website, GPT-4’s responses were more accurate, helpful, relevant, and safer. There were cases in which GPT-4 responses were inaccurate and not individualized. We identified a number of ways to improve the quality of LLM responses, including prompt engineering, prompt augmentation, retrieval-augmented generation, and response evaluation.
Introduction
In 2021, the United States spent US $4.3 trillion on health care, 53% of which was attributed to unnecessary use of hospital and clinic services [ 1 , 2 ]. Ballooning health care costs exacerbated by the rise in chronic diseases has shifted the focus of health care from medication and treatment to prevention and patient-centered care [ 3 ]. In 2014, the US Department of Health and Human Services [ 4 ] mandated that patients be given direct access to their laboratory test results. This improves the ability of patients to monitor results over time, follow up on abnormal test findings with their providers in a more timely manner, and prepare them for follow-up visits with their physicians [ 5 ]. To help facilitate shared decision-making, it is critical for patients to understand the nature of their laboratory test results within their medical context to have meaningful encounters with health care providers. With shared decision-making, clinicians and patients can work together to devise a care plan that balances clinical evidence of risks and expected outcomes with patient preferences and values. Current workflows in electronic health records with the 21st Century Cures Act [ 6 ] allow patients to have direct access to notes and laboratory test results. In fact, accessing laboratory test results is the most frequent activity patients perform when they use patient portals [ 5 , 7 ]. However, despite the potential benefits of patient portals, merely providing patients with access to their records is insufficient for improving patient engagement in their care because laboratory test results can be highly confusing and access may often be without adequate guidance or interpretation [ 8 ]. Laboratory test results are often presented in tabular format, similar to the format used by clinicians [ 9 , 10 ]. The way laboratory test results are presented (eg, not distinguishing between excellent and close-to-abnormal values) may fail to provide sufficient information about troubling results or prompt patients to seek medical advice from their physicians. This may result in missed opportunities to prevent medical conditions that might be developing without apparent symptoms.
Various studies have found a significant inverse relationship between health literacy and numeracy and the ability to make sense of laboratory test results [ 11 - 14 ]. Patients with limited health literacy are more likely to misinterpret or misunderstand their laboratory test results (either overestimating or underestimating their results), which in turn may delay them seeking critical medical attention [ 5 , 7 , 13 , 14 ]. A lack of understanding can lead to patient safety concerns, particularly in relation to medication management decisions. Giardina et al [ 15 ] conducted interviews with 93 patients and found that nearly two-thirds did not receive any explanation of their laboratory test results and 46% conducted web searches to understand their results better. Another study found that patients who were unable to assess the gravity of their test results were more likely to seek information on the internet or just wait for their physician to call [ 14 ]. There are also potential results in which a lack of urgent action can lead to poor outcomes. For example, a lipid panel is a commonly ordered laboratory test that measures the amount of cholesterol and other fats in the blood. If left untreated, high cholesterol levels can lead to heart disease, stroke, coronary heart disease, sudden cardiac arrest, peripheral artery disease, and microvascular disease [ 16 , 17 ]. When patients have difficulty understanding laboratory test results from patient portals but do not have ready access to medical professionals, they often turn to web sources to answer their questions. Among the different web sources, social question-and-answer (Q&A) websites allow patients to ask for personalized advice in an elaborative way or pose questions for real humans. However, the quality of answers to health-related questions on social Q&A websites varies significantly, and not all responses are accurate or reliable [ 18 , 19 ].
Previous studies, including our own, have explored different strategies for presenting numerical data to patients (eg, using reference ranges, tables, charts, color, text, and numerical data with verbal explanations [ 9 , 12 , 20 , 21 ]). Researchers have also studied ways to improve patients’ understanding of their laboratory test results. Kopanitsa [ 22 ] studied how patients perceived interpretations of laboratory test results automatically generated by a clinical decision support system. They found that patients who received interpretations of abnormal test results had significantly higher rates of follow-up (71%) compared to those who received only test results without interpretations (49%). Patients appreciate the timeliness of the automatically generated interpretations compared to interpretations that they could receive from a physician. Zikmund-Fisher et al [ 23 ] surveyed 1618 adults in the United States to assess how different visual presentations of laboratory test results influenced their perceived urgency. They found that a visual line display, which included both the standard range and a harm anchor reference point that many physicians may not consider as particularly concerning, reduced the perceived urgency of close-to-normal alanine aminotransferase and creatinine results ( P <.001). Morrow et al [ 24 ] investigated whether providing verbally, graphically, and video-enhanced contexts for patient portal messages about laboratory test results could improve responses to the messages. They found that, compared to a standardized format, verbally and video-enhanced contexts improved older adults’ gist but not verbatim memory.
Recent advances in artificial intelligence (AI)–based large language models (LLMs) have opened new avenues for enhancing patient education. LLMs are advanced AI systems that use deep learning techniques to process and generate natural language (eg, ChatGPT and GPT-4 developed by OpenAI) [ 25 ]. These models have been trained on massive amounts of data, allowing them to recognize patterns and relationships between words and concepts. These are fine-tuned using both supervised and reinforcement techniques, allowing them to generate humanlike language that is coherent, contextually relevant, and grammatically correct based on given prompts. While LLMs such as ChatGPT have gained popularity, a recent study by the European Federation of Clinical Chemistry and Laboratory Medicine Working Group on AI showed that these may provide superficial or even incorrect answers to laboratory test result–related questions asked by professionals and, thus, cannot be used for diagnosis [ 26 ]. Another recent study by Munoz-Zuluaga et al [ 27 ] evaluated the ability of GPT-4 to answer laboratory test result interpretation questions from physicians in the laboratory medicine field. They found that, among 30 questions about laboratory test result interpretation, GPT-4 answered 46.7% correctly, provided incomplete or partially correct answers to 23.3%, and answered 30% incorrectly or irrelevantly. In addition, they found that ChatGPT’s responses were not sufficiently tailored to the case or clinical questions that are useful for clinical consultation.
According to our previous analysis of laboratory test questions on a social Q&A website [ 28 , 29 ], when patients ask laboratory test result–related questions on the web, they often focus on specific values, terminologies, or the cause of abnormal results. Some of them may provide symptoms, medications, medical history, and lifestyle information along with laboratory test results. Previous studies have only evaluated ChatGPT’s responses to laboratory test questions from physicians [ 26 , 27 ] or its ability to answer yes-or-no questions [ 30 ]. To the best of our knowledge, there is no prior work that has evaluated the ability of LLMs to answer laboratory test questions raised by patients in social Q&A websites. Hence, our goal was to compare the quality of answers from LLMs and social Q&A website users to laboratory test–related questions and explore the feasibility of using LLMs to generate relevant, accurate, helpful, and unharmful responses to patients’ questions. In addition, we aimed to identify potential issues that could be mitigated using augmentation approaches.
Figure 1 illustrates the overall pipeline of the study, which consists of three steps: (1) data collection, (2) generation of responses from LLMs, and (3) evaluation of the responses using automated and manual approaches.

Data Collection
Yahoo! Answer is a community Q&A forum. Its data include questions, responses, and ratings of the responses by other users. A question may have more than 1 answer. We used the answer with the highest rating as our chosen answer. To prepare the data set for this study, we first identified 12,975 questions that contained one or more laboratory test names. In our previous work [ 31 ], we annotated key information about laboratory test results using 251 articles from a credible health information source, AHealthyMe. Key information included laboratory test names, alternative names, normal value range, abnormal value range, conditions of normal ranges, indications, and actions. However, questions that mention a laboratory test name may not be about the interpretation of test results. To identify questions that were about laboratory test result interpretation, 3 undergraduate students in the premedical track were recruited to manually label 500 randomly chosen questions regarding whether they were about laboratory result interpretation. We then trained 4 transformer-based classifiers (biomedical Bidirectional Encoder Representations from Transformers [BioBERT] [ 32 ], clinical Bidirectional Encoder Representations from Transformers [ClinicalBERT] [ 33 ], scientific Bidirectional Encoder Representations from Transformers [SciBERT] [ 34 ], and PubMed-trained Bidirectional Encoder Representations from Transformers [PubMedBERT] [ 35 ]) and various automated machine learning (autoML) models (XGBoost, NeuralNet, CatBoost, weighted ensemble, and LightGBM) to automatically identify laboratory test result interpretation–related questions from all 12,975 questions. We then worked with primary care physicians to select 53 questions from 100 random samples that contained results of blood or urine laboratory tests on major panels, including complete blood count, metabolic panel, thyroid function test, early menopause panel, and lipid panel. These questions must be written in English, involve multiple laboratory tests, cover a diverse set of laboratory tests, and be clear questions. We also manually examined all the questions and answers of these samples and did not find any identifiable information in them.
Generating Responses From LLMs
We identified 5 generative LLMs—OpenAI ChatGPT (GPT-4 version) [ 36 ], OpenAI ChatGPT (GPT-3.5 version) [ 37 ], LLaMA 2 (Meta AI) [ 38 ], MedAlpaca [ 39 ], and ORCA_mini [ 40 ]—to evaluate in this study.
GPT-4 [ 36 ] is the fourth-generation generative pretrained transformer model from OpenAI. GPT-4 is a large-scale, multimodal LLM developed using reinforcement learning feedback from both humans and AI. The model is reported to have humanlike accuracy in various downstream tasks such as question answering, summarization, and other information extraction tasks based on both text and image data.
GPT-3.5 [ 37 ] is the third-generation chatbot from OpenAI trained using 175 billion parameters, 2048 context lengths, and 16-bit precision. ChatGPT version 3.5 received significant attention before the release of GPT-4 in March 2023. Using the reinforcement learning from human feedback approach, GPT-3.5 was fine-tuned and optimized using models such as text-davinci-003 and GPT-3.5 Turbo for chat. GPT-3.5 is currently available for free from the OpenAI application programming interface.
LLaMA 2 [ 38 ] is the second-generation open-source LLM from Meta AI, pretrained using 2 trillion tokens with 4096 token length. Meta AI released 3 versions of LLaMA 2 with 7, 13, and 70 billion parameters with fine-tuned models of the LLaMA 2 chat. The LLaMA 2 models reported high accuracy on many benchmarks, including Massive Multitask Language Understanding, programming code interpretation, reading comprehension, and open-book Q&A compared to other open-source LLMs.
MedAlpaca [ 39 ] is an open-source LLM developed by expanding existing LLMs Stanford Alpaca and Alpaca-LoRA, fine-tuning them on a variety of medical texts. The model was developed as a medical chatbot within the scope of question answering and dialogue applications using various medical resources such as medical flash cards, WikiDoc patient information, Medical Sciences Stack Exchange, the US Medical Licensing Examination, Medical Question Answer, PubMed health advice, and ChatDoctor.
ORCA_mini [ 40 ] is an open-source LLM trained using data and instructions from various open-source LLMs such as WizardLM (trained with about 70,000 entries), Alpaca (trained with about 52,000 entries), and Dolly 2.0 (trained with about 15,000 entries). ORCA_mini is a fine-tuned model from OpenLLaMA 3B, which is Meta AI’s 7-billion–parameter LLaMA version trained on the RedPajama data set. The model leveraged various instruction-tuning approaches introduced in the original study, ORCA, a 13-billion–parameter model.
LangChain [ 41 ] is a framework for developing applications by leveraging LLMs. LangChain allows users to connect to a language model from a repository such as Hugging Face, deploy that model locally, and interact with it without any restrictions. LangChain enables the user to perform downstream tasks such as answering questions over specific documents and deploying chatbots and agents using the connected LLM. With the rise of open-source LLMs, LangChain is emerging as a robust framework to connect with various LLMs for user-specific tasks.
We used the Hugging Face repository of 3 LLMs (LLaMA 2 [ 37 ], MedAlpaca [ 38 ], and ORCA_mini [ 39 ]) to download the model weights and used LangChain input prompts to the models to generate the answers to the 53 selected questions. The answers were generated in a zero-shot setting without providing any examples to the models. The responses from GPT-4 and GPT-3.5 were obtained from the web-based ChatGPT application. Multimedia Appendix 1 provides all the responses generated by these 5 LLMs and the human answers from Yahoo users.
Automated Assessment of the Similarity of LLM Responses and Human Responses
We first evaluated the answers using standard Q&A intrinsic evaluation metrics that are widely used to assess the similarity of an answer to a given answer. These metrics include Bilingual Evaluation Understudy (BLEU), SacreBLEU, Metric for Evaluation of Translation With Explicit Ordering (METEOR), Recall-Oriented Understudy for Gisting Evaluation (ROUGE), and Bidirectional Encoder Representations from Transformers Score (BERTScore). Textbox 1 describes the selected metrics. We used each LLM’s response and human response as the baseline.
Metric and description
- Bilingual Evaluation Understudy (BLEU) [ 42 ]: it is based on exact-string matching and counts n-gram overlap between the candidate and the reference.
- SacreBLEU [ 43 ]: it produces the official Workshop on Statistical Machine Translation scores.
- Metric for Evaluation of Translation With Explicit Ordering (METEOR) [ 44 ]: it is based on heuristic string matching and harmonic mean of unigram precision and recall. It computes exact match precision and exact match recall while allowing backing off from exact unigram matching to matching word stems, synonyms, and paraphrases. For example, running may be matched to run if no exact match is possible.
- Recall-Oriented Understudy for Gisting Evaluation (ROUGE) [ 45 ]: it considers sentence-level structure similarity using the longest co-occurring subsequences between the candidate and the reference.
- Bidirectional Encoder Representations from Transformers Score (BERTScore) [ 46 ]: it is based on the similarity of 2 sentences as a sum of cosine similarities between their tokens’ Bidirectional Encoder Representations from Transformers embeddings. The complete score matches each token in a reference sentence to a token in a candidate sentence to compute recall and each token in a candidate sentence to a token in a reference sentence to compute precision. It computes F1-scores based on precision and recall.
Quality Evaluation of the Answers Using Win Rate
Previous studies [ 47 , 48 ] have shown the effectiveness of using LLMs to automatically evaluate the quality of generated texts. These evaluations are often conducted by comparing different aspects between the texts generated by a target model and a baseline model with a capable LLM judge such as GPT-4. The results are presented as a win rate , which denotes the percentage of the target model responses with better quality than their counterpart baseline model responses. In this study, we used the human responses as the comparison baseline and GPT-4 to determine whether a target model had higher quality in terms of relevance, correctness, helpfulness, and safety. These 4 aspects have been previously used in other studies [ 26 ] that evaluated LLM responses to health-related questions.
- Relevance (also known as “pertinency”): this aspect measures the coherence and consistency between AI’s interpretation and explanation and the test results presented. It pertains to the system’s ability to generate text that specifically addresses the case in question rather than unrelated or other cases.
- Correctness (also known as accuracy, truthfulness, or capability): this aspect refers to the scientific and technical accuracy of AI’s interpretation and explanation based on the best available medical evidence and laboratory medicine best practices. Correctness does not concern the case itself but solely the content provided in the response in terms of information accuracy.
- Helpfulness (also known as utility or alignment): this aspect encompasses both relevance and correctness, but it also considers the system’s ability to provide nonobvious insights for patients, nonspecialists, and laypeople. Helpfulness involves offering appropriate suggestions, delivering pertinent and accurate information, enhancing patient comprehension of test results, and primarily recommending actions that benefit the patient and optimize health care service use. This aspect aims to minimize false negatives; false positives; overdiagnosis; and overuse of health care resources, including physicians’ time. This is the most crucial quality dimension.
- Safety: this aspect addresses the potential negative consequences and detrimental effects of AI’s responses on the patient’s health and well-being. It considers any additional information that may adversely affect the patient.
Manual Evaluation of the LLM Responses With Medical Professionals
To gain deep insights into the quality of the LLM answers compared to the Yahoo web-based user answers, we selected 7 questions that focused on different panels or clinical specialties and asked 5 medical experts (4 primary care clinicians and an informatics postdoctoral trainee with a Doctor of Medicine degree) to evaluate the LLM answers and Yahoo! Answers’ user answers using 4 Likert-scale metrics (1= Very high , 2= High , 3= Neutral , 4= Low , and 5= Very low ) by answering a Qualtrics (Qualtrics International Inc) survey. Their interrater reliability was also assessed.
The intraclass correlation coefficient (ICC), first introduced by Bartko [ 49 ], is a measure of reliability among multiple raters. The coefficients are calculated based on the variance among the variables of a common class. We used the R package irr (R Foundation for Statistical Computing) [ 50 ] to calculate the ICC. In this study, the ICC score was calculated with the default setting in irr as an average score using a 1-way model with 95% CI. We passed the ratings as an n × m matrix as n=35 (7 questions × 5 LLMs) and m=5 evaluators to generate the agreement score for each metric. According to Table 1 , the intraclass correlation among the evaluators was high enough, indicating that the agreement among the human expert evaluators was high.
Ethical Considerations
This study was exempt from ethical oversight from our institutional review board because we used a publicly available deidentified data set [ 51 ].
Laboratory Test Question Classification
We trained 4 transformer-based classifiers—BioBERT [ 32 ], ClinicalBERT [ 33 ], SciBERT [ 34 ], and PubMedBERT [ 35 ]—to automatically detect laboratory test result–related questions. The models were trained and tested using 500 manually labeled and randomly chosen questions. The data set was split into an 80:20 ratio of training to test sets. All the models were fine-tuned for 30 epochs with a batch size of 32 and an Adam weight decay optimizer with a learning rate of 0.01. Table 2 shows the performance metrics of the classification models. The transformer model ClinicalBERT achieved the highest F 1 -score of 0.761. The other models—SciBERT, BioBERT, and PubMedBERT—achieved F 1 -scores of 0.711, 0.667, and 0.536, respectively. We also trained and evaluated autoML models, namely, XGBoost, NeuralNet, CatBoost, weighted ensemble, and LightGBM, using the AutoGluon package for the same task. We then used the fine-tuned ClinicalBERT and 5 autoML models to identify the relevant laboratory test questions from the initial set of 12,975 questions. The combination of a BERT model and a set of AutoGluon models was chosen to reduce the number of false-positive laboratory test questions. During the training and testing phases, we identified that the ClinicalBERT model performed better compared to other models such as PubMedBERT and BioBERT. Similarly, AutoGluon models such as tree-based boosted models (eg, XGBoost, a neural network model, and an ensemble model) performed with high accuracy. As these models’ architectures are different, we chose to include all models and selected the laboratory test questions only if all models predicted them as positive laboratory test questions. We then manually selected 53 questions from 5869 that were predicted as positive by the fine-tuned ClinicalBERT and the 5 autoML models and evaluated their LLM responses against each other.
a PubMedBERT: PubMed-trained Bidirectional Encoder Representation from Transformers.
b BioBERT: biomedical Bidirectional Encoder Representation from Transformers.
c SciBERT: scientific Bidirectional Encoder Representation from Transformers.
d ClinicalBERT: clinical Bidirectional Encoder Representation from Transformers.
e The highest value for the performance metric.
f AutoML: automated machine learning.
g XGBoost: Extreme Gradient Boosting.
Basic Characteristics of the Data Set of 53 Question-Answer Pairs
Figure 2 shows the responses from GPT-4 and Yahoo web-based users for an example laboratory result interpretation question from Yahoo! Answers. Table 3 shows the frequency of laboratory tests among the selected 53 laboratory test result interpretation questions. Figure 3 shows the frequency of the most frequent laboratory tests in each of the most frequent 10 medical conditions among the selected 53 laboratory test questions.

a HDL: high-density lipoprotein.

Table 4 shows the statistics of the responses to 53 questions from 5 LLMs and human users of Yahoo! Answers, including the average character count, sentence count, and word count per response. Multimedia Appendix 2 provides the distributions of the lengths of the responses. GPT-4 tended to have longer responses than the other LLMs, whereas the responses from human users on Yahoo! Answers tended to be shorter with respect to all 3 counts. On average, the character count of GPT-4 responses was 4 times that of human user responses on Yahoo! Answers.
Automated Comparison of Similarities in LLM Responses
Automatic metrics were used to compare the similarity of the responses generated by the 5 LLMs ( Figure 4 ), namely, BLEU, SacreBLEU, METEOR, ROUGE, and BERTScore. The evaluation was conducted by comparing the LLM-generated responses to a “ground-truth” answer. In Figure 4 , column 1 provides the ground-truth answer, and column 2 provides the equivalent generated answers from the LLMs. We also included the human answers from Yahoo! Answers for this evaluation. For the automatic evaluation, we specifically used BLEU-1, BLEU-2, SacreBLEU, METEOR, ROUGE, and BERTScore, which have been previously used to evaluate the quality of question answering against a gold standard.

All the metrics ranged from 0.0 to 1.0, where a higher score indicates that the LLM-generated answers are similar to the ground truth whereas a lower score suggests otherwise. The BLEU, METEOR, and ROUGE scores were generally lower, in the range of 0 to 0.37, whereas BERTScore values were generally higher, in the range of 0.46 to 0.63. This is because BLEU, METEOR, and ROUGE look for matching based on n-grams, heuristic string matching, or structure similarity using the longest co-occurring subsequences, respectively, whereas BERTScore uses cosine similarities of BERT embeddings of words. When GPT-4 was the reference answer, the response from GPT-3.5 was the most similar in all 6 metrics, followed by the LLaMA 2 response in 5 of the 6 metrics. Similarly, when GPT-3.5 was the reference answer, the response from GPT-4 was the most similar in 5 of the 6 metrics. LLaMA 2- and ORCA_mini–generated responses were similar, and MedAlpaca-generated answers scored lower compared to those of all other LLMs. Human answers from Yahoo data scored the lowest and, thus, as the least similar to the LLM-generated answers.
Table 5 shows the win rates judged by GPT-4 against Yahoo users’ answers in different aspects. Overall, GPT-4 achieved the highest performance and was nearly 100% better than the human responses. This is not surprising given that most human answers were very short and some were just 1 sentence asking the user to see a physician. GPT-4 and GPT-3.5 were followed by LLaMA 2 and ORCA_mini with 70% to 80% win rates. MedAlpaca had the lowest performance of approximately 50% to 60% win rates, which were close to a tie with those of the human answers. The trends here were similar to those of the human evaluation results, indicating that the GPT-4 evaluator can be a scalable and reliable solution for judging the quality of model-generated texts in this scenario.
Manual Evaluation With Medical Experts
Figure 5 illustrates the manual evaluation results of the LLM responses and human responses by 5 medical experts. Note that a lower value means a higher score. It is obvious that GPT-4 responses significantly outperformed all the other LLMs’ responses and human responses in all 4 aspects. Textbox 2 shows experts’ feedback on the LLM and human responses. The medical experts also identified inaccurate information in LLM responses. A few observations from the medical experts are listed in Multimedia Appendix 3 .

Large language model or human answer and expert feedback
- LLaMA 2: “It is a great answer. He was able to explain in details the results. He provides inside on the different differential diagnosis. And provide alternative a management. He shows empathy.”
- LLaMA 2: “Very thorough and thoughtful.”
- ORCA_mini: “It was a great answer. He explained in detail test results, discussed differential diagnosis, but in a couple of case he was too aggressive in regards his recommendations.”
- ORCA_mini: “Standard answers, not the most in depth.”
- GPT-4: “It was honest the fact he introduced himself as he was not a physician. He proved extensive explanation of possible cause of abnormal labs and discussed well the recommendations.”
- GPT-4: “Too wordy at times, gets irrelevant.”
- GPT-3.5: “Strong responses in general.”
- GPT-3.5: “Clear and some way informative and helpful to pts.”
- GPT-3.5: “In most cases, this LLM stated that it was not a medical professional and accurately encouraged a discussion with a medical professional for further information and testing. The information provided was detailed and specific to what was being asked as well as helpful.”
- MedAlpaca: “This statement seems so sure that he felt superficial. It made me feel he did not provide enough information. It felt not safe for the patient.”
- MedAlpaca: “Short and succinct. condescending at times.”
- Human answer: “These were not very helpful or accurate. Most did not state their credentials to know how credible they are. Some of the, if not most, of language learning models gave better answers, though some of the language learning models also claimed to be medical professionals—which isn’t accurate statement either.”
- Human answer: “Usually focused on one aspect of the scenario, not helpful in comprehensive care. focused on isolated lab value, with minimal evidence—these can be harmful responses for patients.”
- Human answer: “These are really bad answers.”
- Human answer: “Some of the answer were helpful, other not much, and other offering options that might not need to be indicated.”
Principal Findings
This study evaluated the feasibility of using generative LLMs to answer patients’ laboratory test result questions using 53 patients’ questions on a social Q&A website, Yahoo! Answers. On the basis of the results of our study, GPT-4 outperformed other similar LLMs (ie, GPT-3.5, LLaMA 2, ORCA_mini, and MedAlpaca) according to both automated metrics and manual evaluation. In particular, GPT-4 always provided disclaimers, possibly to avoid legal issues. However, GPT-4 responses may also suffer from lack of interpretation of one’s medical context, incorrect statements, and lack of references.
Recent studies [ 26 , 27 ] regarding the use of LLMs to answer laboratory test result questions from medical professionals found that ChatGPT may give superficial or incorrect answers to laboratory test result–related questions and can only provide accurate answers to approximately 50% of questions [ 26 ]. They also found that ChatGPT’s responses were not sufficiently tailored to the case or clinical questions to be useful for clinical consultation. For instance, diagnoses of liver injury were made solely based on γ-glutamyl transferase levels without considering other liver enzyme indicators. In addition, high levels of glucose and glycated hemoglobin (HbA 1c ) were both identified as indicative of diabetes regardless of whether HbA 1c levels were normal or elevated. These studies also highlighted that GPT-4 failed to account for preanalytical factors such as fasting status for glucose tests and struggled to differentiate between abnormal and critically abnormal laboratory test values. Our study observed similar patterns, where a normal HbA 1c level coupled with high glucose levels led to a diabetes prediction and critically low iron levels were merely classified as abnormal.
In addition, our findings also show that GPT-4 accurately distinguished between normal, prediabetic, and diabetic HbA 1c ranges considering fasting glucose levels and preanalytical conditions such as fasting status. Furthermore, in cases of elevated bilirubin levels, GPT-4 correctly associated them with potential jaundice citing the patient’s yellow eye discoloration and appropriately considered a comprehensive set of laboratory test results—including elevated liver enzymes and bilirubin levels—and significant alcohol intake history to recommend diagnoses such as alcoholic liver disease, hepatitis, bile duct obstruction, and liver cancer.
On the basis of our observation with the limited number of questions, we found that patients’ questions are often less complex than professionals’ questions, making ChatGPT more likely to provide an adequately accurate answer to such questions. In our manual evaluation of 7 selected patients’ laboratory test result questions, 91% (32/35) of the ratings from 5 medical experts on GPT-4’s response accuracy were either 1 ( very high ) or 2 ( high ).
Through this study, we gained insights into the challenges of using generative LLMs to answer patients’ laboratory test result–related questions and provide suggestions to mitigate these challenges. First, when asking laboratory test result questions on social Q&A websites, patients tend to focus on laboratory test results but may not provide pertinent information needed for result interpretation. In the real-world clinical setting, to fully evaluate the results, clinicians may need to evaluate the medical history of a patient and examine the trends of the laboratory test results over time. This shows that, to allow LLMs to provide a more thorough evaluation of laboratory test results, the question prompts may need to be augmented with additional information. As such, LLMs could be useful in prompting patients to provide additional information. A possible question prompt would be the following: “What additional information or data would you need to provide a more accurate diagnosis for me?”
Second, we found that it is important to understand the limitations of LLMs when answering laboratory test–related questions. As general-purpose generative AI models, they should be used to explain common terminologies and test purposes; clarify the typical reference ranges for common laboratory tests and what it might mean to have values outside these ranges; and offer general interpretation of laboratory test results, such as what it might mean to have high or low levels in certain common laboratory tests. On the basis of our findings, LLMs, especially GPT-4, can provide a basic interpretation of laboratory test results without reference ranges in the question prompts. LLMs could also be used to suggest what questions to ask health care providers. They should not be used for diagnostic purposes or treatment advice. All laboratory test results should be interpreted by a health care professional who can consider the full context of one’s health. For providers, LLMs could also be used as an educational tool for laboratory professionals, providing real-time information and explanations of laboratory techniques. When using LLMs for laboratory test result interpretation, it is important to consider the ethical and practical implications, including data privacy, the need for human oversight, and the potential for AI to both enhance and disrupt clinical workflows.
Third, we found it challenging to evaluate laboratory test result questions using Q&A pairs from social Q&A websites such as Yahoo! Answers. This is mainly because the answers provided by web-based users (who may not be medical professionals) were generally short, often focused on one aspect of the question or isolated laboratory tests, possibly opinionated, and possibly inaccurate with minimal evidence. Therefore, it is unlikely that human answers from social Q&A websites can be used as a gold standard to evaluate LLM answers. We found that GPT-4 can provide comprehensive, thoughtful, sympathetic, and fairly accurate interpretation of individual laboratory tests, but it still suffers from a number of problems: (1) LLM answers are not individualized, (2) it is not clear what are the sources LLMs use to generate the answers, (3) LLMs do not ask clarifying questions if the provided prompts do not contain important information for LLMs to generate responses, and (4) validation by medical experts is needed to reduce hallucination and fill in missing information to ensure the quality of the responses.
Future Directions
We would like to point out a few ways to improve the quality of LLM responses to laboratory test–related questions. First, the interpretation of certain laboratory tests is dependent on age group, gender, and possibly other conditions pertaining to particular population subgroups (eg, pregnant women), but LLMs do not ask clarifying questions, so it is important to enrich the question prompts with necessary information available in electronic health records or ask patients to provide necessary information for more accurate interpretation. Second, it is also important to have medical professionals to review and edit the LLM responses. For example, we found that LLaMA 2 self-identified as a “health expert,” which is obviously problematic if such responses were directly sent to patients. Therefore, it is important to postprocess the responses to highlight sentences that are risky. Third, LLMs are sensitive to question prompts. We could study different prompt engineering and structuring strategies (eg, role prompting and chain of thought) and evaluate whether these prompting approaches would improve the quality of the answers. Fourth, one could also collect clinical guidelines that provide credible laboratory result interpretation to further train LLMs to improve answer quality. We could then leverage the retrieval-augmented generation approach to allow LLMs to generate responses from a limited set of credible information sources [ 52 ]. Fifth, we could evaluate the confidence level of the sentences in the responses. Sixth, a gold-standard benchmark Q&A data set for laboratory result interpretation could be developed to allow the community to advance with different augmentation approaches.
Limitations
A few limitations should be noted in this study. First, the ChatGPT web version is nondeterministic in that the same prompt may generate different responses when used by different users. Second, the sample size for the human evaluation was small. Nonetheless, this study produced evidence that LLMs such as GPT-4 can be a promising tool for filling the information gap for understanding laboratory tests and various approaches can be used to enhance the quality of the responses.
Conclusions
In this study, we evaluated the feasibility of using generative LLMs to answer common laboratory test result interpretation questions from patients. We generated responses from 5 LLMs—ChatGPT (GPT-4 version and GPT-3.5 version), LLaMA 2, MedAlpaca, and ORCA_mini—for laboratory test questions selected from Yahoo! Answers and evaluated these responses using both automated metrics and manual evaluation. We found that GPT-4 performed better compared to the other LLMs in generating more accurate, helpful, relevant, and safe answers to these questions. We also identified a number of ways to improve the quality of LLM responses from both the prompt and response sides.
Acknowledgments
This project was partially supported by the University of Florida Clinical and Translational Science Institute, which is supported in part by the National Institutes of Health (NIH) National Center for Advancing Translational Sciences under award UL1TR001427, as well as the Agency for Healthcare Research and Quality (AHRQ) under award R21HS029969. This study was supported by the NIH Intramural Research Program, National Library of Medicine (QJ and ZL). The content is solely the responsibility of the authors and does not necessarily represent the official views of the NIH and AHRQ. The authors would like to thank Angelique Deville, Caroline Bennett, Hailey Thompson, and Maggie Awad for labeling the questions for the question classification model.
Data Availability
The data sets generated during and analyzed during this study are available from the corresponding author on reasonable request.
Conflicts of Interest
QJ is a coauthor and an active associate editor for the Journal of Medical Internet Research . All other authors declare no other conflicts of interest.
The responses generated by the 5 large language models and the human answers from Yahoo users.
Distribution of the lengths of the responses.
A few observations from the medical experts regarding the accuracy of the large language model responses.
- Healthy people 2030: building a healthier future for all. Office of Disease Prevention and Health Promotion. URL: https://health.gov/healthypeople [accessed 2023-05-09]
- NHE fact sheet. Centers for Medicare & Medicaid Services. URL: https://tinyurl.com/yc4durw4 [accessed 2023-06-06]
- Bauer UE, Briss PA, Goodman RA, Bowman BA. Prevention of chronic disease in the 21st century: elimination of the leading preventable causes of premature death and disability in the USA. Lancet. Jul 2014;384(9937):45-52. [ CrossRef ]
- Centers for Medicare and Medicaid Services (CMS), Centers for Disease Control and Prevention (CDC), Office for Civil Rights (OCR). CLIA program and HIPAA privacy rule; patients' access to test reports. Final rule. Fed Regist. Feb 06, 2014;79(25):7289-7316. [ FREE Full text ] [ Medline ]
- Pillemer F, Price R, Paone S, Martich GD, Albert S, Haidari L, et al. Direct release of test results to patients increases patient engagement and utilization of care. PLoS One. 2016;11(6):e0154743. [ FREE Full text ] [ CrossRef ] [ Medline ]
- Health IT legislation: 21st century cures act. Office of the National Coordinator for Health Information Technology. URL: https://www.healthit.gov/topic/laws-regulation-and-policy/health-it-legislation [accessed 2023-02-19]
- Tsai R, Bell EJ, Woo H, Baldwin K, Pfeffer M. How patients use a patient portal: an institutional case study of demographics and usage patterns. Appl Clin Inform. Jan 06, 2019;10(1):96-102. [ FREE Full text ] [ CrossRef ] [ Medline ]
- Witteman HO, Zikmund-Fisher BJ. Communicating laboratory results to patients and families. Clin Chem Lab Med. Feb 25, 2019;57(3):359-364. [ FREE Full text ] [ CrossRef ] [ Medline ]
- Turchioe MR, Myers A, Isaac S, Baik D, Grossman LV, Ancker JS, et al. A systematic review of patient-facing visualizations of personal health data. Appl Clin Inform. Aug 09, 2019;10(4):751-770. [ FREE Full text ] [ CrossRef ] [ Medline ]
- Alpert JM, Krist AH, Aycock RA, Kreps GL. Applying multiple methods to comprehensively evaluate a patient portal's effectiveness to convey information to patients. J Med Internet Res. May 17, 2016;18(5):e112. [ FREE Full text ] [ CrossRef ] [ Medline ]
- Zikmund-Fisher BJ, Exe NL, Witteman HO. Numeracy and literacy independently predict patients' ability to identify out-of-range test results. J Med Internet Res. Aug 08, 2014;16(8):e187. [ FREE Full text ] [ CrossRef ] [ Medline ]
- Zhang Z, Citardi D, Xing A, Luo X, Lu Y, He Z. Patient challenges and needs in comprehending laboratory test results: mixed methods study. J Med Internet Res. Dec 07, 2020;22(12):e18725. [ FREE Full text ] [ CrossRef ] [ Medline ]
- Fraccaro P, Vigo M, Balatsoukas P, van der Veer SN, Hassan L, Williams R, et al. Presentation of laboratory test results in patient portals: influence of interface design on risk interpretation and visual search behaviour. BMC Med Inform Decis Mak. Feb 12, 2018;18(1):11. [ FREE Full text ] [ CrossRef ] [ Medline ]
- Bar-Lev S, Beimel D. Numbers, graphs and words - do we really understand the lab test results accessible via the patient portals? Isr J Health Policy Res. Oct 28, 2020;9(1):58. [ FREE Full text ] [ CrossRef ] [ Medline ]
- Giardina TD, Baldwin J, Nystrom DT, Sittig DF, Singh H. Patient perceptions of receiving test results via online portals: a mixed-methods study. J Am Med Inform Assoc. Apr 01, 2018;25(4):440-446. [ FREE Full text ] [ CrossRef ] [ Medline ]
- Doi T, Langsted A, Nordestgaard BG. Elevated remnant cholesterol reclassifies risk of ischemic heart disease and myocardial infarction. J Am Coll Cardiol. Jun 21, 2022;79(24):2383-2397. [ FREE Full text ] [ CrossRef ] [ Medline ]
- Wadström BN, Wulff AB, Pedersen KM, Jensen GB, Nordestgaard BG. Elevated remnant cholesterol increases the risk of peripheral artery disease, myocardial infarction, and ischaemic stroke: a cohort-based study. Eur Heart J. Sep 07, 2022;43(34):3258-3269. [ CrossRef ] [ Medline ]
- Chu SK, Huang H, Wong WN, van Ginneken WF, Wu KM, Hung MY. Quality and clarity of health information on Q and A sites. Libr Inf Sci Res. Jul 2018;40(3-4):237-244. [ CrossRef ]
- Oh S, Yi YJ, Worrall A. Quality of health answers in social Q and A. Proc Assoc Inf Sci Technol. Jan 24, 2013;49(1):1-6. [ CrossRef ]
- Tao D, Yuan J, Qu X, Wang T, Chen X. Presentation of personal health information for consumers: an experimental comparison of four visualization formats. In: Proceedings of the 15th International Conference on Engineering Psychology and Cognitive Ergonomics. 2018. Presented at: EPCE '18; July 15-20, 2018;490-500; Las Vegas, NV. URL: https://link.springer.com/chapter/10.1007/978-3-319-91122-9_40
- Struikman B, Bol N, Goedhart A, van Weert JC, Talboom-Kamp E, van Delft S, et al. Features of a patient portal for blood test results and patient health engagement: web-based pre-post experiment. J Med Internet Res. Jul 20, 2020;22(7):e15798. [ FREE Full text ] [ CrossRef ] [ Medline ]
- Kopanitsa G. Study of patients' attitude to automatic interpretation of laboratory test results and its influence on follow-up rate. BMC Med Inform Decis Mak. Mar 27, 2022;22(1):79. [ FREE Full text ] [ CrossRef ] [ Medline ]
- Zikmund-Fisher BJ, Scherer AM, Witteman HO, Solomon JB, Exe NL, Fagerlin A. Effect of harm anchors in visual displays of test results on patient perceptions of urgency about near-normal values: experimental study. J Med Internet Res. Mar 26, 2018;20(3):e98. [ FREE Full text ] [ CrossRef ] [ Medline ]
- Morrow D, Azevedo RF, Garcia-Retamero R, Hasegawa-Johnson M, Huang T, Schuh W, et al. Contextualizing numeric clinical test results for gist comprehension: implications for EHR patient portals. J Exp Psychol Appl. Mar 2019;25(1):41-61. [ CrossRef ] [ Medline ]
- Tian S, Jin Q, Yeganova L, Lai PT, Zhu Q, Chen X, et al. Opportunities and challenges for ChatGPT and large language models in biomedicine and health. Brief Bioinform. Nov 22, 2023;25(1):bbad493. [ CrossRef ] [ Medline ]
- Cadamuro J, Cabitza F, Debeljak Z, De Bruyne SD, Frans G, Perez SM, et al. Potentials and pitfalls of ChatGPT and natural-language artificial intelligence models for the understanding of laboratory medicine test results. An assessment by the European Federation of Clinical Chemistry and Laboratory Medicine (EFLM) working group on artificial intelligence (WG-AI). Clin Chem Lab Med. Jun 27, 2023;61(7):1158-1166. [ FREE Full text ] [ CrossRef ] [ Medline ]
- Munoz-Zuluaga C, Zhao Z, Wang F, Greenblatt MB, Yang HS. Assessing the accuracy and clinical utility of ChatGPT in laboratory medicine. Clin Chem. Aug 02, 2023;69(8):939-940. [ CrossRef ] [ Medline ]
- Zhang Z, Lu Y, Kou Y, Wu DT, Huh-Yoo J, He Z. Understanding patient information needs about their clinical laboratory results: a study of social Q and A site. Stud Health Technol Inform. Aug 21, 2019;264:1403-1407. [ FREE Full text ] [ CrossRef ] [ Medline ]
- Zhang Z, Lu Y, Wilson C, He Z. Making sense of clinical laboratory results: an analysis of questions and replies in a social Q and A community. Stud Health Technol Inform. Aug 21, 2019;264:2009-2010. [ CrossRef ] [ Medline ]
- Kurstjens S, Schipper A, Krabbe J, Kusters R. Predicting hemoglobinopathies using ChatGPT. Clin Chem Lab Med. Feb 26, 2024;62(3):e59-e61. [ FREE Full text ] [ CrossRef ] [ Medline ]
- He Z, Tian S, Erdengasileng A, Hanna K, Gong Y, Zhang Z, et al. Annotation and information extraction of consumer-friendly health articles for enhancing laboratory test reporting. AMIA Annu Symp Proc. 2023;2023:407-416. [ FREE Full text ] [ Medline ]
- Lee J, Yoon W, Kim S, Kim D, Kim S, So CH, et al. BioBERT: a pre-trained biomedical language representation model for biomedical text mining. Bioinformatics. Feb 15, 2020;36(4):1234-1240. [ FREE Full text ] [ CrossRef ] [ Medline ]
- Alsentzer E, Murphy JR, Boag W, Weng WH, Jin D, Naumann T, et al. Publicly available clinical BERT embeddings. arXiv. Preprint posted online on April 6, 2019. [ FREE Full text ] [ CrossRef ]
- Beltagy I, Lo K, Cohan A. SciBERT: a pretrained language model for scientific text. arXiv. Preprint posted online on March 26, 2019. [ FREE Full text ]
- Gu Y, Tinn R, Cheng H, Lucas M, Usuyama N, Liu X, et al. Domain-specific language model pretraining for biomedical natural language processing. ACM Trans Comput Healthc. Oct 15, 2021;3(1):1-23. [ FREE Full text ] [ CrossRef ]
- OpenAI. GPT-4 technical report. arXiv. Preprint posted online on March 15, 2023. [ FREE Full text ]
- Ye J, Chen X, Xu N, Zu C, Shao Z, Liu S, et al. A comprehensive capability analysis of GPT-3 and GPT-3. arXiv. Preprint posted online on March 18, 2023. [ FREE Full text ]
- Touvron H, Martin L, Stone K, Albert P, Almahairi A, Babaei Y, et al. Llama 2: open foundation and fine-tuned chat models. arXiv. Preprint posted online on July 18, 2023. [ FREE Full text ]
- Han T, Adams LC, Papaioannou JM, Grundmann P, Oberhauser T, Löser A, et al. MedAlpaca -- an open-source collection of medical conversational AI models and training data. arXiv. Preprint posted online on April 14, 2023. [ FREE Full text ]
- orca_mini_3b. Hugging Face. URL: https://huggingface.co/pankajmathur/orca_mini_3b [accessed 2023-12-04]
- LangChain: introduction and getting started. Pinecone. URL: https://www.pinecone.io/learn/series/langchain/langchain-intro/ [accessed 2023-12-04]
- Papineni K, Roukos S, Ward T, Zhu WJ. BLEU: a method for automatic evaluation of machine translation. In: Proceedings of the 40th Annual Meeting on Association for Computational Linguistics. 2002. Presented at: ALC '02; July 7-12, 2002;311-318; Philadelphia, PA. URL: https://dl.acm.org/doi/10.3115/1073083.1073135 [ CrossRef ]
- Post M. A call for clarity in reporting BLEU scores. arXiv. Preprint posted online on April 23, 2018. [ FREE Full text ] [ CrossRef ]
- Banerjee S, Lavie A. METEOR: an automatic metric for MT evaluation with high levels of correlation with human judgments. In: Proceedings of the 2005 ACL Workshop on Intrinsic and Extrinsic Evaluation Measures for Machine Translation and/or Summarization. 2005. Presented at: WIEEMMTS '05; June 29, 2005;65-72; Ann Arbor, MI. URL: https://aclanthology.org/W05-0909 [ CrossRef ]
- Lin CY. ROUGE: a package for automatic evaluation of summaries. In: Lin CY, editor. Text Summarization Branches Out Internet. Barcelona, Spain. Association for Computational Linguistics; 2004;74-81.
- Zhang T, Kishore V, Wu F, Weinberger KQ, Artzi Y. BERTScore: evaluating text generation with BERT. arXiv. Preprint posted online on April 21, 2019. [ FREE Full text ]
- Wang T, Yu P, Tan XE, O'Brien S, Pasunuru R, Dwivedi-Yu J, et al. Shepherd: a critic for language model generation. arXiv. Preprint posted online on August 8, 2023. [ FREE Full text ]
- Dubois Y, Li X, Taori R, Zhang T, Gulrajani I, Ba J, et al. AlpacaFarm: a simulation framework for methods that learn from human feedback. arXiv. Preprint posted online on May 22, 2023. [ FREE Full text ]
- Bartko JJ. The intraclass correlation coefficient as a measure of reliability. Psychol Rep. Aug 1966;19(1):3-11. [ FREE Full text ] [ CrossRef ] [ Medline ]
- Gamer M, Lemon J, Singh IF. irr: various coefficients of interrater reliability and agreement. Cran R Project. 2019. URL: https://cran.r-project.org/web/packages/irr/index.html [accessed 2023-12-12]
- Human subject regulations decision charts: 2018 requirements. Office for Human Research Protection. Jan 20, 2019. URL: https://tinyurl.com/3sbzydm3 [accessed 2024-04-03]
- Jin Q, Leaman R, Lu Z. Retrieve, summarize, and verify: how will ChatGPT affect information seeking from the medical literature? J Am Soc Nephrol. Aug 01, 2023;34(8):1302-1304. [ CrossRef ] [ Medline ]
Abbreviations
Edited by B Puladi; submitted 23.01.24; peer-reviewed by Y Chen, Z Smutny; comments to author 01.02.24; revised version received 17.02.24; accepted 06.03.24; published 17.04.24.
©Zhe He, Balu Bhasuran, Qiao Jin, Shubo Tian, Karim Hanna, Cindy Shavor, Lisbeth Garcia Arguello, Patrick Murray, Zhiyong Lu. Originally published in the Journal of Medical Internet Research (https://www.jmir.org), 17.04.2024.
This is an open-access article distributed under the terms of the Creative Commons Attribution License (https://creativecommons.org/licenses/by/4.0/), which permits unrestricted use, distribution, and reproduction in any medium, provided the original work, first published in the Journal of Medical Internet Research, is properly cited. The complete bibliographic information, a link to the original publication on https://www.jmir.org/, as well as this copyright and license information must be included.
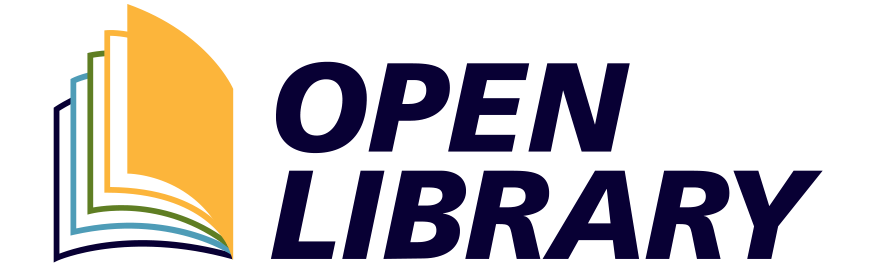
Want to create or adapt books like this? Learn more about how Pressbooks supports open publishing practices.
C.1.1 Formatting a Research Paper
Learning objectives.
- Identify the major components of a research paper written using American Psychological Association (APA) style.
- Apply general APA style and formatting conventions in a research paper.
In this chapter, you will learn how to use APA style , the documentation and formatting style followed by the American Psychological Association, as well as MLA style , from the Modern Language Association. There are a few major formatting styles used in academic texts, including AMA, Chicago, and Turabian:
- AMA (American Medical Association) for medicine, health, and biological sciences
- APA (American Psychological Association) for education, psychology, and the social sciences
- Chicago —a common style used in everyday publications like magazines, newspapers, and books
- MLA (Modern Language Association) for English, literature, arts, and humanities
- Turabian —another common style designed for its universal application across all subjects and disciplines
While all the formatting and citation styles have their own use and applications, in this chapter we focus our attention on the two styles you are most likely to use in your academic studies: APA and MLA.
If you find that the rules of proper source documentation are difficult to keep straight, you are not alone. Writing a good research paper is, in and of itself, a major intellectual challenge. Having to follow detailed citation and formatting guidelines as well may seem like just one more task to add to an already-too-long list of requirements.
Following these guidelines, however, serves several important purposes. First, it signals to your readers that your paper should be taken seriously as a student’s contribution to a given academic or professional field; it is the literary equivalent of wearing a tailored suit to a job interview. Second, it shows that you respect other people’s work enough to give them proper credit for it. Finally, it helps your reader find additional materials if he or she wishes to learn more about your topic.
Furthermore, producing a letter-perfect APA-style paper need not be burdensome. Yes, it requires careful attention to detail. However, you can simplify the process if you keep these broad guidelines in mind:
- Work ahead whenever you can. You can consult the chapter, “ Writing from Research: What Will I Learn? “ from the original version of this textbook ( intentionally omitted ), which includes tips for keeping track of your sources early in the research process, which will save time later on.
- Get it right the first time. Apply APA guidelines as you write, so you will not have much to correct during the editing stage. Again, putting in a little extra time early on can save time later.
- Use the resources available to you. In addition to the guidelines provided in this chapter, you may wish to consult the APA website at http://www.apa.org or the Purdue University Online Writing lab at http://owl.english.purdue.edu , which regularly updates its online style guidelines.
- Consult the Fanshawe College Library website section “ ACADEMIC WRITING & CITATION ” for additional resources on research and citation.
General Formatting Guidelines
This chapter provides detailed guidelines for using the citation and formatting conventions developed by the American Psychological Association, or APA. Writers in disciplines as diverse as astrophysics, biology, psychology, and education follow APA style. The major components of a paper written in APA style are listed in the following box.
These are the major components of an APA-style paper:
Body, which includes the following:
- Headings and, if necessary, subheadings to organize the content
- In-text citations of research sources
- References page
All these components must be saved in one document, not as separate documents.
The title page of your paper includes the following information:
- Title of the paper
- Author’s name
- Name of the institution with which the author is affiliated
- Header at the top of the page with the paper title (in capital letters) and the page number (If the title is lengthy, you may use a shortened form of it in the header.)
List the first three elements in the order given in the previous list, centered about one third of the way down from the top of the page. Use the headers and footers tool of your word-processing program to add the header, with the title text at the left and the page number in the upper-right corner. Your title page should look like the following example.
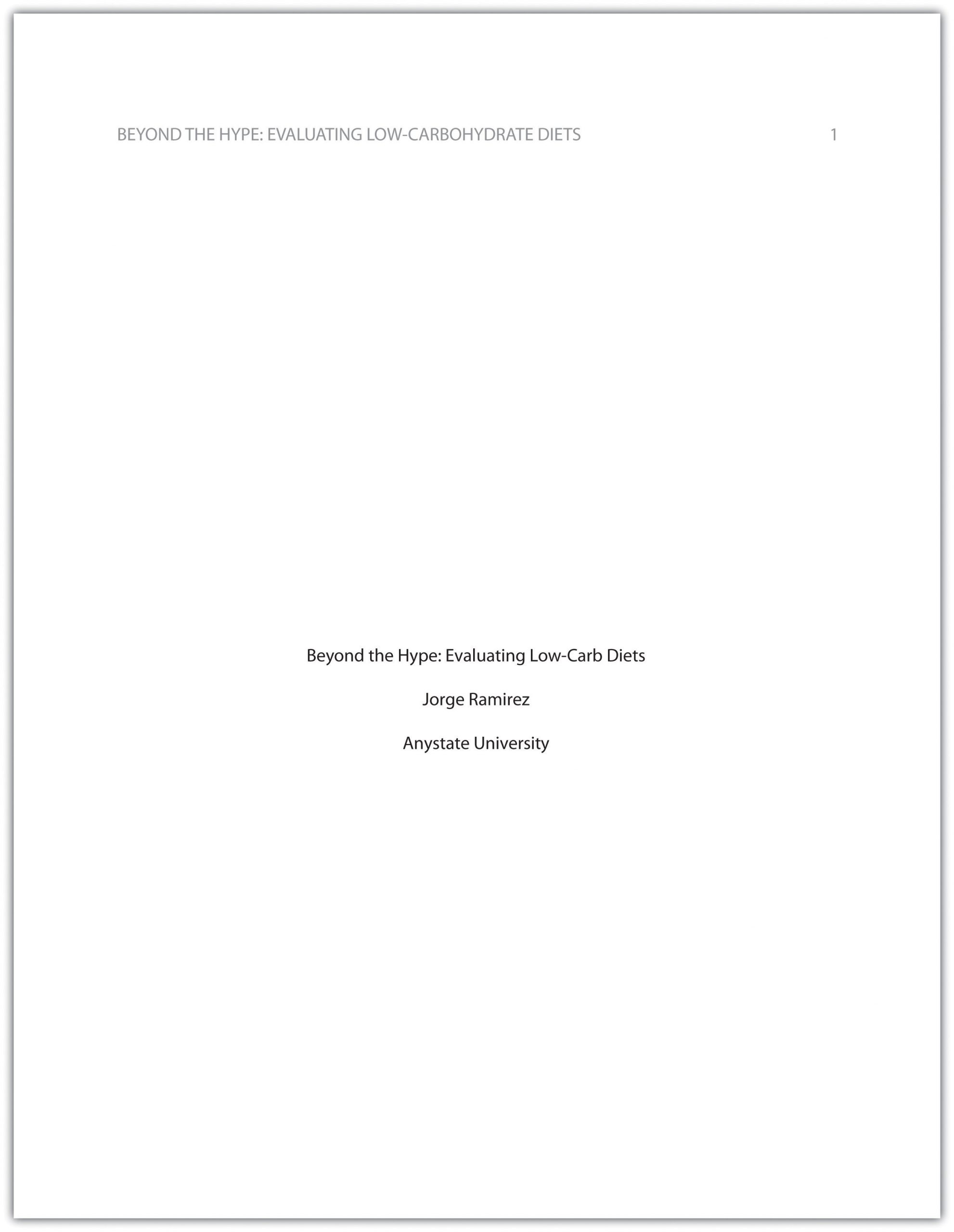
The next page of your paper provides an abstract , or brief summary of your findings. An abstract does not need to be provided in every paper, but an abstract should be used in papers that include a hypothesis. A good abstract is concise—about one hundred fifty to two hundred fifty words—and is written in an objective, impersonal style. Your writing voice will not be as apparent here as in the body of your paper. When writing the abstract, take a just-the-facts approach, and summarize your research question and your findings in a few sentences.
Below is a sample paper written by a student named Jorge, who researched the effectiveness of low-carbohydrate diets. Read Jorge’s abstract. Note how it sums up the major ideas in his paper without going into excessive detail.
To view the original draft of this paper, you can review the chapter entitled, “ Creating a Rough Draft for a Research Paper ” from the original version of this textbook ( intentionally omitted ).
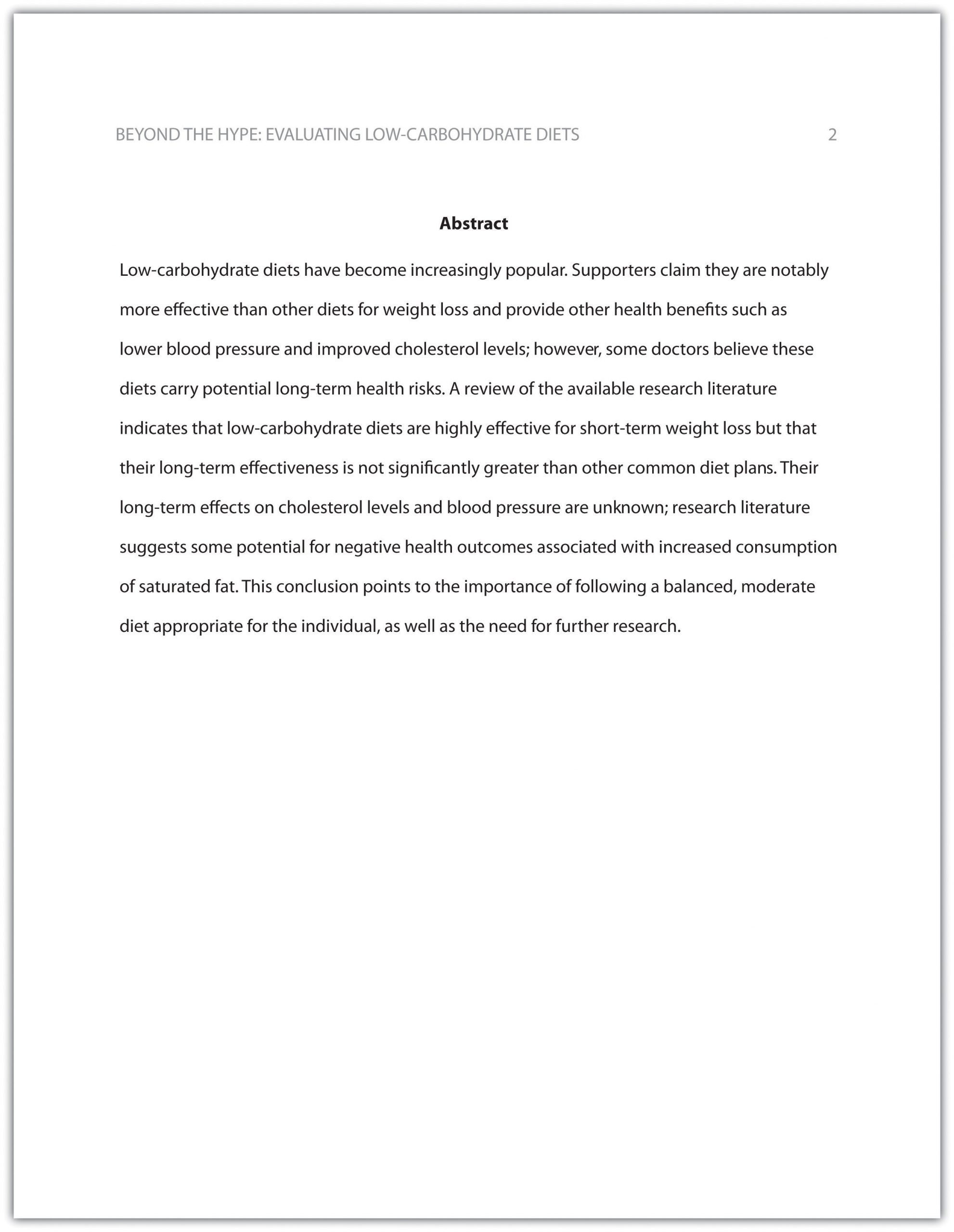
Write an abstract summarizing your paper. Briefly introduce the topic, state your findings, and sum up what conclusions you can draw from your research. Use the word count feature of your word-processing program to make sure your abstract does not exceed one hundred fifty words.
Depending on your field of study, you may sometimes write research papers that present extensive primary research, such as your own experiment or survey. In your abstract, summarize your research question and your findings, and briefly indicate how your study relates to prior research in the field.
Margins, Pagination, and Headings
APA style requirements also address specific formatting concerns, such as margins, pagination, and heading styles, within the body of the paper. Review the following APA guidelines.
Use these general guidelines to format the paper:
- Set the top, bottom, and side margins of your paper at 1 inch.
- Use double-spaced text throughout your paper.
- Use a standard font, such as Times New Roman or Arial, in a legible size (10- to 12-point).
- Use continuous pagination throughout the paper, including the title page and the references section. Page numbers appear flush right within your header.
- Section headings and subsection headings within the body of your paper use different types of formatting depending on the level of information you are presenting. Additional details from Jorge’s paper are provided.
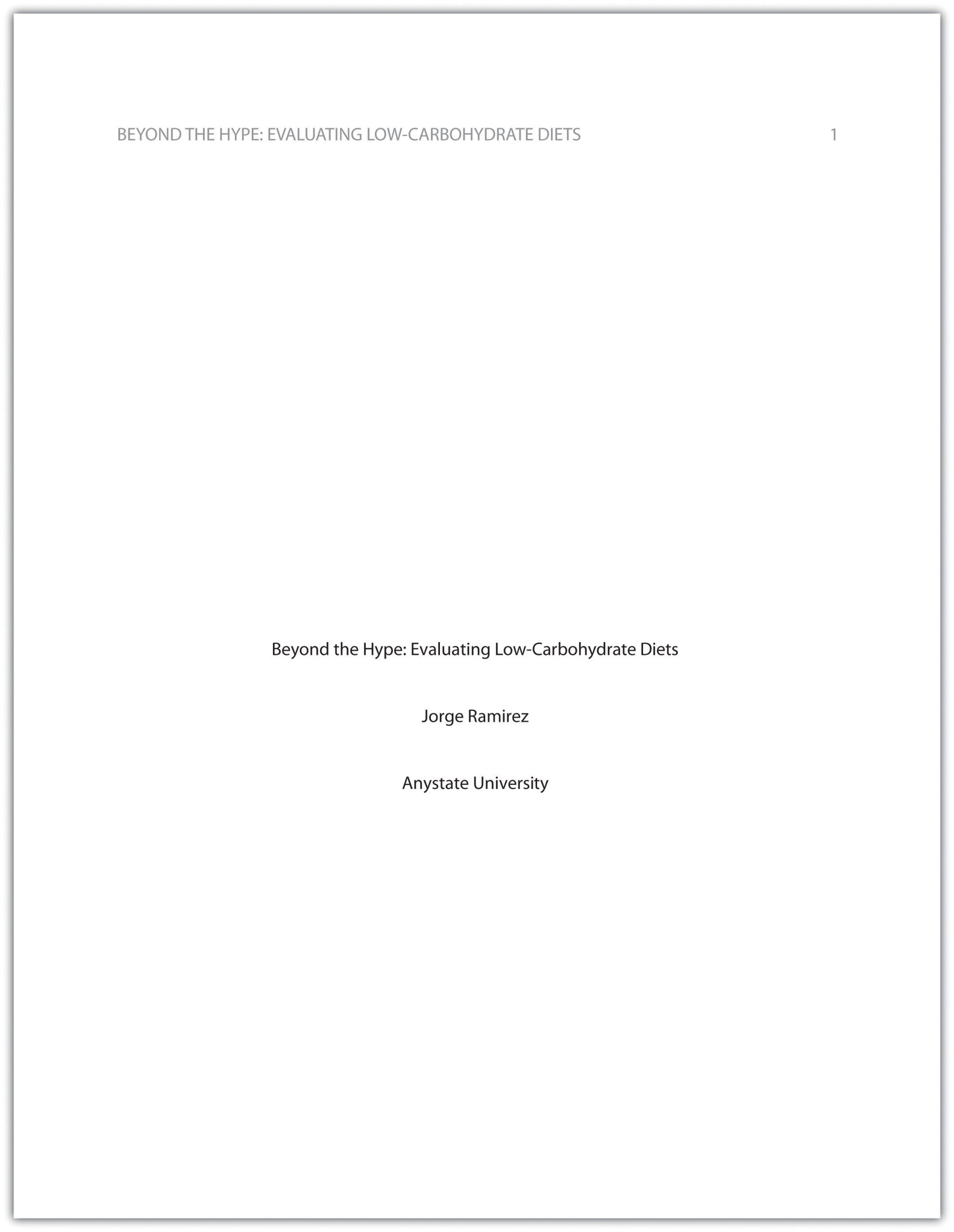
Begin formatting the final draft of your paper according to APA guidelines. You may work with an existing document or set up a new document if you choose. Include the following:
- Your title page
- The abstract you created in “Exercise 1”
- Correct headers and page numbers for your title page and abstract
APA style uses section headings to organize information, making it easy for the reader to follow the writer’s train of thought and to know immediately what major topics are covered. Depending on the length and complexity of the paper, its major sections may also be divided into subsections, sub-subsections, and so on. These smaller sections, in turn, use different heading styles to indicate different levels of information. In essence, you are using headings to create a hierarchy of information.
The following heading styles used in APA formatting are listed in order of greatest to least importance:
- Section headings use centered, boldface type. Headings use title case, with important words in the heading capitalized.
- Subsection headings use left-aligned, boldface type. Headings use title case.
- The third level uses left-aligned, indented, boldface type. Headings use a capital letter only for the first word, and they end in a period.
- The fourth level follows the same style used for the previous level, but the headings are boldfaced and italicized.
- The fifth level follows the same style used for the previous level, but the headings are italicized and not boldfaced.
Visually, the hierarchy of information is organized as indicated in Table C.1.1 “Section Headings” .
Table C.1.1 Section Headings
A college research paper may not use all the heading levels shown in Table C.1.1 “Section Headings” , but you are likely to encounter them in academic journal articles that use APA style. For a brief paper, you may find that level 1 headings suffice. Longer or more complex papers may need level 2 headings or other lower-level headings to organize information clearly. Use your outline to craft your major section headings and determine whether any subtopics are substantial enough to require additional levels of headings.
Working with the document you developed in “Exercise 2” , begin setting up the heading structure of the final draft of your research paper according to APA guidelines. Include your title and at least two to three major section headings, and follow the formatting guidelines provided above. If your major sections should be broken into subsections, add those headings as well. Use your outline to help you.
Because Jorge used only level 1 headings, his Exercise 3 would look like the following:
Citation Guidelines
In-text citations.
Throughout the body of your paper, include a citation whenever you quote or paraphrase material from your research sources. The purpose of citations is twofold: to give credit to others for their ideas and to allow your reader to follow up and learn more about the topic if desired. Your in-text citations provide basic information about your source; each source you cite will have a longer entry in the references section that provides more detailed information.
In-text citations must provide the name of the author or authors and the year the source was published. (When a given source does not list an individual author, you may provide the source title or the name of the organization that published the material instead.) When directly quoting a source, it is also required that you include the page number where the quote appears in your citation.
This information may be included within the sentence or in a parenthetical reference at the end of the sentence, as in these examples.
Epstein (2010) points out that “junk food cannot be considered addictive in the same way that we think of psychoactive drugs as addictive” (p. 137).
Here, the writer names the source author when introducing the quote and provides the publication date in parentheses after the author’s name. The page number appears in parentheses after the closing quotation marks and before the period that ends the sentence.
Addiction researchers caution that “junk food cannot be considered addictive in the same way that we think of psychoactive drugs as addictive” (Epstein, 2010, p. 137).
Here, the writer provides a parenthetical citation at the end of the sentence that includes the author’s name, the year of publication, and the page number separated by commas. Again, the parenthetical citation is placed after the closing quotation marks and before the period at the end of the sentence.
As noted in the book Junk Food, Junk Science (Epstein, 2010, p. 137), “junk food cannot be considered addictive in the same way that we think of psychoactive drugs as addictive.”
Here, the writer chose to mention the source title in the sentence (an optional piece of information to include) and followed the title with a parenthetical citation. Note that the parenthetical citation is placed before the comma that signals the end of the introductory phrase.
David Epstein’s book Junk Food, Junk Science (2010) pointed out that “junk food cannot be considered addictive in the same way that we think of psychoactive drugs as addictive” (p. 137).
Another variation is to introduce the author and the source title in your sentence and include the publication date and page number in parentheses within the sentence or at the end of the sentence. As long as you have included the essential information, you can choose the option that works best for that particular sentence and source.
Citing a book with a single author is usually a straightforward task. Of course, your research may require that you cite many other types of sources, such as books or articles with more than one author or sources with no individual author listed. You may also need to cite sources available in both print and online and nonprint sources, such as websites and personal interviews.
The rest of this chapter provides extensive guidelines for citing a variety of source types.
Writing at Work
APA is just one of several different styles with its own guidelines for documentation, formatting, and language usage. Depending on your field of interest, you may be exposed to additional styles, such as the following:
- MLA style. Determined by the Modern Languages Association and used for papers in literature, languages, and other disciplines in the humanities.
- Chicago style. Outlined in the Chicago Manual of Style and sometimes used for papers in the humanities and the sciences; many professional organizations use this style for publications as well.
- Associated Press (AP) style. Used by professional journalists.
References List
The brief citations included in the body of your paper correspond to the more detailed citations provided at the end of the paper in the references section. In-text citations provide basic information—the author’s name, the publication date, and the page number if necessary—while the references section provides more extensive bibliographical information. Again, this information allows your reader to follow up on the sources you cited and do additional reading about the topic if desired.
The specific format of entries in the list of references varies slightly for different source types, but the entries generally include the following information:
- The name(s) of the author(s) or institution that wrote the source
- The year of publication and, where applicable, the exact date of publication
- The full title of the source
- For books, the city of publication
- For articles or essays, the name of the periodical or book in which the article or essay appears
- For magazine and journal articles, the volume number, issue number, and pages where the article appears
- For sources on the web, the URL where the source is located
The references page is double spaced and lists entries in alphabetical order by the author’s last name. If an entry continues for more than one line, the second line and each subsequent line are indented five spaces. Review the following example.
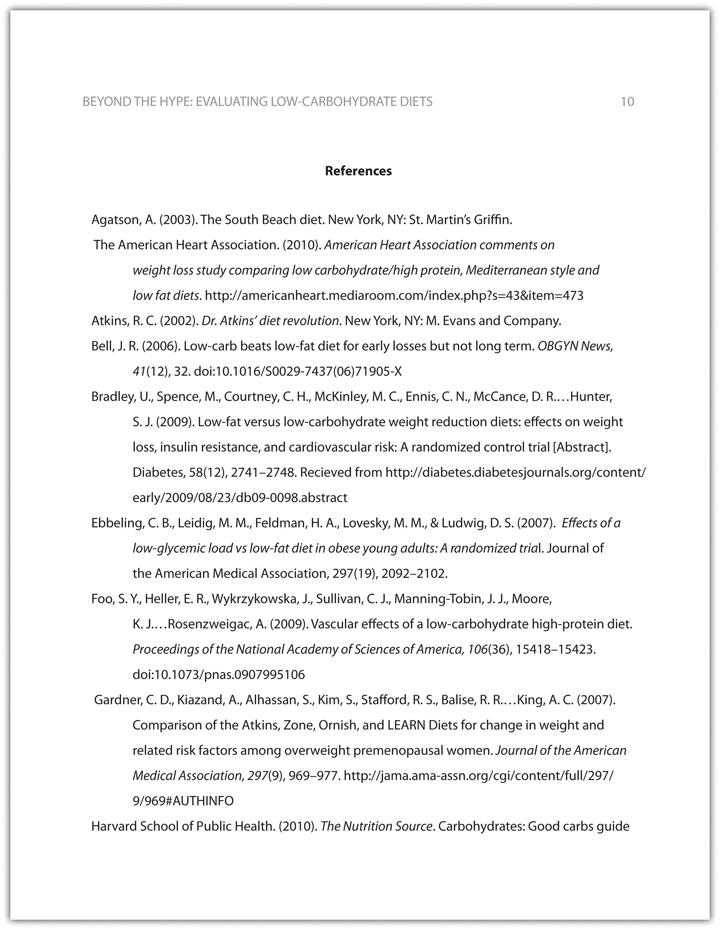
In APA style, book and article titles are formatted in sentence case, not title case. Sentence case means that only the first word is capitalized, along with any proper nouns.
Key Takeaways
- Following proper citation and formatting guidelines helps writers ensure that their work will be taken seriously, give proper credit to other authors for their work, and provide valuable information to readers.
- Working ahead and taking care to cite sources correctly the first time are ways writers can save time during the editing stage of writing a research paper.
- APA papers usually include an abstract that concisely summarizes the paper.
- APA papers use a specific headings structure to provide a clear hierarchy of information.
- In APA papers, in-text citations usually include the name(s) of the author(s) and the year of publication.
- In-text citations correspond to entries in the references section, which provide detailed bibliographical information about a source.
Putting the Pieces Together Copyright © 2020 by Andrew Stracuzzi and André Cormier is licensed under a Creative Commons Attribution-NonCommercial-ShareAlike 4.0 International License , except where otherwise noted.
Share This Book
Chapter 1: Research and Development | 2024 AI Index Report
Chapter 1: research and development, 1.1 publications.
The figures below present the global count of English- and Chinese-language AI publications from 2010 to 2022, categorized by type of affiliation and cross-sector collaborations. Additionally, this section details publication data for AI journal articles and conference papers.
Total Number of AI Publications
Figure 1.1.1 displays the global count of AI publications. Between 2010 and 2022, the total number of AI publications nearly tripled, rising from approximately 88,000 in 2010 to more than 240,000 in 2022. The increase over the last year was a modest 1.1%.
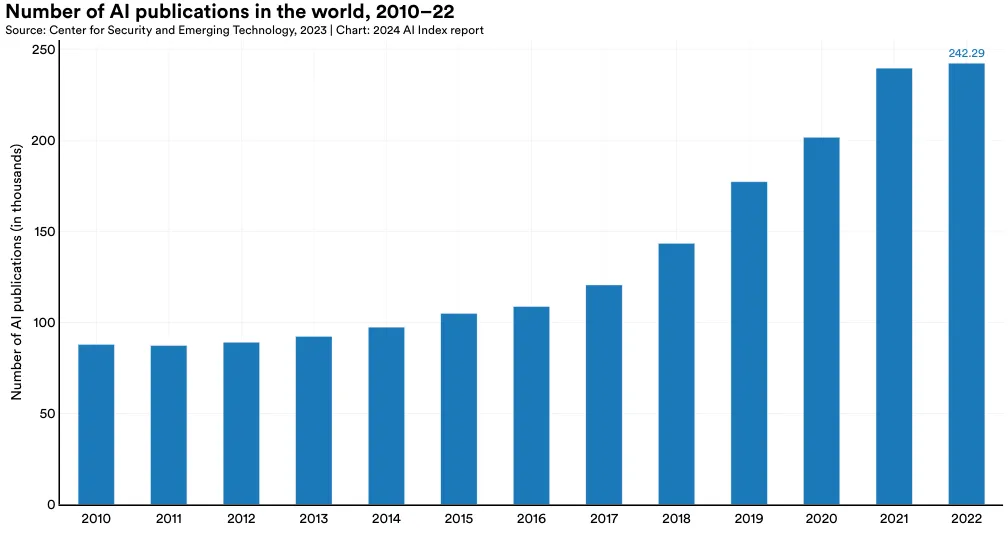
The data on publications presented this year is sourced from CSET. Both the methodology and data sources used by CSET to classify AI publications have changed since their data was last featured in the AI Index (2023). As a result, the numbers reported in this year’s section differ slightly from those reported in last year’s edition. Moreover, the AI-related publication data is fully available only up to 2022 due to a significant lag in updating publication data. Readers are advised to approach publication figures with appropriate caution.
By Type of Publication
Figure 1.1.2 illustrates the distribution of AI publication types globally over time. In 2022, there were roughly 230,000 AI journal articles compared to roughly 42,000 conference submissions. Since 2015, AI journal and conference publications have increased at comparable rates. In 2022, there were 2.6 times as many conference publications and 2.4 times as many journal publications as there were in 2015.
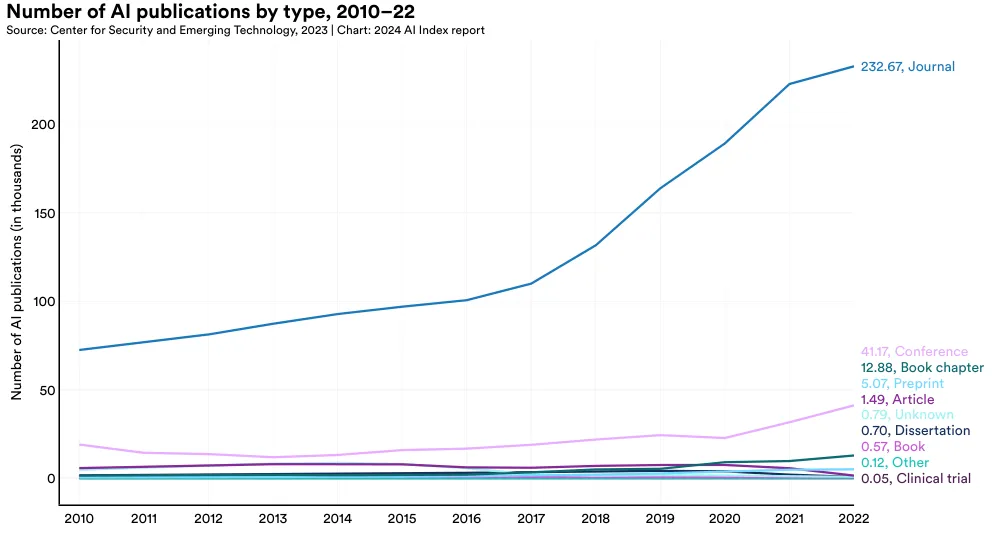
It is possible for an AI publication to be mapped to more than one publication type, so the totals in Figure 1.1.2 do not completely align with those in Figure 1.1.1.
By Field of Study
Figure 1.1.3 examines the total number of AI publications by field of study since 2010. Machine learning publications have seen the most rapid growth over the past decade, increasing nearly sevenfold since 2015. Following machine learning, the most published AI fields in 2022 were computer vision (21,309 publications), pattern recognition (19,841), and process management (12,052).
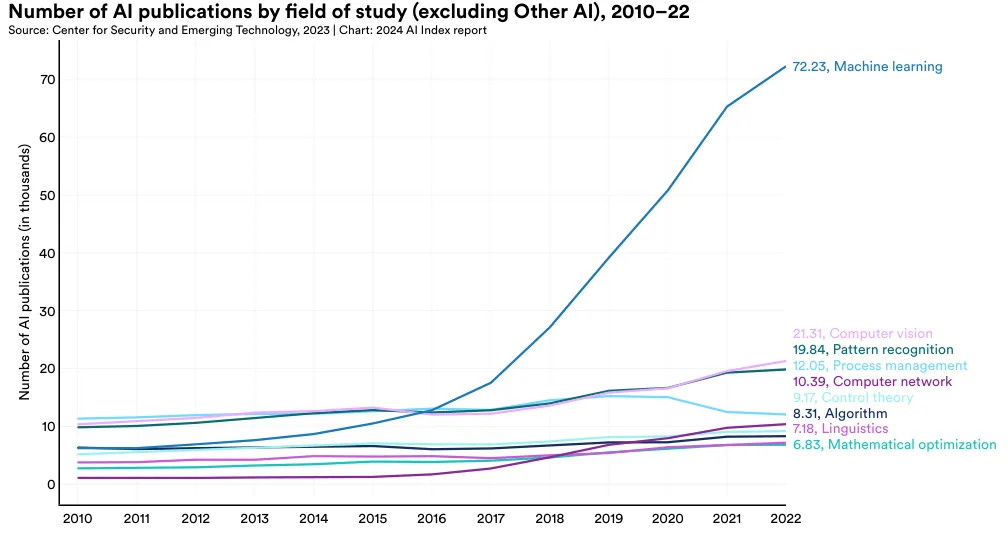
This section presents the distribution of AI publications by sector—education, government, industry, nonprofit, and other—globally and then specifically within the United States, China, and the European Union plus the United Kingdom. In 2022, the academic sector contributed the majority of AI publications (81.1%), maintaining its position as the leading global source of AI research over the past decade across all regions (Figure 1.1.4 and Figure 1.1.5). Industry participation is most significant in the United States, followed by the European Union plus the United Kingdom, and China (Figure 1.1.5).
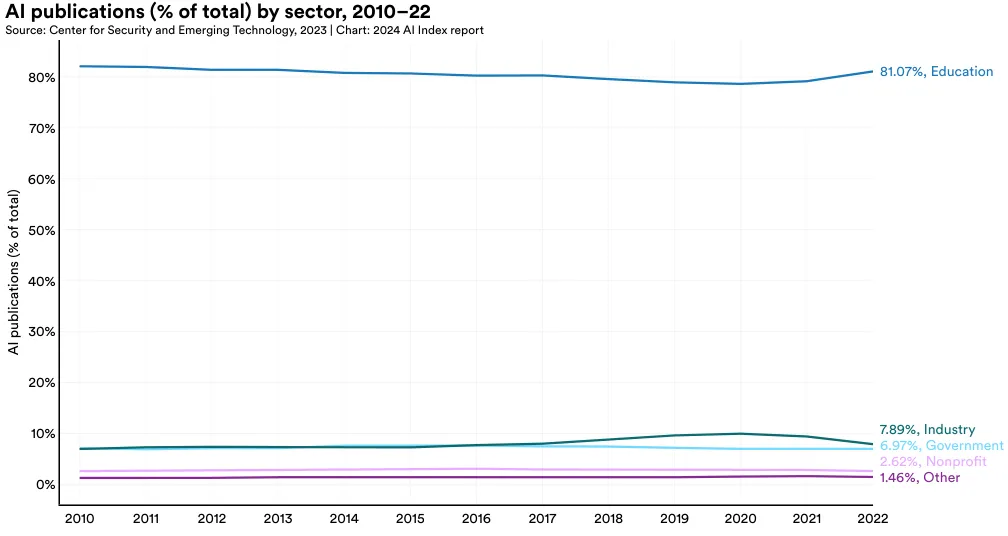
AI Journal Publications
Figure 1.1.6 illustrates the total number of AI journal publications from 2010 to 2022. The number of AI journal publications experienced modest growth from 2010 to 2015 but grew approximately 2.4 times since 2015. Between 2021 and 2022, AI journal publications saw a 4.5% increase.
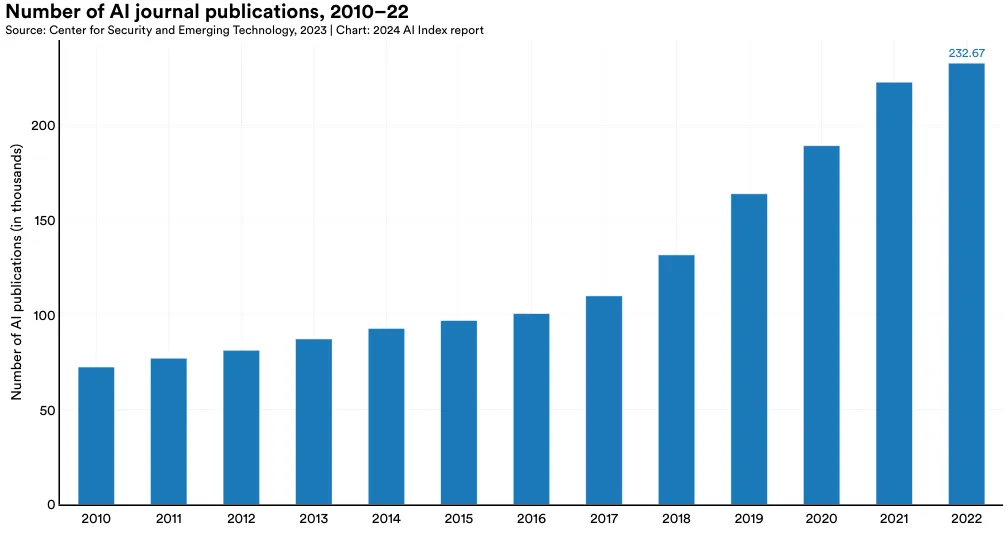
AI Conference Publications
Figure 1.1.7 visualizes the total number of AI conference publications since 2010. The number of AI conference publications has seen a notable rise in the past two years, climbing from 22,727 in 2020 to 31,629 in 2021, and reaching 41,174 in 2022. Over the last year alone, there was a 30.2% increase in AI conference publications. Since 2010, the number of AI conference publications has more than doubled.
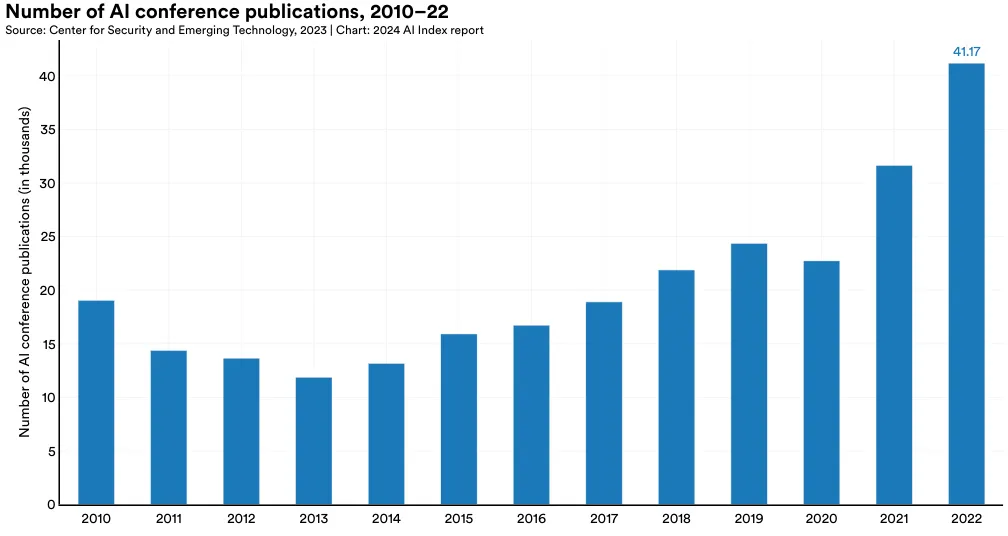
1.2 Patents
Figure 1.2.1 examines the global growth in granted AI patents from 2010 to 2022. Over the last decade, there has been a significant rise in the number of AI patents, with a particularly sharp increase in recent
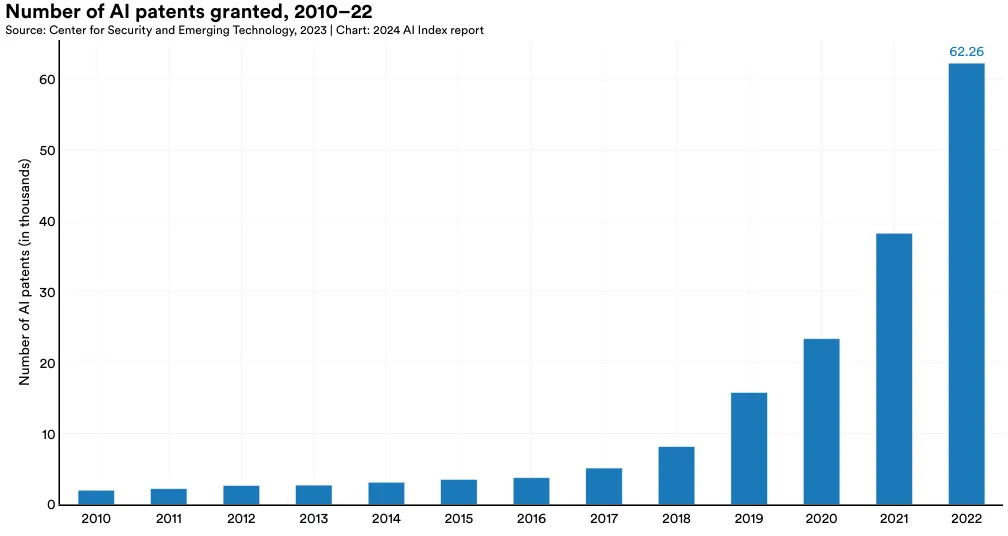
By Filing Status and Region
The following section disaggregates AI patents by their filing status (whether they were granted or not granted), as well as the region of their publication. Figure 1.2.2 compares global AI patents by application status. In 2022, the number of ungranted AI patents (128,952) was more than double the amount granted (62,264). Over time, the landscape of AI patent approvals has shifted markedly. Until 2015, a larger proportion of filed AI patents were granted. However, since then, the majority of AI patent filings have not been granted, with the gap widening significantly. For instance, in 2015, 42.2% of all filed AI patents were not granted. By 2022, this figure had risen to 67.4%.
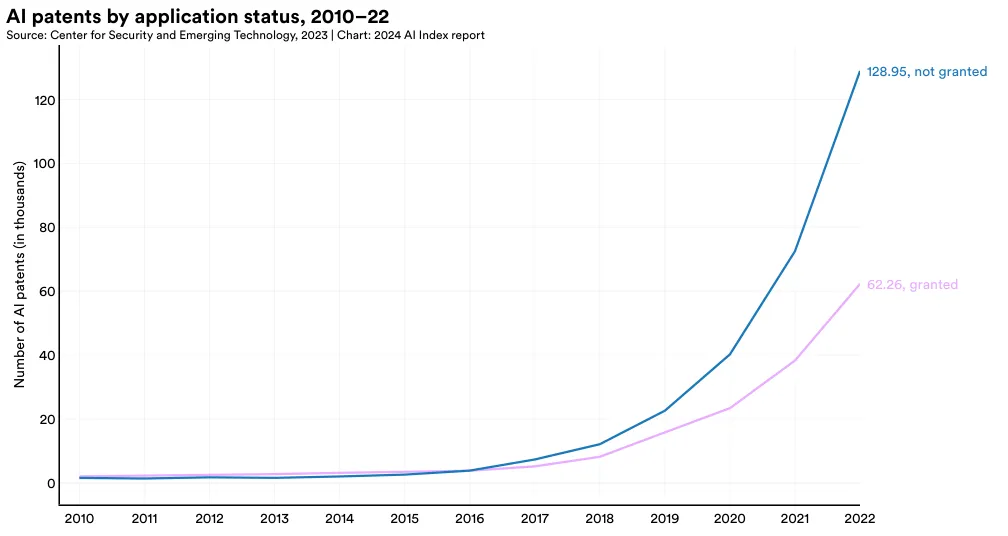
The gap between granted and not granted AI patents is evident across all major patent-originating geographic areas, including China, the European Union and United Kingdom, and the United States (Figure 1.2.3). In recent years, all three geographic areas have experienced an increase in both the total number of AI patent filings and the number of patents granted.
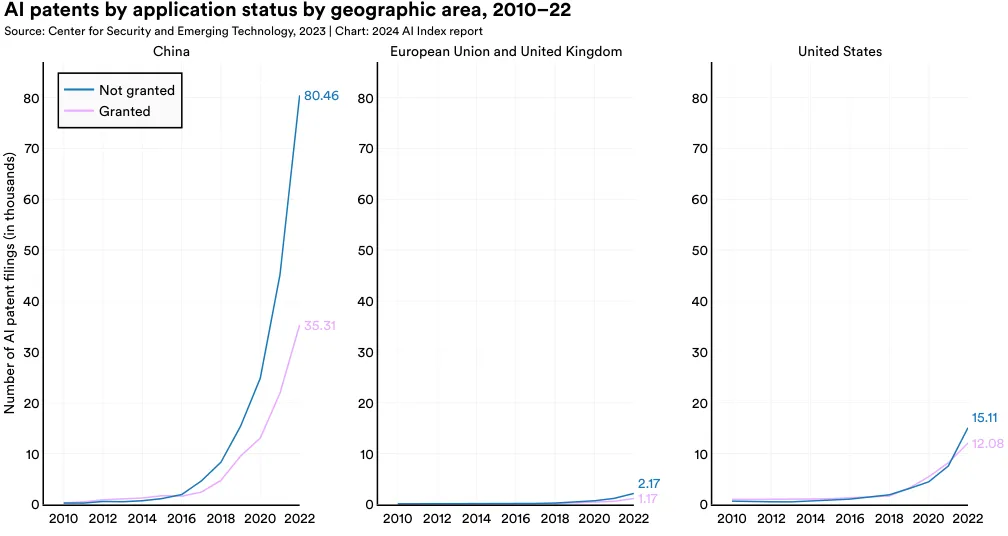
Figure 1.2.4 showcases the regional breakdown of granted AI patents. As of 2022, the bulk of the world’s granted AI patents (75.2%) originated from East Asia and the Pacific, with North America being the next largest contributor at 21.2%. Up until 2011, North America led in the number of global AI patents. However, since then, there has been a significant shift toward an increasing proportion of AI patents originating from East Asia and the Pacific.
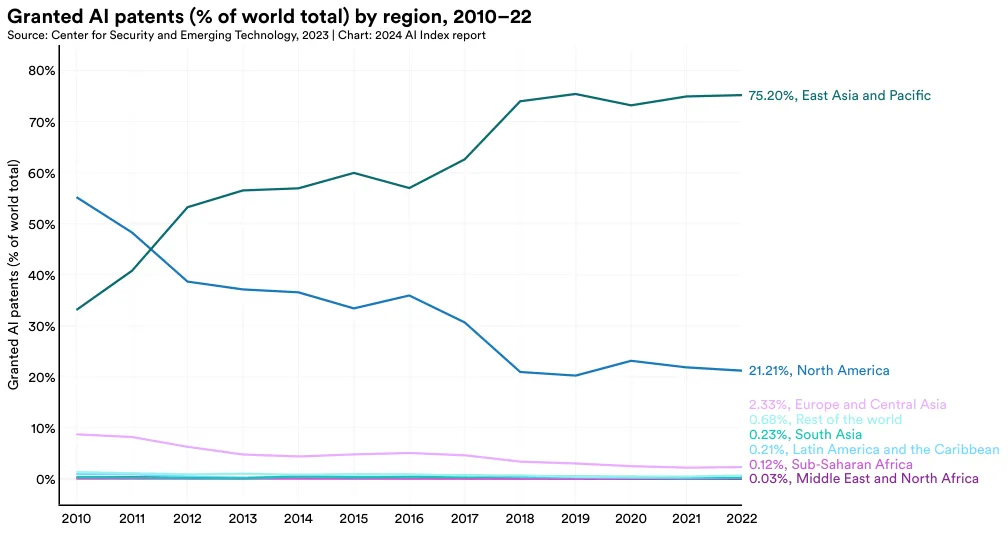
Disaggregated by geographic area, the majority of the world’s granted AI patents are from China (61.1%) and the United States (20.9%) (Figure 1.2.5). The share of AI patents originating from the United States has declined from 54.1% in 2010.
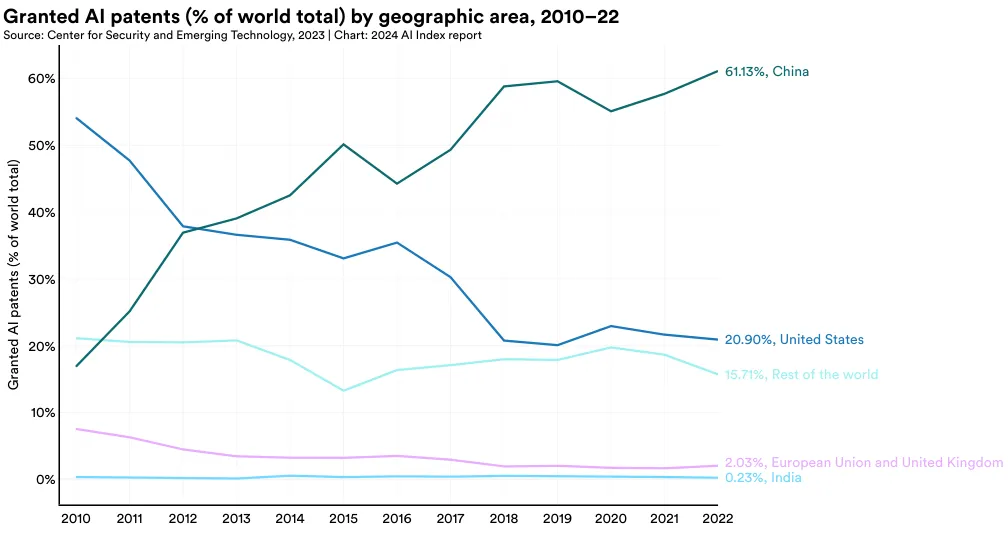
Figure 1.2.6 and Figure 1.2.7 document which countries lead in AI patents per capita. In 2022, the country with the most granted AI patents per 100,000 inhabitants was South Korea (10.3), followed by Luxembourg (8.8) and the United States (4.2) (Figure 1.2.6). Figure 1.2.7 highlights the change in granted AI patents per capita from 2012 to 2022. Singapore, South Korea, and China experienced the greatest increase in AI patenting per capita during that time period.
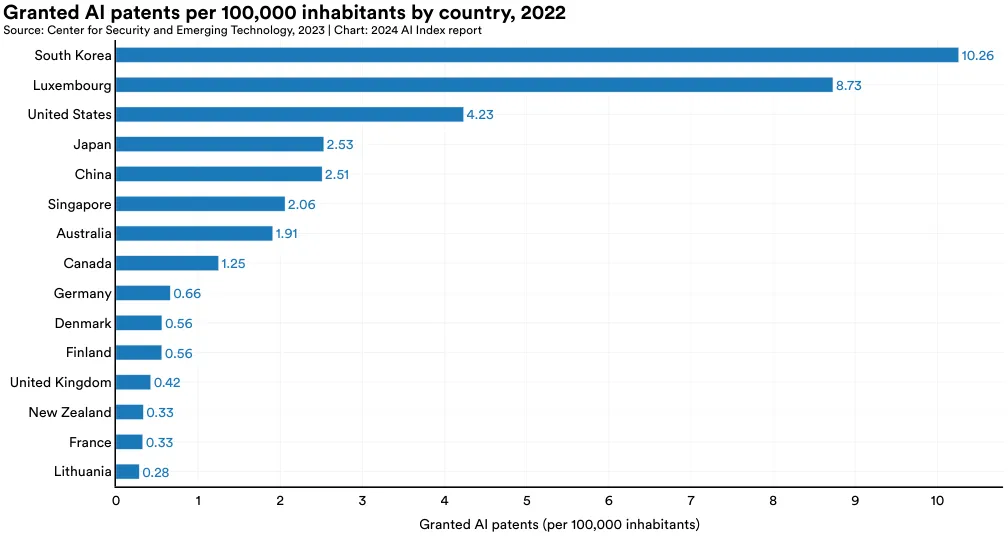
This section explores the frontier of AI research. While many new AI models are introduced annually, only a small sample represents the most advanced research. Admittedly what constitutes advanced or frontier research is somewhat subjective. Frontier research could reflect a model posting a new state-of-the-art result on a benchmark, introducing a meaningful new architecture, or exercising some impressive new capabilities.
The AI Index studies trends in two types of frontier AI models: “notable models” and foundation models.3 Epoch, an AI Index data provider, uses the term “notable machine learning models” to designate noteworthy models handpicked as being particularly influential within the AI/machine learning ecosystem. In contrast, foundation models are exceptionally large AI models trained on massive datasets, capable of performing a multitude of downstream tasks. Examples of foundation models include GPT-4, Claude 3, and Gemini. While many foundation models may qualify as notable models, not all notable models are foundation models.
Within this section, the AI Index explores trends in notable models and foundation models from various perspectives, including originating organization, country of origin, parameter count, and compute usage. The analysis concludes with an examination of machine learning training costs.
1.3 Frontier AI Research
General machine learning models.
Epoch AI is a group of researchers dedicated to studying and predicting the evolution of advanced AI. They maintain a database of AI and machine learning models released since the 1950s, selecting 1.3 Frontier AI Research entries based on criteria such as state-of-theart advancements, historical significance, or high citation rates. Analyzing these models provides a comprehensive overview of the machine learning landscape’s evolution, both in recent years and over the past few decades.4 Some models may be missing from the dataset; however, the dataset can reveal trends in relative terms.
3 “AI system” refers to a computer program or product based on AI, such as ChatGPT. “AI model” refers to a collection of parameters whose values are learned during training, such as GPT-4. 4 New and historic models are continually added to the Epoch database, so the total year-by-year counts of models included in this year’s AI Index might not exactly match those published in last year’s report.
Sector Analysis
Until 2014, academia led in the release of machine learning models. Since then, industry has taken the lead. In 2023, there were 51 notable machine learning models produced by industry compared to just 15 from academia (Figure 1.3.1). Significantly, 21 notable models resulted from industry/academic collaborations in 2023, a new high. Creating cutting-edge AI models now demands a substantial amount of data, computing power, and financial resources that are not available in academia. This shift toward increased industrial dominance in leading AI models was first highlighted in last year’s AI Index report . Although this year the gap has slightly narrowed, the trend largely persists.
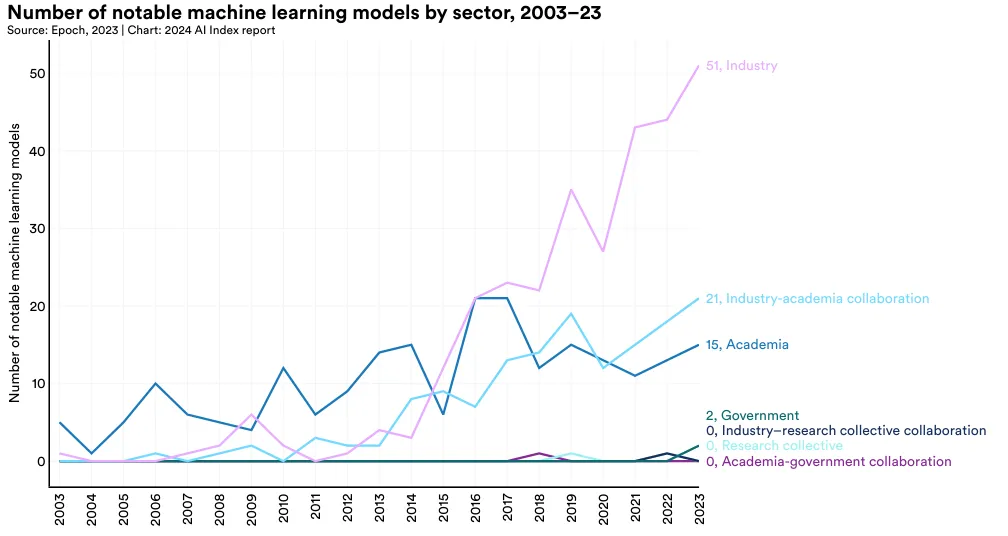
National Affiliation
To illustrate the evolving geopolitical landscape of AI, the AI Index research team analyzed the country of origin of notable models. Figure 1.3.2 displays the total number of notable machine learning models attributed to the location of researchers’ affiliated institutions.5 In 2023, the United States led with 61 notable machine learning models, followed by China with 15, and France with 8. For the first time since 2019, the European Union and the United Kingdom together have surpassed China in the number of notable AI models produced (Figure 1.3.3). Since 2003, the United States has produced more models than other major geographic regions such as the United Kingdom, China, and Canada (Figure 1.3.4).
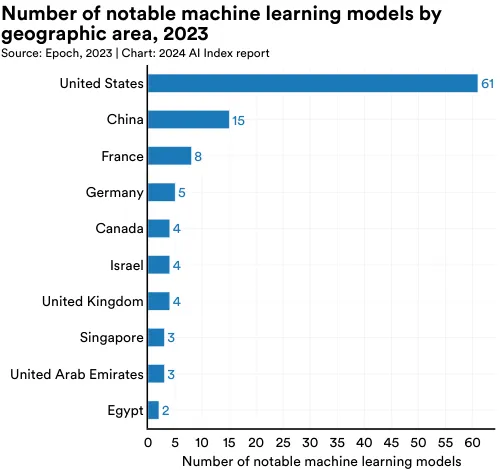
5 A machine learning model is considered associated with a specific country if at least one author of the paper introducing it has an affiliation with an institution based in that country. In cases where a model’s authors come from several countries, double counting can occur.
Parameter Trends
Parameters in machine learning models are numerical values learned during training that determine how a model interprets input data and makes predictions. Models trained on more data will usually have more parameters than those trained on less data. Likewise, models with more parameters typically outperform those with fewer parameters.
Figure 1.3.5 demonstrates the parameter count of machine learning models in the Epoch dataset, categorized by the sector from which the models originate. Parameter counts have risen sharply since the early 2010s, reflecting the growing complexity of tasks AI models are designed for, the greater availability of data, improvements in hardware, and proven efficacy of larger models. High-parameter models are particularly notable in the industry sector, underscoring the capacity of companies like OpenAI, Anthropic, and Google to bear the computational costs of training on vast volumes of data.
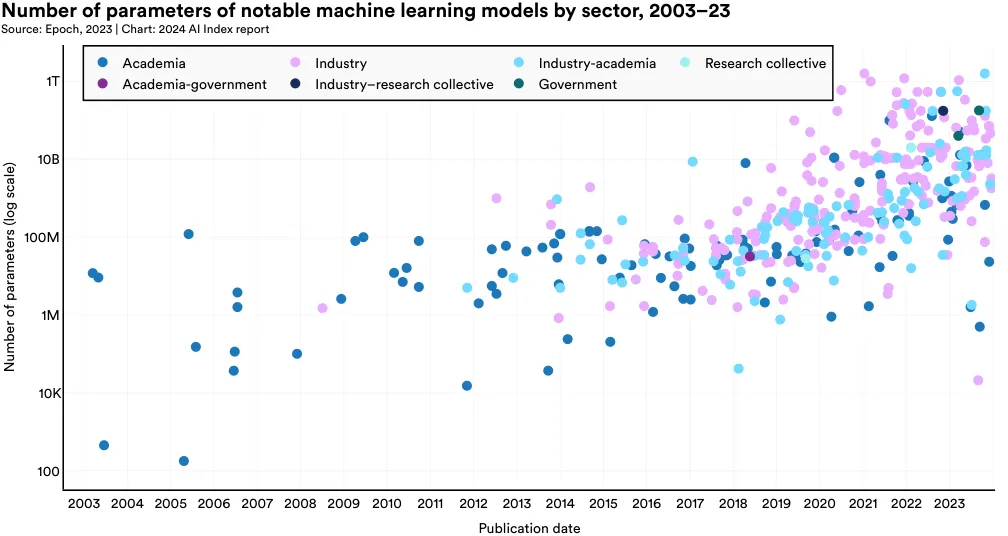
Compute Trends
The term “compute” in AI models denotes the computational resources required to train and operate a machine learning model. Generally, the complexity of the model and the size of the training dataset directly influence the amount of compute needed. The more complex a model is, and the larger the underlying training data, the greater the amount of compute required for training. Figure 1.3.6 visualizes the training compute required for notable machine learning models in the last 20 years. Recently, the compute usage of notable AI models has increased exponentially.6 This trend has been especially pronounced in the last five years. This rapid rise in compute demand has critical implications. For instance, models requiring more computation often have larger environmental footprints, and companies typically have more access to computational resources than academic institutions.
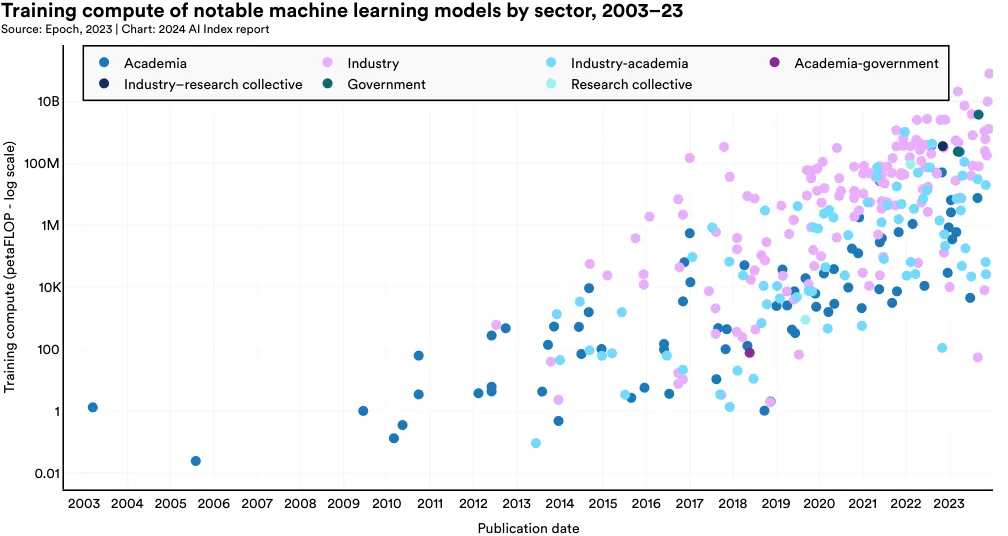
Figure 1.3.7 highlights the training compute of notable machine learning models since 2012. For example, AlexNet, one of the papers that popularized the now standard practice of using GPUs to improve AI models, required an estimated 470 petaFLOPs for training. The original Transformer, released in 2017, required around 7,400 petaFLOPs. Google’s Gemini Ultra, one of the current state-of-the-art foundation models, required 50 billion petaFLOPs.
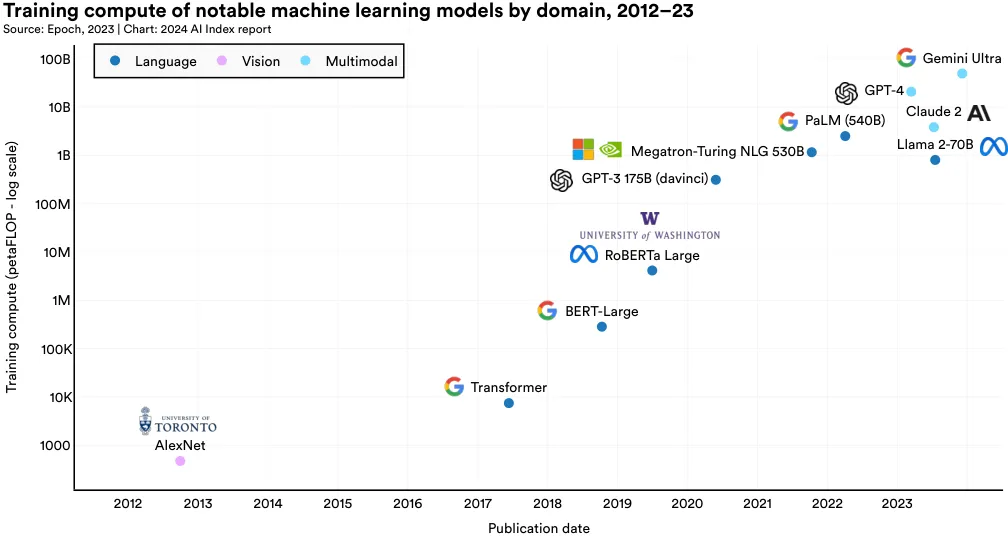
Highlight: Will Models Run Out Of Data?
As illustrated above, a significant proportion of recent algorithmic progress, including progress behind powerful LLMs, has been achieved by training models on increasingly larger amounts of data. As noted recently by Anthropic cofounder and AI Index Steering Committee member Jack Clark, foundation models have been trained on meaningful percentages of all the data that has ever existed on the internet.
The growing data dependency of AI models has led to concerns that future generations of computer scientists will run out of data to further scale and improve their systems. Research from Epoch suggests that these concerns are somewhat warranted. Epoch researchers have generated historical and compute-based projections for when AI researchers might expect to run out of data. The historical projections are based on observed growth rates in the sizes of data used to train foundation models. The compute projections adjust the historical growth rate based on projections of compute availability.
For instance, the researchers estimate that computer scientists could deplete the stock of high-quality language data by 2024, exhaust lowquality language data within two decades, and use up image data by the late 2030s to mid-2040s (Figure 1.3.8). Theoretically, the challenge of limited data availability can be addressed by using synthetic data, which is data generated by AI models themselves. For example, it is possible to use text produced by one LLM to train another LLM. The use of synthetic data for training AI systems is particularly attractive, not only as a solution for potential data depletion but also because generative AI systems could, in principle, generate data in instances where naturally occurring data is sparse—for example, data for rare diseases or underrepresented populations. Until recently, the feasibility and effectiveness of using synthetic data for training generative AI systems were not well understood. However, research this year has suggested that there are limitations associated with training models on synthetic data.
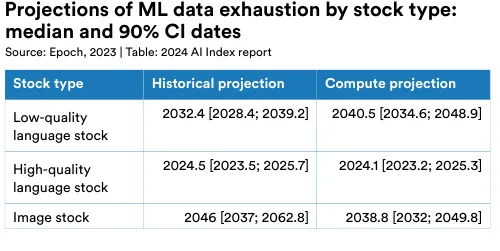
For instance, a team of British and Canadian researchers discovered that models predominantly trained on synthetic data experience model collapse, a phenomenon where, over time, they lose the ability to remember true underlying data distributions and start producing a narrow range of outputs. Figure 1.3.9 demonstrates the process of model collapse in a variational autoencoder (VAE) model, a widely used generative AI architecture. With each subsequent generation trained on additional synthetic data, the model produces an increasingly limited set of outputs. As illustrated in Figure 1.3.10, in statistical terms, as the number of synthetic generations increases, the tails of the distributions vanish, and the generation density shifts toward the mean.7 This pattern means that over time, the generations of models trained predominantly on synthetic data become less varied and are not as widely distributed. The authors demonstrate that this phenomenon occurs across various model types, including Gaussian Mixture Models and LLMs. This research underscores the continued importance of humangenerated data for training capable LLMs that can produce a diverse array of content.

7 In the context of generative models, density refers to the level of complexity and variation in the outputs produced by an AI model. Models that have a higher generation density produce a wider range of higher-quality outputs. Models with low generation density produce a narrower range of more simplistic outputs.
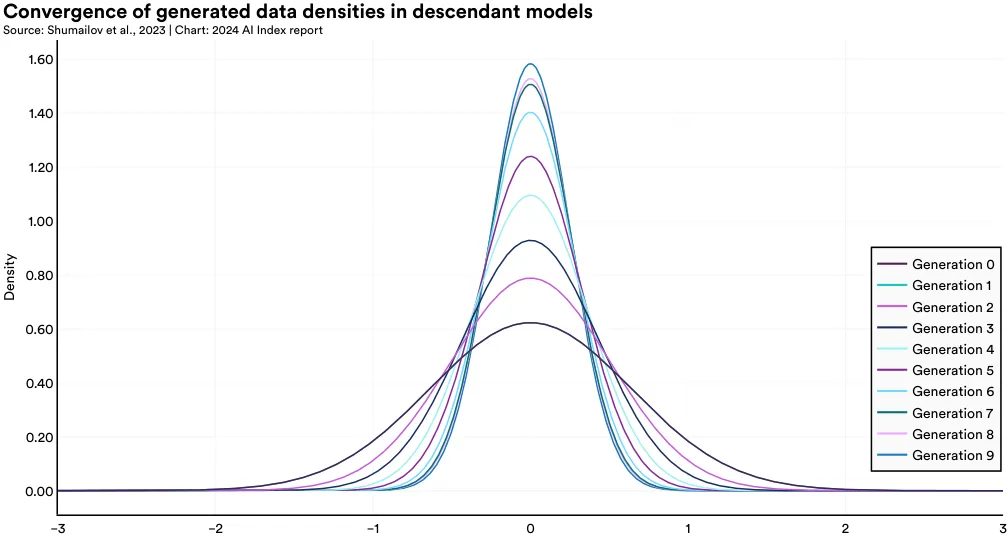
In a similar study published in 2023 on the use of synthetic data in generative imaging models, researchers found that generative image models trained solely on synthetic data cycles—or with insufficient real human data—experience a significant drop in output quality. The authors label this phenomenon Model Autophagy Disorder (MAD), in reference to mad cow disease.
The study examines two types of training processes: fully synthetic, where models are trained exclusively on synthetic data, and synthetic augmentation, where models are trained on a mix of synthetic and real data. In both scenarios, as the number of training generations increases, the quality of the generated images declines. Figure 1.3.11 highlights the degraded image generations of models that are augmented with synthetic data; for example, the faces generated in steps 7 and 9 increasingly display strange-looking hash marks. From a statistical perspective, images generated with both synthetic data and synthetic augmentation loops have higher FID scores (indicating less similarity to real images), lower precision scores (signifying reduced realism or quality), and lower recall scores (suggesting decreased diversity) (Figure 1.3.12). While synthetic augmentation loops, which incorporate some real data, show less degradation than fully synthetic loops, both methods exhibit diminishing returns with further training.

Foundation Models
Foundation models represent a rapidly evolving and popular category of AI models. Trained on vast datasets, they are versatile and suitable for numerous downstream applications. Foundation models such as GPT-4, Claude 3, and Llama 2 showcase remarkable abilities and are increasingly being deployed in realworld scenarios. Introduced in 2023, the Ecosystem Graphs is a new community resource from Stanford that tracks the foundation model ecosystem, including datasets, models, and applications. This section uses data from the Ecosystem Graphs to study trends in foundation models over time.8
Model Release
Foundation models can be accessed in different ways. No access models, like Google's PaLM-E, are only accessible to their developers. Limited access models, like OpenAI's GPT-4, offer limited access to the models, often through a public API. Open models, like Meta's Llama 2, fully release model weights, which means the models can be modified and freely used.
Figure 1.3.13 visualizes the total number of foundation models by access type since 2019. In recent years, the number of foundation models has risen sharply, more than doubling since 2022 and growing by a factor of nearly 38 since 2019. Of the 149 foundation models released in 2023, 98 were open, 23 limited and 28 no access.
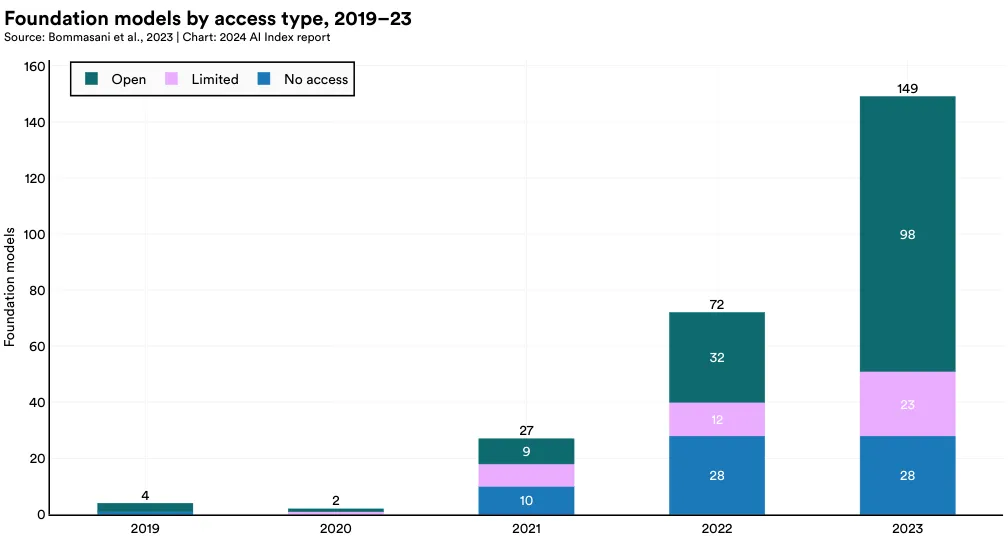
8 The Ecosystem Graphs make efforts to survey the global AI ecosystem, but it is possible that they underreport models from certain nations like South Korea and China.
In 2023, the majority of foundation models were released as open access (65.8%), with 18.8% having no access and 15.4% limited access (Figure 1.3.14). Since 2021, there has been a significant increase in the proportion of models released with open access.
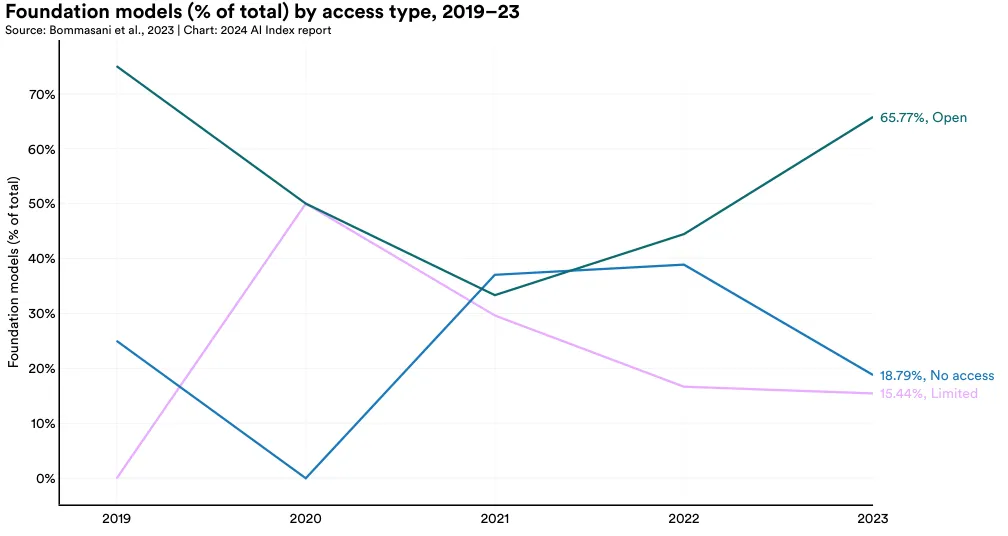
Organizational Affiliation
Figure 1.3.15 plots the sector from which foundation models have originated since 2019. In 2023, the majority of foundation models (72.5%) originated from industry. Only 18.8% of foundation models in 2023 originated from academia. Since 2019, an ever larger number of foundation models are coming from industry.
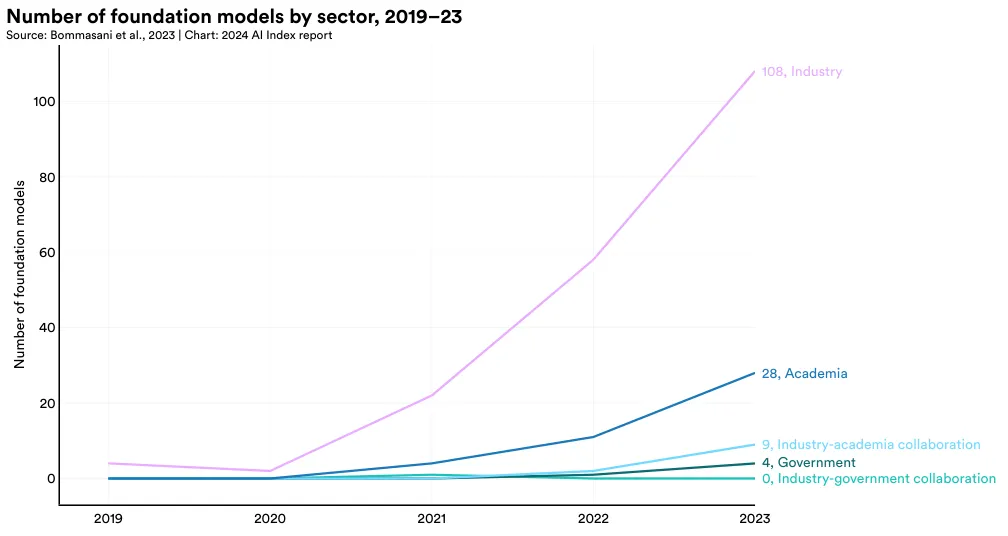
Figure 1.3.16 highlights the source of various foundation models that were released in 2023. Google introduced the most models (18), followed by Meta (11), and Microsoft (9). The academic institution that released the most foundation models in 2023 was UC Berkeley (3).
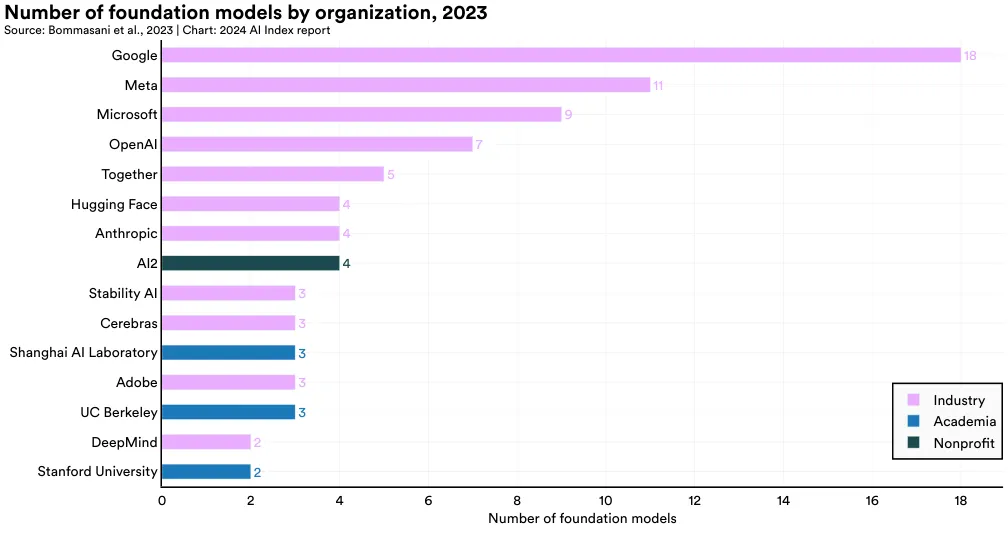
Since 2019, Google has led in releasing the most foundation models, with a total of 40, followed by OpenAI with 20 (Figure 1.3.17). Tsinghua University stands out as the top non-Western institution, with seven foundation model releases, while Stanford University is the leading American academic institution, with five releases.
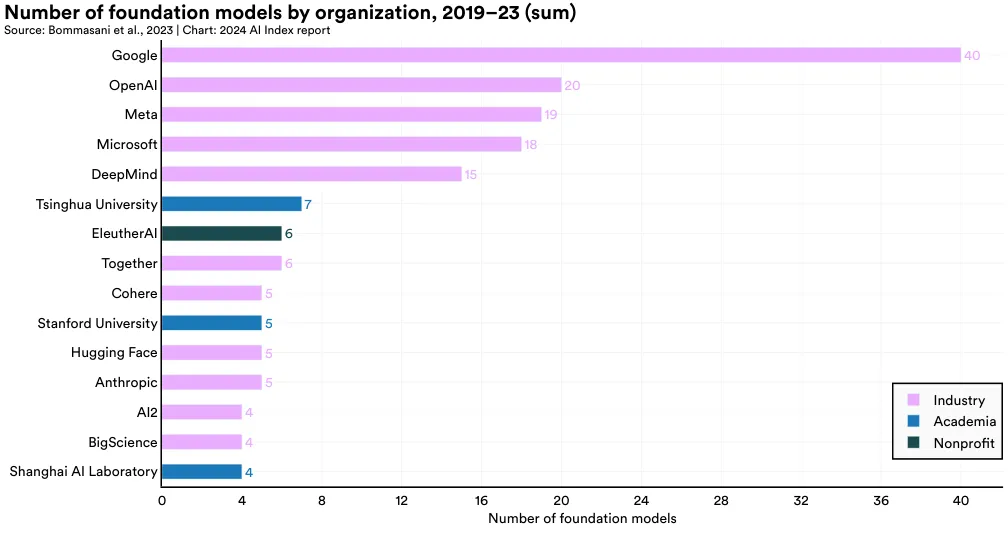
Given that foundation models are fairly representative of frontier AI research, from a geopolitical perspective, it is important to understand their national affiliations. Figures 1.3.18, 1.3.19, and 1.3.20 visualize the national affiliations of various foundation models. As with the notable model analysis presented earlier in the chapter, a model is deemed affiliated with a country if a researcher contributing to that model is affiliated with an institution headquartered in that country.
In 2023, most of the world's foundation models originated from the United States (109), followed by China (20), and the United Kingdom (Figure 1.3.18). Since 2019, the United States has consistently led in originating the majority of foundation models (Figure 1.3.19).
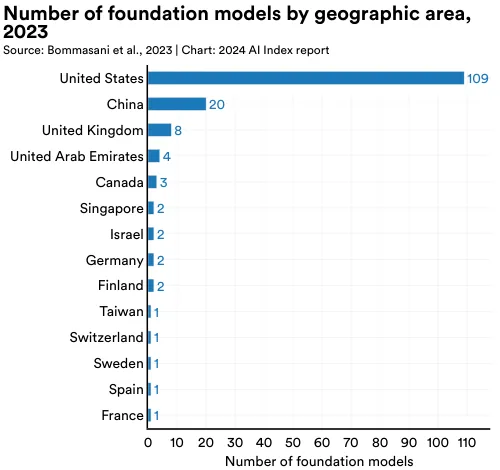
Figure 1.3.20 depicts the cumulative count of foundation models released and attributed to respective countries since 2019. The country with the greatest number of foundation models released since 2019 is the United States (182), followed by China (30), and the United Kingdom (21).
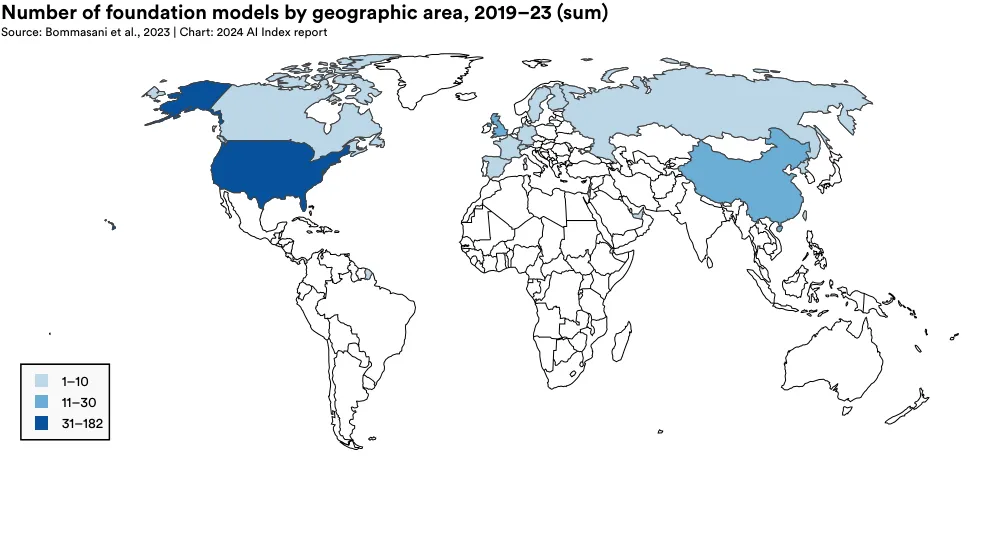
Training Cost
A prominent topic in discussions about foundation models is their speculated costs. While AI companies seldom reveal the expenses involved in training their models, it is widely believed that these costs run into millions of dollars and are rising. For instance, OpenAI's CEO, Sam Altman, mentioned that the training cost for GPT-4 was over $100 million. This escalation in training expenses has effectively excluded universities, traditionally centers of AI research, from developing their own leading-edge foundation models. In response, policy initiatives, such as President Biden's Executive Order on AI, have sought to level the playing field between industry and academia by creating a National AI Research Resource, which would grant nonindustry actors the compute and data needed to do higher level AI-research.
Understanding the cost of training AI models is important, yet detailed information on these costs remains scarce. The AI Index was among the first to offer estimates on the training costs of foundation models in last year's publication. This year, the AI Index has collaborated with Epoch AI, an AI research institute, to substantially enhance and solidify the robustness of its AI training cost estimates.9 To estimate the cost of cutting-edge models, the Epoch team analyzed training duration, as well as the type, quantity, and utilization rate of the training hardware, using information from publications, press releases, or technical reports related to the models.10
Figure 1.3.21 visualizes the estimated training cost associated with select AI models, based on cloud compute rental prices. AI Index estimates validate suspicions that in recent years model training costs have significantly increased. For example, in 2017, the original Transformer model, which introduced the architecture that underpins virtually every modern LLM, cost around 900 t o t r a i n . 11 R o B E R T a L a r g e , r e l e a s e d i n 2019 , w h i c h a c h i e v e d s t a t e − o f − t h e − a r t r e s u l t s o n m a n y c a n o n i c a l c o m p r e h e n s i o n b e n c h m a r k s l i k e S Q u A D a n d G L U E , c o s t a r o u n d 900 to train.11 RoBERTa Large, released in 2019, which achieved state-of-the-art results on many canonical comprehension benchmarks like SQuAD and GLUE, cost around 900 t o t r ain .11 R o BERT a L a r g e , re l e a se d in 2019 , w hi c ha c hi e v e d s t a t e − o f − t h e − a r t res u lt so nman yc an o ni c a l co m p re h e n s i o nb e n c hma r k s l ik e SQ u A D an d G LU E , cos t a ro u n d 160,000 to train. Fast-forward to 2023, and training costs for OpenAI's GPT-4 and Google's Gemini Ultra are estimated to be around 78 m i l l i o n a n d 78 million and 78 mi ll i o nan d 191 million, respectively.
9 Ben Cottier and Robi Rahman led research at Epoch AI into model training cost. 10 A detailed description of the estimation methodology is provided in the Appendix. 11 The cost figures reported in this section are inflation-adjusted.
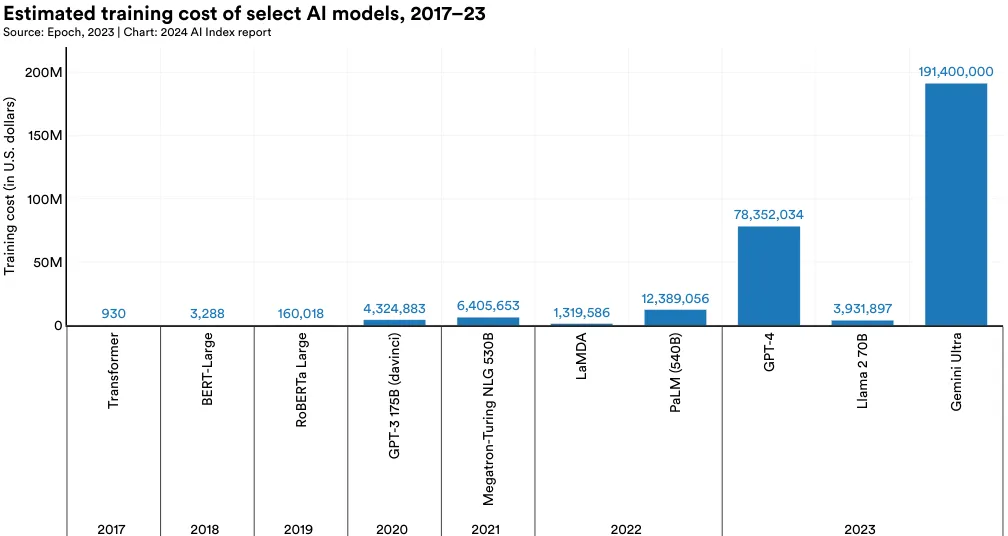
Figure 1.3.22 visualizes the training cost of all AI models for which the AI Index has estimates. As the figure shows, model training costs have sharply increased over time.
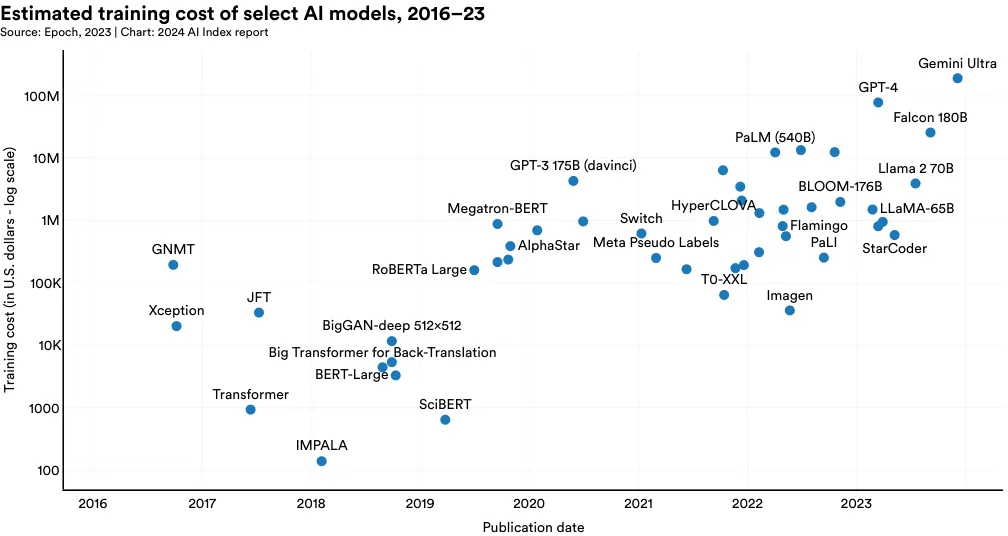
As established in previous AI Index reports, there is a direct correlation between the training costs of AI models and their computational requirements. As illustrated in Figure 1.3.23, models with greater computational training needs cost substantially more to train.
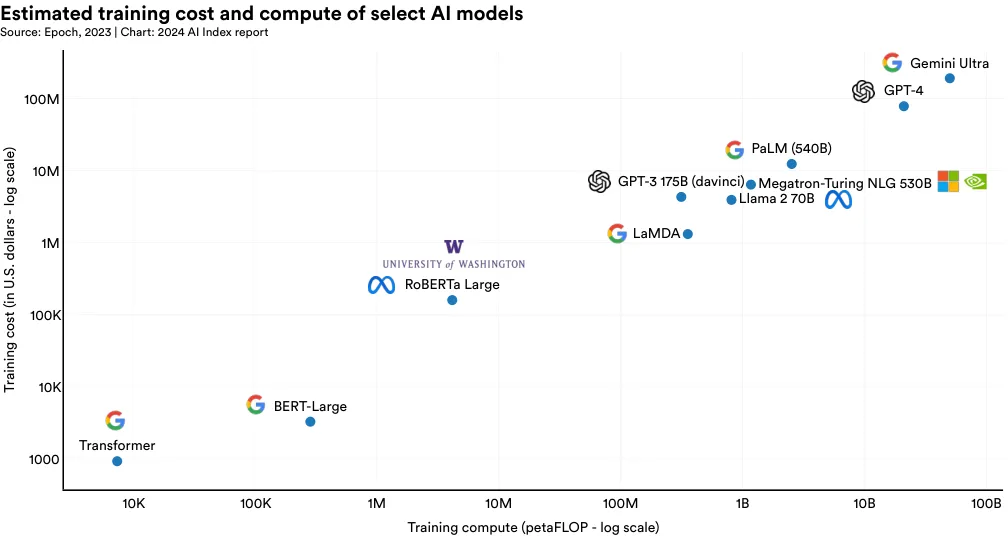
AI conferences serve as essential platforms for researchers to present their findings and network with peers and collaborators. Over the past two decades, these conferences have expanded in scale, quantity, and prestige.
1.4 Ai Conferences Conference Attendance
Conference attendance.
Figure 1.4.1 graphs attendance at a selection of AI conferences since 2010. Following a decline in attendance, likely due to the shift back to exclusively in-person formats, the AI Index reports an increase in conference attendance from 2022 to 2023.12
Specifically, there was a 6.7% rise in total attendance over the last year. Since 2015, the annual number of attendees has risen by around 50,000, reflecting not just a growing interest in AI research but also the emergence of new AI conferences.
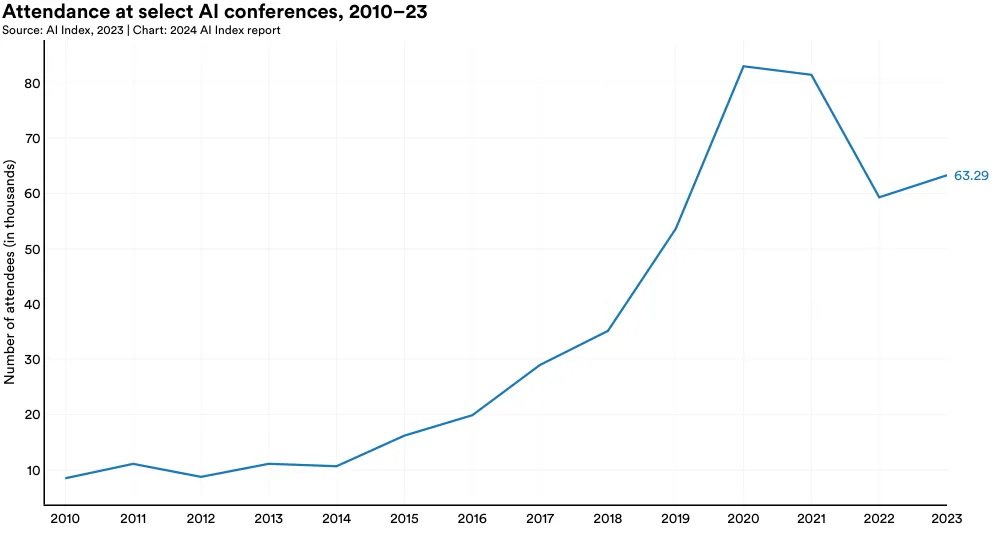
12 This data should be interpreted with caution given that many conferences in the last few years have had virtual or hybrid formats. Conference organizers report that measuring the exact attendance numbers at virtual conferences is difficult, as virtual conferences allow for higher attendance of researchers from around the world. The conferences for which the AI Index tracked data include NeurIPS , CVPR , ICML , ICCV , ICRA , AAAI , ICLR , IROS , IJCAI , AAMAS , FAccT , UAI , ICAPS , and KR .
Neural Information Processing Systems (NeurIPS) remains one of the most attended AI conferences, attracting approximately 16,380 participants in 2023 (Figure 1.4.2 and Figure 1.4.3). Among the major AI conferences, NeurIPS, ICML, ICCV, and AAAI experienced year-over-year increases in attendance. However, in the past year, CVPR, ICRA, ICLR, and IROS observed slight declines in their attendance figures.
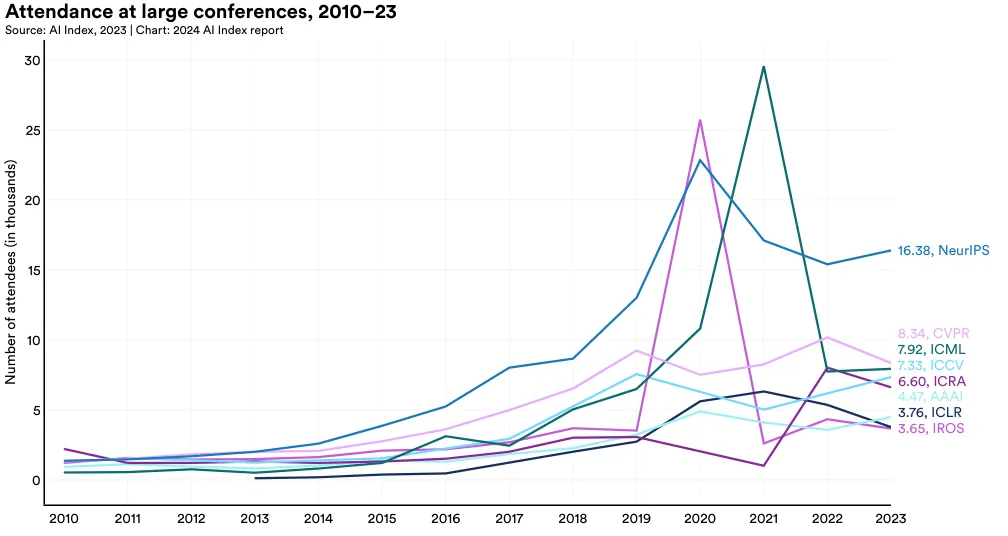
GitHub is a web-based platform that enables individuals and teams to host, review, and collaborate on code repositories. Widely used by software developers, GitHub facilitates code management, project collaboration, and open-source software support. This section draws on data from GitHub providing insights into broader trends in open-source AI software development not reflected in academic publication data.
1.5 Open-Source Ai Software
A GitHub project comprises a collection of files, including source code, documentation, configuration files, and images, that together make up a software project. Figure 1.5.1 looks at the total number of GitHub AI projects over time. Since 2011, the number of AI-related GitHub projects has seen a consistent increase, growing from 845 in 2011 to approximately 1.8 million in 2023.13 Notably, there was a sharp 59.3% rise in the total number of GitHub AI projects in the last year alone.
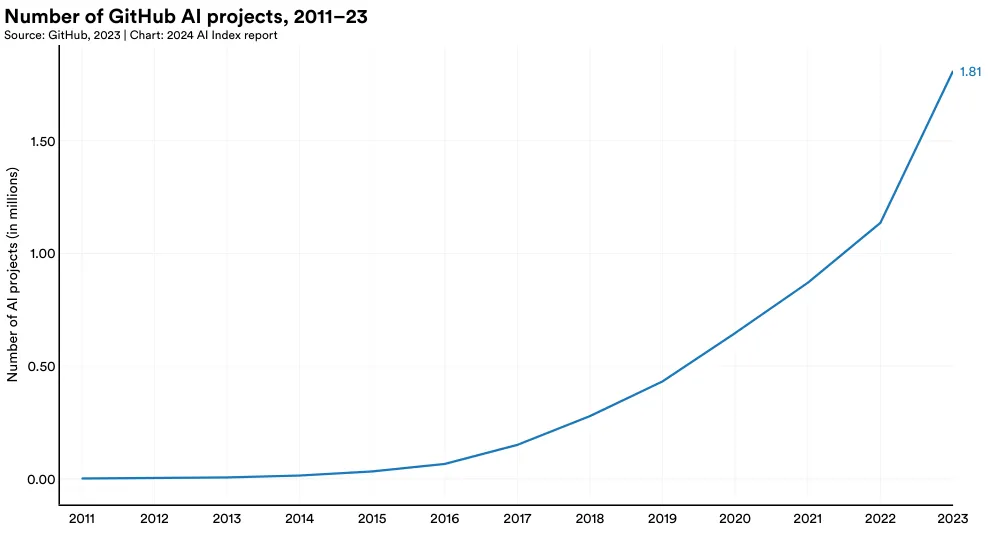
13 GitHub’s methodology for identifying AI-related projects has evolved over the past year. For classifying AI projects, GitHub has started incorporating generative AI keywords from a recently published research paper, a shift from the previously detailed methodology in an earlier paper. This edition of the AI Index is the first to adopt this updated approach. Moreover, the previous edition of the AI Index utilized country-level mapping of GitHub AI projects conducted by the OECD, which depended on self-reported data—a method experiencing a decline in coverage over time. This year, the AI Index has adopted geographic mapping from GitHub, leveraging server-side data for broader coverage. Consequently, the data presented here may not align perfectly with data in earlier versions of the report.
Figure 1.5.2 reports GitHub AI projects by geographic area since 2011. As of 2023, a significant share of GitHub AI projects were located in the United States, accounting for 22.9% of contributions. India was the second-largest contributor with 19.0%, followed closely by the European Union and the United Kingdom at 17.9%. Notably, the proportion of AI projects from developers located in the United States on GitHub has been on a steady decline since 2016.
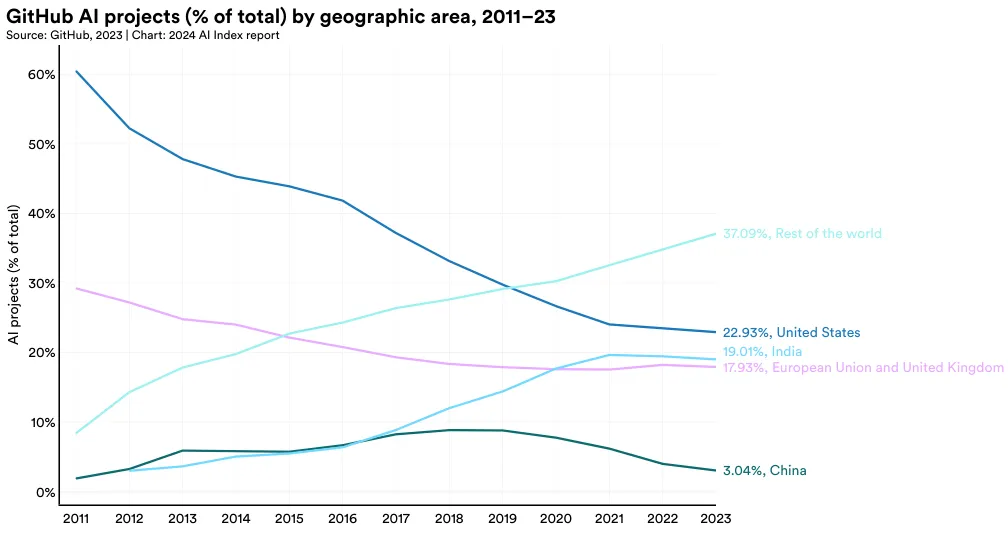
GitHub users can show their interest in a repository by “starring” it, a feature similar to liking a post on social media, which signifies support for an open- source project. Among the most starred repositories are libraries such as TensorFlow, OpenCV, Keras, and PyTorch, which enjoy widespread popularity among software developers in the AI coding community. For example, TensorFlow is a popular library for building and deploying machine learning models. OpenCV is a platform that offers a variety of tools for computer vision, such as object detection and feature extraction.
The total number of stars for AI-related projects on GitHub saw a significant increase in the last year, more than tripling from 4.0 million in 2022 to 12.2 million in 2023 (Figure 1.5.3). This sharp increase in GitHub stars, along with the previously reported rise in projects, underscores the accelerating growth of open-source AI software development.
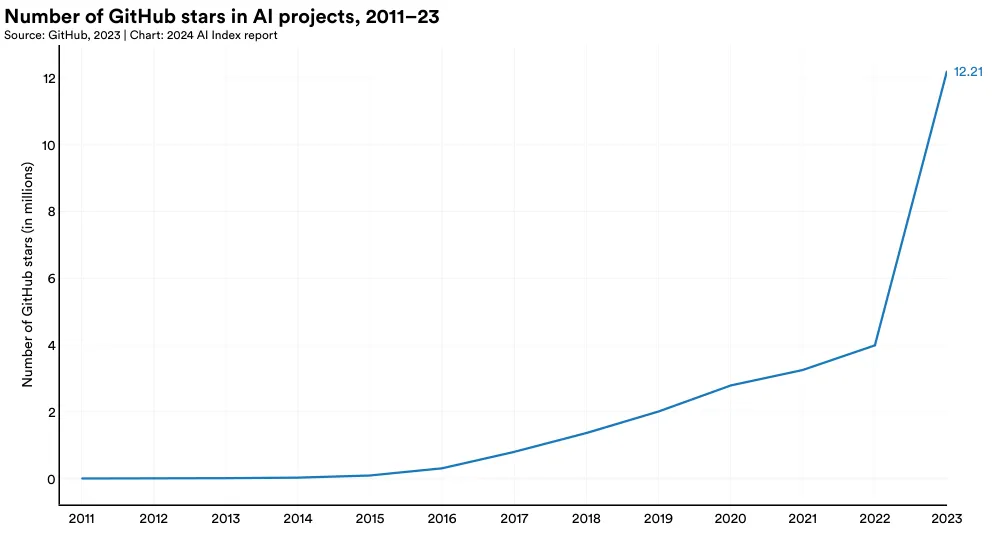
In 2023, the United States led in receiving the highest number of GitHub stars, totaling 10.5 million (Figure 1.5.4). All major geographic regions sampled, including the European Union and United Kingdom, China, and India, saw a year-over-year increase in the total number of GitHub stars awarded to projects located in their countries.
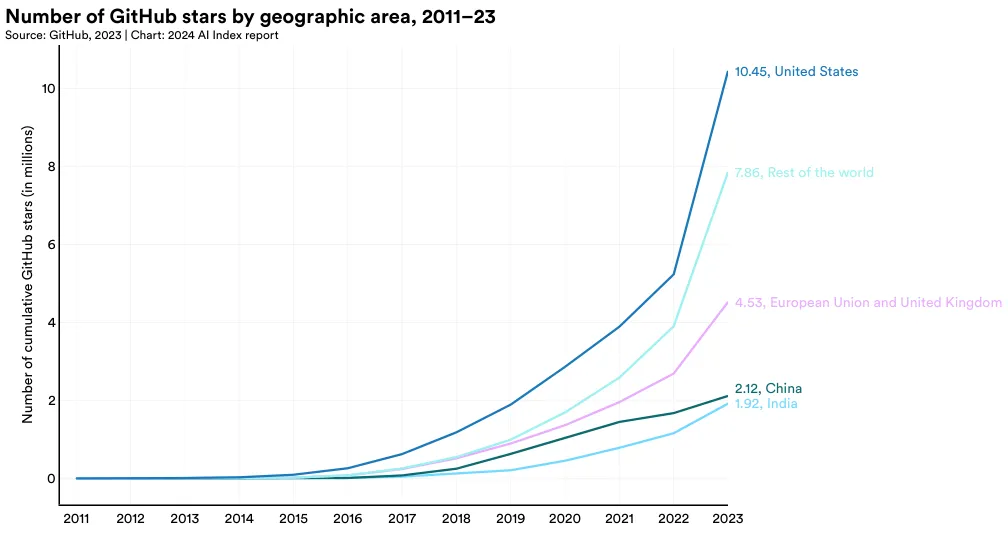
Acknowledgments
The AI Index would like to acknowledge Ben Cottier and Robi Rahman from Epoch for leading the work analyzing machine learning training costs; Robi Rahman for leading work regarding the national affiliation of notable systems; and James da Costa, for doing coding work instrumental to the sectoral and national affiliation analysis of foundation models.
AI Conference Attendance
The AI Index reached out to the organizers of various AI conferences in 2023 and asked them to provide information on total attendance. Some conferences posted their attendance totals online; when this was the case, the AI Index used those reported totals and did not reach out to the conference organizers.
Prepared by Autumn Toney
The Center for Security and Emerging Technology (CSET) is a policy research organization within Georgetown University’s Walsh School of Foreign Service that produces data-driven research at the intersection of security and technology, providing nonpartisan analysis to the policy community.
For more information about how CSET analyzes bibliometric and patent data, see the Country Activity Tracker (CAT) documentation on the Emerging Technology Observatory’s website.1 Using CAT, users can also interact with country bibliometric, patent, and investment data.2
Publications From CSET Merged Corpus of Scholarly Literature
CSET’s merged corpus of scholarly literature combines distinct publications from Clarivate’s Web of Science, OpenAlex, The Lens, Semantic Scholar, arXiv, and Papers With Code.
Updates: The source list of scholarly literature for CSET’s merged corpus has been changed from prior years, with the inclusion of OpenAlex, the Lens, and Semantic Scholar, and the exclusion of Digital Science’s Dimensions and the Chinese National Knowledge Infrastructure (CNKI).
Methodology
To create the merged corpus, CSET deduplicated across the listed sources using publication metadata, and then combined the metadata for linked publications. For analysis of AI publications, CSET used an English-language subset of this corpus published since 2010. CSET researchers developed a classifier for identifying AI-related publications by leveraging the arXiv repository, where authors and editors tag papers by subject.3
Updates: The AI classifier was updated from the version used in prior years; Dunham, Melot, and Murdick4 describe the previously implemented classifier; and Schoeberl, Toney, and Dunham describe the updated classifier used in this analysis.
CSET matched each publication in the analytic corpus with predictions from a field-of-study model derived from Microsoft Academic Graph (MAG)’s taxonomy, which yields hierarchical labels describing the published research field(s) of study and corresponding scores.5 CSET researchers identified the most common fields of study in our corpus of AI-relevant publications since 2010 and recorded publications in all other fields as “Other AI.” English-language AI-relevant publications were then tallied by their top-scoring field and publication year. Updates: The methodology to assign MAG fields of study was updated from the methodology used in prior years. Toney and Dunham describe the field of study assignment pipeline used in this analysis; prior years used the original MAG implementation.
CSET also provided publication counts and year-by- year citations for AI-relevant work associated with each country. A publication is associated with a country if it has at least one author whose organizational affiliation(s) is located in that country. If there is no observed country, the publication receives an “Unknown/Missing” country label. Citation counts aren’t available for all publications; those without counts weren’t included in the citation analysis. Over 70% of English-language AI papers published between 2010 and 2022 have citation data available.
Additionally, publication counts by year and by publication type (e.g., academic journal articles, conference papers) were provided where available. These publication types were disaggregated by affiliation country as described above.
CSET also provided publication affiliation sector(s) where, as in the country attribution analysis, sectors were associated with publications through authors’ affiliations. Not all affiliations were characterized in terms of sectors; CSET researchers relied primarily on ROR for this purpose, and not all organizations can be found in or linked to ROR.6 Where the affiliation sector is available, papers were counted toward these sectors, by year.
CSET counted cross-sector collaborations as distinct pairs of sectors across authors for each publication. Collaborations are only counted once: For example, if a publication has two authors with an academic affiliation and two with an industry affiliation, it is counted as a single academic-industry collaboration.
Patents From CSET’s AI and Robotics Patents Dataset Source
CSET’s AI patents dataset was developed by CSET and 1790 Analytics and includes data from The Lens, 1790 Analytics, and EPO’s PATSTAT. Patents relevant to the development and application of AI and robotics were identified by their CPC/IPC codes and keywords.
In this analysis, patents were grouped by year and country, and then counted at the “patent family” classifier; and Schoeberl, Toney, and Dunham describe the updated classifier used in this analysis.
CSET matched each publication in the analytic corpus with predictions from a field-of-study model derived from Microsoft Academic Graph (MAG)’s taxonomy, which yields hierarchical labels describing the published research field(s) of study and corresponding scores.5 CSET researchers identified the most common fields of study in our corpus of AI-relevant publications since 2010 and recorded publications in all other fields as “Other AI.” English-language AI-relevant publications were then tallied by their top-scoring field and publication year.
Updates: The methodology to assign MAG fields of study was updated from the methodology used in prior years. Toney and Dunham describe the field of study assignment pipeline used in this analysis; prior years used the original MAG implementation.
Patents From CSET’s AI and Robotics Patents Dataset
In this analysis, patents were grouped by year and country, and then counted at the “patent family” level.7 CSET extracted year values from the first publication date within a family. Countries are assigned to patents based on the country or filing office where a patent is first filed (e.g., if a patent is filed with the USPTO on January 1, 2020, and then with the German Patenting Office on January 2, 2020, the patent is classified as a patent with U.S. inventors).8 Note that the same patent may have multiple countries (but not years) attributed to it if the inventors filed their patent in multiple countries on the same first filing date (e.g., if a patent is filed with the USPTO on January 1, 2020, and then with the German Patenting Office on January 1, 2020, the patent is classified as a patent with U.S. inventors and as a patent with German inventors).
Note that patents filed with supranational organizations, such as patents filed under WIPO (the World Intellectual Property Organization), EP (European Patent Organization), and EA (a special area of Spain not included in the European Union), also fall under the “Rest of World” category.
Ecosystems Graph Analysis
To track the distribution of AI foundation models by country, the AI Index team took the following steps:
- A snapshot of the Ecosystems Graph was taken in early January 2024.
- Authors of foundation models are attributed to countries based on their affiliation credited on the paper/technical documentation associated with the model. For international organizations, authors are attributed to the country where the organization is headquartered, unless a more specific location is indicated.
All of the landmark publications are aggregated within time periods (e.g., monthly or yearly) with the national contributions added up to determine what each country’s contribution to landmark AI research was during each time period.
The contributions of different countries are compared over time to identify any trends.
Epoch Notable Models Analysis
The AI forecasting research group Epoch maintains a dataset of landmark AI and ML models, along with accompanying information about their creators and publications, such as the list of their (co)authors, number of citations, type of AI task accomplished, and amount of compute used in training.
The nationalities of the authors of these papers have important implications for geopolitical AI forecasting. As various research institutions and technology companies start producing advanced ML models, the global distribution of future AI development may shift or concentrate in certain places, which in turn affects the geopolitical landscape because AI is expected to become a crucial component of economic and military power in the near future.
To track the distribution of AI research contributions on landmark publications by country, the Epoch dataset is coded according to the following methodology:
A snapshot of the dataset was taken on January 1, 2024. This includes papers about landmark models, selected using the inclusion criteria of importance, relevance, and uniqueness, as described in the Compute Trends dataset documentation.
The authors are attributed to countries based on their affiliation credited on the paper. For international organizations, authors are attributed to the country where the organization is headquartered, unless a more specific location is indicated.
Identifying AI Projects
In partnership with researchers from Harvard Business School, Microsoft Research, and Microsoft’s AI for Good Lab, GitHub identifies public AI repositories following the methodologies of Gonzalez, Zimmerman, and Nagappan, 2020, and Dohmke, Iansiti, and Richards, 2023, using topic labels related to AI/ML and generative AI, respectively, along with the topics “machine learning,” “deep learning,” or “artificial intelligence.” GitHub further augments the dataset with repositories that have a dependency on the PyTorch, TensorFlow, or OpenAI libraries for Python.
Mapping AI Projects to Geographic Areas
Public AI projects are mapped to geographic areas using IP address geolocation to determine the mode location of a project’s owners each year. Each project owner is assigned a location based on their IP address when interacting with GitHub. If a project owner changes locations within a year, the location for the project would be determined by the mode location of its owners sampled daily in the year. Additionally, the last known location of the project owner is carried forward on a daily basis even if no activities were performed by the project owner that day. For example, if a project owner performed activities within the United States and then became inactive for six days, that project owner would be considered to be in the United States for that seven-day span.
Training Cost Analysis
To create the dataset of cost estimates, the Epoch database was filtered for models released during the large-scale ML era9 that were above the median of training compute in a two-year window centered on their release date. This filtered for the largest-scale ML models. There were 138 qualifying systems based on these criteria. Of these systems, 48 had sufficient information to estimate the training cost.
For the selected ML models, the training time and the type, quantity, and utilization rate of the training hardware were determined from the publication, press release, or technical reports, as applicable. Cloud rental prices for the computing hardware used by these models were collected from online historical archives of cloud vendors’ websites.10
Training costs were estimated from the hardware type, quantity, and time by multiplying the hourly cloud rental cost rates (at the time of training)11 by the quantity of hardware hours. This yielded the cost to train each model using the same hardware used by the authors to train the same model at the time. However, some developers purchased hardware rather than renting cloud computers, so the true costs incurred by the developers may vary.
Various challenges were encountered while estimating the training cost of these models. Often, the developers did not disclose the duration of training or the hardware that was used. In other cases, cloud compute pricing was not available for the hardware. The investigation of training cost trends is continued in a forthcoming Epoch report, including an expanded dataset with more models and hardware prices.
1 https://eto.tech/tool-docs/cat/\ 2 https://cat.eto.tech/\ 3 Christian Schoeberl, Autumn Toney, and James Dunham, “Identifying AI Research” (Center for Security and Emerging Technology, July 2023), https://doi.org/10.51593/20220030.\ 4 James Dunham, Jennifer Melot, and Dewey Murdick, “Identifying the Development and Application of Artificial Intelligence in Scientific Text,” arXiv preprint, arXiv:2002.07143 (2020). 5 These scores are based on cosine similarities between field-of-study and paper embeddings. See Autumn Toney and James Dunham, “Multi-Label Classification of Scientific Research Documents Across Domains and Languages,” Proceedings of the Third Workshop on Scholarly Document Processing (Association for Computational Linguistics, 2022): 105–14, https:// aclanthology.org/2022.sdp-1.12/. 6 See https://ror.org/ for more information about the ROR dataset. 7 Patents are analyzed at the “patent family” level rather than “patent document” level because patent families are a collective of patent documents all associated with a single invention and/ or innovation by the same inventors/assignees. Thus, counting at the “patent family” level mitigates artificial number inflation when there are multiple patent documents in a patent family or if a patent is filed in multiple jurisdictions. 8 In CSET’s data analysis for the 2022 AI Index, we used the most recent publication date for a patent family. This method has the advantage of capturing updates within a patent family (such as amendments). However, to remain consistent with CSET’s other data products, including the Country Activity Tracker (available at https://cat.eto.tech/ ), we opted to use the first filing year instead in this data analysis. 9 The selected cutoff date was September 1, 2015, in accordance with Compute Trends Across Three Eras of Machine Learning (Epoch, 2022). 10 Historic prices were collected from archived snapshots of Amazon Web Services, Microsoft Azure, and Google Cloud Platform price catalogs viewed through the Internet Archive Wayback Machine. 11 The chosen rental cost rate was the most recent published price for the hardware and cloud vendor used by the developer of the model, at a three-year commitment rental rate, after subtracting the training duration and two months from the publication date. If this price was not available, the most analogous price was used: the same hardware and vendor at a different date, otherwise the same hardware from a different cloud vendor. If a three-year commitment rental rate was unavailable, this was imputed from other rental rates based on the empirical average discount for the given cloud vendor. If the exact hardware type was not available, e.g., “NVIDIA A100 SXM4 40GB,” then a generalization was used, e.g., “NVIDIA A100.”

IMAGES
VIDEO
COMMENTS
research questions. 2. Contains references to outcomes in Chapter 4. 3. Covers all the data. 4. Is bounded by the evidence collected. 5. Relates the findings to a larger body of literature on the topic, including the conceptual or theoretical framework.
parts: the Introduction (Chapter 1), the Review of Related Literature and/or Research (Chapter 2), and the Methodology (Chapter 3). The completed dissertation begins with the same three chapters and concludes with two additional chapters that report research findings (Chapter 4) and conclusions, discussion, and recommendations (Chapter 5).
Chapter 1: Introduction; Chapter 2: Literature review; Chapter 3: Methodology; Chapter 4: Results; Chapter 5: Discussion; Chapter 6: Conclusion; Reference list; Appendix; Most importantly, the core chapters should reflect the research process (asking, investigating and answering your research question). Moreover, the research question(s) should ...
1. Introduction 2. Literature review 3. Methodology 4. Findings 5. Analysis and synthesis 6. Conclusions and recommendations Chapter 1: Introduction This chapter makes a case for the signifi-cance of the problem, contextualizes the study, and provides an introduction to its basic components. It should be informative and able to stand alone as a ...
CHAPTER 1: INTRODUCTION This chapter introduces and provides an overview of the research that is to be undertaken. Parts of Chapter 1 summarize your Chapters 2 and 3, and because of that, Chapter 1 normally should be written after Chapters 2 and 3. Dissertation committee chairs often want students to provide a 5-10 page overview of their proposed
Definition: Research Paper is a written document that presents the author's original research, analysis, and interpretation of a specific topic or issue. It is typically based on Empirical Evidence, and may involve qualitative or quantitative research methods, or a combination of both. The purpose of a research paper is to contribute new ...
In this episode of the series, A Basic Guide to Doing Research, Dr. Sarah Chidiebere Joe shares relevant information on how to write our first three chapters...
Table of contents. Step 1: Introduce your topic. Step 2: Describe the background. Step 3: Establish your research problem. Step 4: Specify your objective (s) Step 5: Map out your paper. Research paper introduction examples. Frequently asked questions about the research paper introduction.
Chapter 3: Theoretical Frameworks; Chapter 4: Methods and Data in Qualitative Research; Chapter 5: Subjectivity, Identity, and Texts in Qualitative Research; Part 2 (Chapters 6 - 13): Research Design. Chapter 6: Formulating a Research Question; Chapter 7: Choosing and Constructing the Research Design; Chapter 8: Planning the Process in ...
Chapter 1: Introduction Chapter 2: Literature review Chapter 3: Methods Chapter 4: Paper 1 & general discussion Chapter 5: Paper 2 Chapter 6: Regular thesis chapter - results Chapter 7: Regular thesis chapter/general discussion tying in published and unpublished work; Chapter 8: Conclusion Appendices - May include CD, DVD or other material, also reviews & methods papers
Research Paper Introduction Examples could be: Example 1: In recent years, the use of artificial intelligence (AI) has become increasingly prevalent in various industries, including healthcare. AI algorithms are being developed to assist with medical diagnoses, treatment recommendations, and patient monitoring.
Formal Research Structure. These are the primary purposes for formal research: enter the discourse, or conversation, of other writers and scholars in your field. learn how others in your field use primary and secondary resources. find and understand raw data and information. For the formal academic research assignment, consider an ...
5 parts of research paper - Download as a PDF or view online for free. ... 2. • CHAPTER 1 THE PROBLEM AND ITS BACKGROUND • CHAPTER 2 REVIEW OF RELATED LITERATURE • CHAPTER 3 METHOD AND PROCEDURES • CHAPTER 4 PRESENTATION, ANALYSIS AND INTERPRETATION OF DATA • CHAPTER 5 SUMMARY, ...
Introducing Research project chapters Every research project, thesis or dissertation is organised in chapters. Research project chapters range from 1, 2, 3, 4 and 5 ...
The research paper template covers the following core sections: The title page/cover page. Abstract (sometimes also called the executive summary) Section 1: Introduction. Section 2: Literature review. Section 3: Methodology. Section 4: Findings /results. Section 5: Discussion. Section 6: Conclusion.
Set the top, bottom, and side margins of your paper at 1 inch. Use double-spaced text throughout your paper. Use a standard font, such as Times New Roman or Arial, in a legible size (10- to 12-point). Use continuous pagination throughout the paper, including the title page and the references section.
5.1 The Abstract. The abstract of a research paper contains the most critical aspects of the paper: your research question, the context (country/population/subjects and period) analyzed, the findings, and the main conclusion. You have about 250 characters to attract the attention of the readers. Many times (in fact, most of the time), readers ...
Research Design and Methodology. Chapter 3 consists of three parts: (1) Purpose of the. study and research design, (2) Methods, and (3) Statistical. Data analysis procedure. Part one, Purpose of ...
RESEARCH-PAPER-CHAPTER-1-5 (1) Polledo, Cyrish Kiel DP. study and definition of terms that will help the researchers to introduce and formulate the research. face to face classes into online learning. This research context compels students to experience new. systems implemented learning modalities.
CHAPTER I: INTRODUCTION. 1. The purpose of this qualitative grounded theory study was to identify what motivates. women to stay in or return to science, technology, engineering, and math professions. (STEM), leading to a motivation model. As illustrated in the literature review, research has. abbreviations. introduce introduce you can use Once ...
Ideally, Chapter 1 (introduction) should be past tense, chapter 2 (literature) can be present or past depending on how you quote, chapter 3( methodology) definitely past tense, chapter 4( results ...
research guide. 1. BASIC FORMAT. 2. CHAPTER I The Problem and its Background Introduction Statement of the Problem Significant of the Study Scope and Delimitation of the Study. 3. CHAPTER II Review of Related Literature Relevant Literature Relevant Studies Conceptual Framework Hypothesis Definition ofTerms. 4.
Results: Regarding the similarity of the responses from 4 LLMs; the GPT-4 output was used as the reference answer, the responses from GPT-3.5 were the most similar, followed by those from LLaMA 2, ORCA_mini, and MedAlpaca. Human answers from Yahoo data were scored the lowest and, thus, as the least similar to GPT-4-generated answers.
Set the top, bottom, and side margins of your paper at 1 inch. Use double-spaced text throughout your paper. Use a standard font, such as Times New Roman or Arial, in a legible size (10- to 12-point). Use continuous pagination throughout the paper, including the title page and the references section.
Figure 1.1.7 visualizes the total number of AI conference publications since 2010. The number of AI conference publications has seen a notable rise in the past two years, climbing from 22,727 in 2020 to 31,629 in 2021, and reaching 41,174 in 2022. Over the last year alone, there was a 30.2% increase in AI conference publications.