Thank you for visiting nature.com. You are using a browser version with limited support for CSS. To obtain the best experience, we recommend you use a more up to date browser (or turn off compatibility mode in Internet Explorer). In the meantime, to ensure continued support, we are displaying the site without styles and JavaScript.
- View all journals
- My Account Login
- Explore content
- About the journal
- Publish with us
- Sign up for alerts
- Open access
- Published: 14 October 2021

Epidemiological and economic impact of COVID-19 in the US
- Jiangzhuo Chen 1 ,
- Anil Vullikanti 1 , 2 ,
- Joost Santos 3 ,
- Srinivasan Venkatramanan 1 ,
- Stefan Hoops 1 ,
- Henning Mortveit 1 , 4 ,
- Bryan Lewis 1 ,
- Wen You 5 ,
- Stephen Eubank 1 , 5 ,
- Madhav Marathe 1 , 2 ,
- Chris Barrett 1 , 2 &
- Achla Marathe 1 , 5
Scientific Reports volume 11 , Article number: 20451 ( 2021 ) Cite this article
17k Accesses
36 Citations
12 Altmetric
Metrics details
- Computational models
- Infectious diseases
This research measures the epidemiological and economic impact of COVID-19 spread in the US under different mitigation scenarios, comprising of non-pharmaceutical interventions. A detailed disease model of COVID-19 is combined with a model of the US economy to estimate the direct impact of labor supply shock to each sector arising from morbidity, mortality, and lockdown, as well as the indirect impact caused by the interdependencies between sectors. During a lockdown, estimates of jobs that are workable from home in each sector are used to modify the shock to labor supply. Results show trade-offs between economic losses, and lives saved and infections averted are non-linear in compliance to social distancing and the duration of the lockdown. Sectors that are worst hit are not the labor-intensive sectors such as the Agriculture sector and the Construction sector, but the ones with high valued jobs such as the Professional Services, even after the teleworkability of jobs is accounted for. Additionally, the findings show that a low compliance to interventions can be overcome by a longer shutdown period and vice versa to arrive at similar epidemiological impact but their net effect on economic loss depends on the interplay between the marginal gains from averting infections and deaths, versus the marginal loss from having healthy workers stay at home during the shutdown.
Similar content being viewed by others
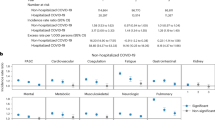
Three-year outcomes of post-acute sequelae of COVID-19
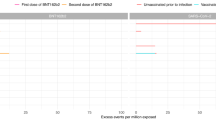
Safety outcomes following COVID-19 vaccination and infection in 5.1 million children in England
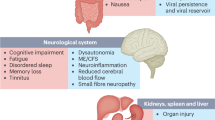
Long COVID: major findings, mechanisms and recommendations
Introduction.
According to the Bureau of Labor Statistics, the US unemployment rate in October 2020 stood at 6.9% and the number of unemployed at 11.1 million. This is likely an underestimated number since it does not include individuals who have stopped looking for employment 1 due to poor economic prospects. Even though both measures have declined for 6 months consecutively, the unemployment rate is still higher by 3.5% and the number of unemployed by 5.3 million, compared to pre COVID-19 levels in February 2020. The US economy shrank by an annual rate of 4.8% in the first quarter of 2020 and by a shocking 32.9% in the second quarter, which has been the largest drop seen since 1945. The number of COVID-19 cases have crossed 12 million and number of deaths over 258,000 in November 2020 2 .
This research builds a comprehensive system that combines the epidemiological model developed to study the spread of COVID-19 with a detailed model of the US economy to understand a sector wise economic impact from a shock to labor supply caused by the pandemic. Note that the focus of this paper is only on the shock encountered by the economic sectors from the supply side, and not on the demand side which has also dropped due to the high unemployment rate and a bleak economic outlook. We consider a number of counterfactual scenarios that comprise of various social distancing measures such as the stay-home order, voluntary home isolation of the symptomatic individuals, and school closure. We measure economic losses from the drop in labor supply in each sector due to the stay-home order, absenteeism due to illness and deaths, cascading loss to/from other sectors due to interdependencies between sectors, and the economic burden caused by the medical treatment of the infected. We vary compliance to interventions and duration of the stay-home order to determine their impact on economic and epidemiological outcomes and the trade-offs between them.
This research is an extension of the work done in 3 which only focused on estimating the medical cost of treatment for COVID-19 cases under the same mitigation scenarios and the disease model. Here we calculate overall economic losses from a societal perspective which include the medical cost of illness, cost of intervention or social distancing i.e. healthy individuals unable to go to work, direct loss in productivity due to morbidity and mortality of workers, and the indirect loss caused by the interdependencies between sectors. We also estimate the effect of intervention scenarios on cases and deaths averted in the US.
This level of detailed analysis has not been done in the literature before for COVID-19, and can provide guidance to public health officials for developing strategies to balance the emergence of infections and deaths with the economic costs of the social distancing strategies. A longer duration of stay-home order causes economic losses even after accounting for telework, but it also significantly reduces infections and deaths, and losses caused by the medical treatment of the infected.
Related work
There have been several papers that study the economic impact of COVID-19. Eichenbaum et al. 4 study the interaction between economic decisions and epidemic outcomes and find that the competitive equilibrium is not socially optimal because infected people do not fully internalize the effect of their economic decisions on the spread of the virus. Their results show that an optimal containment strategy that starts early and ramps up with infections, can cause a large recession but save about half a million lives, assuming no treatment or vaccines are available. A counterfactual scenario analysis in 5 shows that a delay of 4 weeks in applying control measures would have slowed the decay of the epidemic by 49 days in China. Work by 6 shows that strict control measures in China led to a steep drop in the temporal effective reproduction number, a metric used for assessing the efficacy of interventions.
Work by 7 shows that differential targeting of risk/age groups outperform uniform social distancing policies. Most of the economic gains in this study are realized from implementing stricter lockdown policies on the oldest age group. However a fully targeted policy can be challenging to implement and ethically questionable. Baker et al. 8 characterize the uncertainty using stock market volatility measures, newspaper-based measures of uncertainty and survey-based perceptions of business level uncertainty; and find that more than half of the contraction in US economy is caused by COVID-induced uncertainty.
Toda (2020) 9 uses an SIR model to study the impact of the epidemic on the stock market. Jones et al. 10 use an SIR contagion model and a model for consumption and production to analyze optimal mitigation policies and interactions between economic activity and epidemic dynamics. They discuss congestion externality i.e. when hospital capacity is exceeded, the risk of death becomes higher but agents do not internalize the impact of their decisions on others and therefore behave in a socially sub-optimal way. Other papers that study the economic impact of COVID-19 and pandemics are 11 , 12 , 13 , 14 .
The ripple effects of pandemics across a regional economy are studied using an input–output model in 15 , 16 . The impacts of pandemic-induced workforce disruptions are assessed using economic losses as well as inoperability, which measures the extent to which sectors are unable to produce their ideal level of output.
The novelty of our research lies in building a detailed integrated system that combines a network based population model with an epidemiological model and an economic model. The disease spreads on the social network, as determined by the COVID-19 disease model; non-pharmaceutical interventions remove particular edges in the social network depending upon the type of interventions and compliance rate; the duration of the interventions determine the length of the time edges are removed for; the outcome of the spread is captured in terms of infections and deaths, which determine the shock to labor-supply in specific economic sectors, as determined by the occupation of individuals who are sick or dead, as well as those who are healthy but unable to work due to a lockdown. These shocks as well as the interdependencies between the sectors determine the sectoral and overall economic impact.
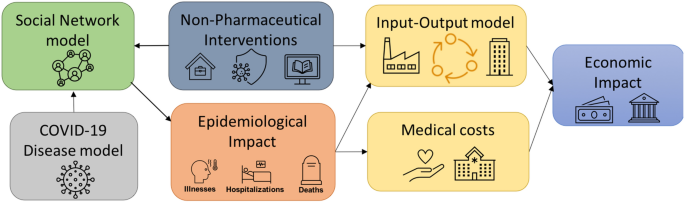
This figure shows the overall modeling framework, its various components and their linkages.
Data and methods
This research integrates a variety of datasets to build a comprehensive model that includes individuals, their interactions, their health states over time as the disease spreads over the social contact network, their behaviors in terms of compliance with interventions and its effect on their health states, and the impact of their health outcomes on each economic sectors’ labor supply and hence sectors’ output. These datasets include, but are not limited to, demographics data from the US Census, daily activity data from American Time Use Survey, travel data from National Household Travel Survey, location data from Open Street Maps, disease model parameters from the US Centers for Disease Control and Prevention, medical costs from Kaiser Family Foundation, data on dependencies between industries from the US Bureau of Economic Analysis, and telecommunting data from 17 . Further details on how these datasets and other data are used in our models are given in the subsections below. Figure 1 shows the overall systems level architecture of the modeling framework, its various components and how they are linked together. Below we describe the various models used in this framework and how they have been synthesized to build an integrated system.
Social contact networks
We use a synthetic social contact network generated using the methodology provided in 18 , 19 , 20 and used in 3 , 21 , 22 , 23 , 24 , 25 , 26 , 27 to study the spread of COVID-19. The social contact network is constructed using a first-principles approach that integrates various commercial and open databases through the following 4 steps. Step 1 constructs a synthetic population of the US by using datasetssuch as the US census block group level distribution data and Public Use Microdata Sample (PUMS) data. Step 2 assigns daily activities to individuals within each household using activity and time-use surveys (American Time Use Survey data and National Household Travel Survey Data). Step 3 assigns a geo-location to each activity that each person performs. The geolocations are based on data from Dun and BradStreet, land-use, Open Street Maps etc. Step 4 constructs a dynamic social bipartite visitation network, when people visit locations for performing activities. A dynamic social contact network is obtained from the colocation of individuals, where nodes are individuals and edges are the contact times. These types of networks have been validated and used to study various infectious diseases, interventions, and public health policy questions. For details on these studies and on the methodology to generate synthetic social contact networks, see 19 , 20 , 21 , 23 , 28 , 29 , 30 , 31 .
Each individual in the social network is endowed with a list of demographic attributes such as age, gender, income, occupation, family size, family income etc. consistent with the data provided by the US Census. A person’s occupation and the associated sector to which the occupation is linked, along with the health state (susceptible, infected or dead) of the person, are used to determine the sector level interruption in labor supply on any day that arises from sickness, mortality or stay-home order. This is the critical piece that joins the disease model with the economic model.
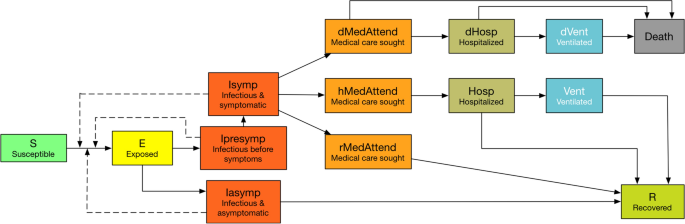
Disease states and transition paths in the COVID-19 disease model.
Disease model
The disease model is the best guess version of “COVID-19 Pandemic Planning Scenarios” prepared by the US Centers for Disease Control and Prevention (CDC) SARS-CoV-2 Modeling Team 32 . It is an SEIR (Susceptible-Exposed-Infectious-Recovered/Dead) model where each individual at any given time is in one of these health states. Everyone starts in the susceptible health state except for the seed nodes who begin in the infected state. Once a susceptible person is exposed to the disease, s/he stays in the exposed state for the incubation period. After that, they move to infectious state. The infected individuals are further divided into presymptomatic, asymptomatic, and symptomatic health states. Only the symptomatic individuals may seek medical care and some of them may become hospitalized while others recover. The hospitalized individuals may further need to be on ventilators. The final health state of the infected is either recovered or dead.
The disease states and transition paths are shown in Fig. 2 . The final disease state can be reached through multiple paths. The transition probabilities for each health state are shown in the Supplemental Information . The model is also age stratified for the following categories i.e. preschool (0–4 years), students (5–17) adults (18–49), older adults (50–64) and seniors (65+) and calibrated for each of the age groups separately. We use the disease model parameters as given by the CDC and do not analyze the sensitivities of disease model parameters to infections and deaths since our focus here is on understanding the effect of interventions and the parameters associated with interventions. The number of deaths simply depend on the number of infections. Age stratified probabilities of death are assigned to infected individuals at different stages of their health state. More details on the disease model, its parameters, and the dynamic values of effective reproduction number under different scenarios are available in the Supplemental Information .
Non-pharmaceutical interventions
We apply a number of social distancing strategies to mitigate the spread of COVID-19 3 . We assume there are no vaccines available and non-pharmaceutical interventions (NPI) are the only way to control the spread of COVID-19. We use the following NPI strategies: (i) Voluntary home isolation (VHI)—symptomatic people choose to stay at home (non-home type contacts are disabled) for 14 days. (ii) School closure (SC)—schools and colleges are closed (school type contacts are disabled). (iii) Stay home (SH)—a lockdown order directs people to “stay-home” (non-home type contacts are disabled).
School closure and stay-home interventions start on different days in different states as stated in 33 , 34 . Once closed, schools are assumed to remain closed until end of August. The duration and compliance to social distancing measures vary across scenarios as shown in Table 1 .
Stay-home durations are set at 0, 30, 45 to 60 days. Compliance to SH and VHI are set at 60%, 70%, 80% and 90%. Table 1 lists all the scenarios including the unmitigated one. For each experimental cell, 25 simulation replicates are run and results are shown based on the average values across these runs. Table 2 shows the parameters used in the experiments for easy reference.
Medical costs
Medical cost of treating COVID-19 patients under different health states are taken from 3 , 35 , which provide the average payment for treating pneumonia cases among “large employer health insurance” plans, and under different severity levels. See Table 3 . In the absence of COVID-19 treatment cost data, the pneumonia estimates have been used as a proxy. Note that each infected individual’s medical cost is counted only once. For example if a person is in ventilated state, after having gone through “medAttend” and “Hosp” state, costs are cumulative to the “vent” state 3 .
To estimate the medical costs of COVID-19 for each scenario, we multiply the number of medically attended, hospitalized, and ventilated with the estimated treatment costs per person given in Table 3 . This is repeated for each replicate in the simulation and the average estimates are reported. Note that an earlier paper focuses entirely on the medical costs 3 and provides more details on medical costs to the interested reader.
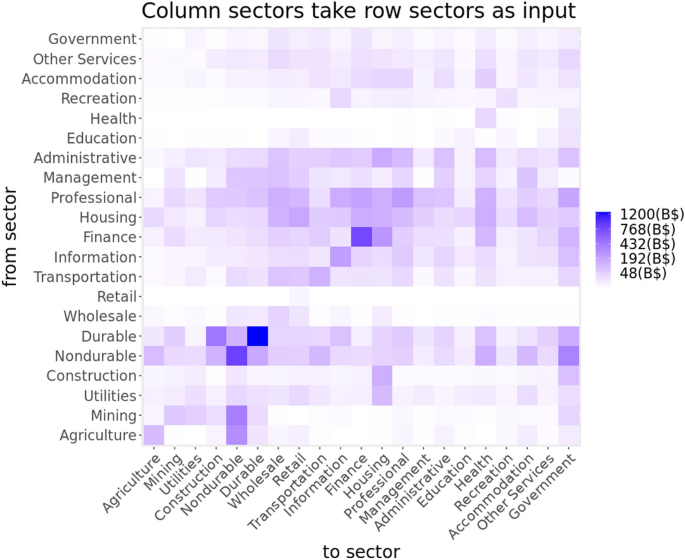
This figure shows interdependencies between sectors as given by the US Bureau of Economic Analysis. The left sector flows are input to right sectors.
Economic sectors and their interdependencies
We use the summary level input–output (I–O) tables for 2018 downloaded from the US Bureau of Economic Analysis (BEA) 36 , which quantify how industries depend on each other and interact with each other, to capture the cascading effect of labor supply shock across industries. The entire economy is divided into 71 industries; the I–O data reflects the structure of the US economy and the relative importance of each industry with respect to all other industries. We follow the NAICS (North American Industry Classification System) codes to aggregate the I–O data to sector level (21 sectors). Figure 3 shows the interdependencies between the 21 sectors. The row sectors of Fig. 3 provide input to the column sectors of the figure.
Data on telework by sector
During the stay-home order, some individuals are able to work from home. However, the ability to work from home (WFH) and the productivity of WFH workers vary by the type of sector the individuals are employed in. Authors in 17 , 37 estimate the number of jobs that can be done from home in the US. Work in 17 combines the feasibility of working from home by occupation, with occupational employment counts, and determines that 37% of all jobs in the US can be done from home.
Although this is not uniform across all sectors and cities; sectors like computing, education, legal and financial can be largely operational from home but construction, farming and hospitality cannot be 17 , provides the fraction of jobs that can be done from home by NAICS (North American Industry Classification System) and by SOC (Standard Occupational Classification) occupation. We use this fraction for each sector (as shown in Fig. 4 ) to determine the fraction of labor that can work from home. In addition 17 , provides the fraction of teleworkable wages for each sector. Together, these fractions determine the level of productivity that can be maintained during a lockdown by the healthy workforce in each sector. The health of each individual is tracked by the disease model given in section 3.2.
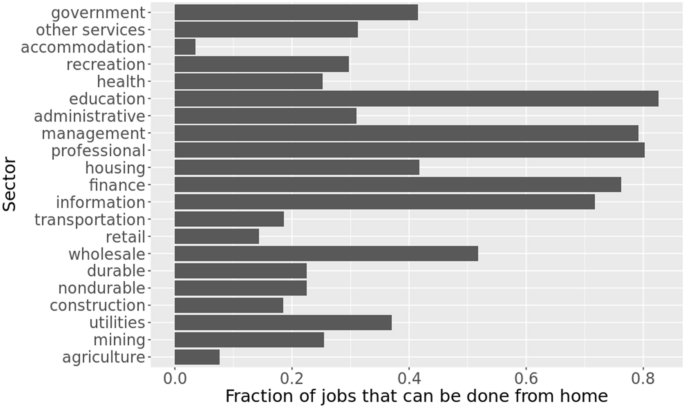
This figure shows the fraction of jobs in each sector that can be done from home. While jobs in accommodation, agriculture, retail, construction, and transportation sectors are difficult to be done from home, those in education, professional, management, finance, and information sectors can be largely operational from home.
Input–output model
We use the Dynamic Inoperability Input–Output Model (DIIM) stated in 16 , 38 to study the effect of labor supply shock arising from the morbidity and mortality caused by COVID-19, as well as the enforcement of the stay-home order, on national productivity. The DIIM model uses the classic input–output (I–O) economic analysis of Leontief (1935) 39 to account for the interdependencies between sectors.
I–O models have proven useful in accounting for the flows of goods and services across producing and consuming sectors of the economy. In the US, the Bureau of Economic Analysis is responsible for publishing I–O tables, gross domestic product, and other economic multipliers that are useful for conducting impact analysis of disasters. Each sector requires inputs from other sectors, and in turn produces outputs that are either used as intermediate inputs by other sectors or finished goods or services to satisfy exogenous final demands. Aside from the intermediate inputs, the so-called “value added” is a category of production inputs that are considered exogenous to the interdependent sectors. Within the “value added” is labor, which is the focus of this paper since it is the factor of production that is rendered “inoperable” by the pandemic. The DIIM quantifies the initial sector inoperability parameters by determining the extent to which labor is impacted in each sector.
Additionally, it allows modeling of resiliency parameters within the I–O model to signify sector wise recovery rates. Of particular relevance to this paper are resilience strategies, such as teleworking, that a sector can implement in order to reduce the impact of labor availability on its production of goods and services. We use the DIIM model to estimate the direct effect of drop in labor supply to each sector due to sickness, deaths and lockdown, as well as the indirect effect to sectors that arise due to interdependencies between sectors.
Depending upon the scenario considered in the simulation, appropriate interventions are applied to the social network. The interventions result in removal of edges on a temporal basis in the social contact network. For example, a stay-home order results in removal of all non-home edges of the compliant individuals for the duration of the order. The COVID-19 disease model is seeded and run on this time-varying social contact network over a period of one year. Everyone in the population is assumed to be susceptible at the beginning of the simulations except the seed nodes or the index cases, which are assumed to be infected. As the disease spreads through the network, the simulation generates a time series of daily infections. The infected individuals are further divided into medically attended, hospitalized, ventilated and dead, based on the probabilities assumed in the disease model.
To calculate the labor supply shock to each sector and its impact on productivity, we estimate (i) the number of infected and dead each day in each sector (using occupation and NAICS codes) and calculate the fraction of labor that is unable to work; (ii) the healthy individuals who comply with the stay-home order and do not go to work, and also cannot work from home given their occupation-type, as determined by telecommuting data for each sector 17 ; and (iii) healthy individuals who can work from home but their productivity is reduced as suggested in the teleworkable wages for each sector in 17 .
Results and discussion
We calculate the economic losses under the unmitigated scenario and the mitigation scenarios. Mitigation efforts help control the spread of the disease and hence reduce the total number of infections but they also increase the economic losses due to social distancing measures like the stay-home order. The compliance to NPIs and the length of the NPIs determine the extent of the loss, which can be weighed against the benefits measured in terms of reduced number of infections and deaths.
Economic losses due to inoperability and NPIs
Figure 5 shows the economic losses due to the inoperability of sectors under different NPIs and the infections caused by the pandemic. The left subfigure does not include the economic burden imposed by the treatment and medical services given to the infected individuals.
Lockdown and other social distancing measures reduce the labor supply to sectors but these measures do not uniformly affect each sector’s output. Depending upon how labor-intensive a sector is, how many jobs can be done from home, and how much value each job generates in a sector, the lockdown has a differential impact on each sector. For example, Education, Professional services, and Management sectors are teleworkable at 80% or higher levels whereas Accommodation (includes hospitality and food services) is at 3% and Agriculture is at 7%.
Inability to work from home in Construction and Agriculture sectors should imply more losses in these sectors. However we find that the losses are higher in Education, Professional services and Management sectors because jobs in these sectors pay more on average than the jobs in Construction and Agriculture sectors. Hence even a 20% loss in work in the former sectors can result in a higher total loss in value compared to a 90% loss in work in the latter sectors.
Overall economic losses from inoperability also depend on the level of dependency each sector has on others. Agriculture and Construction sectors have a higher level of dependency on other sectors compared to Education, Professional and Management sectors as shown in Fig. 3 . The lack of self-reliance increases the potential for losses caused by the cascading effect from other sectors.
The results in left Fig. 5 show that as the duration of SH order increases, the economic losses increase for a given compliance rate. This is because a longer SH order implies that healthy individuals are not able to work. A longer SH order also reduces the number of infections and deaths and hence improves labor supply and productivity. There is less absenteeism due to sickness and death, and less cascading effect on other sectors. The overall drop in productivity from a longer SH order shows that the gain in productivity from fewer infections and deaths is less than the loss from a longer shutdown. However SH order saves tens of thousands of lives and millions of infections as described in Section 4.4.
In the unmitigated base case, the economic loss is low but the loss due to morbidity and mortality is high. The healthy individuals are assumed to be working in the unmitigated scenario since no NPIs are in effect. The drop in productivity is caused only by the drop in labor supply due to illness and deaths since there is no lockdown in place. However, in the unmitigated case, more than 117,000 lives are lost and over 116 million infections occur.
Economic losses due to inoperability, NPIs and medical treatments
In Fig. 5 , the right subfigure shows the losses that are included in the left Fig. 5 plus the economic burden caused by the medical treatment of the ill. Note that the total loss in the unmitigated case without medical costs is $0.38 trillion in left Fig. 5 whereas with medical costs, this loss increases to $1.15 trillion as shown in the right Fig. 5 . The extra $0.8 trillion is solely due to the medical costs of treating infections in the unmitigated case. Note that for a given compliance level, a longer SH always results in a higher loss. However for a given SH duration, a higher compliance may result in a lower or higher loss. This would depend upon the relative gain from reduced infections versus the losses from SH of healthy individuals.
For example, in right Fig. 5 when the SH duration is set at 60 days, increasing compliance from 60 to 70% decreases the economic loss but increasing compliance from 70 to 80% increases the economic loss. This is because compliance has a non-linear effect on losses. At low levels of compliance, the marginal effect of a small increase in compliance is high because it helps get the pandemic under control which implies less absenteeism due to illness and lower medical costs. An increase in compliance from 70 to 80% does not have the same incremental effect on infections because 70% compliance is already quite effective, but has a large effect on the inoperability of sectors because a larger critical mass of workers are staying home.
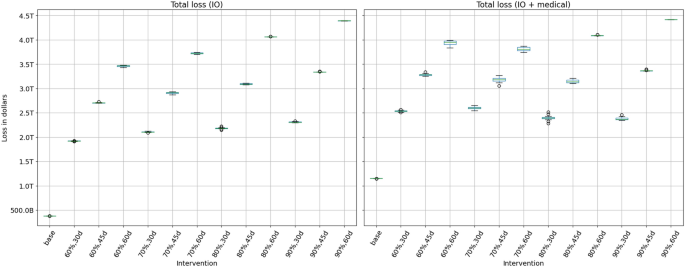
The left subfigure shows I–O economic losses (without medical costs) due to NPI measures and infections for each of the scenarios. The percentages show compliance to NPIs and “d” is for the duration of the Stay-home order. These losses arise from the drop in labor supply to sectors, caused by the lockdown, illness and mortality, and from interdependencies between sectors. It does not include the economic burden imposed by the treatment and medical services provided to the infected individuals, whereas the right subfigure includes this medical burden.
Trade-offs between compliance and duration of lockdown
Both subfigures in Fig. 5 show that there are tradeoffs between compliance and the length of the SH order. Low compliance can be compensated by a longer SH order and a shorter SH order can be combined with a higher compliance level to reach the same level of total loss. For example, in left Fig. 5 , a 60% compliance rate combined with a 60 days of SH results in similar total loss as a 90% compliance rate combined with a 45 days of SH.
The best outcome is reached when the lockdown is for 30 days and the compliance rate is at least 80%, as shown in the right Fig. 5 . It is clear that a lengthy SH order is harmful to the economy so a short SH order combined with a high level of compliance is ideal. Note that these tradeoffs and losses do not include the long term effect of deaths, i.e. the permanent loss in productivity, and only consider loss in labor supply for the duration of the simulation. The number of deaths depend on the duration and compliance to NPIs and are an important metric in measuring the outcomes. Later plots show the number of infections and deaths averted under each scenario.
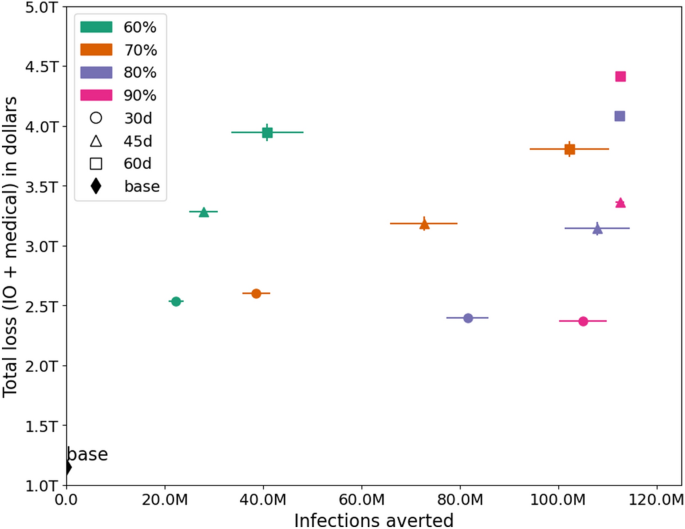
Trade-off between the number of infections averted and economic losses under each scenario. The vertical and horizontal bars show the inter-quartile range.
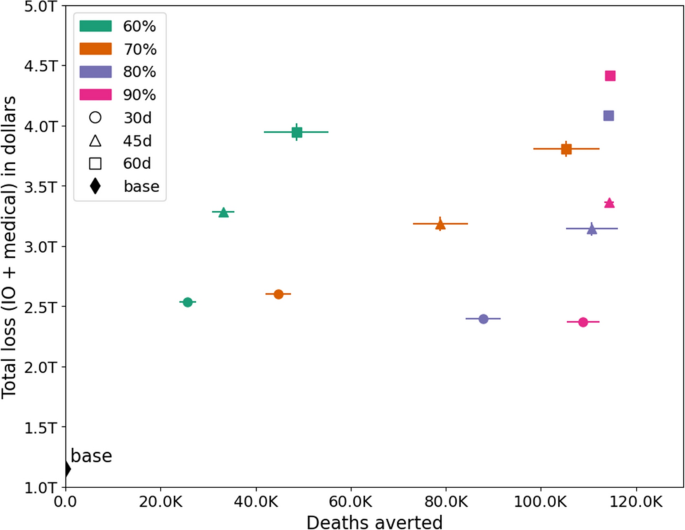
Trade-off between the number of deaths averted and economic losses under each scenario. The vertical and horizontal bars show the inter-quartile range.
Infections and deaths averted versus economic loss
Figures 6 and 7 show the trade-off between the number of infections-averted and economic losses, as well as the number of deaths-averted vs. economic losses respectively, under each of the intervention scenarios. Several important observations can be made from these plots: (i) The base case, where no NPIs are in place has the least loss but results in over 100 million infections and over 100,000 deaths. (ii) The trend for both morbidity and mortality is the same under different scenarios. (iii) Losses rise with longer durations of SH order. (iv) A SH order of 45 days results in same economic loss whether the compliance is at 70% or 80%. However the numbers of infections and deaths averted are much higher at 80% compliance. (v) Similarly, once 90% compliance is reached, an increase in SH duration from 45 to 60 days does not reduce infections and deaths but adds more than one trillion in economic losses. (vi) A longer lockdown can compensate for the lack of compliance and a higher compliance can reduce the duration of the lockdown in order to achieve similar number of infections and deaths but these trade-offs are non-linear. These kinds of analytics are useful in informing public health policy.
An intuitive display of differences in medical loss, total loss, infections averted and deaths averted, by scenarios, is shown through the heat maps in the Supplemental Information .
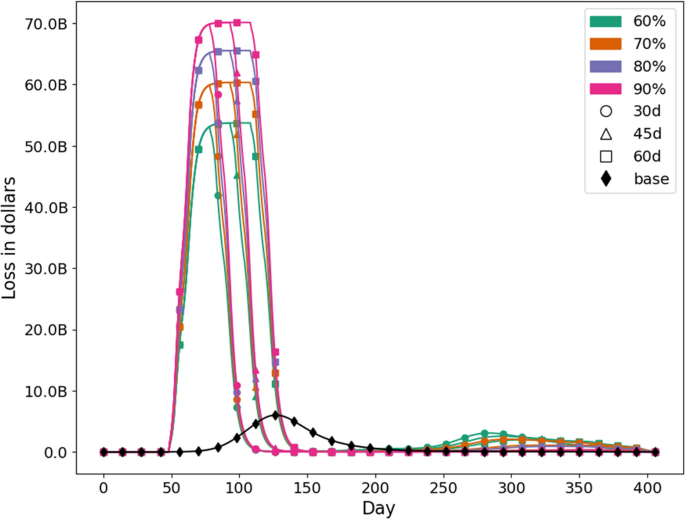
Daily I–O loss across all sectors for each scenario. The unmitigated base case is shown by the black curve where no NPIs are in place. Percentages are compliance to NPIs and “d” is the duration of SH order.
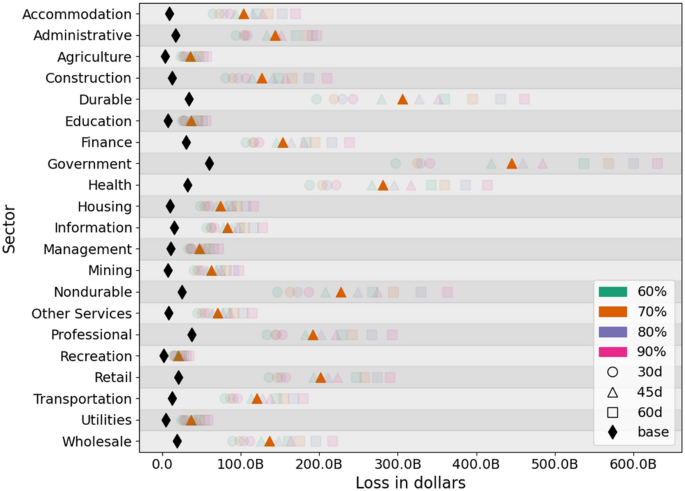
Sector level total loss for each scenario. The black and orange markers are highlighted to show the unmitigated and mitigated case \(VHI\_70\_SH\_70\_45\) respectively. Percentages are compliance to NPIs and “d” is the duration of SH order.
Sector level economic losses
We calculate sector level losses to understand how each sector will be impacted under different intervention scenarios. Figure 8 shows the daily loss across all sectors for each of the scenarios, including the unmitigated one. The percentages show compliance to VHI and SH and “d” reflects the duration of SH order. On the top the curves are clustered by the duration of SH order. The longer the SH order, the wider the top is; reflecting a more sustained loss at peak level during the lockdown period. Note that a second peak occurs only in scenarios where the compliance is low or compliance and duration both are low. As expected, the economic loss is higher in all intervention scenarios compared to the unmitigated scenario, since NPIs keep healthy people from going to work. However, as shown in Figs. 6 and 7 , these NPIs are able to avert over 100 million infections and over 100,000 deaths.
Figure 9 shows total loss for each sector and for each scenario, across time. The unmitigated case, marked in black, shows the least amount of economic loss since there are no NPIs in place. For comparative analysis, we select a medium level scenario, \(VHI\_70\_SH\_70\_45\) , and discuss in more detail. This is highlighted and marked in orange. In most of the cases, the sectors that encounter biggest losses are Government, Durables, Health and Non-durables.
Figure 10 shows a detailed comparison of sectoral loss for the unmitigated and a mitigated scenario over time. These do not include any medical costs. The left subfigure shows that without any mitigation, the highest losses occur in Government, Professional, Durables, Health and Finance sectors. Note that these losses are caused by loss in labor force due to sickness and deaths. There are no NPIs in effect in the unmitigated case. Even though inoperability is higher in sectors like Agriculture, Construction and Accommodation which tend to be more labor intensive, the value generated by the same proportional loss in labor is higher in Government, Professional, Durables, Health and Finance sectors due to their higher per capita productivity.
Detailed analysis of an intervention scenario
Here we provide a detailed sector level analysis of one of the 12 mitigation scenarios. We pick \(VHI\_70\_SH\_70\_45\) as an example case since it represents a mid-level scenario. The right subfigure in Fig. 10 shows daily losses in each of the sectors under this scenario and relative rankings of sectors when NPIs are in effect. The top 5 sectors in terms of biggest economic losses are Government, Durables, Non-durables, Health and Retail.
Even though the inoperability in these sectors is not that high due to NPIs, these sectors have higher wages and represent higher values compared to sectors which are more labor intensive. Top 5 sectors that have the highest inoperability due to labor supply shock from mitigation are Accommodation, Retail, Agriculture, Transportation and Construction but their losses are relatively low because of the low wages in these sectors. Other major factors that affect the losses in each sector are the extent to which the employment and wages are teleworkable. For example, in the unmitigated case, the worst performers include Finance because even a small shock to labor supply in this sector causes a big loss in value compared to a similar shock to sectors like Agriculture; but in the mitigated case, Finance sector performs relatively better because 76% of its jobs and 85% of the wages in Finance are teleworkable, whereas in Agriculture it is only 7% and 13% respectively.
Even in the mitigated case the Government sector has the highest loss, partly because it is also the largest sector in the economy and partly because it has a very high dependency on Durables, Non-durables and Professional which themselves are hit hard. Additionally in the Government sector, only 41% of the jobs and 46% of the wages are teleworkable.
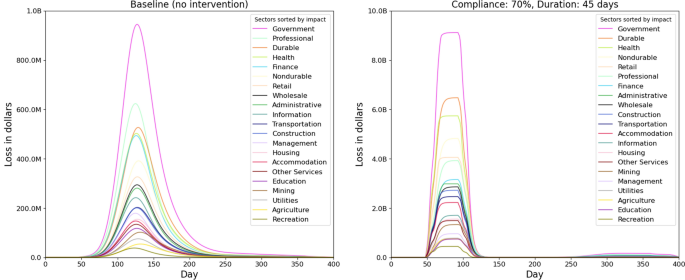
Sector level daily losses caused by the inoperability of each sector and its cascading impact on other sectors due to interdependencies between sectors. The left subfigure shows losses for the unmitigated case. The right subfigure shows losses for the mitigated scenario VHI_70_SH_70_45. The ordering of sectors in the legend is ranked by the height of the curve. Note that the scale of loss (y-axis) in the right subfigure is ten times of the left subfigure.
Best mitigation scenario
The best mitigation scenario in terms of lives saved and infections averted is when the compliance is at 90% and SH duration is 45 days. See Figs. 6 and 7 . This scenario results in a total economic loss of about $3.4 trillion dollars. However, it also saves more than 110,000 lives and 115 million infections compared to the unmitigated case. Assuming US federal government’s estimate of value of life which is $10 million per person 40 , 41 , lowering the number of deaths would save $1.1 trillion and lowering number of infections would save medical costs equivalent to $0.8 trillion, resulting in a gain of about $1.9 trillion from the mitigation efforts and a net economic loss of $1.5 trillion. This kind of simulation based analysis can help prioritize epidemiological and economic goals, understand their trade-offs, and guide public health policy.
Limitations
This study does not consider the demand side shock to the economy that results in drop in demand for goods and services due to lower employment, lost wages, and uncertain economic conditions. Unlike the general equilibrium model where demand and supply shock result in price adjustment, the input–output model does not capture the price dynamics that arise from changes in demand and supply. The treatment costs are average costs for treating pneumonia patients as available from 35 which do not vary by age, but only by severity of the case and these are used as proxies for COVID-19 medical costs.
Summary and conclusions
This study estimates the epidemiological and economic impact of several counterfactual intervention scenarios to contain the spread of COVID-19. Results show that any intervention involving a stay-home order will result in significant economic losses. However, the epidemiological impact of these interventions is dramatic. We find that interventions scenarios involving 45 days of SH order and a high compliance to NPIs can save more than 110,000 lives and 115 million infections compared to the unmitigated case.
We perform a sector level impact analysis and find that losses depend on the level of labor supply shock, the ability of employees to work from home, the productivity of workers who work from home and the dependency between sectors. The sectors that are more labor intensive such as Agriculture and Construction are not the worst performers because the per capita value generated is lower in these sectors compared to sectors like Government and Health.
Our results also show trade-offs between the economic losses and the number of deaths and infections averted. A longer lockdown and/or a high compliance to NPIs result in higher economic losses but save lives and reduce the number of COVID-19 infections. There is also a trade-off between duration of the lockdown and the rate of compliance to NPIs. If people are non-compliant to NPIs, public health policy-makers can increase the duration of the lockdown to get the same level of results in terms of infections and deaths averted.
Data availability
All the output data reported in the paper is available upon request, but restrictions apply on the commercially available data used in the construction of the social contact network and hence the availability of the social network data itself.
Code availability
Code developed to analyze the results and support the findings in this paper is available upon request, from the corresponding author.
Coibion, O., Gorodnichenko, Y. & Weber, M. Labor markets during the covid-19 crisis: A preliminary view (Tech. Rep, National Bureau of Economic Research, 2020).
New York Times. Covid in the u.s.: Latest map and case count. https://www.nytimes.com/interactive/2020/us/coronavirus-us-cases.html (2020). [Online, accessed November 24, 2020].
Chen, J. et al. Medical costs of keeping the us economy open during Covid-19. Sci. Rep. 10 , 1–10 (2020).
Google Scholar
Eichenbaum, M. S., Rebelo, S. & Trabandt, M. The Macroeconomics of Epidemics . Working Paper 26882, National Bureau of Economic Research (2020). http://www.nber.org/papers/w26882 .
Chen, D. & Zhou, T. Evaluating the effect of Chinese control measures on covid-19 via temporal reproduction number estimation. PLoS ONE 16 , e0246715 (2021).
Article CAS Google Scholar
Liu, Q.-H. et al. The covid-19 outbreak in Sichuan, China: Epidemiology and impact of interventions. PLoS Comput. Biol. 16 , 66 (2020).
Article Google Scholar
Acemoglu, D., Chernozhukov, V., Werning, I. & Whinston, M. D. Optimal Targeted Lockdowns in a Multi-group Sir Model (2020).
Baker, S. R., Bloom, N., Davis, S. J. & Terry, S. J. Covid-induced economic uncertainty (Tech. Rep, National Bureau of Economic Research, 2020).
Toda, A. A. Susceptible-infected-recovered (sir) dynamics of covid-19 and economic impact. (2020) https://arxiv.org/pdf/2003.11221.pdf
Jones, C. J., Philippon, T. & Venkateswaran, V. Optimal mitigation policies in a pandemic: Social distancing and working from home (Tech. Rep, National Bureau of Economic Research, 2020).
Barro, R. J., Ursúa, J. F. & Weng, J. The coronavirus and the great influenza pandemic: Lessons from the “panish flu” for the coronavirus’s potential effects on mortality and economic activity (Tech. Rep, National Bureau of Economic Research, 2020).
Correia, S., Luck, S. & Verner, E. Pandemics Depress the Economy, Public Health Interventions Do Not (2020).
Smith, R. D., Keogh-Brown, M. R., Barnett, T. & Tait, J. The economy-wide impact of pandemic influenza on the UK: A computable general equilibrium modelling experiment. Brit. Med. J. 339 , b4571 (2009).
Brahmbhatt, M. & Dutta, A. On SARS Type Economic Effects During Infectious Disease Outbreaks . Policy Research Working Papers (2008) http://hdl.handle.net/10986/6440 .
Santos, J. Perspectives on the effects of pandemic mitigation and suppression measures on interdependent economic sectors. Sustain. Prod. Consum. 66 , 249–255 (2020).
Orsi, M. J. & Santos, J. R. Probabilistic modeling of workforce-based disruptions and input–output analysis of interdependent ripple effects. Econ. Syst. Res. 22 , 3–18 (2010).
Dingel, J. I. & Neiman, B. How many jobs can be done at home?. J. Public Econ. 189 , 104235 (2020).
Bisset, K. & Marathe, M. A cyber-environment to support pandemic planning and response. DOE SciDAC Mag. 66 , 36–47 (2009).
Barrett, C. et al. Generation and Analysis of Large Synthetic Social Contact Networks (2009).
Halloran, M. E. et al. Modeling targeted layered containment of an influenza pandemic in the United States. In Proceedings of the National Academy of Sciences (PNAS) , 4639–4644 (2008).
Chen, J., Marathe, A. & Marathe, M. Feedback between behavioral adaptations and disease dynamics. Sci. Rep. 8 , 1–15 (2018).
Dorratoltaj, N. et al. Epidemiological and economic impact of pandemic influenza in Chicago: Priorities for vaccine interventions. PLoS Comput. Biol. 13 , e1005521 (2017).
Eubank, S. et al. Modelling disease outbreaks in realistic urban social networks. Nature 429 , 180–184 (2004).
Article ADS CAS Google Scholar
Chen, J. et al. Networked epidemiology for covid-19. SIAM News 53 , 3–7 (2020).
Adiga, A. et al. Evaluating the impact of international airline suspensions on the early global spread of covid-19 . Tech. Rep. (medRxiv (2020).) https://www.medrxiv.org/content/10.1101/2020.02.20.20025882v2.full.pdf .
Machi, D. et al. Scalable epidemiological workflows to support covid-19 planning and response . Tech. Rep. SC-TR-2020, Network Systems Science and Advanced Computing Division, BII, University of Virginia (2020). https://www.dropbox.com/s/2uk2wnbte95mk5y/main.v87.7020f2c.pdf?dl=0
Singh, M. et al. Impact of demographic disparities in social distancing and vaccination on influenza epidemics in urban and rural regions of the United States. BMC Infect. Dis. 19 , 221 (2019).
Barrett, C., Eubank, S. & Marathe, M. An interaction-based approach to computational epidemiology. In AAAI Conference on Artificial Intelligence 1590–1593 (2008).
Eubank, S. et al. Detail in network models of epidemiology: Are we there yet?. J. Biol. Dyn. 4 , 446–455 (2010).
Article MathSciNet Google Scholar
Marathe, A. et al. Comparing effectiveness of top-down and bottom-up strategies in containing influenza. PLoS ONE 6 , e25149 (2011).
Rivers, C., Lofgren, E., Marathe, M., Eubank, S. & Lewis, B. Modeling the impact of interventions on an epidemic of Ebola in Sierra Leone and Liberia. PLoS. Curr. 6 , 66 (2014).
Centers for Disease Control and Prevention. Covid-19 pandemic planning scenarios. https://www.cdc.gov/coronavirus/2019-ncov/hcp/planning-scenarios-h.pdf (2020). [Online, accessed July 8, 2020].
Education Week. Map: Coronavirus and school closures. https://www.edweek.org/ew/section/multimedia/map-coronavirus-and-school-closures.html (2020). [Online, accessed June 30, 2020].
Wikipedia contributors. U.S. state and local government response to the covid-19 pandemic. https://en.wikipedia.org/wiki/U.S._state_and_local_government_response_to_the_COVID-19_pandemic (2020). [Online, accessed June 30, 2020].
Rae, M., Claxton, G., Kurani, N., McDermott, D. & Cox, C. Potential costs of coronavirus treatment for people with employer coverage. Peterson Center on Healthcare and Kaiser Family Foundation vol. 13, (2020). https://www.healthsystemtracker.org/brief/potential-costs-of-coronavirus-treatment-for-people-with-employer-coverage/
Bureau of Economic Analysis. Input–output accounts data. https://www.bea.gov/industry/input-output-accounts-data . Accessed on April 30, 2020.
Bick, A., Blandin, A. & Mertens, K. Work from home after the covid-19 outbreak. CEPR Discussion Paper No. DP15000 (2020).
Lian, C. & Haimes, Y. Y. Managing the risk of terrorism to interdependent infrastructure systems through the dynamic inoperability input–output model. Syst. Eng. 9 , 241–258 (2006).
Leontief, W. W. Quantitative input and output relations in the economic systems of the united states. Rev. Econ. Stat. 66 , 105–125 (1936).
Merrill, D. No one values your life more than the federal government . Bloomberg (2017) https://www.bloomberg.com/graphics/2017-value-of-life
Alvarez, F. E., Argente, D. & Lippi, F. A simple planning problem for covid-19 lockdown Tech. Rep, National Bureau of Economic Research (2020).
Download references
Acknowledgements
This work was partially supported by National Institutes of Health (NIH) Grant R01GM109718, NSF BIG DATA Grant IIS-1633028, NSF DIBBS Grant ACI-1443054, NSF Grant No.: OAC-1916805, NSF Expeditions in Computing Grant CCF-1918656, CCF-1917819, US Centers for Disease Control and Prevention 75D30119C05935, DTRA subcontract/ARA S-D00189-15-TO-01-UVA, and collaborative seed grants from the UVA Global Infectious Disease Institute. The content is solely the responsibility of the authors and does not necessarily represent the official views of the sponsoring agencies.
Author information
Authors and affiliations.
Network Systems Science and Advanced Computing Division, Biocomplexity Institute, University of Virginia, Charlottesville, VA, 22904, USA
Jiangzhuo Chen, Anil Vullikanti, Srinivasan Venkatramanan, Stefan Hoops, Henning Mortveit, Bryan Lewis, Stephen Eubank, Madhav Marathe, Chris Barrett & Achla Marathe
Department of Computer Science, University of Virginia, Charlottesville, USA
Anil Vullikanti, Madhav Marathe & Chris Barrett
Department of Engineering Management and Systems Engineering, George Washington University, Washington, DC, 20052, USA
Joost Santos
Department of Engineering Systems and Environment, University of Virginia, Charlottesville, USA
Henning Mortveit
Department of Public Health Sciences, University of Virginia, Charlottesville, USA
Wen You, Stephen Eubank & Achla Marathe
You can also search for this author in PubMed Google Scholar
Contributions
A.V., J.C., A.M., M.M. and C.B. conceived the project. J.C., S.H., H.M., A.M., S.E., S.V., J.S. and B.L. built the model and the software. A.V., J.C., J.S., S.V., W.Y. and A.M. processed and analyzed the data. All authors helped write, edit and review the paper.
Corresponding author
Correspondence to Achla Marathe .
Ethics declarations
Competing interests.
The authors declare no competing interests.
Additional information
Publisher's note.
Springer Nature remains neutral with regard to jurisdictional claims in published maps and institutional affiliations.
Supplementary Information
Supplementary information., rights and permissions.
Open Access This article is licensed under a Creative Commons Attribution 4.0 International License, which permits use, sharing, adaptation, distribution and reproduction in any medium or format, as long as you give appropriate credit to the original author(s) and the source, provide a link to the Creative Commons licence, and indicate if changes were made. The images or other third party material in this article are included in the article's Creative Commons licence, unless indicated otherwise in a credit line to the material. If material is not included in the article's Creative Commons licence and your intended use is not permitted by statutory regulation or exceeds the permitted use, you will need to obtain permission directly from the copyright holder. To view a copy of this licence, visit http://creativecommons.org/licenses/by/4.0/ .
Reprints and permissions
About this article
Cite this article.
Chen, J., Vullikanti, A., Santos, J. et al. Epidemiological and economic impact of COVID-19 in the US. Sci Rep 11 , 20451 (2021). https://doi.org/10.1038/s41598-021-99712-z
Download citation
Received : 07 December 2020
Accepted : 28 September 2021
Published : 14 October 2021
DOI : https://doi.org/10.1038/s41598-021-99712-z
Share this article
Anyone you share the following link with will be able to read this content:
Sorry, a shareable link is not currently available for this article.
Provided by the Springer Nature SharedIt content-sharing initiative
This article is cited by
Evaluating return on investment for cyberinfrastructure using the international integrated reporting framework.
- Winona G. Snapp-Childs
- David L. Hart
- Craig A. Stewart
SN Computer Science (2024)
The COVID-19 Pandemic and Routine Prenatal Care: Use of Online Visits
- Sadia Mobeen
- Joshua Fogel
- Allan J. Jacobs
Maternal and Child Health Journal (2024)
The optimisation of public health emergency governance: a simulation study based on COVID-19 pandemic control policy
Globalization and Health (2023)
Substance use in rural trauma patients admitted for motor vehicle injuries before and during the COVID-19 pandemic
- Toni Marie Rudisill
- Lucie Steinmetz
- James M. Bardes
Injury Epidemiology (2023)
Epidemic Management via Imperfect Testing: A Multi-criterial Perspective
- Giuseppe Palma
- Damiano Caprioli
- Lorenzo Mari
Bulletin of Mathematical Biology (2023)
By submitting a comment you agree to abide by our Terms and Community Guidelines . If you find something abusive or that does not comply with our terms or guidelines please flag it as inappropriate.
Quick links
- Explore articles by subject
- Guide to authors
- Editorial policies
Sign up for the Nature Briefing newsletter — what matters in science, free to your inbox daily.

ORIGINAL RESEARCH article
The covid-19 pandemic and economic growth: theory and simulation.
- School of Finance, Shandong University of Finance and Economics, Jinan, China
The outbreak of COVID-19 pandemic has caused profound consequences on world economy. In order to explore the long-term impact of the pandemic on economic growth and the effects of different policy responses, this paper combines economic theory with epidemiological model to construct an interdisciplinary model, in which labor supply is dynamically constrained by pandemic conditions. Analysis of model equilibrium suggests that outbreaks of infectious disease reduce labor supply and negatively affect economic output. The accumulation of health capital can suppress the spread of disease and improve the recovery rate of infected individuals, which will alleviate the labor supply constraint caused by the pandemic and lead to an increase in output and consumption. The model is then calibrated to Chinese economy. The simulation results imply that government's public health policy can enhance the role of health capital in promoting economic growth. But the marginal effect of certain policies is diminishing. Therefore, the government needs to balance pandemic prevention and control costs and marginal benefits when formulating public health policies. When the pandemic is under control, the resumption of production is feasible and the economic stimulus package could lead to economic recovery.
Introduction
In recent years, outbreaks of infectious diseases, such as severe acute respiratory syndrome (SARS) and Middle East respiratory syndrome (MERS), bring severe negative shock to the world economy. And, the cross-border trade and travel have facilitated the international spread of the pathogens ( 1 ).
By the end of 2019, several cases of COVID-19 have been identified in China. A total of 830 cases were confirmed between the discovery of the virus in early 2020 and the “Lockdown” of Wuhan city on 23 January 2020. The Chinese government has extended the Chinese New Year holiday to reducing population mobility across regions. And social distancing and community quarantine procedures are carried out to slow down the spread of the virus. By 16 February 2020, more than 70,000 cases had been confirmed nationwide, while the number of new cases continued to fall and the number of cured cases rose sharply. After hard fighting against the pandemic, Wuhan City reopens at 8 April. Since then, only occasional imported infections have been detected and properly handled in a couple of regions. And there is no second wave of tremendous outbreak in China. On 23 May 2021, a newly-discovered COVID-19 patient in Guangzhou was confirmed to be infected with the “delta variant” of the novel coronavirus. Fortunately, under regional lockdown and strict epidemic control measures, regional spread was quickly blocked.
These facts reflect the importance of timely and effective infectious disease prevention and intervention measures by the government. However, the prevention and control of infectious diseases requires limiting resident mobility and aggregation and reducing social and economic activities, that significantly suppress both the demand and supply of the economy ( 35 ). At the same time, the fight against the pandemic delays the resumption of production in various industries and causes additional prevention costs, which greatly affects economic output. So, it is crucial for the authorities to balance the efforts to control the epidemic and the resumption of economic activity.
The main contribution of this paper to the existing literature lies in the following aspects: (i) In the theoretical model, through the endogenous health capital accumulation, the spread of infectious diseases is linked to the dynamics of labor supply in the economy, so as to realize the interdisciplinary integration of economic theory and infectious disease dynamics model; (ii) After+ deriving the differential equation of uninfected population from the infectious disease model, it is introduced into the central planner's optimization problem as a dynamic constraint for labor. The interdisciplinary theoretical framework makes it possible to obtain the steady-state of both endogenous economic variables and health capital and to ravel the dynamic nexus of COVID-19 pandemic and the macroeconomic fluctuation; and (iii) The simulation studies based on the calibrated model shed light on policymakers' trade-off between pandemic prevention and economic development. From a social-economic perspective, we discuss the effects of public health policy implementation and economic stimulus under different conditions.
The following sections of this paper are organized as follows: section Literature Review reviews existing literatures. Section Theoretical Model develops an interdisciplinary theoretical framework to explore multiple equilibria that may exist in the economic system during different stages of pandemic. The fourth section calibrates the model and perform steady-state analysis. The fifth section includes analysis of the policy response and the effects. And the last section concludes the paper.
Literature Review
We review related studies from following aspects: (i) the empirical evidence of economic consequences after the hit of pandemic; (ii) the pandemic induced poverty trap; (iii) the mechanism that how pandemic affects the economic growth; and (iv) the policy response.
Pandemic and Economic Growth
The economies of many low-income countries have been hit hard by infectious diseases. Gallup and Sachs ( 2 ) analyze the relationship between poverty and malaria for tropical and subtropical countries, and find that the areas with severe malaria have been in poverty for a long time and accompanied by anemic economic growth. For developed countries, Holtkamp et al. ( 3 ) assessed the impact of the swine fever epidemic on the US economy from 2005 to 2010 using data on agricultural enterprises disclosed by the US Department of Agriculture. The results showed that the average annual economic loss caused by the epidemic was about 664 million US dollars. Joo et al. ( 4 ) document a significant negative impact on South Korea's overall economic growth. Zhang et al. ( 5 ) illustrate that pandemic leads to unpredictable socioeconomic and long-term effects in low- and middle-income countries, and people or countries with lower socioeconomic status are worse off in these situations. Brown et al. ( 6 ) state that the COVID-19 pandemic has had a profound impact on American lives. In the months since the COVID-19 outbreak was first diagnosed, it has spread to more than 200 countries and all U.S. states. The pandemic has had a negative impact on global economic growth for more than nearly a century. Estimates to date suggest that the virus reduces global economic growth to an annualized rate of −4.5 to −6.0% in 2020.
Pandemic Induced Poverty Trap
The infectious diseases will weaken or even deprive the infected person's ability to work, which leads to lower income level and labor supply. As a result, there would be lack of investment and other factor input, which eventually suppresses economic growth. In addition, the impact of infectious diseases and related pandemic prevention measures on human capital accumulation will inhibit the improvement of production efficiency and weaken the momentum of economic growth.
First of all, infectious diseases directly lead to a decline in the income of infected persons, creating a poverty trap. For hundreds of years, the economies of most countries in the world have achieved rapid economic growth, but more than one-sixth of the world's population is still in poverty for a long time. This widespread and persistent poverty is closely related to infectious diseases [7, Gallup and Sachs, ( 2 )]. A large number of studies have shown that extreme poverty is often accompanied by infectious diseases in areas where poverty induces the occurrence and spread of diseases, and disease-driven poverty traps further restrict economic development ( 8 , 9 ). The reason for the formation of the poverty trap is that low-income people will quickly lose their means of production due to infectious diseases. After the epidemic is over, the lack of labor tools and labor capital has aggravated the persistence of poverty ( 10 ). Bonds et al. ( 11 ) and Ngonghala ( 12 ) integrated economic analysis on the basis of infectious disease models and found that in the poverty trap, initial economic, and epidemiological conditions determine the health of a society and the long-term trajectory of economic growth. The government's commitment to improving the health of the population is a necessary condition for getting out of poverty. Shen et al. ( 13 ) provide evidence that links rise in regional income inequality in China to the outbreak of COVID-19.
The Mechanism
The poverty trap caused by infectious diseases may cause economic growth to stagnate in the long run. Chakraborty et al. ( 14 ) believe that the poverty trap caused by infectious diseases will change the investment tendency of economic entities. At the same time, disease will directly lead to a decline in labor supply, and the input of factors in the economy will be significantly negatively affected. Therefore, in the long run, infectious diseases may cause a “growth trap”: the economy deviates from the original equilibrium growth path, and growth slows or even stagnates.
Infectious diseases weaken the accumulation of human capital and restrict the improvement of productivity. The impact of the spread of the virus on human health will eventually be transmitted to the economic production process through human capital channels ( 15 , 16 ). In the long run, the continuous spread and repeated outbreaks of infectious diseases will also reduce the life expectancy of the population, thereby further reducing the accumulation of human capital in society. This phenomenon is very common in low-income countries where AIDS and malaria are endemic ( 17 , 18 ). Fortson ( 19 ) studied the impact of the AIDS epidemic on human capital investment and economic growth in Africa. He found that areas with high HIV infection rates have a relatively large decline in education and a decline in human capital investment. The slow accumulation of human capital has dragged down the African economy. The theoretical research of Goenka and Liu ( 20 ) based on the endogenous growth model including human capital pointed out that there are multiple balanced growth paths for economic growth under the epidemic of infectious diseases. The restriction of infectious diseases on the accumulation of human capital is an important reason why many underdeveloped countries are unable to achieve economic growth. And the financial markets are also subject to the COVID-19 pandemic ( 21 ).
Policy Response
Zhang et al. ( 5 ) concluded from a review of the response to the COVID-19 pandemic in China and some other middle-income countries that the most important aspect of responding to an infectious disease outbreak is the preparedness and response of hospitals as the first line of defense against the pandemic. They argue that as the influenza season progresses, hospitals use the experience and lessons learned in the early stages of the pandemic to continually adapt their response to effectively deal with potential public health emergencies. And as Pardhan and Drydakis ( 22 ) point out that GDP per capita is associated with a lower rate of new COVID-19 cases, thus the economic performance should be a critical health priority for the policy makers.
Using combined the data of “SARS” cases with the epidemiological parameters of the COVID-19 pandemic, Yang et al. ( 23 ) adopt the method of recurrent neural network to carry out SEIR model estimation in different scenarios. They find that the government's swift intervention against the spread of the virus played a significant role in controlling the scale of infection. Goenka and Liu ( 24 ) analyzed the endogenous fluctuations of macro variables caused by infectious diseases in a growth model, and explored how to stabilize the macro economy through countermeasures such as vaccines or isolation. They argue that even when the virus cannot be completely eliminated, the prevention and control measures against the spread of the disease can smooth economic fluctuations and bring welfare gains.
Bogoch ( 25 ) use flight data from Guinea, Liberia, and Sierra Leone to study and found that thanks to timely flight control by the government, the number of passengers potentially infected with Ebola virus in the three countries decreased by 51, 66, and 85%, respectively. According to the counterfactual analyses by Qiu et al. ( 26 ), more than 1.4 million cases and 56,000 deaths may have been avoided as a result of the national and provincial public health measures imposed in late January in China.
In summary, existing studies have explored the negative impact of the pandemic on economy and the government's response measures. This paper introduces dynamic labor supply and time varying health capital accumulation in a broad sense into the canonical growth model. Our approach models the constraints imposed to the labor supply by the pandemic, and dynamically links government intervention, health capital, and disease transmission. We have developed an interdisciplinary theoretical model and discuss in depth the pandemic induced economic fluctuation and the government's response.
Theoretical Model
Almond ( 27 ) have pointed out that infectious diseases will directly increase the health costs of residents, hinder the accumulation of human capital, reduce labor time, and ultimately have a negative impact on economic growth in the long-term. Therefore, using the variable of labor supply as an intermediary can link the dynamic spread of infectious disease with the fluctuation of macroeconomy. Thus, it is able to carry out economic analysis under a pandemic shock [24, Augier and Yaly, ( 36 )].
This article starts from the perspective of infectious disease dynamics, and theoretically explores the differential equations that must be satisfied by the dynamic change of the labor force scale (healthy population) in the presence of an epidemic. At the same time, from an economic perspective, the most core supply and demand variables are closely related to labor. Residents provide labor for manufacturers to obtain income, and then allocate income to consumption and investment. Manufacturers use the labor provided by residents and the capital generated by investment accumulation to carry out product production activities. Therefore, through labor supply, a core macroeconomic variable, the impact of infectious diseases can be introduced into the economic model. At the same time, macroeconomic changes will change household income, affect the accumulation of health capital, and in turn change the spread of infectious diseases. The diagram of the interdisciplinary theoretical model is shown in Figure 1 .
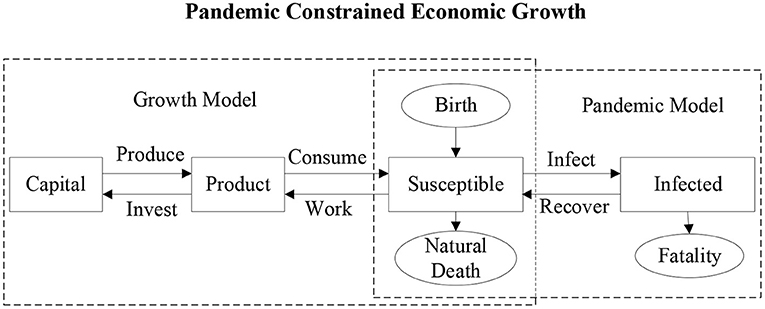
Figure 1 . Interdisciplinary theoretical model.
The Dynamic Spread of Infectious Diseases
The literature on mathematical modeling of the dynamic process of spread of infectious diseases can be traced back to Kermack and McKendrick ( 28 ). At present, the most widely used classical theoretical framework in the field of infectious diseases is the Susceptible-Infective-Susceptible (SIS) model ( 29 , 30 ). Different infectious disease dynamics models have certain differences in the settings. In the SIS model, the infected person will be cured (or self-healed) at a certain rate and reclassified into the susceptible population. For diseases that can obtain immunity after cure, according to the duration of immunity, they can be divided into two types: Susceptible-Infective-Recovered (SIR) and Susceptible-Infective-Recovered-Susceptible (SIRS). Among them, the situation where the cured population obtains permanent immunity applies the SIR model, and the situation where the cured population only obtains temporary immunity applies the SIRS model.
In order to ensure that the results will not underestimate the negative impact of the epidemic on the macro economy, we use the SIS model framework to characterize the dynamic spread of the novel coronavirus. The model assumes that the cured infected cases can be re-infected 1 . And in such settings, the threat of rapid virus mutation and the emerge of new variants will not be overlooked. Goenka and Liu ( 24 ) simplify the SIS model into a two-equation differential equation system under the additional assumption that the total population size is constant. They explore the endogenous fluctuations of macro variables caused by infectious diseases in a growth model. However, the assumption of population size is too strict to match economic reality. This paper follows the basic hypothesis of Gersovitz and Hammer ( 30 ) and believes that the population size is continuously increasing, which means that the birth rate is greater than the death rate.
We denote the total population during the period t as N t , which includes the susceptible population who are not infected by the virus N t , and the infected population I t 2 . Thus, the total population can be expressed as the sum of the numbers of two types of people:
The law of motion for the total population is characterized by Equation (2):
where, the birth rate and death rate are denoted as b and d , respectively.
Susceptible population will become infected because of contact with infected cases. Part of infected population will become susceptible again because of self-healing or being cured. Such a dynamic relationship can be expressed by the following differential equations:
Among them, the death rate of the infected person is set as d , the recovery rate is γ, and the contact rate between individuals is α. Under the assumption of random contact, the probability of susceptible people contact with infected cases is the proportion of infected people in the total population: i t = I t / N t . Naturally, the susceptible population accounts for the proportion of the total population is s t = S t / N t = 1 − i t . The death rate of infected cases is a redundant parameter for the above-mentioned dynamic system. For the sake of simplicity, it is set to be the same as the death rate of the whole population, which will not affect the subsequent analysis 3 .
By further simplifying the Equations (2, 3, 4), we can get:
It can be found that the change in the proportion of susceptible population is closely related to the birth rate, disease recovery rate, and the contact rate. Under the impact of the pandemic, the susceptible population constitute as the real labor supply in economic activities, not the total population. Therefore, Equation (5) can portrait the dynamic changes of labor supply in the economy when the pandemic is raging.
Government Intervention, Health Capital Accumulation, and Labor Supply
In this theoretical model, lowercase letters are used to represent the per capita level of each economic variable. According to the previous analysis, labor supply comes from uninfected population, formally L t = S t . Thus, according to Equation (5), we have:
Where, l t = L t / N t not only represents the proportion of uninfected people in the population, but also per capita labor supply.
Representative firm uses the physical capital and labor from representative household to produce products. The production function is:
The final product produced by the manufacturer is consumed by the household or invested in physical capital and health capital. The health capital is used to maintain the health of the labor force, and the physical capital is basic input for production activities. The product market clearing condition is:
Where, c t is consumption, physical capital and health capital investment are v t and e t , respectively.
The law of motion equations for the physical capital and health capital are:
For the newly increased population in the economy with a proportion of ( b − d ), the corresponding investment is needed to form the initial natural endowment of physical capital and health capital. The family's investment in physical capital is v t , and the depreciation rate is δ k . The family's investment in health capital is e t , and the depreciation rate of health capital is δ h .
This paper introduces health capital, which can be understood as the sum of capital investment closely related to disease prevention, public medical infrastructure and residents' medical care. On the one hand, health capital can be used for the prevention and control of infectious diseases, reducing the contact rate between infected and uninfected population, and thereby inhibiting the spread of the virus; on the other hand, the health capital can also be used for the treatment of infectious diseases to increase the recovery rate of infected cases.
Based on Smith and McKenzie ( 31 ) and Smith et al. ( 32 ) we set the contact rate and recovery rate parameters to be time-varying and subject to government's public health policies. The parameters are defined as functions of health capital as follows:
Where, the contact rate is a monotonic decreasing function of health capital, and the recovery rate is a monotonic increasing function of the level of health capital. From a purely mathematical point of view, they are endogenous variables closely related to other endogenous variables. But in order to be consistent with the previous literature, we choose to treat them as time-varying parameters. Their connotations are just the same as the corresponding parameters in the canonical infectious disease dynamics model. The only difference is that they will change over time due to fluctuations in health capital. The parameters ξ and ζ represent the government's efforts in preventing and controlling the spread of the virus and improving the level of medical treatment, respectively. The former achieves control of the epidemic by increasing investment in prevention and control measures such as disease prevention and control of personnel contacts; the latter achieves control of the epidemic by increasing investment in patient diagnosis and treatment facilities and vaccine drugs and other treatment measures. The greater the prevention and control of the spread of the virus (the greater the ζ), the lower the contact rate (the smaller the α) will be; the greater the investment in the medical treatment system (the greater the ζ), the greater the recovery rate (the larger the γ) will be. Therefore, under different policy combinations, limited health capital will be distributed differently, which impose different effects on the control of the pandemic and macroeconomic dynamics.
Utility Optimization
During the period of pandemic, the real labor supply in the economic system will be dynamically constrained by Equation (6), which in turn affects economic output and consumption. Therefore, compared with the canonical growth model, this paper emphasizes the influence of the pandemic on utility and production inputs. In order to explore the utility optimization problem of representative economic agents under the multiple constraints of economic and health conditions, this paper incorporates the variable of the proportion of uninfected population into the utility. The utility function is of the following form:
Not only the consumption, the proportion of uninfected population ( l t ) will also change the utility. The larger the proportion, the higher the utility level. If the economic system is not affected by the pandemic, which means l t = 1, then the utility function degenerates to the form widely used in the growth literature: U t ( c t , l t ) = ln ( c t ). Deviated from the literature, our set up makes it possible to depict the utility in both scenarios of pandemic shock and regular situation in one unified theoretical framework.
Different from the analysis of competitive equilibrium in the economy in the literature, this paper examines the equilibrium solution of the utility optimization problem from the perspective of the central planner. In central planner problem, labor in the economy will be affected by both the pandemic shock and government public policies. There will be endogenous interactions between labor force and health capital. For the central planner, the utility optimization problem can be written as:
where, the subjective discount rate is ρ, the corresponding constraints are Equations (6–11) and k t ≥ 0, h t ≥ 0, 0 < l t ≤ 1.
The Lagrangian function is written as:
We solve for the first-order condition:
In addition, the following conditions must be met:
Also, the following three transversality conditions should be met: lim t → ∞ e - ρ t λ 1 , t k t = 0 , lim t → ∞ e - ρ t λ 2 , t h t = 0 , lim t → ∞ e - ρ t λ 3 , t l t = 0 .
Based on the above conditions, it is obvious that the model has multiple equilibria. We start to analyze economic equilibrium from the dynamic Equation (6). When the equilibrium is achieved, all endogenous variables, both pandemic and economic ones, reach their steady-state values. And the market clears. Therefore, we let i t = 0 to get:
It means that the labor supply reaches a steady-state value and its growth rate is 0. It also means that under the government's intervention against the pandemic, the proportion of uninfected population in the economy also remains unchanged. It is easy to find there are two different equilibria, corresponding to l t = 1 and l t = ( b + γ)/α, respectively. The former equilibrium means that there is no spread of virus, and the proportion of population get infected in the economy is zero. This equilibrium state is similar to that of the classic growth model that does not subject to infectious disease. The latter equilibrium represents that although infectious diseases have not been completely eliminated, their marginal impact on labor supply has been offset by intervention measures and the accumulation of healthy capital, and the proportion of healthy people in the total population is in a stable state.
For economic variables x , we denote x - as the steady-state value in the equilibrium without any infectious diseases, and x ~ as the steady-state value in the equilibrium subject to a pandemic. Thus, there are two propositions can be obtained.
Proposition 1
When the economic system is not subjected to infectious disease, equilibrium values of labor, and health capital satisfy the following equations:
And the steady state capital will be:
When the economic system is not subjected to infectious disease, the whole population can provide labor, so l - = 1 . From Equations (15, 16, 18), we know that in the equilibrium, λ 5 > 0. Because the condition λ 5 h - = 0 holds, it is obvious that h - = 0 . Since Equation (17) equals 0, the steady state capital can be obtained by combining Equation (7,17).
It can be found that in the absence of infectious diseases, the steady state of the model is quite similar to that of canonical growth model. But the introduction of infectious disease transmission changes the results significantly. First, there are dynamic interactions among labor supply, health capital, and disease transmission; second, due to the investment in health capital, the optimal state of resource allocation deviates from the optimal steady state. Finally, the steady-state values of output and consumption have also changed due to the impact of infectious diseases. The following proposition defines the steady-state value of economic variables in the presence of infectious diseases.
Proposition 2
When there is an infectious disease, only uninfected population can provide labor. If the economy is in equilibrium, the labor supply satisfies l ~ - ( b + γ ( h ~ ) ) / α ( h ~ ) , and health capital satisfies 0 < ( b + γ ( h ~ ) ) / α ( h ~ ) < 1 . The steady-state values of capital and labor are determined by the following equations:
When the economic system is subjected to infectious disease, only uninfected people provide labor. The labor supply should satisfy l ~ ≠ 1 . According to Equation (21), the labor supply should be l ~ = ( b + γ ( h ~ ) ) / α ( h ~ ) , and the health capital is defined by the inverse function of health capital as h ~ = l ~ - 1 ( h ~ ) . At the same time, since 0 < l ~ < 1 holds, the inequality 0 < ( b + γ ( h ~ ) ) / α ( h ~ ) < 1 . Similar to the proof for Proposition 1, the steady-state value of capital can be obtained as Equation (24). In the equilibrium, Equations (18,19) are equal to 0, combined with Equations (15,16), the steady state value of labor can be obtained as shown in Equation (25).
Calibration and Steady State Analysis
Parameter calibration.
In order to further analyze the impact of pandemic on economic equilibrium, this paper calibrates the model to the Chinese economy and solve the steady-state values of macroeconomic variables. Based on the birth rate and death rate reported by National Bureau of Statistics of China from 2009 to 2019 4 , we set the birth rate ( b ) in the model to 1.2% and the death rate ( d ) to 0.7%. Based on the average Shibor interest rate in China from 1999 to 2019 5 , we set the discount rate (ρ) to 2.4%. In the production function, the productivity ( a ) is standardized to 1, and the capital share (η) is calibrated to be 0.33. We set the physical capital depreciation rate (δ k ) and the health capital depreciation rate (δ h ) to 5%. In the model, government's pandemic prevention and control efforts are captured by the parameter ξ in Equation (11), which is set to be 1.0. At the same time, the government's initiative to invest in medical treatment are captured by the parameter ζ which is also calibrated to be 1.0. The connotations and values of model parameters are summarized in Table 1 .
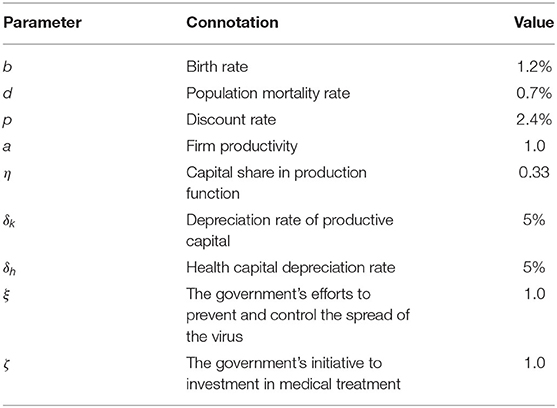
Table 1 . Model parameter calibration.
Steady State Analysis
Based on the calibrated model, we solve for the economic equilibrium before and after the outbreak of infectious disease. We assume that after the pandemic shock, the economy reaches the new equilibrium instantly. Two steady states are presented in Figure 2 for comparative analysis. The horizontal axis is time, and we assume that the time of outbreak is t = 0. Before the outbreak, the entire population in the economy was able to provide labor to manufacturers. After the outbreak, labor supply will be restricted by the spread of infectious diseases.
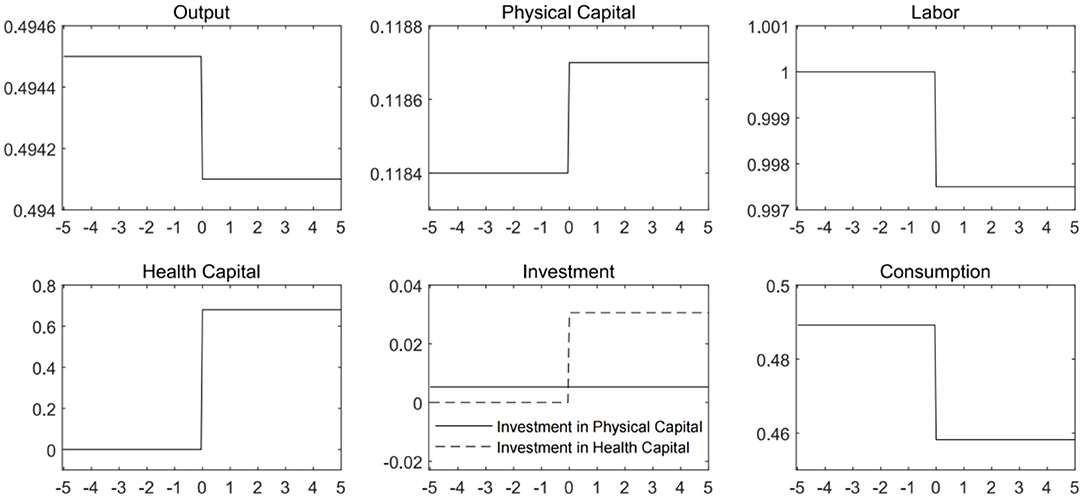
Figure 2 . Economic steady states and pandemic shock. It is assumed that the pandemic shock hit the economy at time t = 0. Before that, the economy is not subjected to any infectious disease.
Before the outbreak of infectious disease, there is no need to accumulate health capital. After the outbreak, some individuals are unable to work due to illness or the pandemic prevention and control measures. After the pandemic hitting economy, the equilibrium labor supply declines. In order to contain the spread of the virus, economic agents need to accumulate health capital. The investment in health capital increases from 0 to a positive level, and the stock of health capital rises since the outbreak. Due to the decrease in labor supply, physical capital investment rose slightly, but the increase is much smaller than that of health capital investment. The increase in physical capital cannot completely offset the negative impact on output caused by the reduction in labor supply. The steady-state value of aggregate output drops below the level before the pandemic outbreak. Along with the decrease in output and the increase in investment, consumption has dived significantly. These results are consistent with previous literatures such as Chakraborty et al. ( 14 ) and Goenka and Liu ( 20 ).
The above analysis justifies that outbreak of pandemic will directly lead to a decline in aggregate demand and output in the economy. At the same time, fighting the epidemic requires the accumulation of additional health capital. Healthy capital investment consumes part of the economic output. And the production of healthy capital will squeeze out the factor inputs required for the production of other products. The more investment flows into the health sector, the more effective the prevention and control of infectious diseases will be. As a result, there would be higher recovery rate for the infected population. The optimal health capital level is endogenously related to the dynamics of spread of the virus. When formulating relevant pandemic prevention and control policies, the government must consider the combination of different types of measures targeting the contact rate and recovery rate, as well as the trade-off between disease prevention and control and economic development.
Policy Analysis
Public health policy.
To contain spread of virus, the government should resort to serious measures. The most direct responses from the Chinese government are social distancing, mask wearing and quarantine the infected cases. After that, the government provides vigorously support for the research and development of vaccines and treatment methods. By doing so, it is able to improve the recovery rate of patients, and reduce the scale of infection.
The research conducted by Zhong Nanshan's team fully demonstrated the important role of the government's swift and effective prevention and control measures against the spread of the virus in curbing the pandemic ( 23 ). And our recognition of the positive impact of health capital accumulation in fighting the pandemic is quite consistent with them.
Besides that, we have considered the possibility that limitation of resources leads to the non-linear benefits of the accumulation of health capital, and also that excessive accumulation of health capital may bring undesirable consequences. The steady-state of health capital represents the optimal value under the dual goals of curbing the pandemic and ensuring economic growth. The changes in the government's public health policies will not only directly lead to changes in pandemic related parameters, but also affect health capital and other economic variables through the dynamic interaction between the spread of virus and health capital. In order to further discuss the choice of government's public health policy, we have solved for the steady-state values of economic variables with different parameters portrait the pandemic prevention and control efforts and medical treatment investment.
In Figure 3 , we plot the economic steady states that solved with different ξ values, which represent varied pandemic prevention and control efforts. From Equation (11), it can be inferred that the greater the government's efforts to prevent and control the spread of the virus, the lower the contact rate and the demand for health capital will be. Given that the proportion of the uninfected population in the total population follows the path of change described by the differential Equation (6), the decline in the contact rate can increase the supply of labor, and at the same time, the substitution effect of labor on capital causes a decrease in the level of physical capital investment. It can be seen from the figure that the comprehensive effect of changes in labor and capital on the production of firms is to promote the increase in output, while the consumption level rises accordingly. However, it is worth noting that under the condition that the intensity of medical treatment remains unchanged, the decline in health capital causes a decline in the recovery rate, which has a negative impact on labor supply and economic output.
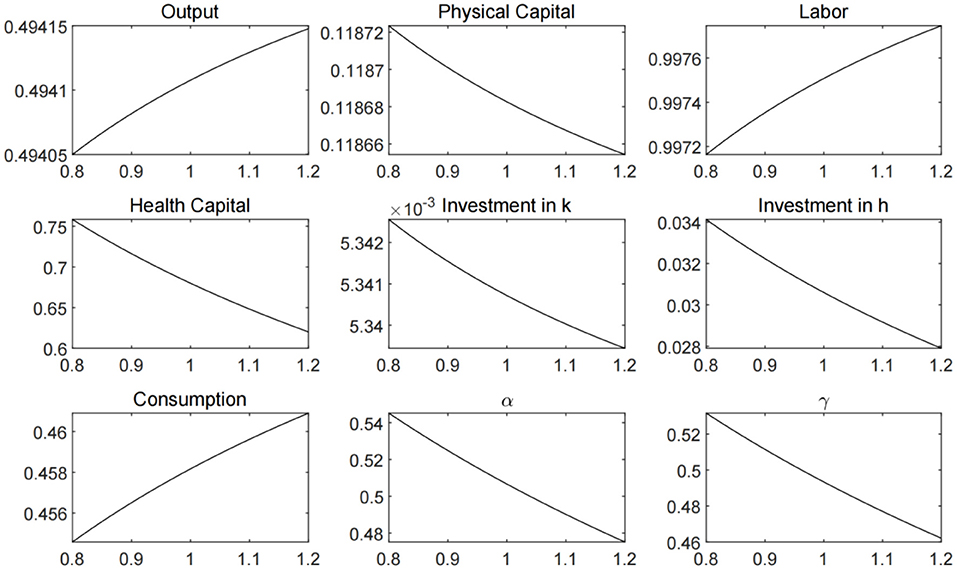
Figure 3 . Economic steady states under different ξ values.
In Figure 4 , we plot the economic steady states that solved with different ζ values, which represent government's initiative to investment in medical treatment. The increase in medical treatment investment can bring about the growth of labor supply, output and consumption. However, health capital investment will fall, triggering an increase in contact rates, and also have a negative impact on labor supply and economic output. Therefore, we need to further analyze the different combinations of public health policy parameters.
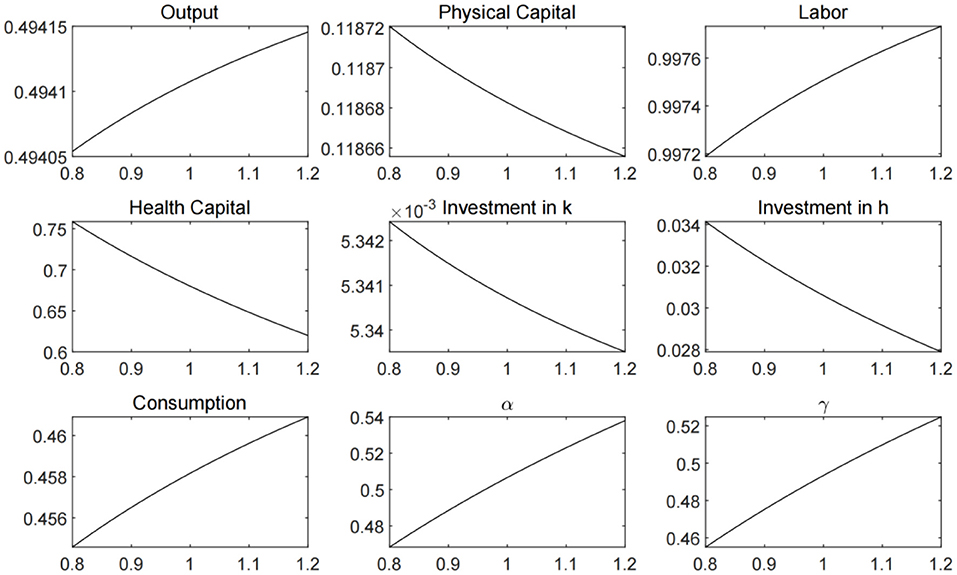
Figure 4 . Economic steady states under different ζ values.
In order to better analyze the effect of the government's public health policy, we set different values for the parameters ξ and ζ, and plot the steady states of labor, output, and consumption in Figure 5 . The results suggest that the strengthening of public health policies can increase labor supply in the economy and promote output and consumption. However, the marginal effect of public health policy is diminishing. When the parameters reach the level above 1.5, the marginal increment of output and consumption becomes extremely small. Moreover, for pandemic prevention and control measures or medical treatment investment policy alone, the effect on economic development is limited. Only when the two directions of public health policies are combined can we achieve the best results.
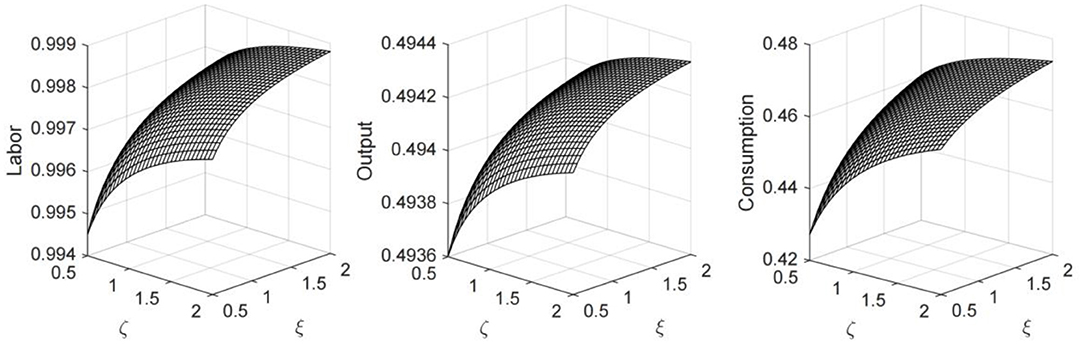
Figure 5 . Economic steady states under different combination of ξ and ζ values.
Economic Policy
The outbreak of the COVID-19 imposes severe constraints on labor supply and total demand. China has experienced economic austerity and predicament. The previous steady state analysis shows that the implementation of public health policies can lift the steady state of economic variables such as output and consumption to a certain extent. But further economic recovery requires more support and macroeconomic stimulus. When the spread of virus is gradually under control, the resumption of production and restore of social order become the priority for the government. However, the normalization of economic activity is accompanied by more frequent interpersonal interaction and contact, which may lead to higher probability of an outbreak. So, it is absolutely necessary to pay attention to the counterproductive effect of economic stimulus policy for the policy makers.
Resumption of Production
The China Enterprise Confederation established a survey on the resumption of work and production of China's top 500 manufacturing companies. 6 The survey shows that during the survey period from February 18 to 20, 97.08% of surveyed companies have resumed production, the average employee attendance rate was 66.17%, the average operating rate of member enterprises was 75.24%, and the average capacity utilization rate was 58.98%. The experiences from the firms that have been back to business imply that resuming work and production under the premise of doing a good job in epidemic prevention and control can directly increase capacity utilization and bring about an increase in productivity.
We are not able to directly investigate the epidemiological and economic impact of the resumption of firm production based on our model. But it can be inferred from the change of firm productivity and its consequences. The degree of enterprise's resumption of production is highly positively correlated with its productivity. We set different values for the productivity parameters to approximate the degree of resumption. And then, the economic steady states are calculated plotted in Figure 6 . There is a very intuitive conclusion that incremental productivity boost output and consumption. But the impact of productivity increase on labor is two-fold. On the one hand, the increase in productivity will reduce the demand for capital and labor by manufacturers. On the other hand, higher productivity level leads to output growth, which is beneficial for the health capital accumulation. The increase in health capital can reduce the infection and increase the recovery rate, resulting in better pandemic prevention and higher level of labor supply.
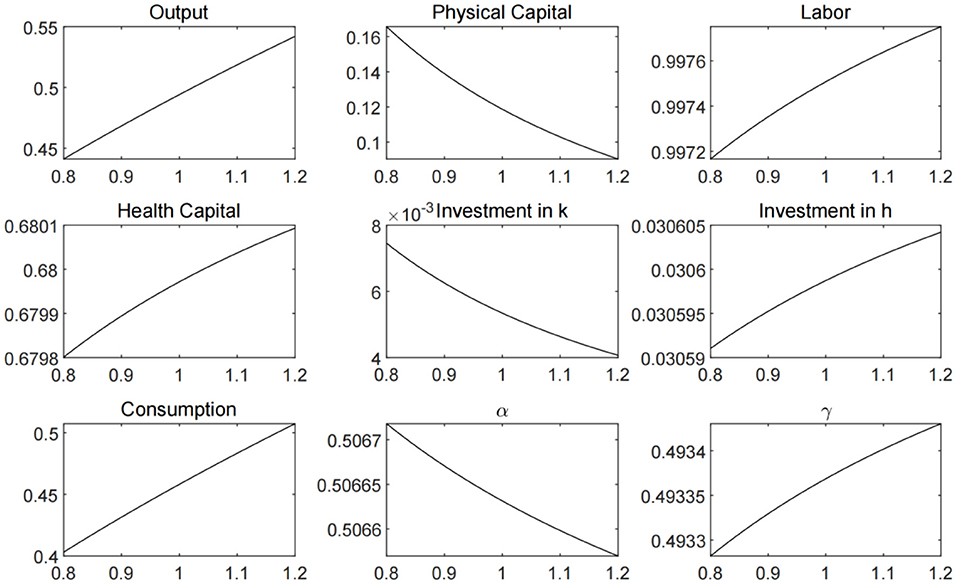
Figure 6 . Economic steady state under different productivity levels.
The above analysis suggests that as long as the resumption of production is conducted under the premise of splendid prevention and control measures, not only can it effectively stimulate economic recovery, but also help the accumulation of health capital and resulting in significant inhibitory effect on the pandemic. At the same time, timely and effective pandemic prevention and control are inseparable from the use of health capital. Overly strict prevention measures may cause delays in resuming work and production, and impose a negative impact on the accumulation of health capital. Therefore, it is necessary to carefully weigh the prevention costs brought by the resumption of work and production and the economic output.
Economic Stimulus Policy
After resumption of production, the economic activities are brought back gradually. In order to achieve economic recovery, most countries in the world have proposed economic stimulus plans. From the historical experience, infrastructure investment is one of the most direct and effective means to boost the economy, especially in the context of relatively insufficient domestic demand following the pandemic shock. Compared with stimulating consumption, increased investment is more likely to help the economy to recover effectively in the short term.
It's worth noting that different direction of infrastructure investment imposes differentiating impact on labor. There exists significant substitution of technology for labor in certain industries. Investing in such industries may actually lead to a decline in the labor demand that is already not optimistic after the pandemic shock. China is also facing downward pressure on the economic growth caused by deleveraging and trade frictions in the post pandemic period. In order to avoid extensive investment in traditional infrastructure investment, the Chinese government choose to identify a number of high-tech industries, like AI and 5G, as the destination of “new infrastructure” stimulus package 7 .
The logic that “new infrastructure” investment can promote output by increasing the scale of investment in the economy is intuitive. However, we also need to be aware of the potential “labor substitution” effect of this type of infrastructure investment. Schumpeter ( 33 ) pointed out that technological innovation will reduce the demand for labor in the production process and cause unemployment. As advanced forms of automation technology, high-tech applications such as artificial intelligence have the ability to replace part of mental labor, which may impose a more significant substitution effect on labor ( 34 ). As mentioned above, most of the investment in the “new infrastructure” goes to the high-tech fields. There is a complementary relationship between the capital leaned technological progress and capital investment, which will naturally impose substitution effect on labor. In order to explore the potential impact of increasing investment in capital-leaned high-tech industries on labor and output, we solve the steady state under different capital share and present the results in Figure 7 . It can be seen that when capital share rises, other macroeconomic variables decline, including labor, output, and consumption. More importantly, the increase in the share of capital will also crowd out the investment of health capital, which will further push up the contact rate and reduce the recovery rate.
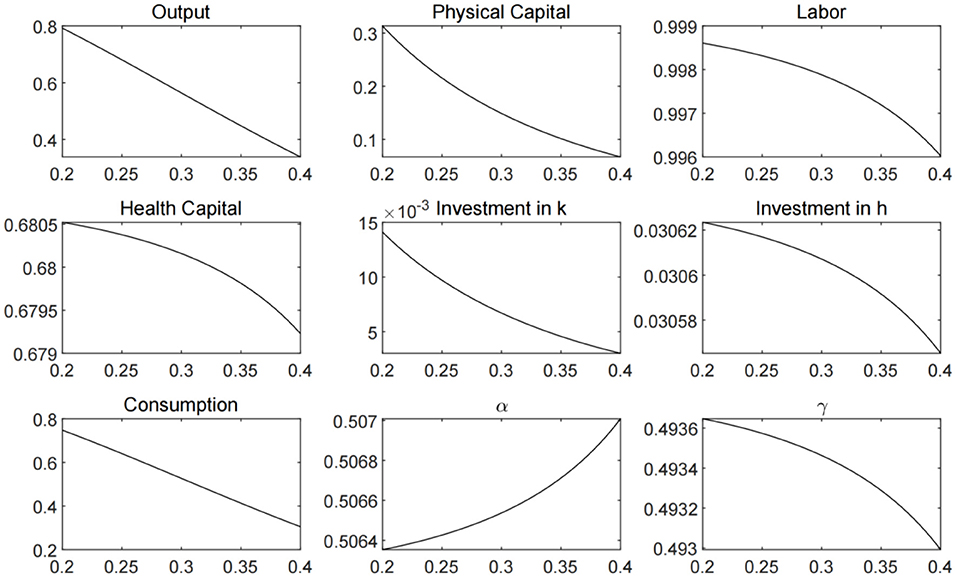
Figure 7 . Economic steady state under different capital shares.
Based on the above discussion, the government needs to carefully assess the potential impact on employment and output when increasing investment in high-tech industries. Optimal stimulus policies should consider the construction of both capital-intensive and labor-intensive projects, and weigh multiple policy goals such as stimulating output and ensuring employment. In this way, the excessive substitution of labor by technological progress and unemployment can be avoid.
This article combines economic theory with infectious disease models, and analyzes the long-term impact of the novel coronavirus pandemic on labor supply, household consumption and economic growth. And the simulation shed light on public health policies and economic stimulus policies under different conditions Implementation Effect.
The results show that the outbreak of COVID-19 pandemic directly affect labor supply and output, resulting in a significant negative impact on the economic growth. The accumulation of health capital can inhibit the spread of infectious diseases and improve the recovery rate. Thus, the pandemic prevention and control become more promising and ultimately lead to the growth of economic output and consumption. Public health policies can enhance the significant role of health capital in promoting pandemic prevention and economic growth, but the effects are marginal diminishing. So, overinvestment in public health should be avoided. And, only when the government increases investment in the prevention measures and medical treatment at the same time, the negative consequences of pandemic are able to be minimized. Resuming production and work is not only conducive to economic recovery, but also imposes a positive effect on pandemic prevention through the accumulation of health capital. Investing infrastructure is definitely an effective way to boost the economy. However, “new infrastructure” investment in high-tech industries may otherwise impose substitution effect on labor, which could push up unemployment rate. It is clear that only on the premise of effectively dealing with the spread of the epidemic, economic development is a viable option.
As the COVID-19 pandemic has repeatedly erupted around the world, China's experience has its unique value. In the early stage of the outbreak, strict quarantine measures, tracking of close contacts, and the control of social distancing had a considerable negative impact on the Chinese economy. However, the advantage is that the severe measures have created prerequisite for the rapid restoration of the social and economic order. The Chinese economy has begun a strong rebound in the second quarter after the outbreak of the epidemic.
The theoretical model constructed in this article is a highly abstract macroeconomic system under the pandemic. So, it has its limitations. This article assumes that all participants in the economic system are fully rational. The impact of the epidemic on economic agents' expectations and preventive savings are not considered in the model. And the model is not able to characterize the positive effect of vaccination on suppressing the spread of the virus. In future research, the authors hope to further correlate the dynamics of virus transmission with vaccines, economic man expectations, and financial activities within a dynamic general equilibrium framework.
Data Availability Statement
Publicly available datasets were analyzed in this study. This data can be found here: http://www.stats.gov.cn/ .
Author Contributions
LX: conceptualization, validation, writing original draft, and supervision. MT: methodology, writing—review and editing, and visualization. ZY: writing—review and editing, project administration, and funding acquisition. MZ: software, resources, and data curation. SL: formal analysis and investigation. All authors contributed to the article and approved the submitted version.
We acknowledge the financial support from Shandong Provincial Natural Science Foundation (Grant Number: ZR2020QG032), Shandong Provincial Social Science Planning Office (Grant Numbers: 21DGLJ12; 21DJJJ02), Taishan Scholars Program of Shandong Province, China (Grant Numbers: ts201712059; tsqn201909135), and Youth Innovative Talent Technology Program of Shandong Province, China (Grant Number: 2019RWE004). All errors remain our own.
Conflict of Interest
The authors declare that the research was conducted in the absence of any commercial or financial relationships that could be construed as a potential conflict of interest.
Publisher's Note
All claims expressed in this article are solely those of the authors and do not necessarily represent those of their affiliated organizations, or those of the publisher, the editors and the reviewers. Any product that may be evaluated in this article, or claim that may be made by its manufacturer, is not guaranteed or endorsed by the publisher.
1. ^ On January 31, 2020, the National Health Commission of China stated at a press conference that patients who have recovered from the new coronavirus pneumonia are subject to reinfection. On October 27, 2020, the CDC of USA confirmed cases of reinfection.
2. ^ This paper chooses the more intuitive term “uninfected population” when discussing relevant economic scenarios. In the following text, we will use “susceptible people” and “uninfected people” interchangeably.
3. ^ See Gersovitz and Hammer ( 30 ) for a detailed discussion.
4. ^ Data source: official website of the National Bureau of Statistics of China.
5. ^ Shibor stands for the Shanghai inter-bank offering rate.
6. ^ Survey report available at: https://www.acfic.org.cn/zt_home/zcmq2020/zcmq2020_dt/202002/t20200226_158162.html .
7. ^ The high-tech industries include: (1) 5G base station construction; (2) UHV; (3) Intercity high-speed railway and urban rail transit; (4) New energy vehicle charging pile; (5) Big data; (6) Artificial intelligence; (7) Industrial Internet.
1. Wu T, Perrings C, Kinzig A, Collins JP, Minteer BA, Daszak P. Economic growth, urbanization, globalization, and the risks of emerging infectious diseases in China: a review. Ambio. (2017) 46:18–29. doi: 10.1007/s13280-016-0809-2
PubMed Abstract | CrossRef Full Text | Google Scholar
2. Gallup JL, Sachs JD. The economic burden of malaria. Am J Trop Med Hyg . (2000) 64(1–2 Suppl.):85–96. doi: 10.4269/ajtmh.2001.64.85
3. Holtkamp DJ, Kliebenstein JB, Neumann EJ, Zimmerman JJ, Rotto HF, Yoder TK, et al. Assessment of the economic impact of porcine reproductive and respiratory syndrome virus on US pork producers. J Swine Health Prod. (2013) 21:72–84. doi: 10.31274/ans_air-180814-28
4. Joo H, Maskery BA, Berro AD, Rotz LD, Lee YK, Brown CM. Economic impact of the 2015 MERS outbreak on the Republic of Korea's tourism-related industries. Health Secur. (2019) 17:100–8. doi: 10.1089/hs.2018.0115
5. Zhang J, Lu X, Jin Y, Zheng Z. Hospitals' responsibility in response to the threat of infectious disease outbreak in the context of the coronavirus disease 2019 (COVID-19) pandemic: Implications for low- and middle-income countries. Global Health J. (2020) 4:113–7. doi: 10.1016/j.glohj.2020.11.005
6. Brown KM, Lewis JY, Davis SK. An ecological study of the association between neighborhood racial and economic residential segregation with COVID-19 vulnerability in the United States' capital city. Ann Epidemiol. (2021) 59:33–6. doi: 10.1016/j.annepidem.2021.04.003
7. Bloom DE, Sevilla CJ. Geography and poverty traps. J Econ Growth. (2003) 8:355–78. doi: 10.1023/A:1026294316581
CrossRef Full Text | Google Scholar
8. Farmer P. Infections and Inequalities: The Modern Plagues . Berkeley, CA: University of California Press (2001).
Google Scholar
9. Jong-Wook L. Global health improvement and WHO: shaping the future. Lancet. (2003) 362:2083–8. doi: 10.1016/S0140-6736(03)15107-0
10. Ngonghala CN, Pluciński MM, Murray MB, Farmer PE, Barrett CB, Keenan DC, et al. Poverty, disease, and the ecology of complex systems. PLoS Biol. (2014) 12:e1001827. doi: 10.1371/journal.pbio.1001827
11. Bonds MH, Keenan DC, Rohani P, Sachs JD. Poverty trap formed by the ecology of infectious diseases. Proc R Soc B Biol Sci. (2010) 277:1185–92. doi: 10.1098/rspb.2009.1778
12. Ngonghala CN, De Leo GA, Pascual MM, Keenan DC, Dobson AP, Bonds MH. General ecological models for human subsistence, health and poverty. Nat Ecol Evol. (2017) 1:1153–9. doi: 10.1038/s41559-017-0221-8
13. Shen J, Shum WY, Cheong TS, Wang L. COVID-19 and regional income inequality in China. Front Public Health. (2021) 9:687152. doi: 10.3389/fpubh.2021.687152
14. Chakraborty S, Papageorgiou C, Sebastián FP. Diseases, infection dynamics, and development. J Monet Econ. (2010) 57:859–72. doi: 10.1016/j.jmoneco.2010.08.004
15. Nokes C, Grantham-Mcgregor SM, Sawyer AW, Cooper ES, Bundy DA. Parasitic helminth infection and cognitive function in school children. Proc R Soc B Biol Sci. (1992) 247:77–81. doi: 10.1098/rspb.1992.0011
16. Holding PA, Snow RW. Impact of plasmodium falciparum malaria on performance and learning: review of the evidence. Am J Trop Med Hyg . (2001) 64(1–2 Suppl.):68–75. doi: 10.4269/ajtmh.2001.64.68
17. Kalemli-Ozcan S, Ryder HE, Weil DN. Mortality decline, human capital investment, and economic growth. J Dev Econ. (2000) 62:1–23. doi: 10.1016/S0304-3878(00)00073-0
18. Glewwe P. Schools and skills in developing countries: education policies and socioeconomic outcomes. J Econ Lit. (2002) 40:436–82. doi: 10.1257/jel.40.2.436
19. Fortson JG. Mortality risk and human capital investment: the impact of HIV/AIDS in Sub-Saharan Africa. Rev Econ Stat. (2011) 93:1–15. doi: 10.1162/REST_a_00067
20. Goenka A, Liu L. Infectious diseases, human capital and economic growth. Econ Theory. (2019) 7:1–47. doi: 10.1007/s00199-019-01214-7
21. Tao R, Su C, Yaqoob T, Hammal M. Do financial and non-financial stocks hedge against lockdown in Covid-19? An event study analysis. Econ Res . (2021). doi: 10.1080/1331677X.2021.1948881
22. Pardhan S, Drydakis N. Associating the change in new COVID-19 cases to GDP per capita in 38 European Countries in the first wave of the pandemic. Front Public Health. (2021) 8:582140. doi: 10.3389/fpubh.2020.582140
23. Yang J, Zeng Z, Wang K, Wong SS, Liang W, Zanin M, et al. Modified SEIR and AI prediction of the epidemics trend of COVID-19 in China under public health interventions. J Thorac Dis. (2020) 12:165–74. doi: 10.21037/jtd.2020.02.64
24. Goenka A, Liu L. Infectious diseases and endogenous fluctuations. Econ Theory. (2012) 50:125–49. doi: 10.1007/s00199-010-0553-y
25. Bogoch II, Creatore MI, Cetron MS, Brownstein JS, Pesik N, Miniota J, et al. Assessment of the potential for international dissemination of Ebola virus via commercial air travel during the 2014 west African outbreak. Lancet. (2014) 385:29–35. doi: 10.1016/S0140-6736(14)61828-6
26. Qiu Y, Chen X, Shi W. Impacts of social and economic factors on the transmission of coronavirus disease 2019 (COVID-19) in China. J Popul Econ. (2020) 33:1127–72. doi: 10.1007/s00148-020-00778-2
27. Almond D. Is the 1918 influenza pandemic over? long-term effects of in utero influenza exposure in the post-1940 US population. J Polit Econ. (2006) 114:672–712. doi: 10.1086/507154
28. Kermack WO, McKendrick AG. A contribution to the mathematical theory of epidemics. Proc R Soc Lond A. (1927) 115:700–21. doi: 10.1098/rspa.1927.0118
29. Anderson RM, May RM. Infectious Diseases of Humans . Oxford: Oxford University Press (1991).
30. Gersovitz M, Hammer JS. The economical control of infectious diseases. Econ J. (2004) 114:1–27. doi: 10.1046/j.0013-0133.2003.0174.x
31. Smith DL, McKenzie FE. Statics and dynamics of malaria infection in Anopheles mosquitoes. Malar J. (2004) 3:13. doi: 10.1186/1475-2875-3-13
32. Smith DL, Dushoff J, Snow RW, Hay SI. The entomological inoculation rate and Plasmodium falciparum infection in African children. Nature. (2005) 438:492–5. doi: 10.1038/nature04024
33. Schumpeter J. The Theory of Economic Development . Cambridge: Harvard Economic Studies (1911).
34. Acemoglu D, Restrepo P. The race between man and machine: implications of technology for growth, factor shares, and employment. Am Econ Rev. (2018) 108:1488–542. doi: 10.1257/aer.20160696
35. Zhou Y, Xu R, Hu D, Yue Y, Li Q, Xia J. Effects of human mobility restrictions on the spread of COVID-19 in Shenzhen, China: a modelling study using mobile phone data. Lancet. (2020) 2:e417–e424. doi: 10.1016/S2589-7500(20)30165-5
36. Augier L, Yaly A. Economic growth and disease in the OLG model: The HIV/AIDS case. Econ. Model. (2013) 33:471–481. doi: 10.1016/j.econmod.2013.04.033
Keywords: COVID-19 pandemic, economic growth, health capital, public health, interdisciplinary analysis
Citation: Xiang L, Tang M, Yin Z, Zheng M and Lu S (2021) The COVID-19 Pandemic and Economic Growth: Theory and Simulation. Front. Public Health 9:741525. doi: 10.3389/fpubh.2021.741525
Received: 14 July 2021; Accepted: 20 August 2021; Published: 17 September 2021.
Reviewed by:
Copyright © 2021 Xiang, Tang, Yin, Zheng and Lu. This is an open-access article distributed under the terms of the Creative Commons Attribution License (CC BY) . The use, distribution or reproduction in other forums is permitted, provided the original author(s) and the copyright owner(s) are credited and that the original publication in this journal is cited, in accordance with accepted academic practice. No use, distribution or reproduction is permitted which does not comply with these terms.
*Correspondence: Zhichao Yin, yzcyzc210@126.com ; Mengmeng Zheng, 20044040@sdufe.edu.cn
Disclaimer: All claims expressed in this article are solely those of the authors and do not necessarily represent those of their affiliated organizations, or those of the publisher, the editors and the reviewers. Any product that may be evaluated in this article or claim that may be made by its manufacturer is not guaranteed or endorsed by the publisher.

The Economic Impact of COVID-19 around the World
Working Paper 2022-030A by Fernando M. Martin, Juan M. Sánchez, and Olivia Wilkinson
For over two years, the world has been battling the health and economic consequences of the COVID‐19 pandemic. This paper provides an account of the worldwide economic impact of the COVID‐19 shock, measured by GDP growth, employment, government spending, monetary policy, and trade. We find that the COVID‐19 shock severely impacted output growth and employment in 2020, particularly in middle‐income countries. The government response, mainly consisting of increased expenditure, implied a rise in debt levels. Advanced countries, having easier access to credit markets, experienced the highest increase in indebtedness. All regions also relied on monetary policy to support the fiscal expansion. The specific circumstances surrounding the COVID‐19 shock implied that the expansionary fiscal and monetary policies did not put upward pressure on prices until 2021. We also find that the adverse effects of the COVID‐19 shock on output and prices have been significant and persistent, especially in emerging and developing countries.
Read Full Text
https://doi.org/10.20955/wp.2022.030
- Subscribe to Our Newsletter
Related Links
- Economist Pages
- JEL Classification System
- Fed In Print
SUBSCRIBE TO THE RESEARCH DIVISION NEWSLETTER
Research division.
- Legal and Privacy
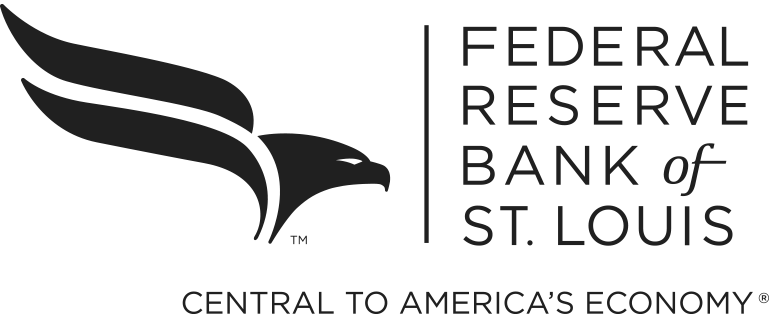
One Federal Reserve Bank Plaza St. Louis, MO 63102
Information for Visitors

Chapter 1. The economic impacts of the COVID-19 crisis
The COVID-19 pandemic sent shock waves through the world economy and triggered the largest global economic crisis in more than a century. The crisis led to a dramatic increase in inequality within and across countries. Preliminary evidence suggests that the recovery from the crisis will be as uneven as its initial economic impacts, with emerging economies and economically disadvantaged groups needing much more time to recover pandemic-induced losses of income and livelihoods . 1
In contrast to many earlier crises, the onset of the pandemic was met with a large, decisive economic policy response that was generally successful in mitigating its worst human costs in the short run. However, the emergency response also created new risks—such as dramatically increased levels of private and public debt in the world economy—that may threaten an equitable recovery from the crisis if they are not addressed decisively.
Worsening inequality within and across countries
The economic impacts of the pandemic were especially severe in emerging economies where income losses caused by the pandemic revealed and worsened some preexisting economic fragilities. As the pandemic unfolded in 2020, it became clear that many households and firms were ill-prepared to withstand an income shock of that scale and duration. Studies based on precrisis data suggest, for example, that more than 50 percent of households in emerging and advanced economies were not able to sustain basic consumption for more than three months in the event of income losses . 2 Similarly, the average business could cover fewer than 55 days of expenses with cash reserves . 3 Many households and firms in emerging economies were already burdened with unsustainable debt levels prior to the crisis and struggled to service this debt once the pandemic and associated public health measures led to a sharp decline in income and business revenue.
The crisis had a dramatic impact on global poverty and inequality. Global poverty increased for the first time in a generation, and disproportionate income losses among disadvantaged populations led to a dramatic rise in inequality within and across countries. According to survey data, in 2020 temporary unemployment was higher in 70 percent of all countries for workers who had completed only a primary education. 4 Income losses were also larger among youth, women, the self-employed, and casual workers with lower levels of formal education . 5 Women, in particular, were affected by income and employment losses because they were likelier to be employed in sectors more affected by lockdown and social distancing measures . 6
Similar patterns emerge among businesses. Smaller firms, informal businesses, and enterprises with limited access to formal credit were hit more severely by income losses stemming from the pandemic. Larger firms entered the crisis with the ability to cover expenses for up to 65 days, compared with 59 days for medium-size firms and 53 and 50 days for small and microenterprises, respectively. Moreover, micro-, small, and medium enterprises are overrepresented in the sectors most severely affected by the crisis, such as accommodation and food services, retail, and personal services.

The short-term government responses to the crisis
The short-term government responses to the pandemic were extraordinarily swift and encompassing. Governments embraced many policy tools that were either entirely unprecedented or had never been used on this scale in emerging economies. Examples are large direct income support measures, debt moratoria, and asset purchase programs by central banks. These programs varied widely in size and scope (figure 1.1), in part because many low-income countries were struggling to mobilize resources given limited access to credit markets and high precrisis levels of government debt. As a result, the size of the fiscal response to the crisis as a share of the gross domestic product (GDP) was almost uniformly large in high-income countries and uniformly small or nonexistent in low-income countries. In middle-income countries, the fiscal response varied substantially, reflecting marked differences in the ability and willingness of governments to spend on support programs.
Figure 1.1 Fiscal response to the COVID-19 crisis, selected countries, by income group
Similarly, the combination of policies chosen to confront the short-term impacts differed significantly across countries, depending on the availability of resources and the specific nature of risks the countries faced (figure 1.2). In addition to direct income support programs, governments and central banks made unprecedented use of policies intended to provide temporary debt relief, including debt moratoria for households and businesses. Although these programs mitigated the short-term liquidity problems faced by households and businesses, they also had the unintended consequence of obscuring the true financial condition of borrowers, thereby creating a new problem: lack of transparency about the true extent of credit risk in the economy.
Figure 1.2 Fiscal, monetary, and financial sector policy responses to the COVID-19 crisis, by country income group
The large crisis response, while necessary and effective in mitigating the worst impacts of the crisis, led to a global increase in government debt that gave rise to renewed concerns about debt sustainability and added to the widening disparity between emerging and advanced economies. In 2020, 51 countries—including 44 emerging economies—experienced a downgrade in their government debt risk rating (that is, the assessment of a country’s creditworthiness) . 7
Emerging threats to an equitable recovery
Although households and businesses have been most directly affected by income losses stemming from the pandemic, the resulting financial risks have repercussions for the wider economy through mutually reinforcing channels that connect the financial health of households, firms, financial institutions, and governments (figure 1.3). Because of this interconnection, elevated financial risk in one sector can spill over and destabilize the economy as a whole. For example, if households and firms are under financial stress, the financial sector faces a higher risk of loan defaults and is less able to provide credit. Similarly, if the financial position of the public sector deteriorates (for example, as a result of higher government debt and lower tax revenue), the ability of the public sector to support the rest of the economy is weakened.
Figure 1.3 Conceptual framework: Interconnected balance sheet risks
This relationship is, however, not predetermined. Well-designed fiscal, monetary, and financial sector policies can counteract and reduce these intertwined risks and can help transform the links between sectors of the economy from a vicious doom loop into a virtuous cycle.
One example of policies that can make a critical difference are those targeting the links between the financial health of households, businesses, and the financial sector. In response to the first lockdowns and mobility restrictions, for example, many governments supported households and businesses using cash transfers and financial policy tools such as debt moratoria. These programs provided much-needed support to households and small businesses and helped avert a wave of insolvencies that could have threatened the stability of the financial sector.
Similarly, governments, central banks, and regulators used various policy tools to assist financial institutions and prevent risks from spilling over from the financial sector to other parts of the economy. Central banks lowered interest rates and eased liquidity conditions, making it easier for commercial banks and nonbank financial institutions such as microfinance lenders to refinance themselves, thereby allowing them to continue to supply credit to households and businesses.
The crisis response will also need to include policies that address the risks arising from high levels of government debt to ensure that governments preserve their ability to effectively support the recovery. This is an important policy priority because high levels of government debt reduce the government’s ability to invest in social safety nets that can counteract the impact of the crisis on poverty and inequality and provide support to households and firms in the event of setbacks during the recovery.
➜ Read the Full Chapter (.pdf) : English ➜ Read the Full Report (.pdf) : English ➜ WDR 2022 Home
CHAPTER SUMMARIES
➜ Chapter 1 ➜ Chapter 2 ➜ Chapter 3 ➜ Chapter 4 ➜ Chapter 5 ➜ Chapter 6
RELATED LINKS
➜ Blog Posts ➜ Press and Media ➜ Consultations ➜ WDR 2022 Core Team
WDR 2022 Video | Michael Schlein: President & CEO of Accion
Wdr 2022 video | chatib basri: former minister of finance of indonesia.
This site uses cookies to optimize functionality and give you the best possible experience. If you continue to navigate this website beyond this page, cookies will be placed on your browser. To learn more about cookies, click here .
COVID-19’s Economic Impact around the World
Key takeaways.
- Although the COVID-19 pandemic affected all parts of the world in 2020, low-, middle- and high-income nations were hit in different ways.
- In low-income countries, average excess mortality reached 34%, followed by 14% in middle-income countries and 10% in high-income ones.
- However, middle-income nations experienced the largest hit to their gross domestic product (GDP) growth, followed by high-income nations.
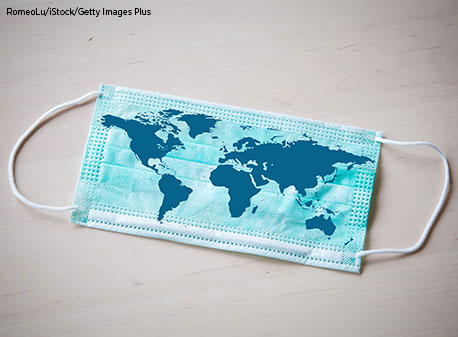
Since the COVID-19 pandemic began in March 2020, the world economy has been affected in many ways. Poorer countries have suffered the most, but, despite their greater resources, wealthier countries have faced their own challenges. This article looks at the impact of COVID-19 in different areas of the world.
First, I put 171 nations into three groups according to per capita income: low, middle and high income. Second, I examined health statistics to show how hard-hit by the virus these nations were. Then, by comparing economic forecasts the International Monetary Fund (IMF) made in October 2019 (pre-pandemic) for 2020 with their actual values, I obtained estimates for the pandemic’s impact on growth and key economic policy variables.
Low- and high-income groups each compose 25% of the world’s countries, and the middle-income group makes up 50%. Average income per capita in 2019 was more than five times larger in the middle-income group than in the low-income group. In the high-income countries, it was almost 20 times larger.
Health Outcomes and Policies
The first table shows that COVID-19 had a significant impact on all three groups. Average excess mortality, which indicates how much larger the number of deaths was relative to previous years, was more than 34% in low-income countries, almost 14% in middle-income countries and about 10% in high-income countries. And even though poorer countries were more affected by deaths, their COVID-19 testing was much more limited given their smaller resources.
Since the beginning of the pandemic, high-income countries did more than one test per person, while low-income countries did only one test per 27 people (or 0.037 per person). Given the significant differences in testing, it is not surprising that reported cases were much higher in wealthier countries. Finally, note that there were significant differences in the progress of vaccination. As of June 2021, nearly 20% of the population in the wealthiest countries was fully vaccinated compared to about 2% in the poorest countries.
Impact on GDP Growth
COVID-19-related lockdowns were very common during 2020-21, directly impacting economic activity. The figure below shows the impact on GDP. To isolate the impact of COVID-19 from previous trends, I plotted the difference between the actual GDP growth in 2020 and the IMF forecast made in October 2019.
The immediate consequence of closing many sectors of the economy was a significant decline in GDP growth, which was as large as 8.7 percentage points for the median middle-income countries. Wealthier countries suffered a bit less, with a median of 6.4 percentage points, mainly because they began to recover before the end of 2020. The impact of COVID-19 was smaller in poorer countries because many did not have the resources to implement strict lockdowns. However, even in this group of countries, median GDP growth was 5.2 percentage points lower than expected.
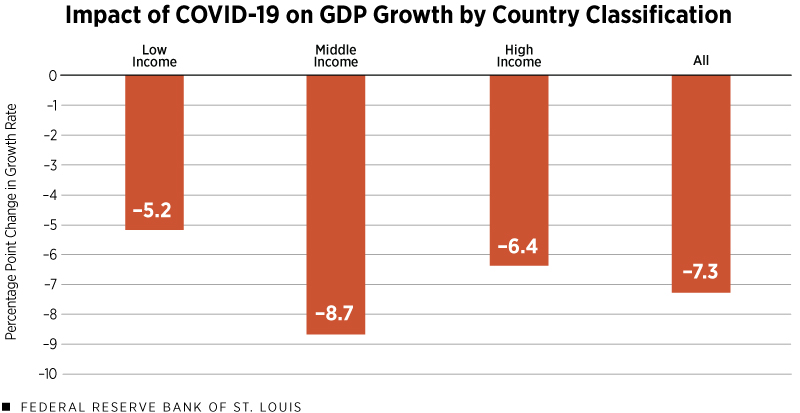
SOURCES: IMF World Economic Outlook Reports (April 2021 and October 2019), Penn World Table (version 10.0) and author’s calculations.
NOTE: The COVID-19 impact is the difference between the actual gross domestic product growth rate in 2020 and the IMF forecast for it made in October 2019.
Economic Policies
Differences in GDP performance are not only related to lockdowns but also to economic policy responses. The second table contains information about six policy variables.
In particular, the first three rows present the fiscal response to the pandemic computed as the difference between the actual value in 2020 and the IMF forecast made before the pandemic in October 2019 relative to GDP. Revenue relative to GDP declined slightly in all regions, but mostly in middle-income countries, reaching more than 1 percentage point of GDP.
Expenditures relative to GDP, however, increased in middle- and high-income countries while remaining stable in low-income countries. These expenditures increased by nearly 7 percentage points of GDP in high-income countries. The more significant fiscal deficit relative to GDP implied a larger increase in net government borrowing, which reached 7 percentage points of GDP in the median high-income countries.
Finally, COVID-19 also had a clear impact on the evolution of monetary aggregates such as cash and deposits. In the table, to isolate the impact of COVID-19 from previous trends, I present the growth rate of M1 and M2 M1 generally includes physical currency, demand deposits, traveler’s checks and other checkable deposits. M2 generally includes M1 plus savings deposits, money market securities, mutual funds and other time deposits. Note that the above definitions can differ slightly by country. net of the yearly growth rates of these variables between 2017 and 2019. The pandemic implied an increase in the growth rate of monetary aggregates across countries in all income groups, but more significantly in wealthier countries.
For instance, the growth rate in M1 was over 10 percentage points larger than in the previous two years in the median high-income countries. Without a change in money demand, such an acceleration in the quantity of money would have implied increasing inflation.
However, the last row of the table shows that inflation remained stable in 2020. In fact, for middle- and high-income countries, inflation in 2020 was lower than the IMF forecast made in October 2019.
Conclusions
COVID-19 impacted health outcomes in all regions of the world. Wealthier countries responded with more testing and quicker vaccination rates. Comparing actual outcomes with pre-pandemic forecasts, I found a significant impact of the pandemic on GDP growth, which is more prominent in middle-income countries.
I conjecture that the impact on GDP growth was less significant in the poorest countries because of less restrictive lockdowns and in the wealthiest countries because of more aggressive economic policies.
- M1 generally includes physical currency, demand deposits, traveler’s checks and other checkable deposits. M2 generally includes M1 plus savings deposits, money market securities, mutual funds and other time deposits. Note that the above definitions can differ slightly by country.
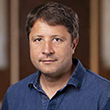
Juan M. Sánchez is an economist and senior economic policy advisor at the Federal Reserve Bank of St. Louis. He has conducted research on several topics in macroeconomics involving financial decisions by firms, households and countries. He has been at the St. Louis Fed since 2010. View more about the author and his research.
Related Topics
Views expressed in Regional Economist are not necessarily those of the St. Louis Fed or Federal Reserve System.
For the latest insights from our economists and other St. Louis Fed experts, visit On the Economy and subscribe .
Media questions
The Economic Impacts of COVID-19: Evidence from a New Public Database Built Using Private Sector Data
We build a publicly available database that tracks economic activity in the U.S. at a granular level in real time using anonymized data from private companies. We report weekly statistics on consumer spending, business revenues, job postings, and employment rates disaggregated by county, sector, and income group. Using the publicly available data, we show how the COVID- 19 pandemic affected the economy by analyzing heterogeneity in its impacts across subgroups. High-income individuals reduced spending sharply in March 2020, particularly in sectors that require in-person interaction. This reduction in spending greatly reduced the revenues of small businesses in affluent, dense areas. Those businesses laid off many of their employees, leading to widespread job losses, especially among low-wage workers in such areas. High-wage workers experienced a “V-shaped” recession that lasted a few weeks, whereas low-wage workers experienced much larger, more persistent job losses. Even though consumer spending and job postings had recovered fully by December 2021, employment rates in low-wage jobs remained lower in areas that were initially hard hit, indicating that the job losses due to the demand shock led to a persistent reduction in labor supply. Building on this diagnostic analysis, we evaluate the impacts of fiscal stimulus policies designed to stem the downward spiral in economic activity. Cash stimulus payments led to sharp increases in spending early in the pandemic, but much smaller responses later in the pandemic, especially for high-income households. Real-time estimates of marginal propensities to consume provided better forecasts of the impacts of subsequent rounds of stimulus payments than historical estimates. Overall, our findings suggest that fiscal policies can stem secondary declines in consumer spending and job losses, but cannot restore full employment when the initial shock to consumer spending arises from health concerns. More broadly, our analysis demonstrates how public statistics constructed from private sector data can support many research and real-time policy analyses, providing a new tool for empirical macroeconomics.
We thank the corporate partners who provided the underlying data used to construct the public database built in this paper: Affinity Solutions (especially Atul Chadha and Arun Rajagopal), Lightcast (Anton Libsch and Bledi Taska), CoinOut (Jeff Witten), Earnin (Arun Natesan and Ram Palaniappan), Homebase (Ray Sandza and Andrew Vogeley), Intuit (Christina Foo and Krithika Swaminathan), Kronos (David Gilbertson), Paychex (Mike Nichols and Shadi Sifain), Womply (Derek Doel and Ryan Thorpe), and Zearn (Billy McRae and Shalinee Sharma). We are very grateful to Nathaniel Hendren, who collaborated with us to launch the initial version of the database and helped conduct preliminary analyses for the first draft of this paper in Spring 2020. We are also grateful to Ryan Rippel of the Gates Foundation for his support in launching this project and to Gregory Bruich for early conversations that helped spark this work. We thank David Autor, Gabriel Chodorow-Reich, Haley O'Donnell, Emmanuel Farhi, Jason Furman, Steven Hamilton, Erik Hurst, Xavier Jaravel, Lawrence Katz, Fabian Lange, Emmanuel Saez, Ludwig Straub, Danny Yagan, and numerous seminar participants for helpful comments. The work was funded by the Chan-Zuckerberg Initiative, Bill & Melinda Gates Foundation, Overdeck Family Foundation, and Andrew and Melora Balson. The project was approved under Harvard University IRB 20-0586. The Opportunity Insights Economic Tracker Team as of April 2023 has consisted of Hamidah Alatas, Camille Baker, Harvey Barnhard, Matt Bell, Gregory Bruich, Tina Chelidze, Lucas Chu, Westley Cineus, Sebi Devlin-Foltz, Michael Droste, Dhruv Gaur, Federico Gonzalez, Rayshauna Gray, Abigail Hiller, Matthew Jacob, Tyler Jacobson, Margaret Kallus, Fiona Kastel, Laura Kincaide, Cailtin Kupsc, Sarah LaBauve, Lucía Lamas, Maddie Marino, Kai Matheson, Jared Miller, Christian Mott, Kate Musen, Danny Onorato, Sarah Oppenheimer, Trina Ott, Lynn Overmann, Max Pienkny, Jeremiah Prince, Sebastian Puerta, Daniel Reuter, Peter Ruhm, Tom Rutter, Emanuel Schertz, Shannon Felton Spence, Krista Stapleford, Kamelia Stavreva, Ceci Steyn, James Stratton, Clare Suter, Elizabeth Thach, Nicolaj Thor, Amanda Wahlers, Kristen Watkins, Alanna Williams, David Williams, Chase Williamson, Shady Yassin, Ruby Zhang, and Austin Zheng. The views expressed herein are those of the authors and do not necessarily reflect the views of the National Bureau of Economic Research.
MARC RIS BibTeΧ
Download Citation Data
- Economic Tracker and Dowloadable Data
- July 26, 2020
- November 19, 2020
- November 2, 2022
Non-Technical Summaries
- Panel on Social Security and COVID-19 at NBER Summer Institute Author(s): Alexander W. Bartik Marianne Bertrand Feng Lin Jesse Rothstein Matt Unrath Raj Chetty John N. Friedman Michael Stepner The Opportunity Insights Team Olivier Coibion Yuriy Gorodnichenko Michael Weber Austan Goolsbee Chad Syverson Sumedha Gupta Thuy D. Nguyen Felipe Lozano Rojas Shyam Raman Byungkyu Lee Ana I. Bento Kosali I. Simon Coady Wing Thuy D. Nguyen Sumedha Gupta Martin Andersen Ana I. Bento Kosali I. Simon Coady Wing Sumedha Gupta Laura Montenovo Thuy D. Nguyen Felipe Lozano Rojas Ian M. Schmutte Kosali I. Simon Bruce A. Weinberg Coady Wing Eliza Forsythe Lisa B. Kahn Fabian Lange David G. Wiczer Peter Zhixian Lin Christopher M. Meissner The 2020 NBER Summer Institute's Economics of Social Security meeting featured a panel discussion on the implications of the COVID-19...
Published Versions
Raj Chetty & John N Friedman & Michael Stepner & Hamidah Alatas & Camille Baker & Harvey Barnhard & Matt Bell & Gregory Bruich & Tina Chelidze & Lucas Chu & Westley Cineus & Sebi Devlin-Foltz & Michael Droste & Dhruv Gaur & Federico Gonzalez & Rayshauna Gray & Abigail Hiller & Matthew Jacob & Tyler Jacobson & Margaret Kallus & Fiona Kastel & Laura Kincaide & Caitlin Kupsc & Sarah LaBauve & Lucía Lamas & Maddie Marino & Kai Matheson & Jared Miller & Christian Mott & Kate Musen & Danny Onorato & Sarah Oppenheimer & Trina Ott & Lynn Overmann & Max Pienkny & Jeremiah Prince & Sebastian Puerta & Daniel Reuter & Peter Ruhm & Tom Rutter & Emanuel Schertz & Shannon Felton Spence & Krista Stapleford & Kamelia Stavreva & Ceci Steyn & James Stratton & Clare Suter & Elizabeth Thach & Nicolaj Thor & Amanda Wahlers & Kristen Watkins & Alanna Williams & David Williams & Chase Williamson & Shady Yassin & Ruby Zhang & Austin Zheng, 2024. " The Economic Impacts of COVID-19: Evidence from a New Public Database Built Using Private Sector Data, " The Quarterly Journal of Economics, vol 139(2), pages 829-889.
Working Groups
Mentioned in the news, more from nber.
In addition to working papers , the NBER disseminates affiliates’ latest findings through a range of free periodicals — the NBER Reporter , the NBER Digest , the Bulletin on Retirement and Disability , the Bulletin on Health , and the Bulletin on Entrepreneurship — as well as online conference reports , video lectures , and interviews .
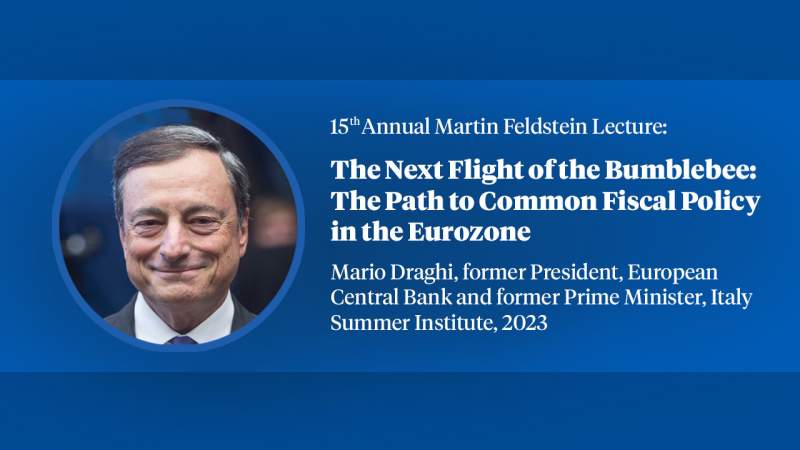
Social and economic impact of COVID-19
Download the full working paper
Subscribe to Global Connection
Eduardo levy yeyati and eduardo levy yeyati former nonresident senior fellow - global economy and development @eduardoyeyati federico filippini federico filippini visiting professor - universidad torcuato di tella @efefilippini.
June 8, 2021
Introduction
The impact of the pandemic on world GDP growth is massive. The COVID-19 global recession is the deepest since the end of World War II (Figure 1). The global economy contracted by 3.5 percent in 2020 according to the April 2021 World Economic Outlook Report published by the IMF, a 7 percent loss relative to the 3.4 percent growth forecast back in October 2019. While virtually every country covered by the IMF posted negative growth in 2020 (IMF 2020b), the downturn was more pronounced in the poorest parts of the world (Noy et al. 2020) (Figure 2).

The impact of the shock is likely to be long-lasting. While the global economy is expected to recover this year, the level of GDP at the end of 2021 in both advanced and emerging market and developing economies (EMDE) is projected to remain below the pre-virus baseline (Figure 3). As with the immediate impact, the magnitude of the medium-term cost also varies significantly across countries, with EMDE suffering the greatest loss. The IMF (2021) projects that in 2024 the World GDP will be 3 percent (6 percent for low-income countries (LICs)) below the no-COVID scenario. Along the same lines, Djiofack et al. (2020) estimate that African GDP would be permanently 1 percent to 4 percent lower than in the pre-COVID outlook, depending on the duration of the crisis.

The pandemic triggered a health and fiscal response unprecedented in terms of speed and magnitude. At a global scale, the fiscal support reached nearly $16 trillion (around 15 percent of global GDP) in 2020. However, the capacity of countries to implement such measures varied significantly. In this note, we identify three important preexisting conditions that amplified the impact of the shock:
- Fiscal space: The capacity to support household and firms largely depends on access to international financial markets,
- State capacity: Fast and efficient implementation of policies to support household and firms requires a substantial state capacity and well-developed tax and transfer infrastructure; and
- Labor market structure: A large share of informal workers facing significant frictions to adopt remote working, and high levels of poverty and inequality, deepen the deleterious impact of the crisis.
Additionally, the speed and the strength of the recovery will be crucially dependent on the capacity of the governments to acquire and roll out the COVID-19 vaccines.
This paper presents a succinct summary of the existing economic literature on the economic and fiscal impact of the pandemic, and a preliminary estimate of the associated economic cost. It documents the incidence of initial conditions (with a particular focus on the role of the labor market channel) on the transmission of the shock and the speed and extent of the expected recovery, summarizes how countries attempted to attenuate the economic consequences and the international financial institutions assisted countries, reports preliminary accounts of medium-term COVID-related losses, and concludes with some forward-looking considerations based on the lessons learned in 2020.
Related Content
Homi Kharas, Meagan Dooley
June 2, 2021
Wolfgang Fengler, Homi Kharas
May 20, 2021
Global Economy and Development
Sam Boocker, Alexander Conner, David Wessel
May 29, 2024
Raymond Gilpin, Daouda Sembene, Daniel Cash, Jacob Assa, Aloysius Uche Ordu
Amit Jain, Landry Signé
- Research article
- Open access
- Published: 08 April 2021
Health, psychosocial, and economic impacts of the COVID-19 pandemic on people with chronic conditions in India: a mixed methods study
- Kavita Singh ORCID: orcid.org/0000-0003-4330-666X 1 , 2 ,
- Dimple Kondal 1 ,
- Sailesh Mohan 1 , 2 , 3 ,
- Suganthi Jaganathan 2 ,
- Mohan Deepa 4 ,
- Nikhil Srinivasapura Venkateshmurthy 1 , 2 ,
- Prashant Jarhyan 1 ,
- Ranjit Mohan Anjana 4 ,
- K. M. Venkat Narayan 5 ,
- Viswanathan Mohan 4 ,
- Nikhil Tandon 6 ,
- Mohammed K. Ali 5 ,
- Dorairaj Prabhakaran 1 , 2 , 7 &
- Karen Eggleston 8
BMC Public Health volume 21 , Article number: 685 ( 2021 ) Cite this article
36k Accesses
83 Citations
26 Altmetric
Metrics details
People with chronic conditions are disproportionately prone to be affected by the COVID-19 pandemic but there are limited data documenting this. We aimed to assess the health, psychosocial and economic impacts of the COVID-19 pandemic on people with chronic conditions in India.
Between July 29, to September 12, 2020, we telephonically surveyed adults ( n = 2335) with chronic conditions across four sites in India. Data on participants’ demographic, socio-economic status, comorbidities, access to health care, treatment satisfaction, self-care behaviors, employment, and income were collected using pre-tested questionnaires. We performed multivariable logistic regression analysis to examine the factors associated with difficulty in accessing medicines and worsening of diabetes or hypertension symptoms. Further, a diverse sample of 40 participants completed qualitative interviews that focused on eliciting patient’s experiences during the COVID-19 lockdowns and data analyzed using thematic analysis.
One thousand seven hundred thirty-four individuals completed the survey (response rate = 74%). The mean (SD) age of respondents was 57.8 years (11.3) and 50% were men. During the COVID-19 lockdowns in India, 83% of participants reported difficulty in accessing healthcare, 17% faced difficulties in accessing medicines, 59% reported loss of income, 38% lost jobs, and 28% reduced fruit and vegetable consumption. In the final-adjusted regression model, rural residence (OR, 95%CI: 4.01,2.90–5.53), having diabetes (2.42, 1.81–3.25) and hypertension (1.70,1.27–2.27), and loss of income (2.30,1.62–3.26) were significantly associated with difficulty in accessing medicines. Further, difficulties in accessing medicines (3.67,2.52–5.35), and job loss (1.90,1.25–2.89) were associated with worsening of diabetes or hypertension symptoms. Qualitative data suggest most participants experienced psychosocial distress due to loss of job or income and had difficulties in accessing in-patient services.
People with chronic conditions, particularly among poor, rural, and marginalized populations, have experienced difficulties in accessing healthcare and been severely affected both socially and financially by the COVID-19 pandemic.
Peer Review reports
As the global burden of novel coronavirus disease 2019 (COVID-19) continues to increase, particularly in low- and middle- income countries such as India, it imposes huge costs on individuals, communities, health systems, and economies [ 1 ]. Although some countries and regions are seeing improvements in hospitalization and death rates, COVID-19 remains a major concern for vulnerable and underserved populations globally [ 2 , 3 ]. People with chronic conditions are disproportionately prone to COVID-19–related hospitalizations, intensive care admissions, and mortality, compared to those without chronic conditions [ 4 , 5 , 6 , 7 ]. Moreover, they may be particularly susceptible to adverse health impacts from delayed or foregone care during the pandemic. The 2020 World Health Organization (WHO) report on the impact of COVID-19 on noncommunicable diseases (NCDs) in 163 countries highlighted that nearly half of the countries report that patients experienced partial or complete disruption of services for hypertension, diabetes, and related complications during the pandemic. One-third reported disrupted services for cardiovascular emergencies. Further, most countries reassigned the health staff towards COVID-19 support, which affected routine care for NCDs [ 8 ]. Several reports indicated change in routine care to virtual consultations and worsened mental health problems during the pandemic. Diabetes, chronic obstructive pulmonary disease, and hypertension were the most impacted conditions due to significant reduction in access to care [ 9 , 10 , 11 , 12 , 13 , 14 ]. Given the syndemic interaction ─interrelationship between COVID-19 and various socio-ecological and biological factors contributing to preexisting NCD epidemics─ people with chronic conditions are more vulnerable to COVID-19 infection [ 15 , 16 ].
As of March 11, 2021 more than 11 million people in India had been infected with COVID-19, and about 158,000 had died [ 6 ]. The spread of COVID-19 in India is of great concern due to the country’s large and densely populated areas with widespread poverty and high migration rates, coupled with a high prevalence of chronic conditions [ 17 , 18 , 19 ] that are generally poorly controlled [ 20 , 21 , 22 ]. Further, the progression of COVID-19 from urban to rural areas, the strict lockdown measures, and the associated economic shocks are likely to impede efforts to address other health scourges in India such as diabetes, hypertension, and cardiovascular diseases. On March 24, 2020, the Indian government ordered a nationwide lockdown, which was extended until June in four phases, and later further extended to specific containment zones. During the lockdowns, many health facilities were functioning sub-optimally or were converted to COVID facilities and provided only essential and emergency services.
Measures to address coronavirus spread including lockdowns may have serious economic consequences and unintended effect of exacerbating rather than mitigating health disparities [ 8 , 9 , 23 , 24 ]. However, to date, few data document the impact of the COVID-19 pandemic on disparities in chronic disease management in India. Given the unprecedented and rapidly evolving COVID-19 situation in India, we aimed to assess the health, psychosocial and economic impacts of COVID-19 pandemic on people with chronic conditions in India.
Study setting and participants
We conducted a cross-sectional study using sequential mixed methods design, comprising a quantitative survey and qualitative interviews to describe the impact of COVID-19 on the health, psychosocial, and economic well-being of people with chronic conditions in India. Adults with one or more chronic conditions (hypertension, diabetes mellitus, cardiovascular disease, or chronic kidney disease), from the two large existing cohorts (the Centre for Cardio-metabolic Risk Reduction in South Asia, CARRS [ 25 ]; and a comprehensive diabetes and hypertension prevention and management program in India-UDAY [ 26 ]) were invited to participate in this study. The CARRS and UDAY study protocols and main study results have been published previously [ 14 , 15 ]. Briefly, CARRS enrolled 12,271 adults aged ≥20 years that were sampled to be representative of Delhi and Chennai in 2010–2011 and has followed them annually since. The UDAY study enrolled 12,243 adults in 2014–15 aged ≥30 years from rural and urban communities in Sonipat (Haryana), and Visakhapatnam (Vizag), Andhra Pradesh, India. For this study, we used stratified random sampling of participants with chronic conditions by age and sex. We randomly selected and approached around 600 participants at each of the four sites (Delhi, Chennai Haryana and Vizag) in India. Furthermore, a diverse sample of 40 participants stratified by age, sex, comorbidities, and urban/rural sites were purposively selected for the qualitative interview. This study was approved by the Institutional Ethics Committees of the Centre for Chronic Disease Control, New Delhi, India, and the Madras Diabetes Research Foundation, Chennai, India. All participants provided verbal consent to this study over the phone.
Data collection
Between July 29 and September 12, 2020, we collected data on participants’ demographic, socio-economic status, comorbidities, access to healthcare, difficulty in accessing medicines due to financial and non-financial (COVID-19 related) reasons, and treatment satisfaction. Quantitative survey questionnaire and qualitative study interview guide were developed by the authors for this mixed-methods study ( Supplementary file 1 ). Participants were asked if their diabetes or hypertension symptoms worsened after lockdown. In addition, health status was assessed using EQ. 5D-VAS [ 27 ], and anxiety assessed using a generalized anxiety disorder (GAD) questionnaire [ 28 ]. Data on self-monitoring of blood glucose, adherence to diet plan, changes in physical activity, fruits and vegetables consumption pre- and post-lockdowns, employment status, and household income were collected using pre-tested questionnaire. Centralized online training was provided to the field workers to administer the survey over the telephone. Survey data were captured using Commcare application. Qualitative interviews were conducted in participant’s local language by trained researchers (KS, SJ) and focused on eliciting patient’s views on the challenges posed by the COVID-19 lockdowns and their mitigation.
Statistical analysis
We used a sequential mixed methods study design to guide our analytical approach [ 29 , 30 ]. Data are reported by study site and presented as a number (proportion) for categorical variables (e.g. access to health facility; diagnosed or hospitalized with COVID-19, loss of job or income) and a mean (SD) for normally distributed continuous variables (e.g., age, body mass index, health status score). GAD score [ 31 ] was defined as 0–4 no anxiety, 5–9 mild anxiety, 10–14 moderate anxiety, 15–21 severe anxiety. We performed bivariable and multivariable logistic regression analyses to find the factors associated with difficulty in accessing medicines and worsening diabetes or hypertension symptoms. We constructed three logistic regression models for each outcome. For the outcome “difficulty in accessing medicines”, Model 1 included demographic variables (age, sex, education and income); Model 2 included demographic variables and chronic conditions (diabetes, hypertension, cardiovascular disease and chronic kidney disease); Model 3, in addition to model 2 variables, included financial support from government (yes/no), loss of job (yes/no), and loss of income (yes/no) during the COVID-19 lockdowns. Next, for the outcome “worsening diabetes or hypertension symptoms”, Model 1 included demographic variables (age, sex, education and income); Model 2 included demographic variables and chronic conditions (diabetes, hypertension), GAD score (minimal, mild, moderate/severe), physical activity level, changes in fruit consumption during lockdown, and difficulty in accessing medicines (yes/no); Model 3, in addition to Model 2 variables, included financial support from the government (yes/no), loss of job (yes/no), and loss of income (yes/no) during the COVID-19 lockdowns. All data were analyzed using Stata version 16.0.
Qualitative data analyses focused on identifying views of individuals with chronic conditions, as well as the context, challenges, and mitigating factors or efforts to better manage chronic conditions during the COVID-19 pandemic in India. In-depth interviews with participants were audio-recorded, transcribed (verbatim), translated, anonymized, and checked for accuracy. The interview transcripts were coded thematically using MAXQDA software version 2020 [ 30 ]. Initial codes were developed and applied initially to a small number of transcripts, enabling further iteration of the thematic index [ 29 , 32 ]. We used illustrative non-attributable quotations.
Participant characteristics
Overall, 1734 out of 2335 contacted participants (74.3% response rate) completed the survey. We found 58 cohort members (2.5%) had died, 34 (1.5%) refused to participate, and 509 (22%) were not reachable for various reasons. Mean age (SD) of respondents was 57.8 (11.3) years, 50% were men, a majority had secondary school or college level education, and one-quarter of participants reported monthly household income of >INR 30,000 (Table 1 ). Most prevalent chronic conditions were hypertension (56%), diabetes (43%), and cardiovascular disease (13%). Of the participants surveyed, 3% were diagnosed or treated for COVID-19, 1% were hospitalized, and 69% reported that they had heard of a confirmed case of COVID-19 in their locality, more in urban (72%) than rural (58%) sites. About two-third of respondents experienced fear/anxiety related to COVID-19 and nearly half reported moderate difficulty in coping with stress during the COVID-19 lockdowns.
Rural versus urban comparison
Rural participants were disproportionately affected by the COVID-19 lockdowns compared with urban participants (Fig. 1 ). A greater proportion of rural participants experienced acute medical illness (rural 14.2%; urban 6.4%), difficulties in accessing health facilities (rural 95.0%; urban 75.0%) and medicines (rural 36.9%; urban 10.9%), worsened diabetes or hypertension symptoms (rural 16.0%; urban 11.0%), a lower treatment satisfaction rate (rural 3.5%; urban 23.8%), reduced fruit or vegetable consumption (rural 68.8%, urban 28.7%), and loss of household income (rural 67.3%, urban 56.9%).
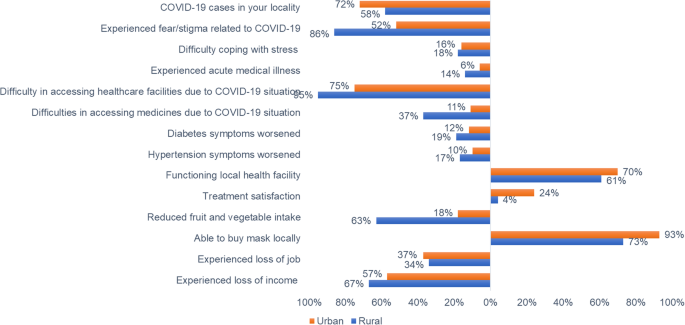
COVID-19 pandemic impacts on urban and rural people living with chronic conditions in India
Health impacts
Across the four sites, 8% of study participants experienced an acute medical illness during the COVID-19 related lockdowns (Table 2 ) with higher proportions being affected in rural sites (14%). Two-thirds of patients reported that a local health clinic/hospital was functional during the COVID-19 lockdowns. Overall, the average health status score on EQ-VAS was 76.1; this was significantly lower in rural Vizag, 71.1. Nearly half the participants with diabetes or hypertension had their fasting blood sugar (FBS) or blood pressure (BP) tested during the lockdowns. Uncontrolled diabetes (FBS > 200 mg/dl) was reported by 19.3% of participants and uncontrolled systolic BP (> 140-160 mmHg) by 15.7%. About one-third of respondents perceived their blood sugar to be controlled and 15% perceived their BP to be under control.
In the final-adjusted multivariable regression model, we found rural participants (odds ratio (OR), 95% confidence interval (CI): 4.01,2.90–5.53), having diabetes (2.42,1.81–3.25) and hypertension (1.70,1.27–2.27), and loss of income (2.30,1.62–3.26) were significantly associated with difficulty in accessing medicines. Financial aid from the government reduced the odds of difficulty in accessing medicines, i.e., had protective effect (OR: 0.69, 95%CI:0.52–0.92) (Fig. 2 and online Table S1 ). Figure 3 and online Table S2 demonstrate the factors associated with worsening of diabetes or hypertension symptoms. In the regression Model 1, adjusted for demographic and socio-economic variables, we found rural participants and females had higher odds of worsening diabetes or hypertension symptoms compared with urban or male counterparts (OR, 95%CI: 1.53,1.07–2.21 and 1.49,1.08–2.06, respectively). However, in the full multivariable-adjusted regression model, we found difficulties in accessing medicines (3.67, 2.52–5.35), loss of job (1.90, 1.25–2.89), and financial support from the government (1.87, 1.25–2.80) to be significantly associated with worsening of diabetes or hypertension symptoms.
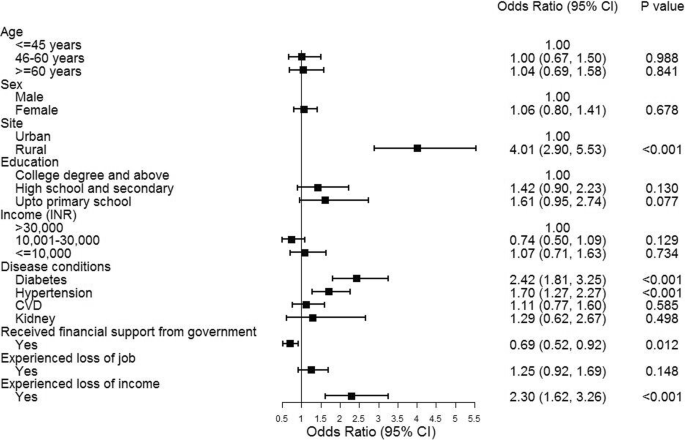
Factors associated with difficulty in accessing medicines due to the COVID-19 situation. Diabetes is defined based on fasting plasma glucose (FPG) > =126 mg/dl (7.0 mmol/l) and/or glycated haemoglobin (HbA1c) > = 6.5% (48 mmol/mol) or self-reported or on anti-diabetic medications. Hypertension was defined as being on antihypertensive medications or a systolic blood pressure > =140 mmHg and/or a diastolic blood pressure > =90 mmHg. Cardiovascular disease and chronic kidney disease were self-reported and/or on medications. INR = Indian rupees, CVD = cardiovascular disease, Kidney = chronic kidney disease, 95% CI = confidence interval
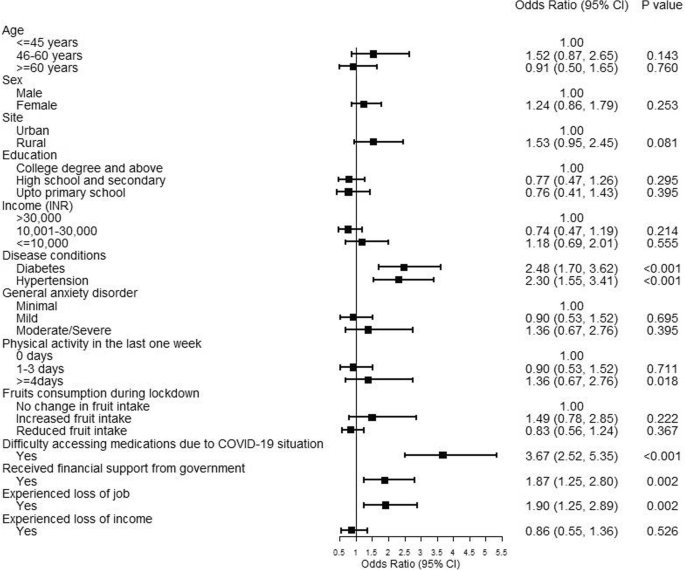
Factors associated with worsening of diabetes or hypertension symptoms during the COVID-19 lockdown. Diabetes is defined based on fasting plasma glucose (FPG) > =126 mg/dl (7.0 mmol/l) and/or glycated haemoglobin (HbA1c) > = 6.5% (48 mmol/mol) or self-reported or on anti-diabetic medications. Hypertension was defined as being on antihypertensive medications or a systolic blood pressure > =140 mmHg and/or a diastolic blood pressure > =90 mmHg. INR = Indian rupees, COVID-19 = coronavirus disease 2019, 95% CI = confidence interval
Psychosocial and economic impacts
One-third of respondents did not adhere to their recommended diet plan and reduced fruit and vegetable consumption during the lockdowns (Table 3 ). About two-third of respondents did not perform physical activity and reported loss of household income, and one-third had lost jobs. Overall, 45% of participants had received financial support from the government, with large variation by site (93% in Chennai vs. 8% in Delhi).
Because of the pandemic and related restrictions imposed to reduce its spread, few participants (15.2%) reported visiting friends/family, although about half were able to leave their locality to buy food or other supplies (52.6%). The majority reported that fruits, vegetables, and essential groceries were available during the lockdowns (96.8%), although we do not know prices or quality. Most participants (99.4%) reported being aware of and following recommended preventive measures such as wearing mask, handwashing, and social distancing (online Table S3 ).
Qualitative study results
Our sample consisted of 40 participants with one or more chronic conditions, mean age: 54.6 years, and 64% were men. Thematic redundancy was achieved with 8th interview, and two participants were then interviewed from each of the four sites (total, N = 40) to confirm thematic redundancy. Interviews lasted about 20–40 min. Two major themes emerged from qualitative data analysis: “challenges faced”, and “resilience and mitigating factors”.
Challenges faced
Most participants faced financial difficulties during the COVID-19 lockdowns. Several participants reported difficulty getting to work because of lack of public transportation. Some participants lost their jobs due to the COVID-19 pandemic, as reflected in the following quotes from study participants:
“We faced difficulties at home because I am into driving. Before lockdown, I went home for some work. Because of lockdown, I had to stay at home for 2.5 months. I, my wife, and children are jobless since then. There was no possibility of doing any work or going anywhere. We had a lot of trouble at that time.” (R-02-V)
“The impact was that there were a lot of problems. We took the ration distributed by the government. We consumed that. There were a few things [at home], we sold one or two things with the help of my daughter. My son drives a rickshaw, and my husband stays at home; we are old. It impacted him [spouse]. He was out of work for three months.” (U-10-D)
Some participants had difficulty accessing inpatient services, since many hospitals were full or refused new admissions due to COVID-19 cases. Many participants were concerned about visiting the hospital or doctor and delayed testing of their blood sugar because of fear and anxiety about COVID-19.
“I was not keeping well and none of the hospitals were taking any admission . . . they [hospital staff] said that due to COVID, beds are not available. And if you are ready to sleep on ground then we will take your admission.” (U-08-V)
“I was scared that I may not have this [coronavirus infection] but because of someone else I may get affected. We have doubt to go to the hospital, to the doctor. I didn’t want to get infected by this (COVID-19).” (U-02-D)
Participants with diabetes and hypertension were almost all aware of their elevated risk of poor outcomes if infected with SARS-CoV-2 and many feared to go out for a walk or other regular exercise.
Resilience and mitigating factors
Participants were well informed and emphasized the importance of wearing masks, practicing social distancing, or handwashing. Few participants utilized teleconsultations with doctors to avoid making in-person clinic visits. Most participants embraced the practice of enhanced personal cleanliness and other measures to proactively reduce risks of COVID-19 infection and transmission.
“We have to be careful from the corona and we have to be safe from this. That’s the only medicine now.” (U-01-D)
COVID-19 pandemic related restrictions implemented to control it had unforeseen adverse impacts on the health status, access to treatment, and achievement of care goals among people with chronic conditions in India. We found rural participants disproportionately experienced acute medical illnesses; difficulties in accessing healthcare; relatively less availability of functioning health facilities; poor treatment satisfaction; and reduced fruit and vegetable consumption.
Infectious disease epidemics have tended to have spillover effects onto the wider economy [ 14 , 33 , 34 , 35 , 36 , 37 ]. This study showed that impacts of the pandemic extend beyond health to encompass adverse effects on household incomes, individual livelihoods, interpersonal relationships, coping skills, nutritional intake, and other factors. Our quantitative and qualitative data underscore significant economic impacts from loss of employment and household income in the study population, due at least in part to restrictions preventing workers from returning to work. Those repercussions may in turn lead to further stress and additional impacts on health. People with diabetes and hypertension were worst affected due to their difficulty in accessing health care and experienced worsening symptoms or uncontrolled BP or FBS during the lockdowns, which might lead to poor health outcomes and avoidable micro- and macrovascular complications. People with chronic conditions are known to be most vulnerable to the complications of COVID-19 as highlighted in the WHO global survey and several published reports [ 8 , 9 , 23 , 38 , 39 , 40 ]. It is unclear how the dual impact of COVID-19 and the health care disruptions affect these individuals in the long-term.
Our study results are consistent with other online surveys conducted among people with chronic conditions and healthcare providers that showed the coronavirus pandemic and its related lockdowns significantly reduced access to healthcare, adversely impacted self-care behaviors, and increased mental health problems [ 11 , 15 , 16 , 24 , 38 ]. A recent study from India reported the effects of COVID-19–related lockdowns on the adoption of newer technologies and changes in glycemic control in patients with diabetes and found that the pandemic did not poorly affect glycemic control (HbA1c levels before vs. during lockdown: 8.2% vs. 7.7%). However, that study was conducted at a single private clinic, and the higher socio-economic status of the surveyed participants could influence the study results [ 41 ]. Another cross-sectional study from India evaluating the impact of COVID-19 related lockdowns on changes in health behaviors and metabolic parameters in people with diabetes found that adherence to therapy, glycemic control, and monitoring did not differ significantly pre- and post-lockdowns [ 42 ]. However, in a sub-analysis of our study, we noted significant increase in the mean FBS reported during lockdown (198 mg/dl) vs. before lockdown (165 mg/dl) in the cohort participants. This indicates that people with diabetes appear to be at greater risk of experiencing uncontrolled blood sugar during the pandemic, which is consistent with the results of another study from India that found diabetes to be the most common comorbidity among COVID-19 decedents [ 43 ]. COVID-19 has also been a major concern in higher-income countries, with many European countries and the United States experiencing significant excess mortality in 2020 and a greater proportion of deaths from NCDs at home [ 44 , 45 , 46 , 47 , 48 , 49 ].
The COVID-19 pandemic is unprecedented and serious, and several of the policy measures taken to mitigate and contain it were necessary and understandable. At the same time, we believe that the data from our study provide insights for policy makers as they consider the asymmetrical psycho-social and economic impacts of the pandemic on people with chronic conditions, especially underprivileged urban residents and underserved rural communities. In our study, rural residents and those of lower educational attainment experienced more difficulties in accessing medicines, controlling for other demographics and self-reported income. Difficulty in access to medicines, in turn was associated with worsening of diabetes or hypertension symptoms. Global supply chain disruptions during the pandemic contributed to reported shortages of essential medicines for chronic conditions [ 50 ]. Furthermore, the pandemic caused people with chronic conditions to face many lifestyle disruptions (unhealthy diet and physical inactivity, sleep disturbances, stress, and anxiety) needing remedial measures [ 16 , 51 ]. Government aid was associated with fewer difficulties in access to medicines, but varied significantly across locations, demonstrating the importance of appropriate policies at the state and local levels. To mitigate the disparities in chronic disease management and reduce the potential longer-run health impacts of the current crisis, a promising approach is to focus on enabling access to medicines for vulnerable populations (i.e., those in rural areas, with lower educational attainment, and those experiencing poverty exacerbated by loss of jobs and household income). New models of healthcare delivery combined with new skills (e.g., patient-centered orientation and leveraging consumer-facing technologies) for the health workforce can promote patient engagement and health literacy, ultimately improving health outcomes.
Our data may assist health authorities to redesign care delivery models to address the urgent needs of people with chronic conditions. We recommend a three-pronged approach to design resilient healthcare systems during and after the COVID-19 pandemic: a) develop and implement digital campaigns to disseminate information on how to adopt healthy behaviors, better self-manage NCDs, and control COVID-19; b) decentralize healthcare delivery for people with chronic conditions by involving trained community health workers and using technology-assisted medical interventions along with home monitoring devices to improve health care services; c) provide effective social and economic support for people with chronic conditions, particularly rural communities, elderly, and those with severe mental health problems. Many have experienced loss of livelihoods, isolation, stress, and anxiety during the pandemic; however, those with preexisting chronic conditions have often experienced compounding effects that exacerbate their illness [ 52 ]. Therefore, social networks and family members have an important role to play within the community and at home in monitoring and enhancing self-care behaviors among patients with chronic conditions. Although regulatory authorities in many countries have approved one or more COVID-19 vaccines for emergency use, important challenges remain in mass producing and distributing vaccines in developing countries. In addition, the lasting and complex syndemic effects of the pandemic may linger; therefore, social health measures remain important. Greater investment in prevention efforts and strengthening primary care can help save future healthcare costs, reduce the burden of NCDs, and enhance resilience against future pandemics [ 53 ].
The strength of this study lies in its empirical mixed methods study design and focus on people with chronic conditions from both urban and rural populations. It is the first such study from a populous country like India. However, future research is needed to evaluate the longer-run impact of the COVID-19 pandemic on healthcare access and health outcomes for those both with and without NCDs.
Limitations
This study has important limitations. First, the cross-sectional nature of this study limits the causal inferences between SES and chronic conditions and the COVID-19 pandemic related restrictions. Second, although the data are derived from a wide cross section of four sites, it cannot be construed as definitively representative of all urban and rural India. Third, because of the ongoing COVID-19 outbreak that we are studying, it was not possible to conduct the interviews in person; phone interviews may have limited the interpretation of qualitative data since they do not allow direct observation of participants’ expressions and body language.
In response to the rapid spread of the COVID-19 pandemic and associated health system disruptions experienced in under-resourced and low-income settings, there needs to be renewed focus on building resilient health systems that can deliver routine care using innovative telehealth approaches during the pandemic and respond to the shocks induced by infectious disease pandemics or other health crises effectively. People living in rural areas and underserved communities in urban areas faced greater challenges in access to healthcare and experienced worsening of diabetes or hypertension symptoms, as well as significant losses of income and employment. The pandemic exposed disparities in chronic disease management, but also provides opportunities to close gaps with innovations in the new post-COVID India.
Availability of data and materials
The datasets generated and analyzed during the current study are not publicly available because the datasets are currently used for another project but are available from the corresponding author on reasonable request.
Abbreviations
Blood pressure
Centre for Cardiometabolic Risk Reduction in South Asia
Centre for Chronic Disease Control
Confidence Interval
Coronavirus disease 2019
European Quality of Life 5 Dimension - Visual Analogue Scale
Fasting Blood Sugar
Generalized Anxiety Disorder
Madras Diabetes Research Foundation
Non-Communicable Disease
Severe Acute Respiratory Syndrome
Standard Deviation
World Health Organization
Coronavirus disease 2019 (COVID-19) Situation Report - 33.pdf. https://www.who.int/docs/default-source/coronaviruse/situation-reports/20200222-sitrep-33-363 (Accessed 24 Sept 2020).
Garg S, Kim L, Whitaker M, O'Halloran A, Cummings C, Holstein R, et al. Hospitalization rates and characteristics of patients hospitalized with laboratory-confirmed coronavirus disease 2019 - COVID-NET, 14 states, March 1-30, 2020. MMWR Morb Mortal Wkly Rep. 2020;69(15):458–64. https://doi.org/10.15585/mmwr.mm6915e3 .
Article CAS PubMed PubMed Central Google Scholar
Price-Haywood EG, Burton J, Fort D, Seoane L. Hospitalization and mortality among black patients and white patients with Covid-19. N Engl J Med. 2020;382(26):2534–43. https://doi.org/10.1056/NEJMsa2011686 .
Article CAS PubMed Google Scholar
Thienemann F, Pinto F, Grobbee DE, Boehm M, Bazargani N, Ge J, et al. World heart federation briefing on prevention: coronavirus disease 2019 (COVID-19) in low-income countries. Glob Heart. 2020;15(1):31. https://doi.org/10.5334/gh.778 .
Article PubMed PubMed Central Google Scholar
Thienemann F, Ntusi NAB, Battegay E, Mueller BU, Cheetham M. Multimorbidity and cardiovascular disease: a perspective on low- and middle-income countries. Cardiovasc Diagn Ther. 2020;10(2):376–85. https://doi.org/10.21037/cdt.2019.09.09 .
https://www.worldometers.info/coronavirus/?utm_campaign=homeAdvegas1? . Accessed on 30 Sept 2020.
Hamer M, Kivimäki M, Gale CR, Batty GD. Lifestyle Risk Factors for Cardiovascular Disease in Relation to COVID-19 Hospitalization: A Community-Based Cohort Study of 387,109 Adults in UK. medRxiv [Preprint]. 2020;2020.05.09.20096438. https://doi.org/10.1101/2020.05.09.20096438 . Update in: Brain Behav Immun. 2020. PMID: 32511498; PMCID: PMC7273266.
The impact of the COVID-19 pandemic on noncommunicable disease resources and services: results of a rapid assessment. Geneva: World Health Organization; 2020. https://www.who.int/publications/i/item/ncds-covid-rapid-assessment . (Accessed on 10 Mar 2021).
Chudasama YV, Gillies CL, Zaccardi F, Coles B, Davies MJ, Seidu S, et al. Impact of COVID-19 on routine care for chronic diseases: a global survey of views from healthcare professionals. Diabetes Metab Syndr. 2020;14(5):965–7. https://doi.org/10.1016/j.dsx.2020.06.042 .
Su Z, McDonnell D, Wen J, Kozak M, Abbas J, Segalo S, et al. Mental health consequences of COVID-19 media coverage: the need for effective crisis communication practices. Glob Health. 2021;17(1):4. https://doi.org/10.1186/s12992-020-00654-4 .
Article Google Scholar
Abbas J. The impact of coronavirus (SARS-CoV2) epidemic on individuals mental health: the protective measures of Pakistan in managing and sustaining transmissible disease. Psychiatr Danub. 2020;32(3–4):472–7. https://doi.org/10.24869/psyd.2020.472 .
Article PubMed Google Scholar
NeJhaddadgar N, Ziapour A, Zakkipour G, Abbas J, Abolfathi M, Shabani M. Effectiveness of telephone-based screening and triage during COVID-19 outbreak in the promoted primary healthcare system: a case study in Ardabil province, Iran. Z Gesundh Wiss. 2020:1–6. https://doi.org/10.1007/s10389-020-01407-8 . Epub ahead of print. PMID: 33224715; PMCID: PMC7665795.
Addis SG, Nega AD, Miretu DG. Psychological impact of COVID-19 pandemic on chronic disease patients in Dessie town government and private hospitals, Northeast Ethiopia. Diabetes Metab Syndr. 2021;15(1):129–35. https://doi.org/10.1016/j.dsx.2020.12.019 .
Yoosefi Lebni J, Abbas J, Moradi F, Salahshoor MR, Chaboksavar F, Irandoost SF, et al. How the COVID-19 pandemic effected economic, social, political, and cultural factors: a lesson from Iran. Int J Soc Psychiatry. 2020:20764020939984. https://doi.org/10.1177/0020764020939984 . Epub ahead of print. PMID: 32615838.
Islam N, Lacey B, Shabnam S, Erzurumluoglu AM, Dambha-Miller H, Chowell G, et al. Social inequality and the syndemic of chronic disease and COVID-19: county-level analysis in the USA. J Epidemiol Community Health. 2021:jech-2020-215626. https://doi.org/10.1136/jech-2020-215626 .
Yadav UN, Rayamajhee B, Mistry SK, Parsekar SS, Mishra SK. A syndemic perspective on the management of non-communicable diseases amid the COVID-19 pandemic in low- and middle-income countries. Front Public Health. 2020;8:508. https://doi.org/10.3389/fpubh.2020.00508 .
India State-Level Disease Burden Initiative C. Nations within a nation: variations in epidemiological transition across the states of India, 1990-2016 in the global burden of disease study. Lancet. 2017;390(10111):2437–60.
Anjana RM, Deepa M, Pradeepa R, Mahanta J, Narain K, Das HK, et al. Prevalence of diabetes and prediabetes in 15 states of India: results from the ICMR-INDIAB population-based cross-sectional study. Lancet Diabetes Endocrinol. 2017;5(8):585–96. https://doi.org/10.1016/S2213-8587(17)30174-2 .
Prabhakaran D, Jeemon P, Ghosh S, Shivashankar R, Ajay VS, Kondal D, et al. Prevalence and incidence of hypertension: results from a representative cohort of over 16,000 adults in three cities of South Asia. Indian Heart J. 2017;69(4):434–41. https://doi.org/10.1016/j.ihj.2017.05.021 .
Chatterjee P. Is India missing COVID-19 deaths? Lancet. 2020;396(10252):657. https://doi.org/10.1016/S0140-6736(20)31857-2 .
The L. COVID-19 in India: the dangers of false optimism. Lancet. 2020;396(10255):867.
Debnath R, Bardhan R. India nudges to contain COVID-19 pandemic: a reactive public policy analysis using machine-learning based topic modelling. PLoS One. 2020;15(9):e0238972. https://doi.org/10.1371/journal.pone.0238972 .
Danhieux K, Buffel V, Pairon A, Benkheil A, Remmen R, Wouters E, et al. The impact of COVID-19 on chronic care according to providers: a qualitative study among primary care practices in Belgium. BMC Fam Pract. 2020;21(1):255. https://doi.org/10.1186/s12875-020-01326-3 .
Saqib MAN, Siddiqui S, Qasim M, Jamil MA, Rafique I, Awan UA, et al. Effect of COVID-19 lockdown on patients with chronic diseases. Diabetes Metab Syndr. 2020;14(6):1621–3. https://doi.org/10.1016/j.dsx.2020.08.028 .
Nair M, Ali MK, Ajay VS, Shivashankar R, Mohan V, Pradeepa R, et al. CARRS surveillance study: design and methods to assess burdens from multiple perspectives. BMC Public Health. 2012;12(1):701. https://doi.org/10.1186/1471-2458-12-701 .
Mohan S, Jarhyan P, Ghosh S, Venkateshmurthy NS, Gupta R, Rana R, et al. UDAY: a comprehensive diabetes and hypertension prevention and management program in India. BMJ Open. 2018;8(6):e015919. https://doi.org/10.1136/bmjopen-2017-015919 .
Rabin R, de Charro F. EQ-5D: a measure of health status from the EuroQol group. Ann Med. 2001;33(5):337–43. https://doi.org/10.3109/07853890109002087 .
Toussaint A, Husing P, Gumz A, Wingenfeld K, Harter M, Schramm E, et al. Sensitivity to change and minimal clinically important difference of the 7-item generalized anxiety disorder questionnaire (GAD-7). J Affect Disord. 2020;265:395–401. https://doi.org/10.1016/j.jad.2020.01.032 .
Fofana F, Bazeley P, Regnault A. Applying a mixed methods design to test saturation for qualitative data in health outcomes research. PLoS One. 2020;15(6):e0234898. https://doi.org/10.1371/journal.pone.0234898 .
Fisher MP, Hamer MK. Qualitative methods in health policy and systems research: a framework for study planning. Qual Health Res. 2020;30(12):1899–912. https://doi.org/10.1177/1049732320921143 .
Johnson SU, Ulvenes PG, Oktedalen T, Hoffart A. Psychometric properties of the general anxiety disorder 7-item (GAD-7) scale in a heterogeneous psychiatric sample. Front Psychol. 2019;10:1713. https://doi.org/10.3389/fpsyg.2019.01713 .
Johnson GA, Vindrola-Padros C. Rapid qualitative research methods during complex health emergencies: a systematic review of the literature. Soc Sci Med. 2017;189:63–75. https://doi.org/10.1016/j.socscimed.2017.07.029 .
Fagoonee I, Pellicano R. COVID-19 brings the world economy to its knees. Minerva Med. 2020;111(4):297–9. https://doi.org/10.23736/S0026-4806.20.06603-3 .
Bowie C, Hill T. Exit strategy to control covid-19 and relaunch the economy. BMJ. 2020;369:m1851.
PubMed Google Scholar
Tiirinki H, Tynkkynen LK, Sovala M, Atkins S, Koivusalo M, Rautiainen P, et al. COVID-19 pandemic in Finland - preliminary analysis on health system response and economic consequences. Health Policy Technol. 2020;9(4):649–62. https://doi.org/10.1016/j.hlpt.2020.08.005 .
Bonaccorsi G, Pierri F, Cinelli M, Flori A, Galeazzi A, Porcelli F, et al. Economic and social consequences of human mobility restrictions under COVID-19. Proc Natl Acad Sci U S A. 2020;117(27):15530–5. https://doi.org/10.1073/pnas.2007658117 .
L'Angiocola PD, Monti M. COVID-19: the critical balance between appropriate governmental restrictions and expected economic, psychological and social consequences in Italy. Are we going in the right direction? Acta Biomed. 2020;91(2):35–8. https://doi.org/10.23750/abm.v91i2.9575 .
Serlachius A, Badawy SM, Thabrew H. Psychosocial challenges and opportunities for youth with chronic health conditions during the COVID-19 pandemic. JMIR Pediatr Parent. 2020;3(2):e23057. https://doi.org/10.2196/23057 .
Camacho-Rivera M, Islam JY, Vidot DC. Associations between chronic health conditions and COVID-19 preventive behaviors among a nationally representative sample of U.S. adults: an analysis of the COVID impact survey. Health Equity. 2020;4(1):336–44. https://doi.org/10.1089/heq.2020.0031 .
Extance A. Covid-19 and long term conditions: what if you have cancer, diabetes, or chronic kidney disease? BMJ. 2020;368:m1174.
Anjana RM, Pradeepa R, Deepa M, Jebarani S, Venkatesan U, Parvathi SJ, et al. Acceptability and utilization of newer technologies and effects on glycemic control in type 2 diabetes: lessons learned from lockdown. Diabetes Technol Ther. 2020;22(7):527–34. https://doi.org/10.1089/dia.2020.0240 .
Kovil R, Shah T, Chawla M, Karkhanis S, Padhye D, Sanghvi A, et al. Patient reported changes in metabolic health during lockdown: a cross sectional digital connect survey in people with type 2 diabetes. Diabetes Metab Syndr. 2020;14(6):1907–12. https://doi.org/10.1016/j.dsx.2020.09.031 .
Laxminarayan RWB, Dudala SR, Gopal K, Mohan C, Neelima S, Reddy KJ, et al. Epidemiology and transmission dynamics of COVID-19 in two Indian states. Science. 2020;370(6517):691–7. https://doi.org/10.1126/science.abd7672 .
Villani L, McKee M, Cascini F, Ricciardi W, Boccia S. Comparison of deaths rates for COVID-19 across Europe during the first wave of the COVID-19 pandemic. Front Public Health. 2020;8:620416. https://doi.org/10.3389/fpubh.2020.620416 .
Okell LC, Verity R, Watson OJ, Mishra S, Walker P, Whittaker C, et al. Have deaths from COVID-19 in Europe plateaued due to herd immunity? Lancet. 2020;395(10241):e110–1. https://doi.org/10.1016/S0140-6736(20)31357-X .
Khanijahani A, Tomassoni L. Socioeconomic and racial segregation and COVID-19: concentrated disadvantage and black concentration in association with COVID-19 deaths in the USA. J Racial Ethn Health Disparities. 2021. https://doi.org/10.1007/s40615-021-00965-1 .
Gecili E, Ziady A, Szczesniak RD. Forecasting COVID-19 confirmed cases, deaths and recoveries: revisiting established time series modeling through novel applications for the USA and Italy. PLoS One. 2021;16(1):e0244173. https://doi.org/10.1371/journal.pone.0244173 .
Scannell CA, Oronce CIA, Tsugawa Y. Association between county-level racial and ethnic characteristics and COVID-19 cases and deaths in the USA. J Gen Intern Med. 2020;35(10):3126–8. https://doi.org/10.1007/s11606-020-06083-8 .
Khazanchi R, Beiter ER, Gondi S, Beckman AL, Bilinski A, Ganguli I. County-level association of social vulnerability with COVID-19 cases and deaths in the USA. J Gen Intern Med. 2020;35(9):2784–7. https://doi.org/10.1007/s11606-020-05882-3 .
Badreldin HA, Atallah B. Global drug shortages due to COVID-19: impact on patient care and mitigation strategies. Res Social Adm Pharm. 2021;17(1):1946–9. https://doi.org/10.1016/j.sapharm.2020.05.017 .
Giuntella O, Hyde K, Saccardo S, Sadoff S. Lifestyle and mental health disruptions during COVID-19. Proc Natl Acad Sci U S A. 2021;118(9).
Abbas J. Crisis management, transnational healthcare challenges and opportunities: The intersection of COVID-19 pandemic and global mental health. In: Research in Globalization; 2021.
Google Scholar
Wang ZDY, Jin Y, Zheng ZJ. Coronavirus disease 2019 (COVID-19) pandemic: how countries should build more resilient health systems for preparedness and response. Glob Health J. 2020;4(4):139–45. https://doi.org/10.1016/j.glohj.2020.12.001 .
Download references
Acknowledgements
Authors acknowledge the contributions of field teams for data collection, and study participants for their participation in this study.
The CARRS Study was funded in part by the National Heart, Lung, and Blood Institute (NHLBI), National Institutes of Health (NIH), Department of Health and Human Services, under Contract No. HHSN268200900026C, and the United Health Group, Minneapolis, MN, USA. UDAY is supported by an unrestricted educational grant from Eli Lilly and Company under the Lilly NCD Partnership Program. KS is supported by funding from the Fogarty International Centre, National Institutes of Health (NIH), United States (grant award: 1K43TW011164). NSV is supported by the Fogarty International Centre and National Institute of Mental Health, of the National Institutes of Health under Award Number D43 TW010543. KMVN, and MKA, were funded in part by the National Institute of Diabetes and Digestive and Kidney Diseases of the National Institutes of Health under Award Number P30DK111024. DP was supported by Fogarty International Centre of the National Institutes of Health under Award Number U01TW010097. The funding agency had no role in the design, conduct, or analysis of the study, and no role in the decision to submit the manuscript for publication. The content is solely the responsibility of the authors and does not necessarily represent the official views of the National Institutes of Health. KE gratefully acknowledges funding from the Stanford Asia Health Policy Program, Asia-Pacific Research Centre faculty research award, and a seed grant from the Stanford Centre for Asian Health Research and Education.
Author information
Authors and affiliations.
Public Health Foundation of India, Plot number 47, Sector 44, Gurugram, New Delhi, Haryana, 122002, India
Kavita Singh, Dimple Kondal, Sailesh Mohan, Nikhil Srinivasapura Venkateshmurthy, Prashant Jarhyan & Dorairaj Prabhakaran
Centre for Chronic Disease Control, New Delhi, India
Kavita Singh, Sailesh Mohan, Suganthi Jaganathan, Nikhil Srinivasapura Venkateshmurthy & Dorairaj Prabhakaran
Deakin University, Melbourne, Australia
Sailesh Mohan
Madras Diabetes Research Foundation, Chennai, India
Mohan Deepa, Ranjit Mohan Anjana & Viswanathan Mohan
Emory University, Atlanta, GA, USA
K. M. Venkat Narayan & Mohammed K. Ali
All India Institute of Medical Sciences, New Delhi, India
Nikhil Tandon
London School of Hygiene and Tropical Medicine, London, UK
Dorairaj Prabhakaran
Stanford University, Stanford, California, USA
Karen Eggleston
You can also search for this author in PubMed Google Scholar
Contributions
KE, KS, SM, and DP contributed to the conception and design of the study. SJ, DK, DM, SVN, PJ, ARM, KS, DP, EG, KMVN, and MKA had a role in acquisition, analysis, or interpretation of data. KS, DK, MKA, and KE drafted the manuscript, and all authors critically revised the manuscript. KE, DP, MKA, NT, VM, and KMVN provided technical and material support. All authors approved the final manuscript.
Corresponding author
Correspondence to Kavita Singh .
Ethics declarations
Ethics approval and consent to participate.
Ethics approval for the study protocol was obtained from the Ethics Committee of Centre for Chronic Disease Control (CCDC), New Delhi and Madras Diabetes Research Foundation (MDRF), Chennai, Tamil Nadu, India. Verbal consent was obtained from all participants through telephone responses before the start of the survey. The Ethics Committee of CCDC and MDRF approved the procedure for obtaining informed consent.
Consent for publication
Not applicable.
Competing interests
The authors declare no conflict of interest.
Additional information
Publisher’s note.
Springer Nature remains neutral with regard to jurisdictional claims in published maps and institutional affiliations.
Supplementary Information
Additional file 1: table s1..
Factors associated with difficulty in accessing medicines during the COVID-19 lockdowns in India. Table S2 . Factors associated with worsening of diabetes or hypertension symptoms during the COVID-19 lockdowns in India. Table S3. COVID-19 pandemic-related restrictions and preventive measures.
Additional file 2.
Supplementary file.
Rights and permissions
Open Access This article is licensed under a Creative Commons Attribution 4.0 International License, which permits use, sharing, adaptation, distribution and reproduction in any medium or format, as long as you give appropriate credit to the original author(s) and the source, provide a link to the Creative Commons licence, and indicate if changes were made. The images or other third party material in this article are included in the article's Creative Commons licence, unless indicated otherwise in a credit line to the material. If material is not included in the article's Creative Commons licence and your intended use is not permitted by statutory regulation or exceeds the permitted use, you will need to obtain permission directly from the copyright holder. To view a copy of this licence, visit http://creativecommons.org/licenses/by/4.0/ . The Creative Commons Public Domain Dedication waiver ( http://creativecommons.org/publicdomain/zero/1.0/ ) applies to the data made available in this article, unless otherwise stated in a credit line to the data.
Reprints and permissions
About this article
Cite this article.
Singh, K., Kondal, D., Mohan, S. et al. Health, psychosocial, and economic impacts of the COVID-19 pandemic on people with chronic conditions in India: a mixed methods study. BMC Public Health 21 , 685 (2021). https://doi.org/10.1186/s12889-021-10708-w
Download citation
Received : 07 December 2020
Accepted : 24 March 2021
Published : 08 April 2021
DOI : https://doi.org/10.1186/s12889-021-10708-w
Share this article
Anyone you share the following link with will be able to read this content:
Sorry, a shareable link is not currently available for this article.
Provided by the Springer Nature SharedIt content-sharing initiative
- SARS coronavirus
- COVID-19 pandemic
- Chronic conditions
BMC Public Health
ISSN: 1471-2458
- General enquiries: [email protected]
Economic Impact of COVID-19
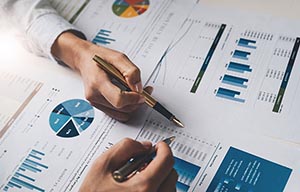
Analysis of the national and regional economic impacts of the novel coronavirus and steps taken to contain the COVID-19 outbreak
Andrew Foerster , Nick Garvey and Pierre-Daniel G. Sarte
Felipe F. Schwartzman
Elizabeth Medlin
Andreas Hornstein
Joshua Weiss and Nikhil Vellodi
Kartik B. Athreya , José Mustre-del-Río and Juan M. Sanchez
Claudia Macaluso
Huberto M. Ennis and Arantxa Jarque
Matthew Murphy
Marios Karabarbounis , Matthew Murphy and Nicholas Trachter
Hailey Phelps and Russell Wong
Zachary Bethune , Guillaume Rocheteau , Russell Wong and Cathy Zhang
Joseph Mengedoth
John Mullin
Kartik B. Athreya , Ryan Mather , José Mustre-del-Río and Juan M. Sanchez
Marios Karabarbounis , Reiko Laski , James Lee and Nicholas Trachter
Paul Ho , Thomas A. Lubik and Christian Matthes
Emily Corcoran and Nicholas Haltom
Borys Grochulski
Emily Corcoran and Sonya Ravindranath Waddell
Felipe F. Schwartzman and Tim Sablik
John Mullin , Jessie Romero and Sonya Ravindranath Waddell
Marios Karabarbounis and Nicholas Trachter
Santiago Pinto and John Bailey Jones
Jacob Crouse , David A. Price , Rachel Rodgers , Jessie Romero and Luna Shen
Abigail Crockett and Nina Mantilla
John Bailey Jones , Tim Sablik and John A. Weinberg
Thomas A. Lubik and Felipe F. Schwartzman
Grey Gordon , John Bailey Jones and Jessie Romero
Thomas A. Lubik and Sonya Ravindranath Waddell
Results from a recent national Fed survey show that community development financial institutions (CDFIs) and the communities they serve are facing less severe disruptions than last year. Still, staffing and other challenges are preventing CDFIs from fully meeting growing demand for their products and services.
Hailey Phelps
The labor force was pushed into a massive remote work experiment in 2020. What are employers in the Fifth District planning, and what challenges do they anticipate?
Sonya Ravindranath Waddell
Sam Louis Taylor
Stephanie Norris and Peter M. Dolkart
Regional businesses are returning to pre-COVID-19 operations as workers return to the office and business travel picks up.
Jason Kosakow
Roisin McCord and Laura Dawson Ullrich
The economic recovery enabled by the vaccine rollout has increased demand for Fifth District goods and services. But can supply keep up with demand?
Jacob Crouse and Sonya Ravindranath Waddell
Nicholas Haltom
Surveyed firms shared how the balance of remote versus in-office work has shifted in the past year and what changes they anticipate in the future.
Roisin McCord
Alexander Marre
Abigail Crockett and Hailey Phelps
Nicholas Haltom and Sonya Ravindranath Waddell
Surekha Carpenter , Sam Louis Taylor and Laura Dawson Ullrich
Firms report how the coronavirus is influencing their employment levels, hiring plans, and wages in our annual survey.
Surekha Carpenter
Peter M. Dolkart and Stephanie Norris
Jacob Crouse
Benjamin Lukas
John Mullin and Santiago Pinto
Stephanie Norris
Sarah Gunn , Nicholas Haltom and Donovan Pearce
Businesses report on their progress since the start of the pandemic in our July and August surveys.
July data from the Household Pulse Survey reveals some of the reasons people have not been working and how households met their spending needs.
Alaina Lee and Alexander Marre
Lucas Moyon and Laura Dawson Ullrich
Surekha Carpenter and Stephanie Norris
Lucas Kenley
The COVID-19 pandemic has put housing stability at the forefront of public policy discussions.
Benjamin Lukas and Eileen Divringi
The number of job postings dropped considerably in the United States and the Fifth District in March and April but showed signs of recovery in May.
Surekha Carpenter and Jacob Crouse
While most businesses surveyed have suffered some negative effects and loss of revenue as result of COVID-19, most remained open and operating, at least partially.
Roisin McCord and Joseph Mengedoth
Surekha Carpenter and Laura Dawson Ullrich
Joseph Mengedoth and Sam Louis Taylor
Surekha Carpenter and Emily Corcoran
Joseph Mengedoth and Roisin McCord
Surekha Carpenter and Tim Sablik
Emily Corcoran
Ann Battle Macheras
Between mid-March and early April, more than 360,000 Virginians filed for unemployment. What does this mean for employment measures and the state's economy?
Sonya Ravindranath Waddell and Jacob Crouse
Joseph Mengedoth and Sonya Ravindranath Waddell
Recent Richmond Fed research has shed new light on the functioning of the discount window and the role that stigma may play in achieving desirable outcomes.
Huberto M. Ennis and David A. Price
Jessie Romero
In response to the financial crisis and recession of 2007-09, the federal government enacted a number of emergency fiscal policies intended to aid recovery. However, the unconventional and transitory nature of these fiscal policies may have contributed to greater economic uncertainty.
Christian Matthes and Tim Sablik
Video is temporarily unavailable.
RSS Feeds of COVID-19 Related Content
- Fed in Print
- Federal Reserve Board of Governors
Phone Icon Contact Us

An official website of the United States government
The .gov means it’s official. Federal government websites often end in .gov or .mil. Before sharing sensitive information, make sure you’re on a federal government site.
The site is secure. The https:// ensures that you are connecting to the official website and that any information you provide is encrypted and transmitted securely.
- Publications
- Account settings
Preview improvements coming to the PMC website in October 2024. Learn More or Try it out now .
- Advanced Search
- Journal List
- PMC11115252

Socioeconomic impact and sufficiency of government financial support during COVID-19 pandemic: A retrospective study
Wee yeap lau.
1 Department of Decision Science, Faculty of Business and Economics, Universiti Malaya, Kuala Lumpur, Malaysia
Guek Nee Ke
2 Department of Psychology, School of Social Sciences, Heriot-Watt University Malaysia, Putrajaya, Malaysia
Tien Ming Yip
Rachel mei ming wong, khalil anwar kamal.
3 Malaysian Institute of Economic Research (MIER), Kuala Lumpur, Malaysia
Shen Ching Lee
Stephen carter.
4 Edinburgh Business School, Heriot-Watt University Edinburgh, Scotland, United Kingdom
Rozainee Khairudin
5 National University of Malaysia, Bangi, Malaysia
Dasha Grajfoner
6 DOBA Business School, Maribor, Slovenia
Associated Data
All relevant data are within the manuscript files.
This study examines the socioeconomic impact of the COVID-19 pandemic and the sufficiency of government support. Based on an online survey with 920 respondents, the cross-tabulation and binary logistic regression results show: firstly, in terms of loss of income, male respondents are more likely to have a loss of income as compared to female counterparts, and secondly, among different categories of employment status, the self-employed respondents are the most vulnerable group, given that more than 20 percent of them experienced loss of income due to the COVID-19 pandemic. Moreover, respondents working in small-and-medium enterprises (SMEs) and the informal sector are more likely to face loss of income as compared to respondents working in other sectors of employment. Likewise, respondents without tertiary education level are more likely to have a loss of income as compared to respondents with university certification. The baseline results highlight the insufficiency of government financial support programs based on the perspective of Malaysians from different demographic backgrounds. As a policy implication, the findings could guide the State in formulating the right policies for target groups who need more assistance than others in the community.
Introduction
Coronavirus disease 2019 (COVID-19) is an illness caused by a novel coronavirus, namely severe acute respiratory syndrome coronavirus 2 (SARS-CoV-2; originally called 2019-nCoV) and was first detected in Wuhan City, Hubei Province, China, during an outbreak of respiratory illness. It was reported to the World Health Organization (WHO) for the first time on 31 December 2019. The WHO labelled the COVID-19 outbreak a worldwide health emergency on 30 January 2020. On 11 March 2020, COVID-19 was declared as a global pandemic by the WHO, the first time since 2009 when H1N1 influenza was proclaimed a pandemic [ 1 ]. The pandemic triggered a variety of effects on socioeconomic mechanisms, including labour markets, consumer behaviour, and global supply chains, all of which influenced the global economy. The financial markets were, and still are, undisputedly critical components of these mechanisms [ 2 ]. In the United States, a survey found that COVID-19 led to job losses among university students [ 3 ].
Moreover, the study found that students from low-income households were more vulnerable to the pandemic crisis, given that they were 55 percent more likely to be affected by COVID-19 as compared to their high-income peers. Meanwhile, a study proposed a household-level model to simulate the socioeconomic impact of COVID-19 on household consumption, saving and poverty rate [ 4 ]. The model found that in the absence of social protection from the government, the pandemic crisis would have had a massive economic shock on households. In particular, the poverty rate would have increased by almost 8.8 percent, while household consumption and saving would have dropped significantly. Likewise, similar findings were observed for developing countries. A survey found that residents from a rural village in Sierra Leone experienced a reduction in their income level compared to the pre-COVID-19 period [ 5 ]. The surveys also revealed that residents were losing their jobs and having difficulty in sustaining their daily lives due to the pandemic. Consistent results were found in African countries [ 6 ] and Nepal [ 7 ]. Overall, the existing findings suggested that COVID-19 would have a bearing on the income level, employment status and savings of economic agents. The coronavirus outbreak caused significant concerns about public health worldwide due to its rapid spread across the globe.
Subsequently, economic consequences were also brought to the forefront as more countries were switching to a work-from-home mandate to slow the spread of the virus, restricting travel, and shutting down schools [ 8 ]. Hence, according to the OECD, the new COVID-19 pandemic had severe economic consequences; potentially posing the biggest threat to the world economy [ 9 ]. While studies have been done on the economic effects of the pandemic, most papers have focused on the economic effects caused by pandemic-related deaths [ 10 – 15 ]. For example, when examining the SARS pandemic, [ 16 ] researched the pandemic’s health cost effects on China, Hong Kong, and Taiwan. In the case of Malaysia, the COVID-19 pandemic was not the first pandemic to hit the country. Before it, there was the SARS in 2002, the H1N1 flu pandemic in 2009 and the hand, foot, and mouth disease outbreak in 2018. However, while these three pandemics have impacted the economy, none of them had as widespread an effect as is currently seen with the COVID-19 pandemic. As the number of COVID-19 cases increased in Malaysia, the Malaysian Government began imposing movement restrictions to contain the spread of the virus. These actions resulted in an economic lockdown whereby economic activity was halted. Subsequently, the lockdown resulted in less consumer demand which thus affected consumption and caused a drop in sales. As sales suffered, the profit of listed companies dropped due to lower cash flow. The drop in profits affected their stock price. Thus, it is believed that there was a need to determine the impact of COVID-19 and the subsequent government responses to it on the Malaysian stock market.
Literature review
Empirical studies have examined the impact of COVID-19 on a country’s macroeconomic performance, such as economic growth [ 17 ], stock market performance [ 18 ] and labour market conditions [ 19 ]. However, another strand of study investigated the impact of COVID-19 from the perspective of microeconomics [ 3 – 7 ]. Specifically, this study discussed the potential impact of COVID-19 on the socioeconomic wellbeing of economic agents, namely income, saving and employment status. This was important as it could inform policymakers on which group of people is more vulnerable to the pandemic crisis. Subsequently, a more focused policy could be implemented to compensate for the socioeconomic losses of economic agents as the result of COVID-19. Given that the focus of this study is on the socioeconomic impact of COVID-19, a review on the respective study was conducted.
In Malaysia, the COVID-19 pandemic crisis has seriously affected the labour market. Specifically, job losses in the country have increased by 42 percent year-on-year for the first quarter of 2020 [ 20 ]. Furthermore, the official figures from the Department of Statistics, Malaysia, show that the unemployment rate in April increased to 5 percent, as the number of people out of jobs went up to 778800, or 48.8 percent, as compared to the previous year [ 21 ]. However, the official reports and figures do not provide information on which group(s) of Malaysians are having a loss of income, loss of jobs and reduction in personal savings as the result of this pandemic. Specifically, there is no insight into which group(s) of Malaysians are most vulnerable to the COVID-19 pandemic crisis. This is important as it could provide input to the government in drafting their recovery policy so that no Malaysian is left behind. Motivated by the limitation, this study aims to investigate the impact of the COVID-19 pandemic on the socioeconomic wellbeing of Malaysians. Specifically, this study aims to reveal which group(s) of Malaysians are vulnerable to this pandemic from the perspective of income, savings, and job loss.
Aside from the psychological impact of COVID-19 on vulnerable groups such as older adults and women [ 22 ] The impact of COVID-19 on the psychological wellbeing of workers in Malaysia was a point of concern [ 23 – 26 ]. In their first study, the authors found that the relationship between psychological states and psychological wellbeing was successfully mediated by coping self-efficacy and resilience. The workers needed to have positive emotion, resilience, and coping efficacy during the pandemic period. The severity of COVID-19 on mental health in such a long-term health crisis cannot be ignored by the community and health authorities [ 23 , 24 ].
In the follow-up study, investigations on the impact of the COVID-19 pandemic on employment-related issues, followed by different response strategies, were conducted [ 25 ]. With retrenchment measures being a common response strategy during economic downturns, many individuals faced unemployment. This study explored the effect of the COVID-19 pandemic-related retrenchment on the psychological wellbeing of the Malaysian workforce.
A purposive sample of 30 retrenched participants was interviewed via telephone during Malaysia’s Movement Control Order (MCO). Thematic analysis was subsequently conducted. Six themes emerged from the thematic analysis: (1) Retrenchment leading to the deterioration of psychological wellbeing; (2) Unemployment, financial strain, and economic uncertainty; (3) Emotions related to the COVID-19 virus; (4) Coping with lifestyle change; (5) Recommendations to improve the psychological wellbeing and mental health of the retrenched workforce, and (6) Career and financial support at the recovery phase. These two studies highlighted the link between the pandemic and psychological effects, and one suggested way to alleviate the hardship was through financial aid from the State or Federal Government. Hence, this study will examine the Impact of Government aid on the citizens.
Government responses to COVID-19
As the number of cases increased, the Malaysian Government began imposing movement restrictions to prevent the spread of COVID-19 that resulted from social contact. Many activities were halted. The restrictions known as the Movement Control Order (MCO) had resulted in lesser consumer demand, and a drop in consumption and sales. Companies faced lower cash flow and drops in profit. In response to the pandemic and negative spill over to the livelihood of people, the government immediately executed several quick responses, including stimulus packages and other initiatives such as allowing employees in the private sectors to withdraw their monies from the Employee Provident Fund (EPF). In addition, the government also advised the commercial banks to impose a moratorium on loan repayment. In other words, borrowers would have additional time to repay their loans without being classified Non-Performing Loans (NPLs) by the commercial banks. Fig 1 shows the various government responses to COVID-19 throughout 2020.

Note : Author’s construction. The information in the above is sourced from various official reports and press releases.
As mentioned above, the Movement Control Order (MCO) was implemented from 18 March 2020 to 3 May 2020, though the MCO and was initially planned for only 2 weeks, from March 2020 until 31 March 2020.
At the end of the MCO, the government relaxed restrictions and implemented the Conditional Movement Control Order (CMCO) from 4 May 2020 to 9 June 2020, followed by the Recovery Movement Control Order (RMCO) from 10 June 2020 onwards, which further relaxed restrictions. Under RMCO, the government allowed non-essential economic activity to restart. Due to increased cases in Selangor and Kuala Lumpur, a Second Conditional Movement Control Order (CMCO2), tightening restrictions, was implemented in those states from 14 October 2020 to 12 January 2021.
Various stimulus packages were announced throughout the year, such as the 2020 Economic Stimulus Package, Bantuan Prihatin Nasional, PRIHATIN SME Economic Stimulus Package, PENJANA Stimulus Package and the KitaPRIHATIN Stimulus Package with a cumulative worth of RM305 billion (based on the MYR-USD exchange rate on 31 December 2020, an amount equivalent to USD 76.25 billion).
On 27 March 2020, the Malaysian Government announced Bantuan Prihatin Nasional (BPN) worth RM230 billion (USD 57.5 billion). The stimulus package was the most extensive stimulus package announced in 2020. Amongst the many highlights, this included an RM500 million (USD 125 million) allocation to the Health Ministry to fight the spread of COVID-19, a one-off cash assistance of RM1,600 to households earning below RM4,000, and an allocation of RM4.5 billion (USD 1.1 billion) prepared to aid small-and-medium enterprises (SMEs) and micro-businesses.
On 6 April 2020, the Malaysian Government announced an additional PRIHATIN SME Economic Stimulus Package worth RM10 billion (USD 2.5 billion), explicitly targeting SMEs. This stimulus package included two additional components to enhance the previously announced BPN. The two components were an additional RM7.9 billion added to the wage subsidy program announced in the BPN and a Special Prihatin Grant worth RM2.1 billion allocated to all eligible SMEs and micro-businesses.
In addition, on 5 June 2020, the Government announced the PENJANA Stimulus Package worth RM35 billion (USD 8.8 billion), which targeted households. The package included benefits for parents, including increased tax exemptions, e-vouchers for childcare services, and one-off cash handouts. Besides that, 1 GB of a free internet data plan was given to all users per day for educational and video conferencing purposes, RM50 was given for e-wallet usage, RM50 million (USD 12.5 million) was allocated for gig economies, and RM50 (USD 12.5 million) million was allocated to the B40 group for healthcare purposes.
On 23 September 2020, the Government announced the KitaPRIHATIN Stimulus Package worth RM10 billion (USD 2.5 billion). It was a stimulus package that targeted SMEs and was introduced due to feedback that many SMEs and micro-businesses were not eligible for the benefits in the BPN due to being unregistered with the Social Security Organisation (SOCSO) before 01 April 2020. This additional stimulus package was estimated to benefit 1.3 million workers. In addition, a Special Prihatin Grant was also introduced to aid micro-businesses further. Besides that, the Government also approved EPF withdrawals via i-Sinar and granted a moratorium on loan repayment.
Data and methodology
Prior to data collection, ethical approval was obtained by the Ethics Committee at School of Social Sciences, Heriot-Watt University (2020-0461-1466) on 8 th June 2020. The sample size was estimated using a single population proportion formula based on Malaysia’s estimated population size of 32.7 million at the time of study, [n = (z 2 *p (1- p)/ d2] [ 27 , 28 ] by considering the total percentage of 53.2% from the distribution of income categories from B40 and M40, a 95% confidence interval for this estimate (z = 1.96), and a 5% margin of error (d = 0.05). A minimum sample size of 383 people was required for the survey. According to the Household Income Estimate and Incidence of Poverty Report in 2019, the income distribution of income categories T20, M40 and B40 was at 46.8%, 37.2% and 16.0%, respectively [ 29 ].
Data collection took place between 24 th June to 19 th July 2020, and participants were presented the information sheet and required to provide informed consent digitally before answering the online questionnaire. Any participant who did not provide informed consent were unable to proceed with answering the questionnaire. As all participants were above the age of 18, parental or guardian consent was not required. No personally identifying information was captured, and responses were anonymised using participant IDs.
A total of 1,217 responses were collected, but those that did not provide sufficient data on the measures of interest were considered invalid and excluded from analysis and participants who did not complete the survey were removed entirely. After considering this criterion, 297 responses were excluded from the analysis, leaving a final sample size of 920, which exceeded the recommended size. Participants completed the survey during the implementation of Malaysia’s Movement Control Order (MCO).
For this study, a bivariate analysis was used to examine the socioeconomic impact of COVID-19 on Malaysians with different socio-demographic characteristics. In particular, the questions on loss of income, loss of job and a reduction in personal savings are the dependent variables and were tabulated by selected socio-demographic variables, namely gender, type of employment, sector of employment, education level, marital status, and place of residence.
This provided a first indication on which group(s) of Malaysians are more likely to be affected by COVID-19 based on the three major aspects. Next, as many of the independent variables are inter-correlated and have confounding effects on the dependent variables, binary logistic regression analyses, which allow for multivariate analysis, were conducted to ensure the robustness of the baseline results. Utilizing binary logistic regression, it was possible to show the odds of Malaysians having loss of income, loss of job and a reduction in personal saving due to the COVID-19 pandemic crisis.
Table 1 shows the list of variables used in this study. Based on the existing studies as mentioned earlier, this study defined the socioeconomic impact of COVID-19 pandemic as loss of income, job loss and a reduction in personal savings. These three dependent variables were numerated by a categorical scale, with 0 equal to ‘no’ and 1 equal to ‘yes’. The three dependent variables were selected based on the past studies [ 3 – 7 ], whereby these studies highlighted the economic impact of COVID-19 pandemic from the perspective of individual’s income level, employment status and saving. Moreover, from the perspective of macroeconomics, the three dependent variables measure the overall health and stability of the economy, thereby providing useful information to guide the conduct of fiscal policy. On the other hand, the selected demographic variables were gender, employment status, sector of employment, education level, marital status, and place of residence.
Demographic information
Table 2 presents the summary distribution of respondents based on selected socio-demographic characteristics. As observed, more than 50 percent of the respondents had a permanent job, whilst self-employed and unemployed respondents comprised 13.7 and 12.7 percent respectively of the total sample. Likewise, 54.2 percent of the respondents worked in private companies, while less than 10 percent of them were civil servants (6.6 percent). Furthermore, more than half of the respondents (59.3 percent) were educated to a tertiary level. Most of the respondents were currently resident in an urban area (84.6 percent).
Baseline results: Socioeconomic impact
Table 3 shows the result for the cross tabulation. By focusing on the loss of income, as observed, male respondents were more likely to have a loss of income as compared to female counterparts. The percentage distribution was 11 and 5.6 percent respectively. Furthermore, the chi-square test of association was significant at the 1 percent level, indicating that there was an association between loss of income and gender. Based on the results, male respondents were found to be affected the most in terms of income as compared to female respondents.
***p<0.01
**p<0.05
*p<0.1
Next, among different categories of employment status, there was a higher proportion of self-employed respondents who experienced a loss of income (22.2 percent) as compared to respondents with permanent job (5.9 percent), unemployed respondents (5.1 percent) and respondents with contract- based jobs (6.1 percent). Likewise, the chi-square test was significant at the 1 percent level, indicating that there were differences in the distribution of loss of income among respondents with different employment status.
For the sector of employment, more than 10 percent of the respondents from SMEs and informal sector were experiencing a loss of income. The percentage distribution was 16.5 and 10.3 percent, respectively. The percentage distribution was relatively higher than respondents from the government sector, listed and private companies. The chi-square test was significant at the 1 percent, suggesting that there was a relationship between loss of income and sector of employment. Based on the results, it was expected that respondents from SMEs and the informal sector were more likely to have a reduction in their income as compared to other sectors of employment.
Among respondents with different education levels, results indicated that respondents without a tertiary education qualification were more likely to have a loss of income (11.2 percent) as compared to those with university certification (5.9 percent). Lastly, the chi-square test of association was insignificant for marital status and place of residence, indicating that the proportion of loss of income was about the same for respondents who were currently single or married and in rural or urban area.
For job loss, the chi-square test of association was significant only for employment status. The proportion of job loss was higher for respondents with contract- based jobs (13.6 percent), unemployed respondents (8.5 percent) and self-employed respondents (4.8 percent) as compared to respondents with a permanent job (1.8 percent).
For reduction in personal savings, similarly, results showed that respondents with contract- based jobs (15.2 percent), unemployed respondents (12 percent) and self-employed respondents (23 percent) were more likely to have a reduction in their personal savings as compared to respondents with a permanent job (11 percent). Given that respondents with a permanent job are less likely to have a loss of income and job as compared to respondents with other employment status, it can be expected that the COVID-19 pandemic had less influence on their saving level.
Among the sector of employment, more than 20 percent of the respondents who were working in SMEs were experiencing a reduction in their personal savings as the result of the COVID-19 pandemic (24.3 percent). The percentage distribution was almost twice as high than respondents working in the government sector, private company, and informal sectors.
Robustness check: Socioeconomic impact
Table 4 shows the binary logistic regression for the three dependent variables. By focusing on the loss of income, notably, the results were in line with the above bivariate analysis, whereby the odds of having loss of income among female respondents were 0.5 times less than their male counterparts. This indicated that male respondents are the more vulnerable group and more likely to have a loss of income as compared to female respondents.
Among different employment status, the odds of having a loss of income among self-employed respondents was 3 times higher than respondents with a permanent job. Moreover, the odds for self-employed respondents were the highest among the four employment statuses. The results were in line with the above bivariate analysis, in which self-employed respondents were the most vulnerable group and they were more likely to have a loss of income as compared to respondents with other employment status.
For the sector of employment, notably, the results were consistent with the bivariate analysis. As such, the odds of having a loss of income among respondents working in a private company, SMEs and informal sector were 5, 8 and 7 times, respectively, greater than respondents working in the government sector. Similarly, respondents without university certification were almost 2 times more likely to have a loss of income as compared to those with university certification.
Furthermore, all the variables mentioned above were significant, indicating that gender, employment status, sector of employment and education level were important in explaining the loss of income among Malaysians during the COVID-19 pandemic. By and large, both cross tabulation and binary logistic regression showed consistent results.
For job loss, consistent with the above bivariate analysis, the odds of losing a job for self-employed respondents, unemployed respondents, and respondents with contract- based jobs were 2, 5 and 7 times higher, respectively, than respondents with a permanent job.
For reduction in personal savings, the binary logistic result showed that the odds of losing personal saving among self-employed respondents was 2 times higher than respondents with a permanent job. This was in line with the earlier results in loss of income and job loss. As such, self-employed respondents were found to be more likely to have a loss of income and job as compared to respondents with a permanent job. In such situations, self-employed respondents had to utilize their own savings to sustain their daily lives. Hence, this led to a reduction in their personal savings during the pandemic crisis. Meanwhile, respondents with a permanent job were less likely to be affected by the COVID-19 pandemic in terms of income and job, therefore it could be expected that they could sustain their daily life without using their private savings.
The marginal effects for each covariate were estimated to complement the odds ratio, and the results were tabulated in Table 5 . By and large, the results were consistent with the odds ratio estimated from the binary logistic regression. Specifically, the female’s probability of having loss of income would be 4.3 percentage points lower than male counterparts. For the employment status, the probability of self-employed respondents to loss their income was 10.4 percentage point higher than respondents with a permanent job. Moreover, respondents working in a private company, SMEs and informal sector exhibited a higher probability of having loss of income than respondents working in the government sector. For education, respondents without education certificate were found to have a higher probability of having loss of income as compared to their counterparts with education certificate.
The above marginal effects are estimated using the MARGIN STATA command.
For job loss, consistent with the above bivariate analysis, the probability of losing a job for unemployed respondents and respondents with contract- based jobs were higher than respondents with a permanent job. For sector of employment, respondents working in private companies were reported to have a higher probability of losing their job.
For reduction in personal savings, the probability of losing personal saving among self-employed respondents was 9.6 percentage points higher than respondents with a permanent job. Conversely, respondents working in listed companies were found to have a lower probability of having reduction in saving as compared to respondents working in the public sector.
In conclusion, the bivariate and multivariate analyses provided insights on which segments of Malaysian citizens are vulnerable to the COVID-19 pandemic. The results found that male respondents, respondents without a permanent job, respondents working in non-public sector and respondents without education certificate have exhibited substantial economic losses, in the form of income, job and savings, as a result of the COVID-19 pandemic.
Results: Sufficiency of government financial support
Fig 2 shows the basic distribution of respondents’ attitudes towards the sufficiency of government financial support programs. Overall, respondents opined that the three government financial support programs were insufficient in providing support to Malaysians during the COVID-19 period.

Baseline results: Sufficiency of government financial support
Table 6 shows the result for the cross tabulation. By focusing on the Prihatin Economic Stimulus Package, as observed, the chi-square test of association was significant for sector of employment, education level, and marital status. This indicated that there were differences in the distribution of opinion toward the sufficiency of Prihatin Economic Stimulus Package among respondents with different sector of employment, education level and marital status.
For sector of employment, it can be observed that respondents working in the non-government sector were more likely to view that the Prihatin Economic Stimulus Package was insufficient in providing financial support to them during the COVID-19 period. For education level, surprisingly, respondents with university certification were more likely to say that the financial support by government was insufficient to sustain their life during COVID-19 period as compared to respondents without university certification. The percentage distribution was 63.2 and 57.8 percent, respectively. Furthermore, there was a higher proportion of married couples who opined that the financial support by government was insufficient to cover expenses as compared to respondents who were single. The percentage distribution was 66.6 and 56.0 percent, respectively. Notably, similar findings could be observed for the Penjana Economic Stimulus Package and State Government Aid.
Robustness check: Sufficiency of government financial support
Table 7 shows the binary logistic regression for the three dependent variables. Notably, the estimation results were consistent with the above bivariate analysis, in which sector of employment, education level and marital status were found to have a significant impact on the dependent variables.
For sector of employment, it can be observed that the odds of opining that the Prihatin Economic Stimulus Package, Penjana Economic Stimulus Package and State Government Aid were insufficient in providing financial support to Malaysian was higher for respondents working in the non-government sector. Likewise, respondents with university certification were found to have a higher probability in arguing that the financial support by government was insufficient to cover their expenses as compared to respondents without university certification. Lastly, the odds of opining government financial supports were insufficient among married couple was about 2 times higher than respondents who were single. By and large, both cross tabulation and binary logistic regression showed consistent results.
Table 8 shows the marginal effect estimates for all the covariates. Notably, the estimation results were consistent with the above odds ratio. For sector of employment, it can be observed that the probability of opining that the Prihatin Economic Stimulus Package, Penjana Economic Stimulus Package and State Government Aid were insufficient in providing financial support to Malaysian was higher for respondents working in the non-government sector. Likewise, respondents without university certification were found to have a lower probability in arguing that the financial support by government was insufficient to cover their expenses as compared to respondents with university certification. Lastly, the probability of opining government financial supports was insufficient among married couples couple was higher than respondents who were single.
In conclusion, the bivariate and multivariate analyses provided insights on which segments of Malaysian citizens are receiving insufficient financial support from the government. The results found that respondents working in the non-government sector, respondents with education certification and married couple have received insufficient financial support from the government during COVID-19 period.
Conclusion and summary of key findings
Several observations can be noted from the results. As mentioned, this study consisted of two parts. The first part of study on the socioeconomic Impact of COVID-19 had the following findings: First, in terms of loss of income, male respondents were more likely to have a loss of income, compared to their female counterparts. Next, among different categories of employment status, a self-employed respondent was the most vulnerable group, given that more than 20 percent of them were having loss of income due to the COVID-19 pandemic. Moreover, respondents working in SMEs and the informal sector were more likely to face loss of income as compared to respondents working in other sectors of employment. Likewise, respondents without tertiary education, were more likely to have a loss of income as compared to respondents with university certification.
Second, in terms of job loss, respondents who were self-employed, unemployed and in contract- based jobs were more likely to have a job loss as compared to those with a permanent position. Third, in terms of personal savings, consistently, respondents with contract- based jobs, unemployed respondents and self-employed respondents were more likely to have a reduction in their personal saving as compared to respondents with a permanent job. Next, among sectors of employment, respondents working in SMEs was the most vulnerable group, given that more than 20 percent them were experiencing a reduction in their personal savings as the result of the COVID-19 pandemic. Overall, the binary logistic regression results were consistent with the baseline bivariate analysis. Hence, the results were robust and not affected by different estimation methods.
The second part of the study focused on the sufficiency of Government financial support and proffered the following findings: The baseline results highlighted the insufficiency of government financial support programs based on the perspective of Malaysians from different demographic backgrounds. Result showed that the government financial support programs, namely Prihatin Economic Stimulus Package, Penjana Economic Stimulus Package and State Government Aid were insufficient in providing financial support to Malaysians during the COVID-19 pandemic period. Respondents working in the non-government sector, respondents with university certification and married couples argued that the financial support given by government was insufficient for them to sustain their daily lives during the COVID-19 pandemic period.
In conclusion, the COVID-19 pandemic has been one of the toughest ordeals faced by human society historically. This study is important for shedding light on which segments of Malaysians are vulnerable to the COVID-19 pandemic and are receiving insufficient financial support from the government. Specifically, the male respondents, respondents without a permanent job, respondents working in non-public sector and respondents without education certificate were vulnerable to the COVID-19 pandemic. In addition, the respondents working in the non-government sector, respondents with education certification and married couples were found to receive insufficient financial support from the government during COVID-19 period. The findings provide useful input to guide the Malaysian government in formulating the right policies for target groups who need more assistance than others in the community.
Funding Statement
This study was funded by the Global Challenges Research Fund (GCRF) (Grant No.: 55-1465–4101–5815-0-R45-0- P20GCRF7). The author KGN was the recipient of the award. The funders had no role in study design, data collection and analysis, decision to publish, or preparation of the manuscript.
Data Availability
Effects on the Economy Based on Financial Crisis During COVID with Exploratory Data Analysis
Ieee account.
- Change Username/Password
- Update Address
Purchase Details
- Payment Options
- Order History
- View Purchased Documents
Profile Information
- Communications Preferences
- Profession and Education
- Technical Interests
- US & Canada: +1 800 678 4333
- Worldwide: +1 732 981 0060
- Contact & Support
- About IEEE Xplore
- Accessibility
- Terms of Use
- Nondiscrimination Policy
- Privacy & Opting Out of Cookies
A not-for-profit organization, IEEE is the world's largest technical professional organization dedicated to advancing technology for the benefit of humanity. © Copyright 2024 IEEE - All rights reserved. Use of this web site signifies your agreement to the terms and conditions.

IMAGES
COMMENTS
For over two years, the world has been battling the health and economic consequences of the COVID-19 pandemic. As of the writing of this article, deaths attributed to COVID-19 have surpassed six-and-a-half million people. Global economic growth was severely impacted: World output by the end of 2021 was more than 4 percentage points below its ...
The global socio-economic impact of COVID-19 includes higher unemployment and poverty rates, lower oil prices, altered education sectors, changes in the nature of work, lower GDPs and heightened risks to health care workers. Thus, social preparedness, as a collaboration between leaders, health care workers and researchers to foster meaningful ...
The US economy shrank by an annual rate of 4.8% in the first quarter of 2020 and by a shocking 32.9% in the second quarter, which has been the largest drop seen since 1945. The number of COVID-19 ...
Fernandes (2020) and McKibbin and Fernando (2020) reported thatthe socio-economic impact of COVID-19 will be felt for many months to come. Open in a separate window. Fig. 3. Daily confirmed new COVID-19 cases of the current 10 most affected countries based on a 5-day moving average. Valid as of August 31st, ...
To understand the potential negative economic impact of COVID‐19, it is important to comprehend the economic transmission channels through which the shocks will adversely affect the economy. ... Federal Reserve Bank of Chicago Research Paper Series. 10.21033/wp-2020-13 Bigio, S. , Zhang, M. , & Zilberman, E. (2020). ...
Brookings Institution 1 1. Introduction The impact of the pandemic on world GDP growth is massive. The COVID-19 global recession is the deepest since the end of World War II (Figure 1).
Some countries, cities, and U.S. states have so far managed to weather the COVID-19 pandemic with relatively few deaths and limited economic losses while others have suffered high death rates and ...
Brown et al. ( 6) state that the COVID-19 pandemic has had a profound impact on American lives. In the months since the COVID-19 outbreak was first diagnosed, it has spread to more than 200 countries and all U.S. states. The pandemic has had a negative impact on global economic growth for more than nearly a century.
This paper provides an account of the worldwide economic impact of the COVID‐19 shock, measured by GDP growth, employment, government spending, monetary policy, and trade. We find that the COVID‐19 shock severely impacted output growth and employment in 2020, particularly in middle‐income countries. The government response, mainly ...
The rapid spread of COVID-19 has incited vast amounts of research into its policy responses and the economic impact. McKibbin and Fernando (2020) expanded the G-Cubed model to COVID-19, analysing the impact on two agendas, one limited to China and another that impacts the whole world. Specifically, they
Global Economic Effects of COVID-19 The COVID-19 viral pandemic is an unprecedented global phenomenon that is also a highly personal experience with wide-ranging effects. On September 20, 2021, U.S. viral deaths surpassed the 675,446 total from the 1918 Spanish flu, the previously worst U.S. pandemic-related death total on record.
WDR 2022 Chapter 1. Introduction. Chapter 1. The economic impacts of the COVID-19 crisis. The COVID-19 pandemic sent shock waves through the world economy and triggered the largest global economic crisis in more than a century. The crisis led to a dramatic increase in inequality within and across countries. Preliminary evidence suggests that ...
SOURCES: IMF World Economic Outlook Reports (April 2021 and October 2019), Penn World Table (version 10.0) and author's calculations. NOTE: The COVID-19 impact is the difference between the actual gross domestic product growth rate in 2020 and the IMF forecast for it made in October 2019. Economic Policies
The Economic Impacts of COVID-19: Evidence from a New Public Database Built Using Private Sector Data. Raj Chetty, John N. Friedman, Michael Stepner & The Opportunity Insights Team. Share. X LinkedIn Email. Working Paper 27431 DOI 10.3386/w27431 Issue Date June 2020.
Epidemiologic and economic modelling of optimal COVID-19 policy: public health and social measures, masks and vaccines in Victoria, Australia, The Lancet Regional Health - Western Pacific, 32 ...
An Abstract of the Thesis of. Lucy Hudson for the degree of Bachelor of Science in the Department of Economics to be taken June 2021. Title: Pandemic Economics: A Case Study of the Economic Effects of COVID-19 Mitigation Strategies in the United States and the European Union. Approved: Assistant Professor Keaton Miller, Ph.D.
This paper undertakes a survey of literature on the economics of COVID-19 1 pandemic. 2 The goal is to explore the economic effects of the COVID-19 and suggest policy directions to mitigate its magnitude. Clark (2016) opined that a pandemic is a serial killer that can have devastating consequences on humans and the global economy.
The COVID-19 global recession is the deepest since the end of World War II (Figure 1). The global economy contracted by 3.5 percent in 2020 according to the April 2021 World Economic Outlook ...
The RER No. 17 is a collection of notes on the Economic and Social Impact of COVID-19 that has been published in three parts. The first part was launched on April 29 and focused on the macroeconomic im-pact of COVID-19. The second part, launched on May 29, shows how the macroeconomic impact affects the people in the region.
Background People with chronic conditions are disproportionately prone to be affected by the COVID-19 pandemic but there are limited data documenting this. We aimed to assess the health, psychosocial and economic impacts of the COVID-19 pandemic on people with chronic conditions in India. Methods Between July 29, to September 12, 2020, we telephonically surveyed adults (n = 2335) with chronic ...
Economic Impact of COVID-19. Analysis of the national and regional economic impacts of the novel coronavirus and steps taken to contain the COVID-19 outbreak. National Impacts. Economic Brief , December 2021, No. 21-40. Anatomy of a Pandemic Recovery Across Sectors and Regions. Andrew Foerster, Nick Garvey and Pierre-Daniel G. Sarte.
The majority of stock markets were open for trading during the lockdown period, and the impact of COVID-19 on economic activities created fear among market participants.It was found that the initial reaction of markets was mild, but with the arrival of the virus in new places, an environment of fear was created. ...
The COVID-19 pandemic has led to a dramatic loss of human life worldwide and presents an unprecedented challenge to public health, food systems and the world of work. The economic and social disruption caused by the pandemic is devastating: tens of millions of people are at risk of falling into extreme poverty, while the number of ...
Tracking the coronavirus across Europe. How countries and regions are coping with the covid-19 pandemic. A selection of our stories about covid-19 and its consequences.
Literature review. Empirical studies have examined the impact of COVID-19 on a country's macroeconomic performance, such as economic growth [], stock market performance [] and labour market conditions [].However, another strand of study investigated the impact of COVID-19 from the perspective of microeconomics [3-7].Specifically, this study discussed the potential impact of COVID-19 on the ...
The purpose of this study is to examine how COVID-19 will affect the economy. A survey was conducted to collect different opinions of people belonging to different fields related to finance. From this survey we came across that, the COVID-19 epidemic has become one of the world's most disruptive catastrophes in recent memory, tremendously impacting tremendous impact on all facets of human ...