- Data Science
- Data Analysis
- Data Visualization
- Machine Learning
- Deep Learning
- Computer Vision
- Artificial Intelligence
- AI ML DS Interview Series
- AI ML DS Projects series
- Data Engineering
- Web Scrapping

Speech Recognition Module Python
Speech recognition, a field at the intersection of linguistics, computer science, and electrical engineering, aims at designing systems capable of recognizing and translating spoken language into text. Python, known for its simplicity and robust libraries, offers several modules to tackle speech recognition tasks effectively. In this article, we'll explore the essence of speech recognition in Python, including an overview of its key libraries, how they can be implemented, and their practical applications.
Key Python Libraries for Speech Recognition
- SpeechRecognition : One of the most popular Python libraries for recognizing speech. It provides support for several engines and APIs, such as Google Web Speech API, Microsoft Bing Voice Recognition, and IBM Speech to Text. It's known for its ease of use and flexibility, making it a great starting point for beginners and experienced developers alike.
- PyAudio : Essential for audio input and output in Python, PyAudio provides Python bindings for PortAudio, the cross-platform audio I/O library. It's often used alongside SpeechRecognition to capture microphone input for real-time speech recognition.
- DeepSpeech : Developed by Mozilla, DeepSpeech is an open-source deep learning-based voice recognition system that uses models trained on the Baidu's Deep Speech research project. It's suitable for developers looking to implement more sophisticated speech recognition features with the power of deep learning.
Implementing Speech Recognition with Python
A basic implementation using the SpeechRecognition library involves several steps:
- Audio Capture : Capturing audio from the microphone using PyAudio.
- Audio Processing : Converting the audio signal into data that the SpeechRecognition library can work with.
- Recognition : Calling the recognize_google() method (or another available recognition method) on the SpeechRecognition library to convert the audio data into text.
Here's a simple example:
Practical Applications
Speech recognition has a wide range of applications:
- Voice-activated Assistants: Creating personal assistants like Siri or Alexa.
- Accessibility Tools: Helping individuals with disabilities interact with technology.
- Home Automation: Enabling voice control over smart home devices.
- Transcription Services: Automatically transcribing meetings, lectures, and interviews.
Challenges and Considerations
While implementing speech recognition, developers might face challenges such as background noise interference, accents, and dialects. It's crucial to consider these factors and test the application under various conditions. Furthermore, privacy and ethical considerations must be addressed, especially when handling sensitive audio data.
Speech recognition in Python offers a powerful way to build applications that can interact with users in natural language. With the help of libraries like SpeechRecognition, PyAudio, and DeepSpeech, developers can create a range of applications from simple voice commands to complex conversational interfaces. Despite the challenges, the potential for innovative applications is vast, making speech recognition an exciting area of development in Python.
FAQ on Speech Recognition Module in Python
What is the speech recognition module in python.
The Speech Recognition module, often referred to as SpeechRecognition, is a library that allows Python developers to convert spoken language into text by utilizing various speech recognition engines and APIs. It supports multiple services like Google Web Speech API, Microsoft Bing Voice Recognition, IBM Speech to Text, and others.
How can I install the Speech Recognition module?
You can install the Speech Recognition module by running the following command in your terminal or command prompt: pip install SpeechRecognition For capturing audio from the microphone, you might also need to install PyAudio. On most systems, this can be done via pip: pip install PyAudio
Do I need an internet connection to use the Speech Recognition module?
Yes, for most of the supported APIs like Google Web Speech, Microsoft Bing Voice Recognition, and IBM Speech to Text, an active internet connection is required. However, if you use the CMU Sphinx engine, you do not need an internet connection as it operates offline.
Similar Reads
- Python Framework
- AI-ML-DS With Python
- Python-Library
Please Login to comment...
Improve your coding skills with practice.
What kind of Experience do you want to share?
Speech Recognition in Python
Turn your code into any language with our Code Converter . It's the ultimate tool for multi-language programming. Start converting now!
Speech Recognition is the technology that allows to transform human speech into digital text. In this tutorial, you will learn how to perform automatic speech recognition with Python.
This is a hands-on guide, you will learn to use the following:
- SpeechRecognition library : This library contains several engines and APIs, both online and offline. We will use Google Speech Recognition, as it's faster to get started and doesn't require any API key. We have a separate tutorial on this .
- The Whisper API : Whisper is a robust general-purpose speech recognition model released by OpenAI . The API was made available on the 1st of March 2023. We will use the OpenAI API to perform speech recognition.
- Perform inference directly on Whisper models : Whisper is open-source. Therefore, we can directly use our computing resources to perform ASR. We will have the flexibility and the choice of which size of the whisper model to use. We also have a separate tutorial for using the Transformers library with Whisper along with Wav2Vec2 .
Table of Content
With SpeechRecognition Library
Using the whisper api, transcribing large audio files.
In this section, we will base our speech recognition system on this tutorial. SpeechRecognition library offers many transcribing engines like Google Speech Recognition, and that's what we'll be using.
Before we get started, let's install the required libraries:
Open up a new file named speechrecognition.py , and add the following:
The below function loads the audio file, performs speech recognition, and returns the text:
Next, we make a function to split the audio files into chunks in silence:
Let's give it a try:
Note: You can get all the tutorial files here .
Related: How to Convert Text to Speech in Python .
If you want a more reliable API, I suggest you use OpenAI's Whisper API. To get started, you have to sign up for an OpenAI account here .
Once you have your account, go to the API keys page , and create an API key:
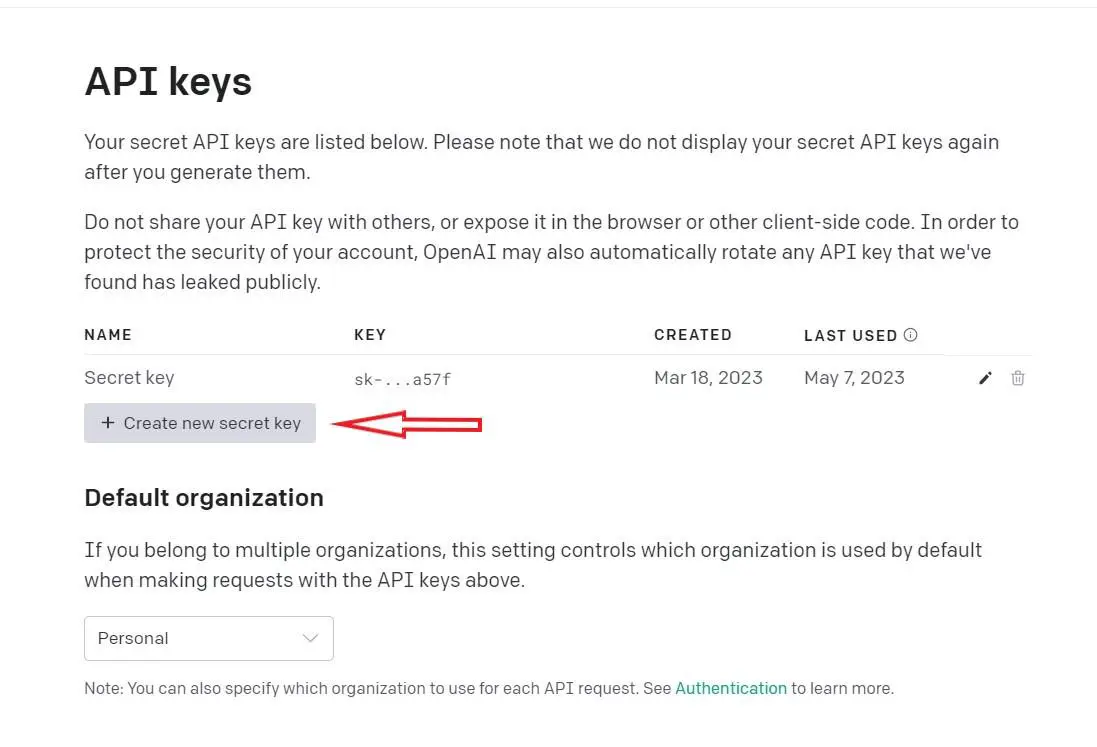
Now copy the API key to a new Python file, named whisper_api.py :
Put your API key there, and add the following code:
As you can see, using the OpenAI API is ridiculously simple, we use the openai.Audio.transcribe() method to perform speech recognition. The returning object is an OpenAIObject in which we can extract the text using the Python dict 's get() method. Here's the output:
For larger audio files, we can simply use the get_large_audio_transcription_on_silence() above function, but replace the transcribe_audio() with the get_openai_api_transcription() function, you can get the complete code here .
Using 🤗 Transformers
Whisper is a general-purpose open-source speech recognition transformer model, trained on a large dataset of diverse weakly supervised audio (680,000 hours) on multiple languages on different tasks (speech recognition, speech translation, language identification, and voice activity detection).
Whisper models demonstrate a strong ability to adjust to different datasets and domains without the necessity for fine-tuning. As in the Whisper paper : "The goal of Whisper is to develop a single robust speech processing system that works reliably without the need for dataset-specific fine-tuning to achieve high-quality results on specific distributions".
To get started, let's install the required libraries for this:
We will be using the Huggingface Transformers library to load our Whisper models. Please check this tutorial if you want a more detailed guide on this.
Open up a new Python file named transformers_whisper.py , and add the following code:
We have imported the WhisperProcessor for processing the audio before inference, and also WhisperForConditionalGeneration to perform speech recognition on the processed audio.
You can see the different model versions and sizes along with their size. The bigger the model, the better the transcription, so keep that in mind. For demonstration purposes, I'm going to use the smallest one, openai/whisper-tiny ; it should work on a regular laptop. Let's load it:
Now let's make the function responsible for loading an audio file:
Of course, the sampling rate should be fixed and only a 16,000 sampling rate works for Whisper, if you don't do this processing step, you'll have very weird and wrong transcriptions in the end.
Next, let's write the inference function:
Inside this function, we're loading our audio file, doing preprocessing, and generating the transcription before decoding the predicted IDs. Again, if you want more details, check this tutorial .
Let's try the function:
For larger audio files, we can simply use the pipeline API offered by Huggingface to perform speech recognition:
Here's the output when running the script:
This guide provided a comprehensive tutorial on how to perform automatic speech recognition using Python. It covered three main approaches: using the SpeechRecognition library with Google Speech Recognition, leveraging the Whisper API from OpenAI, and performing inference directly on open-source Whisper models using the 🤗 Transformers library.
The tutorial included code examples for each method, demonstrating how to transcribe audio files, split large audio files into chunks, and utilize different models for improved transcription accuracy.
Additionally, the article showcased the multilingual capabilities of the Whisper model and provided a pipeline for transcribing large audio files using the 🤗 Transformers library.
You can get the complete code for this tutorial here .
Learn also : Speech Recognition using Transformers in Python .
Happy transcribing ♥
Save time and energy with our Python Code Generator . Why start from scratch when you can generate? Give it a try!
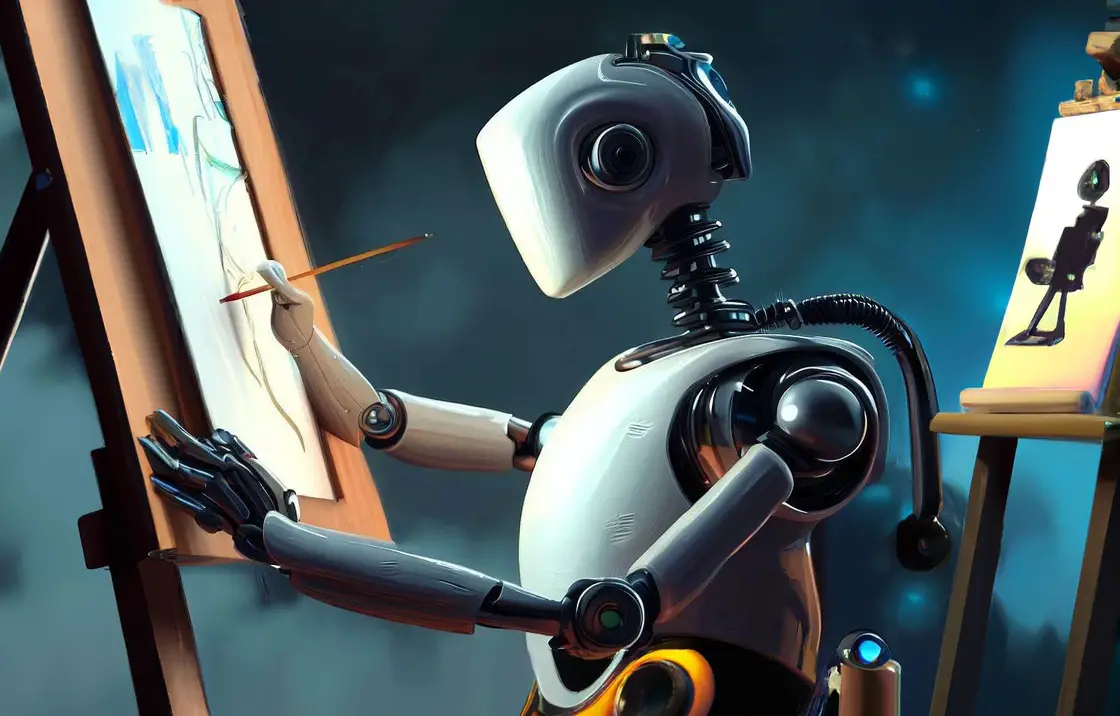
How to Generate Images from Text using Stable Diffusion in Python
Learn how to perform text-to-image using stable diffusion models with the help of huggingface transformers and diffusers libraries in Python.
- How to Convert Speech to Text in Python
Learning how to use Speech Recognition Python library for performing speech recognition to convert audio speech to text in Python.
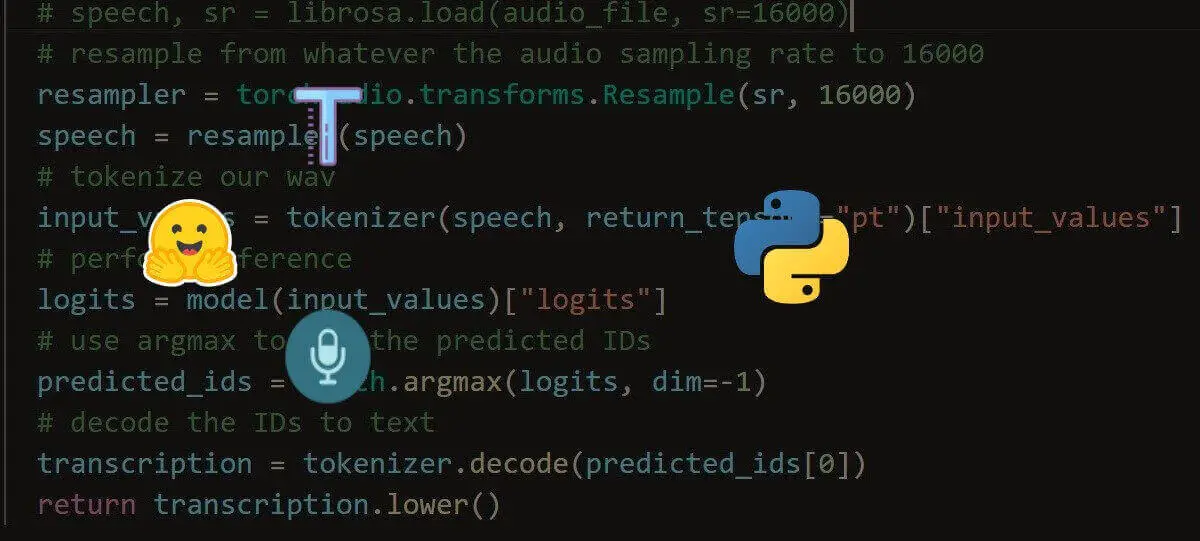
Speech Recognition using Transformers in Python
Learn how to perform speech recognition using wav2vec2 and whisper transformer models with the help of Huggingface transformers library in Python.
Comment panel
Got a coding query or need some guidance before you comment? Check out this Python Code Assistant for expert advice and handy tips. It's like having a coding tutor right in your fingertips!
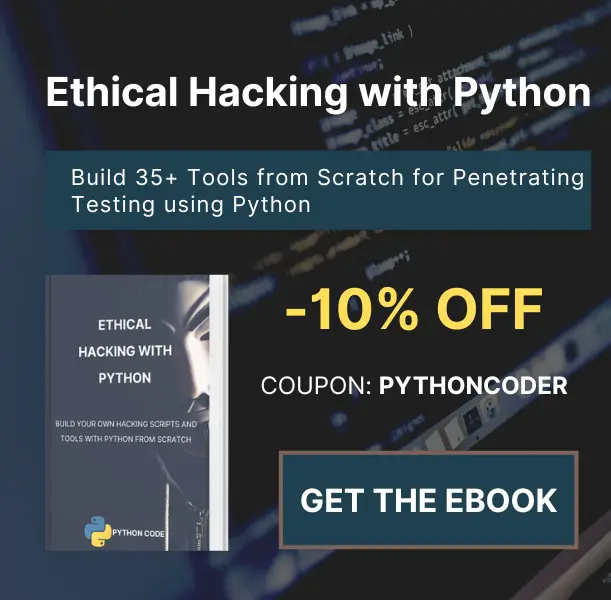
Join 50,000+ Python Programmers & Enthusiasts like you!
- Ethical Hacking
- Machine Learning
- General Python Tutorials
- Web Scraping
- Computer Vision
- Python Standard Library
- Application Programming Interfaces
- Game Development
- Web Programming
- Digital Forensics
- Natural Language Processing
- PDF File Handling
- Python for Multimedia
- GUI Programming
- Cryptography
- Packet Manipulation Using Scapy
New Tutorials
- 3 Best Online AI Code Generators
- How to Validate Credit Card Numbers in Python
- How to Make a Clickjacking Vulnerability Scanner with Python
- How to Perform Reverse DNS Lookups Using Python
- How to Check Password Strength with Python
Popular Tutorials
- How to Read Emails in Python
- How to Extract Tables from PDF in Python
- How to Make a Keylogger in Python
- How to Encrypt and Decrypt Files in Python
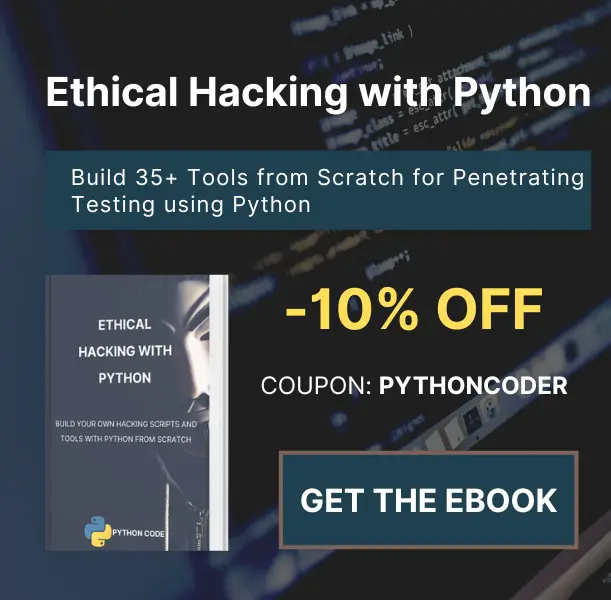
Claim your Free Chapter!
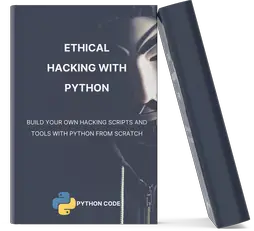

Nick McCullum
Software Developer & Professional Explainer
Python Speech Recognition - a Step-by-Step Guide
Have you used Shazam, the app that identifies music that is playing around you?
If yes, how often have you wondered about the technology that shapes this application?
How about products like Google Home or Amazon Alexa or your digital assistant Siri?
Many modern IoT products use speech recognition . This both adds creative functionality to the product and improves its accessibility features.
Python supports speech recognition and is compatible with many open-source speech recognition packages.
In this tutorial, I will teach you how to write Python speech recognition applications use an existing speech recognition package available on PyPI . We will also build a simple Guess the Word game using Python speech recognition.
Table of Contents
You can skip to a specific section of this Python speech recognition tutorial using the table of contents below:
How does speech recognition work?
Available python speech recognition packages, installing and using the speechrecognition package, the recognizer class, speech recognition from a live microphone recording, final thoughts.
Modern speech recognition software works on the Hidden Markov Model (HMM) .
According to the Hidden Markov Model, a speech signal that is broken down into fragments that are as small as one-hundredth of a second is a stationary process whose properties do not change with respect to time.
Your computer goes through a series of complex steps during speech recognition as it converts your speech to an on-screen text.
When you speak, you create an analog wave in the form of vibrations. This analog wave is converted into a digital signal that the computer can understand using a converter.
This signal is then divided into segments that are as small as one-hundredth of a second. The small segments are then matched with predefined phonemes.
Phonemes are the smallest element of a language. Linguists believe that there are around 40 phonemes in the English language.
Though this process sounds very simple, the trickiest part here is that each speaker pronounces a word slightly differently. Therefore, the way a phoneme sounds varies from speaker-to-speaker. This difference becomes especially significant across speakers from different geographical locations.
As Python developers, we are lucky to have speech recognition services that can be easily accessed through an API. Said differently, we do not need to build the infrastructure to recognize these phonemes from scratch!
Let's now look at the different Python speech recognition packages available on PyPI.
There are many Python speech recognition packages available today. Here are some of the most popular:
- google-cloud-speech
- google-speech-engine
- IBM speech to text
- Microsoft Bing voice recognition
- pocketsphinx
- SpeechRecognition
- watson-developer-cloud
In this tutorial, we will use the SpeechRecognition package, which is open-source and available on PyPI.
In this tutorial, I am assuming that you will be using Python 3.5 or above.
You can install the SpeechRecognition package with pyenv , pipenv , or virtualenv . In this tutorial, we will install the package with pipenv from a terminal.
Verify the installation of the speech recognition module using the below command.
Note: If you are using a microphone input instead of audio files present in your computer, you'll want to install the PyAudio (0.2.11 +) package as well.
The recognizer class from the speech\_recognition module is used to convert our speech to text format. Based on the API used that the user selects, the Recognizer class has seven methods. The seven methods are described in the following table:
{:.blueTable}
In this tutorial, we will use the Google Speech API . The Google Speech API is shipped in SpeechRecognition with a default API key. All the other APIs will require an API key with a username and a password.
First, create a Recognizer instance.
AudioFile is a class that is part of the speech\_recognition module and is used to recognize speech from an audio file present in your machine.
Create an object of the AudioFile class and pass the path of your audio file to the constructor of the AudioFile class. The following file formats are supported by SpeechRecognition:
Try the following script:
In the above script, you'll want to replace D:/Files/my_audio.wav with the location of your audio file.
Now, let's use the recognize_google() method to read our file. This method requires us to use a parameter of the speech_recognition() module, the AudioData object.
The Recognizer class has a record() method that can be used to convert our audio file to an AudioData object. Then, pass the AudioFile object to the record() method as shown below:
Check the type of the audio variable. You will notice that the type is speech_recognition.AudioData .
Now, use the recognize google() to invoke the audio object and convert the audio file into text.
Now that you have converted your first audio file into text, let's see how we can take only a portion of the file and convert it into text. To do this, we first need to understand the offset and duration keywords in the record() method.
The duration keyword of the record() method is used to set the time at which the speech conversion should end. That is, if you want to end your conversion after 5 seconds, specify the duration as 5. Let's see how this is done.
The output will be as follows:
It's important to note that inside a with block, the record() method moves ahead in the file stream. That is, if you record twice, say once for five seconds and then again for four seconds, the output you get for the second recording will after the first five seconds.
What if we want the audio to start from the fifth second and for a duration of 10 seconds?
This is where the offset attribute of the record() method comes to our aid. Here's how to use the offset attribute to skip the first four seconds of the file and then print the text for the next 5 seconds.
The output is as follows:
To get the exact phrase from the audio file that you are looking for, use precise values for both offset and duration attributes.
Removing Noise
The file we used in this example had very little background noise that disrupted our conversion process. However, in reality, you will encounter a lot of background noise in your speech files.
Fortunately, you can use the adjust_for_ambient_noise() method of the Recognizer class to remove any unwanted noise. This method takes the AudioData object as a parameter.
Let's see how this works:
As mentioned above, our file did not have much noise. This means that the output looks very similar to what we got earlier.
Now that we have seen speech recognition from an audio file, let's see how to perform the same function when the input is provided via a microphone. As mentioned earlier, you will have to install the PyAudio library to use your microphone.
After installing the PyAudio library, create an object of the microphone class of the speech_recognition module.
Create another instance of the Recognizer class like we did for the audio file.
Now, instead of specifying the input from a file, let us use the default microphone of the system. Access the microphone by creating an instance of the Microphone class.
Similar to the record() method, you can use the listen() method of the Recognizer class to capture input from your microphone. The first argument of the listen() method is the audio source. It records input from the microphone until it detects silence.
Execute the script and try speaking into the microphone.
The system is ready to translate your speech if it displays the You can speak now message. The program will begin translation once you stop speaking. If you do not see this message, it means that the system has failed to detect your microphone.
Python speech recognition is slowly gaining importance and will soon become an integral part of human computer interaction.
This article discussed speech recognition briefly and discussed the basics of using the Python SpeechRecognition library.

Navigation Menu
Search code, repositories, users, issues, pull requests..., provide feedback.
We read every piece of feedback, and take your input very seriously.
Saved searches
Use saved searches to filter your results more quickly.
To see all available qualifiers, see our documentation .
- Notifications You must be signed in to change notification settings
Speech recognition module for Python, supporting several engines and APIs, online and offline.
Licenses found
Uberi/speech_recognition, folders and files, repository files navigation, speechrecognition.
Library for performing speech recognition, with support for several engines and APIs, online and offline.
UPDATE 2022-02-09 : Hey everyone! This project started as a tech demo, but these days it needs more time than I have to keep up with all the PRs and issues. Therefore, I'd like to put out an open invite for collaborators - just reach out at [email protected] if you're interested!
Speech recognition engine/API support:
- CMU Sphinx (works offline)
- Google Speech Recognition
- Google Cloud Speech API
- Microsoft Azure Speech
- Microsoft Bing Voice Recognition (Deprecated)
- Houndify API
- IBM Speech to Text
- Snowboy Hotword Detection (works offline)
- Vosk API (works offline)
- OpenAI whisper (works offline)
- Whisper API
Quickstart: pip install SpeechRecognition . See the "Installing" section for more details.
To quickly try it out, run python -m speech_recognition after installing.
Project links:
- Source code
- Issue tracker
Library Reference
The library reference documents every publicly accessible object in the library. This document is also included under reference/library-reference.rst .
See Notes on using PocketSphinx for information about installing languages, compiling PocketSphinx, and building language packs from online resources. This document is also included under reference/pocketsphinx.rst .
You have to install Vosk models for using Vosk. Here are models avaiable. You have to place them in models folder of your project, like "your-project-folder/models/your-vosk-model"
See the examples/ directory in the repository root for usage examples:
- Recognize speech input from the microphone
- Transcribe an audio file
- Save audio data to an audio file
- Show extended recognition results
- Calibrate the recognizer energy threshold for ambient noise levels (see recognizer_instance.energy_threshold for details)
- Listening to a microphone in the background
- Various other useful recognizer features
First, make sure you have all the requirements listed in the "Requirements" section.
The easiest way to install this is using pip install SpeechRecognition .
Otherwise, download the source distribution from PyPI , and extract the archive.
In the folder, run python setup.py install .
Requirements
To use all of the functionality of the library, you should have:
- Python 3.9+ (required)
- PyAudio 0.2.11+ (required only if you need to use microphone input, Microphone )
- PocketSphinx (required only if you need to use the Sphinx recognizer, recognizer_instance.recognize_sphinx )
- Google API Client Library for Python (required only if you need to use the Google Cloud Speech API, recognizer_instance.recognize_google_cloud )
- FLAC encoder (required only if the system is not x86-based Windows/Linux/OS X)
- Vosk (required only if you need to use Vosk API speech recognition recognizer_instance.recognize_vosk )
- Whisper (required only if you need to use Whisper recognizer_instance.recognize_whisper )
- openai (required only if you need to use Whisper API speech recognition recognizer_instance.recognize_whisper_api )
The following requirements are optional, but can improve or extend functionality in some situations:
- If using CMU Sphinx, you may want to install additional language packs to support languages like International French or Mandarin Chinese.
The following sections go over the details of each requirement.
The first software requirement is Python 3.9+ . This is required to use the library.
PyAudio (for microphone users)
PyAudio is required if and only if you want to use microphone input ( Microphone ). PyAudio version 0.2.11+ is required, as earlier versions have known memory management bugs when recording from microphones in certain situations.
If not installed, everything in the library will still work, except attempting to instantiate a Microphone object will raise an AttributeError .
The installation instructions on the PyAudio website are quite good - for convenience, they are summarized below:
- On Windows, install with PyAudio using Pip : execute pip install SpeechRecognition[audio] in a terminal.
- If the version in the repositories is too old, install the latest release using Pip: execute sudo apt-get install portaudio19-dev python-all-dev python3-all-dev && sudo pip install SpeechRecognition[audio] (replace pip with pip3 if using Python 3).
- On OS X, install PortAudio using Homebrew : brew install portaudio . Then, install with PyAudio using Pip : pip install SpeechRecognition[audio] .
- On other POSIX-based systems, install the portaudio19-dev and python-all-dev (or python3-all-dev if using Python 3) packages (or their closest equivalents) using a package manager of your choice, and then install with PyAudio using Pip : pip install SpeechRecognition[audio] (replace pip with pip3 if using Python 3).
PyAudio wheel packages for common 64-bit Python versions on Windows and Linux are included for convenience, under the third-party/ directory in the repository root. To install, simply run pip install wheel followed by pip install ./third-party/WHEEL_FILENAME (replace pip with pip3 if using Python 3) in the repository root directory .
PocketSphinx-Python (for Sphinx users)
PocketSphinx-Python is required if and only if you want to use the Sphinx recognizer ( recognizer_instance.recognize_sphinx ).
PocketSphinx-Python wheel packages for 64-bit Python 3.4, and 3.5 on Windows are included for convenience, under the third-party/ directory . To install, simply run pip install wheel followed by pip install ./third-party/WHEEL_FILENAME (replace pip with pip3 if using Python 3) in the SpeechRecognition folder.
On Linux and other POSIX systems (such as OS X), follow the instructions under "Building PocketSphinx-Python from source" in Notes on using PocketSphinx for installation instructions.
Note that the versions available in most package repositories are outdated and will not work with the bundled language data. Using the bundled wheel packages or building from source is recommended.
Vosk (for Vosk users)
Vosk API is required if and only if you want to use Vosk recognizer ( recognizer_instance.recognize_vosk ).
You can install it with python3 -m pip install vosk .
You also have to install Vosk Models:
Here are models avaiable for download. You have to place them in models folder of your project, like "your-project-folder/models/your-vosk-model"
Google Cloud Speech Library for Python (for Google Cloud Speech API users)
Google Cloud Speech library for Python is required if and only if you want to use the Google Cloud Speech API ( recognizer_instance.recognize_google_cloud ).
If not installed, everything in the library will still work, except calling recognizer_instance.recognize_google_cloud will raise an RequestError .
According to the official installation instructions , the recommended way to install this is using Pip : execute pip install google-cloud-speech (replace pip with pip3 if using Python 3).
FLAC (for some systems)
A FLAC encoder is required to encode the audio data to send to the API. If using Windows (x86 or x86-64), OS X (Intel Macs only, OS X 10.6 or higher), or Linux (x86 or x86-64), this is already bundled with this library - you do not need to install anything .
Otherwise, ensure that you have the flac command line tool, which is often available through the system package manager. For example, this would usually be sudo apt-get install flac on Debian-derivatives, or brew install flac on OS X with Homebrew.
Whisper (for Whisper users)
Whisper is required if and only if you want to use whisper ( recognizer_instance.recognize_whisper ).
You can install it with python3 -m pip install SpeechRecognition[whisper-local] .
Whisper API (for Whisper API users)
The library openai is required if and only if you want to use Whisper API ( recognizer_instance.recognize_whisper_api ).
If not installed, everything in the library will still work, except calling recognizer_instance.recognize_whisper_api will raise an RequestError .
You can install it with python3 -m pip install SpeechRecognition[whisper-api] .
Troubleshooting
The recognizer tries to recognize speech even when i'm not speaking, or after i'm done speaking..
Try increasing the recognizer_instance.energy_threshold property. This is basically how sensitive the recognizer is to when recognition should start. Higher values mean that it will be less sensitive, which is useful if you are in a loud room.
This value depends entirely on your microphone or audio data. There is no one-size-fits-all value, but good values typically range from 50 to 4000.
Also, check on your microphone volume settings. If it is too sensitive, the microphone may be picking up a lot of ambient noise. If it is too insensitive, the microphone may be rejecting speech as just noise.
The recognizer can't recognize speech right after it starts listening for the first time.
The recognizer_instance.energy_threshold property is probably set to a value that is too high to start off with, and then being adjusted lower automatically by dynamic energy threshold adjustment. Before it is at a good level, the energy threshold is so high that speech is just considered ambient noise.
The solution is to decrease this threshold, or call recognizer_instance.adjust_for_ambient_noise beforehand, which will set the threshold to a good value automatically.
The recognizer doesn't understand my particular language/dialect.
Try setting the recognition language to your language/dialect. To do this, see the documentation for recognizer_instance.recognize_sphinx , recognizer_instance.recognize_google , recognizer_instance.recognize_wit , recognizer_instance.recognize_bing , recognizer_instance.recognize_api , recognizer_instance.recognize_houndify , and recognizer_instance.recognize_ibm .
For example, if your language/dialect is British English, it is better to use "en-GB" as the language rather than "en-US" .
The recognizer hangs on recognizer_instance.listen ; specifically, when it's calling Microphone.MicrophoneStream.read .
This usually happens when you're using a Raspberry Pi board, which doesn't have audio input capabilities by itself. This causes the default microphone used by PyAudio to simply block when we try to read it. If you happen to be using a Raspberry Pi, you'll need a USB sound card (or USB microphone).
Once you do this, change all instances of Microphone() to Microphone(device_index=MICROPHONE_INDEX) , where MICROPHONE_INDEX is the hardware-specific index of the microphone.
To figure out what the value of MICROPHONE_INDEX should be, run the following code:
This will print out something like the following:
Now, to use the Snowball microphone, you would change Microphone() to Microphone(device_index=3) .
Calling Microphone() gives the error IOError: No Default Input Device Available .
As the error says, the program doesn't know which microphone to use.
To proceed, either use Microphone(device_index=MICROPHONE_INDEX, ...) instead of Microphone(...) , or set a default microphone in your OS. You can obtain possible values of MICROPHONE_INDEX using the code in the troubleshooting entry right above this one.
The program doesn't run when compiled with PyInstaller .
As of PyInstaller version 3.0, SpeechRecognition is supported out of the box. If you're getting weird issues when compiling your program using PyInstaller, simply update PyInstaller.
You can easily do this by running pip install --upgrade pyinstaller .
On Ubuntu/Debian, I get annoying output in the terminal saying things like "bt_audio_service_open: [...] Connection refused" and various others.
The "bt_audio_service_open" error means that you have a Bluetooth audio device, but as a physical device is not currently connected, we can't actually use it - if you're not using a Bluetooth microphone, then this can be safely ignored. If you are, and audio isn't working, then double check to make sure your microphone is actually connected. There does not seem to be a simple way to disable these messages.
For errors of the form "ALSA lib [...] Unknown PCM", see this StackOverflow answer . Basically, to get rid of an error of the form "Unknown PCM cards.pcm.rear", simply comment out pcm.rear cards.pcm.rear in /usr/share/alsa/alsa.conf , ~/.asoundrc , and /etc/asound.conf .
For "jack server is not running or cannot be started" or "connect(2) call to /dev/shm/jack-1000/default/jack_0 failed (err=No such file or directory)" or "attempt to connect to server failed", these are caused by ALSA trying to connect to JACK, and can be safely ignored. I'm not aware of any simple way to turn those messages off at this time, besides entirely disabling printing while starting the microphone .
On OS X, I get a ChildProcessError saying that it couldn't find the system FLAC converter, even though it's installed.
Installing FLAC for OS X directly from the source code will not work, since it doesn't correctly add the executables to the search path.
Installing FLAC using Homebrew ensures that the search path is correctly updated. First, ensure you have Homebrew, then run brew install flac to install the necessary files.
To hack on this library, first make sure you have all the requirements listed in the "Requirements" section.
- Most of the library code lives in speech_recognition/__init__.py .
- Examples live under the examples/ directory , and the demo script lives in speech_recognition/__main__.py .
- The FLAC encoder binaries are in the speech_recognition/ directory .
- Documentation can be found in the reference/ directory .
- Third-party libraries, utilities, and reference material are in the third-party/ directory .
To install/reinstall the library locally, run python -m pip install -e .[dev] in the project root directory .
Before a release, the version number is bumped in README.rst and speech_recognition/__init__.py . Version tags are then created using git config gpg.program gpg2 && git config user.signingkey DB45F6C431DE7C2DCD99FF7904882258A4063489 && git tag -s VERSION_GOES_HERE -m "Version VERSION_GOES_HERE" .
Releases are done by running make-release.sh VERSION_GOES_HERE to build the Python source packages, sign them, and upload them to PyPI.
To run all the tests:
To run static analysis:
To ensure RST is well-formed:
Testing is also done automatically by GitHub Actions, upon every push.
FLAC Executables
The included flac-win32 executable is the official FLAC 1.3.2 32-bit Windows binary .
The included flac-linux-x86 and flac-linux-x86_64 executables are built from the FLAC 1.3.2 source code with Manylinux to ensure that it's compatible with a wide variety of distributions.
The built FLAC executables should be bit-for-bit reproducible. To rebuild them, run the following inside the project directory on a Debian-like system:
The included flac-mac executable is extracted from xACT 2.39 , which is a frontend for FLAC 1.3.2 that conveniently includes binaries for all of its encoders. Specifically, it is a copy of xACT 2.39/xACT.app/Contents/Resources/flac in xACT2.39.zip .
Please report bugs and suggestions at the issue tracker !
How to cite this library (APA style):
Zhang, A. (2017). Speech Recognition (Version 3.11) [Software]. Available from https://github.com/Uberi/speech_recognition#readme .
How to cite this library (Chicago style):
Zhang, Anthony. 2017. Speech Recognition (version 3.11).
Also check out the Python Baidu Yuyin API , which is based on an older version of this project, and adds support for Baidu Yuyin . Note that Baidu Yuyin is only available inside China.
Copyright 2014-2017 Anthony Zhang (Uberi) . The source code for this library is available online at GitHub .
SpeechRecognition is made available under the 3-clause BSD license. See LICENSE.txt in the project's root directory for more information.
For convenience, all the official distributions of SpeechRecognition already include a copy of the necessary copyright notices and licenses. In your project, you can simply say that licensing information for SpeechRecognition can be found within the SpeechRecognition README, and make sure SpeechRecognition is visible to users if they wish to see it .
SpeechRecognition distributes source code, binaries, and language files from CMU Sphinx . These files are BSD-licensed and redistributable as long as copyright notices are correctly retained. See speech_recognition/pocketsphinx-data/*/LICENSE*.txt and third-party/LICENSE-Sphinx.txt for license details for individual parts.
SpeechRecognition distributes source code and binaries from PyAudio . These files are MIT-licensed and redistributable as long as copyright notices are correctly retained. See third-party/LICENSE-PyAudio.txt for license details.
SpeechRecognition distributes binaries from FLAC - speech_recognition/flac-win32.exe , speech_recognition/flac-linux-x86 , and speech_recognition/flac-mac . These files are GPLv2-licensed and redistributable, as long as the terms of the GPL are satisfied. The FLAC binaries are an aggregate of separate programs , so these GPL restrictions do not apply to the library or your programs that use the library, only to FLAC itself. See LICENSE-FLAC.txt for license details.
Releases 30
Contributors 47.
- Python 99.6%
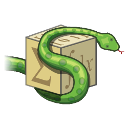
Learn Python Programming
Speech Recognition examples with Python
Speech recognition technologies have experienced immense advancements, allowing users to convert spoken language into textual data effortlessly. Python, a versatile programming language, boasts an array of libraries specifically tailored for speech recognition. Notable among these are the Google Speech Engine, Google Cloud Speech API, Microsoft Bing Voice Recognition, and IBM Speech to Text.
In this tutorial, we’ll explore how to seamlessly integrate Python with Google’s Speech Recognition Engine.
Setting Up the Development Environment
To embark on your speech recognition journey with Python, it’s imperative to equip your development environment with the right tools. The SpeechRecognition library streamlines this preparation. Whether you’re accustomed to using pyenv, pipenv, or virtualenv, this library is seamlessly compatible. For a global installation, input the command below:
It’s vital to acknowledge that the SpeechRecognition library hinges on pyaudio. The installation methodology for pyaudio might differ based on the operating system. For example, Manjaro Linux users can find packages labeled “python-pyaudio” and “python2-pyaudio”. Always ensure that you’ve selected the appropriate package tailored for your OS.
Introduction to Speech Recognition
Are you eager to test the prowess of the speech recognition module firsthand? Simply run the command given below and watch the magic unfold within your terminal:
A Closer Look at Google’s Speech Recognition Engine
Now, let’s delve deeper into the capabilities of Google’s renowned Speech Recognition engine. While this tutorial predominantly showcases its usage in English, it’s noteworthy that the engine is proficient in handling a plethora of languages.
A vital point to consider: this walkthrough employs the standard Google API key. If you’re inclined to integrate an alternate API key, modify the code accordingly:
Eager to witness this engine in action? Incorporate the code detailed below, save the file as “speechtest.py”, and initiate the script using Python 3:
Dive Deeper with Downloadable Speech Recognition Samples
Email address
Send Message
It works quite well for me
It doesn't run for me it just prints on the console "speak:" and doesn't do anything else
Check which microphone inputs are available and set the right input. Also try with different speech engines https://github.com/Uberi/sp...
How can I change the microphone settings? it doesn't seem to pick it up for me, mic is attached via USB
Make sure you have PyAudio installed. Then you can change the id of the microphone like so: Microphone(device_index=3) . Change the device_index to the microphone id you need. You can list the microphones this way:
import speech_recognition as sr for index, name in enumerate(sr.Microphone.list_microphone_names()): print('Microphone with name "{1}" found for `Microphone(device_index={0})`'.format(index, name))
Cookie policy | Privacy policy | Contact | Zen | Get

IMAGES
VIDEO