February 1, 2024
18 min read

Brains Are Not Required When It Comes to Thinking and Solving Problems—Simple Cells Can Do It
Tiny clumps of cells show basic cognitive abilities, and some animals can remember things after losing their head
By Rowan Jacobsen
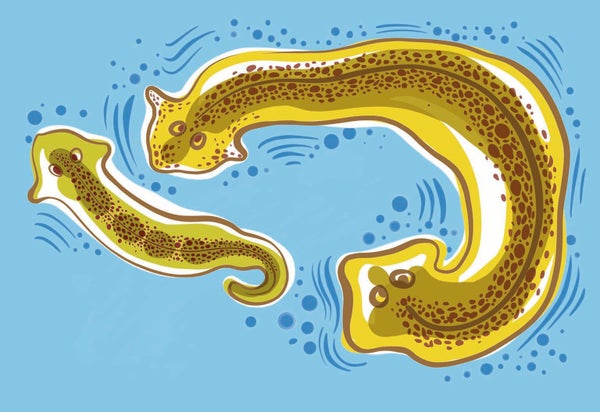
Natalya Balnova
T he planarian is nobody's idea of a genius. A flatworm shaped like a comma, it can be found wriggling through the muck of lakes and ponds worldwide. Its pin-size head has a microscopic structure that passes for a brain. Its two eyespots are set close together in a way that makes it look cartoonishly confused. It aspires to nothing more than life as a bottom-feeder.
But the worm has mastered one task that has eluded humanity's greatest minds: perfect regeneration. Tear it in half, and its head will grow a new tail while its tail grows a new head. After a week two healthy worms swim away.
Growing a new head is a neat trick. But it's the tail end of the worm that intrigues Tufts University biologist Michael Levin. He studies the way bodies develop from single cells , among other things, and his research led him to suspect that the intelligence of living things lies outside their brains to a surprising degree. Substantial smarts may be in the cells of a worm's rear end, for instance. “All intelligence is really collective intelligence, because every cognitive system is made of some kind of parts,” Levin says. An animal that can survive the complete loss of its head was Levin's perfect test subject.
On supporting science journalism
If you're enjoying this article, consider supporting our award-winning journalism by subscribing . By purchasing a subscription you are helping to ensure the future of impactful stories about the discoveries and ideas shaping our world today.
In their natural state planaria prefer the smooth and sheltered to the rough and open. Put them in a dish with a corrugated bottom, and they will huddle against the rim. But in his laboratory, about a decade ago, Levin trained some planaria to expect yummy bits of liver puree that he dripped into the middle of a ridged dish. They soon lost all fear of the rough patch, eagerly crossing the divide to get the treats. He trained other worms in the same way but in smooth dishes. Then he decapitated them all.
Levin discarded the head ends and waited two weeks while the tail ends regrew new heads. Next he placed the regenerated worms in corrugated dishes and dripped liver into the center. Worms that had lived in a smooth dish in their previous incarnation were reluctant to move. But worms regenerated from tails that had lived in rough dishes learned to go for the food more quickly. Somehow, despite the total loss of their brains, those planaria had retained the memory of the liver reward. But how? Where?
It turns out that regular cells—not just highly specialized brain cells such as neurons—have the ability to store information and act on it. Now Levin has shown that the cells do so by using subtle changes in electric fields as a type of memory. These revelations have put the biologist at the vanguard of a new field called basal cognition. Researchers in this burgeoning area have spotted hallmarks of intelligence—learning, memory, problem-solving—outside brains as well as within them.
Until recently, most scientists held that true cognition arrived with the first brains half a billion years ago. Without intricate clusters of neurons, behavior was merely a kind of reflex. But Levin and several other researchers believe otherwise. He doesn't deny that brains are awesome, paragons of computational speed and power. But he sees the differences between cell clumps and brains as ones of degree, not kind. In fact, Levin suspects that cognition probably evolved as cells started to collaborate to carry out the incredibly difficult task of building complex organisms and then got souped-up into brains to allow animals to move and think faster.
That position is being embraced by researchers in a variety of disciplines, including roboticists such as Josh Bongard, a frequent Levin collaborator who runs the Morphology, Evolution, and Cognition Laboratory at the University of Vermont. “Brains were one of the most recent inventions of Mother Nature, the thing that came last,” says Bongard, who hopes to build deeply intelligent machines from the bottom up. “It's clear that the body matters, and then somehow you add neural cognition on top. It's the cherry on the sundae. It's not the sundae.”
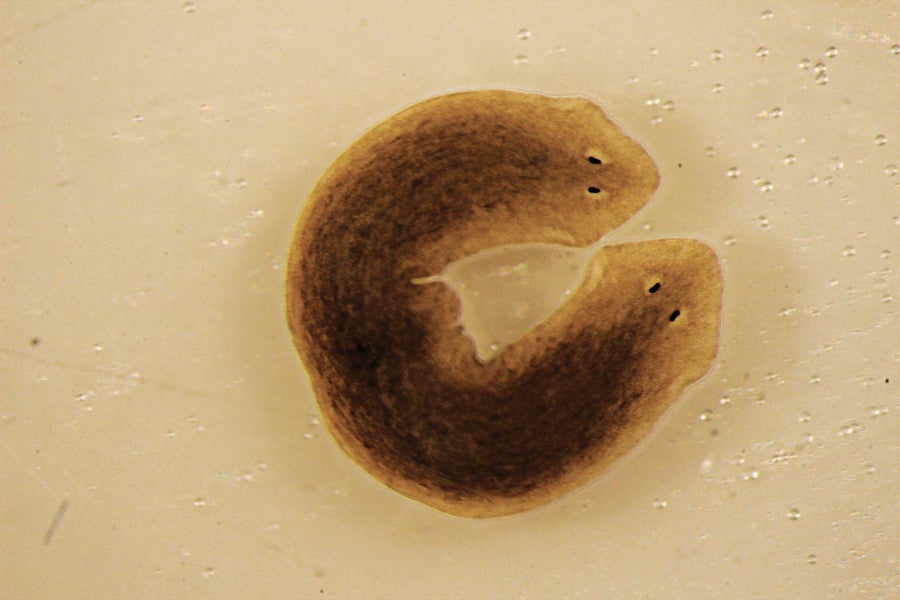
Head cells in the flatworm Dugesia japonica have different bioelectric voltages than tail cells do. Switch the voltages around and cut off the tail, and the head will regenerate a second head. Credit: Michael Levin
In recent years interest in basal cognition has exploded as researchers have recognized example after example of surprisingly sophisticated intelligence at work across life's kingdoms, no brain required. For artificial-intelligence scientists such as Bongard, basal cognition offers an escape from the trap of assuming that future intelligences must mimic the brain-centric human model. For medical specialists, there are tantalizing hints of ways to awaken cells' innate powers of healing and regeneration.
And for the philosophically minded, basal cognition casts the world in a sparkling new light. Maybe thinking builds from a simple start. Maybe it is happening all around us, every day, in forms we haven't recognized because we didn't know what to look for. Maybe minds are everywhere.
Although it now seems like a Dark Ages idea, only a few decades ago many scientists believed that nonhuman animals couldn't experience pain or other emotions. Real thought? Out of the question. The mind was the purview of humans. “It was the last beachhead,” says Pamela Lyon of the University of Adelaide, a scholar of basal cognition, who coined the term for the field in 2018. Lyon sees scientists' insistence that human intelligence is qualitatively different as just another doomed form of exceptionalism. “We've been ripped from every central position we've inhabited,” she points out. Earth is not the center of the universe. People are just another animal species. But real cognition—that was supposed to set us apart.
Now that notion, too, is in retreat as researchers document the rich inner lives of creatures increasingly distant from us. Apes, dogs, dolphins, crows and even insects are proving more savvy than suspected. In his 2022 book The Mind of a Bee , behavioral ecologist Lars Chittka chronicles his decades of work with honeybees, showing that bees can use sign language, recognize individual human faces, and remember and convey the locations of far-flung flowers . They have good moods and bad, and they can be traumatized by near-death experiences such as being grabbed by an animatronic spider hidden in a flower. (Who wouldn't be?)
But bees, of course, are animals with actual brains, so a soupçon of smarts doesn't really shake the paradigm. The bigger challenge comes from evidence of surprisingly sophisticated behavior in our brainless relatives. “The neuron is not a miracle cell,” says Stefano Mancuso, a University of Florence botanist who has written several books on plant intelligence. “It's a normal cell that is able to produce an electric signal. In plants almost every cell is able to do that.”
On one plant, the touch-me-not, feathery leaves normally fold and wilt when touched (a defense mechanism against being eaten), but when a team of scientists at the University of Western Australia and the University of Firenze in Italy conditioned the plant by jostling it throughout the day without harming it, it quickly learned to ignore the stimulus. Most remarkably, when the scientists left the plant alone for a month and then retested it, it remembered the experience. Other plants have other abilities. A Venus flytrap can count, snapping shut only if two of the sensory hairs on its trap are tripped in quick succession and pouring digestive juices into the closed trap only if its sensory hairs are tripped three more times.
These responses in plants are mediated by electric signals, just as they are in animals. Wire a flytrap to a touch-me-not, and you can make the entire touch-me-not collapse by touching a sensory hair on the flytrap. And these and other plants can be knocked out by anesthetic gas. Their electric activity flatlines, and they stop responding as if unconscious.
Plants can sense their surroundings surprisingly well. They know whether they are being shaded by part of themselves or by something else. They can detect the sound of running water (and will grow toward it) and of bees' wings (and will produce nectar in preparation). They know when they are being eaten by bugs and will produce nasty defense chemicals in response. They even know when their neighbors are under attack: when scientists played a recording of munching caterpillars to a cress plant, that was enough for the plant to send a surge of mustard oil into its leaves.
Plants' most remarkable behavior tends to get underappreciated because we see it every day: they seem to know exactly what form they have and plan their future growth based on the sights, sounds and smells around them, making complicated decisions about where future resources and dangers might be located in ways that can't be boiled down to simple formulas. As Paco Calvo, director of the Minimal Intelligence Laboratory at the University of Murcia in Spain and author of Planta Sapiens , puts it, “Plants have to plan ahead to achieve goals, and to do so, they need to integrate vast pools of data. They need to engage with their surroundings adaptively and proactively, and they need to think about the future. They just couldn't afford to do otherwise.”
None of this implies that plants are geniuses, but within their limited tool set, they show a solid ability to perceive their world and use that information to get what they need—key components of intelligence. But again, plants are a relatively easy case—no brains but lots of complexity and trillions of cells to play with. That's not the situation for single-celled organisms, which have traditionally been relegated to the “mindless” category by virtually everyone. If amoebas can think, then humans need to rethink all kinds of assumptions.
Yet the evidence for cogitating pond scum grows daily. Consider the slime mold, a cellular puddle that looks a bit like melted Velveeta and oozes through the world's forests digesting dead plant matter. Although it can be the size of a throw rug, a slime mold is one single cell with many nuclei. It has no nervous system, yet it is an excellent problem solver. When researchers from Japan and Hungary placed a slime mold at one end of a maze and a pile of oat flakes at the other, the slime mold did what slime molds do, exploring every possible option for tasty resources. But once it found the oat flakes, it retreated from all the dead ends and concentrated its body in the path that led to the oats, choosing the shortest route through the maze (of four possible solutions) every time. Inspired by that experiment, the same researchers then piled oat flakes around a slime mold in positions and quantities meant to represent the population structure of Tokyo, and the slime mold contorted itself into a very passable map of the Tokyo subway system.
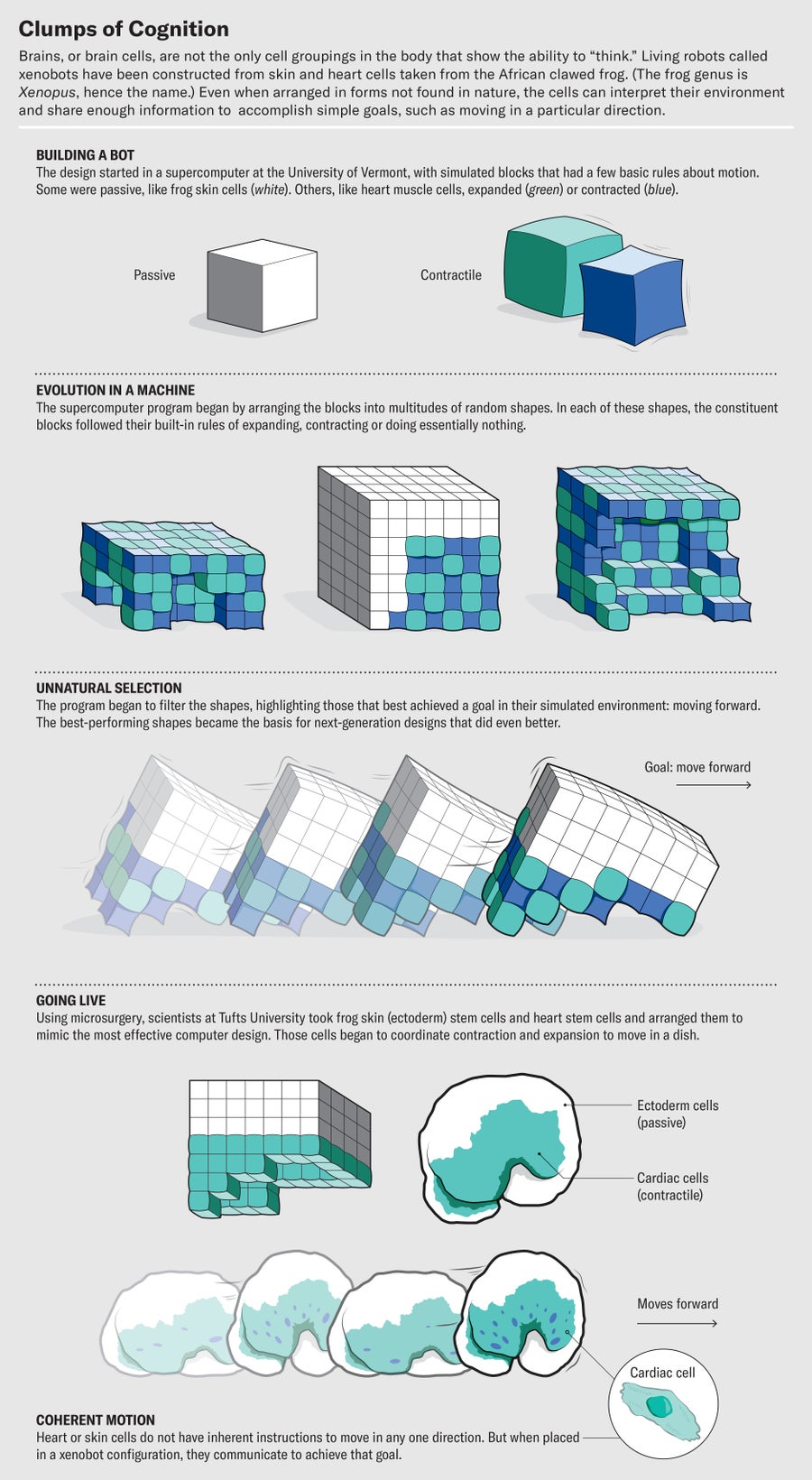
Credit: Brown Bird Design; Source: “A Scalable Pipeline for Designing Reconfigurable Organisms,” by Sam Kriegman et al., in PNAS , Vol. 117; January 2020 ( reference )
Such problem-solving could be dismissed as simple algorithms, but other experiments make it clear that slime molds can learn. When Audrey Dussutour of France's National Center for Scientific Research placed dishes of oatmeal on the far end of a bridge lined with caffeine (which slime molds find disgusting), slime molds were stymied for days, searching for a way across the bridge like an arachnophobe trying to scooch past a tarantula. Eventually they got so hungry that they went for it, crossing over the caffeine and feasting on the delicious oatmeal, and soon they lost all aversion to the formerly distasteful stuff. They had overcome their inhibitions and learned from the experience, and they retained the memory even after being put into a state of suspended animation for a year.
Which brings us back to the decapitated planaria. How can something without a brain remember anything? Where is the memory stored? Where is its mind?
T he orthodox view of memory is that it is stored as a stable network of synaptic connections among neurons in a brain. “That view is clearly cracking,” Levin says. Some of the demolition work has come from the lab of neuroscientist David Glanzman of the University of California, Los Angeles. Glanzman was able to transfer a memory of an electric shock from one sea slug to another by extracting RNA from the brains of shocked slugs and injecting it into the brains of new slugs. The recipients then “remembered” to recoil from the touch that preceded the shock. If RNA can be a medium of memory storage, any cell might have the ability, not just neurons.
Indeed, there's no shortage of possible mechanisms by which collections of cells might be able to incorporate experience. All cells have lots of adjustable pieces in their cytoskeletons and gene regulatory networks that can be set in different conformations and can inform behavior later on. In the case of the decapitated planaria, scientists still don't know for sure, but perhaps the remaining bodies were storing information in their cellular interiors that could be communicated to the rest of the body as it was rebuilt. Perhaps their nerves' basic response to rough floors had already been altered.
Levin, though, thinks something even more intriguing is going on: perhaps the impression was stored not just within the cells but in their states of interaction through bioelectricity, the subtle current that courses through all living things. Levin has spent much of his career studying how cell collectives communicate to solve sophisticated challenges during morphogenesis, or body building. How do they work together to make limbs and organs in exactly the right places? Part of that answer seems to lie in bioelectricity.
The fact that bodies have electricity flickering through them has been known for centuries, but until quite recently most biologists thought it was mostly used to deliver signals. Shoot some current through a frog's nervous system, and the frog's leg kicks. Neurons used bioelectricity to transmit information, but most scientists believed that was a specialty of brains, not bodies.
Since the 1930s, however, a small number of researchers have observed that other types of cells seem to be using bioelectricity to store and share information. Levin immersed himself in this unconventional body of work and made the next cognitive leap, drawing on his background in computer science. He'd supported himself during school by writing code, and he knew that computers used electricity to toggle their transistors between 0 and 1 and that all computer programs were built up from that binary foundation. So as an undergraduate, when he learned that all cells in the body have channels in their membranes that act like voltage gates, allowing different levels of current to pass through them, he immediately saw that such gates could function like transistors and that cells could use this electricity-driven information processing to coordinate their activities.
To find out whether voltage changes really altered the ways that cells passed information to one another, Levin turned to his planaria farm. In the 2000s he designed a way to measure the voltage at any point on a planarian and found different voltages on the head and tail ends. When he used drugs to change the voltage of the tail to that normally found in the head, the worm was unfazed. But then he cut the planarian in two, and the head end regrew a second head instead of a tail. Remarkably, when Levin cut the new worm in half, both heads grew new heads. Although the worms were genetically identical to normal planaria, the one-time change in voltage resulted in a permanent two-headed state.
For more confirmation that bioelectricity could control body shape and growth, Levin turned to African clawed frogs, common lab animals that quickly metamorphose from egg to tadpole to adult. He found that he could trigger the creation of a working eye anywhere on a tadpole by inducing a particular voltage in that spot. By simply applying the right bioelectric signature to a wound for 24 hours, he could induce regeneration of a functional leg. The cells took it from there.
“It's a subroutine call,” Levin says. In computer programming, a subroutine call is a piece of code—a kind of shorthand—that tells a machine to initiate a whole suite of lower-level mechanical actions. The beauty of this higher level of programming is that it allows us to control billions of circuits without having to open up the machine and mechanically alter each one by hand. And that was the case with building tadpole eyes. No one had to micromanage the construction of lenses, retinas, and all the other parts of an eye. It could all be controlled at the level of bioelectricity. “It's literally the cognitive glue,” Levin says. “It's what allows groups of cells to work together.”
Levin believes this discovery could have profound implications not only for our understanding of the evolution of cognition but also for human medicine. Learning to “speak cell”—to coordinate cells' behavior through bioelectricity—might help us treat cancer, a disease that occurs when part of the body stops cooperating with the rest of the body. Normal cells are programmed to function as part of the collective, sticking to the tasks assigned—liver cell, skin cell, and so on. But cancer cells stop doing their job and begin treating the surrounding body like an unfamiliar environment, striking out on their own to seek nourishment, replicate and defend themselves from attack. In other words, they act like independent organisms.
Why do they lose their group identity? In part, Levin says, because the mechanisms that maintain the cellular mind meld can fail. “Stress, chemicals, genetic mutations can all cause a breakdown of this communication,” he says. His team has been able to induce tumors in frogs just by forcing a “bad” bioelectric pattern onto healthy tissue. It's as if the cancer cells stop receiving their orders and go rogue.
Even more tantalizingly, Levin has dissipated tumors by reintroducing the proper bioelectric pattern—in effect reestablishing communication between the breakaway cancer and the body, as if he's bringing a sleeper cell back into the fold. At some point in the future, he speculates, bioelectric therapy might be applied to human cancers, stopping tumors from growing. It also could play a role in regenerating failing organs—kidneys, say, or hearts—if scientists can crack the bioelectric code that tells cells to start growing in the right patterns. With tadpoles, in fact, Levin showed that animals suffering from massive brain damage at birth were able to build normal brains after the right shot of bioelectricity.
L evin's research has always had tangible applications, such as cancer therapy, limb regeneration and wound healing. But over the past few years he's allowed a philosophical current to enter his papers and talks. “It's been sort of a slow rollout,” he confesses. “I've had these ideas for decades, but it wasn't the right time to talk about it.”
That began to change with a celebrated 2019 paper entitled “The Computational Boundary of a Self,” in which he harnessed the results of his experiments to argue that we are all collective intelligences built out of smaller, highly competent problem-solving agents . As Vermont's Bongard told the New York Times , “What we are is intelligent machines made of intelligent machines made of intelligent machines all the way down.”
For Levin, that realization came in part from watching the bodies of his clawed frogs as they developed. In frogs' transformation from tadpole to adult, their faces undergo massive remodeling. The head changes shape, and the eyes, mouth and nostrils all migrate to new positions. The common assumption has been that these rearrangements are hardwired and follow simple mechanical algorithms carried out by genes, but Levin suspected it wasn't so preordained. So he electrically scrambled the normal development of frog embryos to create tadpoles with eyes, nostrils and mouths in all the wrong places. Levin dubbed them “Picasso tadpoles,” and they truly looked the part.
If the remodeling were preprogrammed, the final frog face should have been as messed up as the tadpole. Nothing in the frog's evolutionary past gave it genes for dealing with such a novel situation. But Levin watched in amazement as the eyes and mouths found their way to the right arrangement while the tadpoles morphed into frogs. The cells had an abstract goal and worked together to achieve it. “This is intelligence in action,” Levin wrote, “the ability to reach a particular goal or solve a problem by undertaking new steps in the face of changing circumstances.” Fused into a hive mind through bioelectricity, the cells achieved feats of bioengineering well beyond those of our best gene jockeys.
Some of the most intense interest in Levin's work has come from the fields of artificial intelligence and robotics, which see in basal cognition a way to address some core weaknesses. For all their remarkable prowess in manipulating language or playing games with well-defined rules, AIs still struggle immensely to understand the physical world. They can churn out sonnets in the style of Shakespeare, but ask them how to walk or to predict how a ball will roll down a hill, and they are clueless.
According to Bongard, that's because these AIs are, in a sense, too heady. “If you play with these AIs, you can start to see where the cracks are. And they tend to be around things like common sense and cause and effect, which points toward why you need a body. If you have a body, you can learn about cause and effect because you can cause effects . But these AI systems can't learn about the world by poking at it.”
Bongard is at the vanguard of the “embodied cognition” movement, which seeks to design robots that learn about the world by monitoring the way their form interacts with it. For an example of embodied cognition in action, he says, look no further than his one-and-a-half-year-old child, “who is probably destroying the kitchen right now. That's what toddlers do. They poke the world, literally and metaphorically, and then watch how the world pushes back. It's relentless.”
Bongard's lab uses AI programs to design robots out of flexible, LEGO-like cubes that he calls “ Minecraft for robotics.” The cubes act like blocky muscle, allowing the robots to move their bodies like caterpillars. The AI-designed robots learn by trial and error, adding and subtracting cubes and “evolving” into more mobile forms as the worst designs get eliminated.
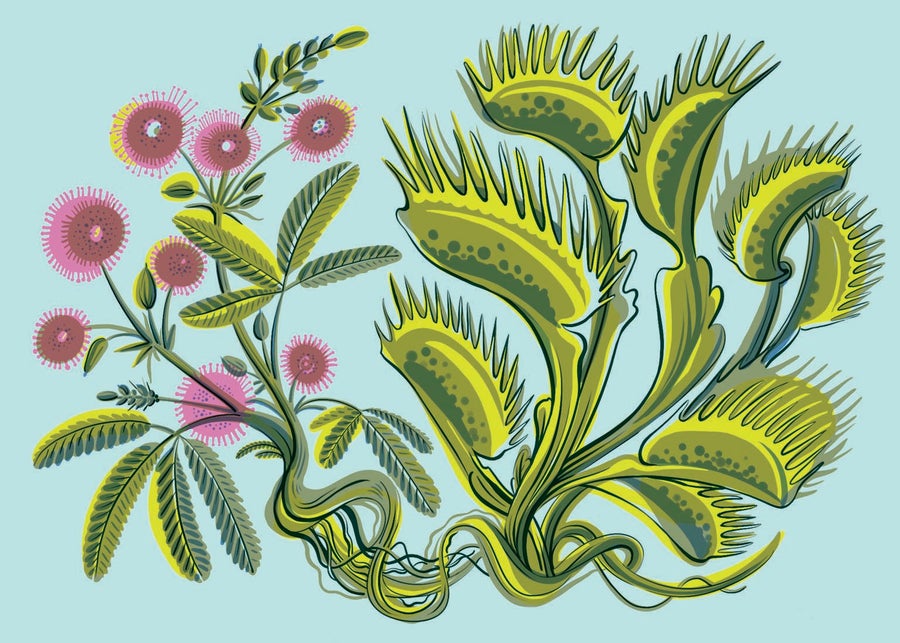
Plants use bioelectricity to communicate and take action. If you brush a sensory hair on a Venus flytrap ( right ), and the flytrap is wired to a touch-me-not plant ( left ), leaves on the touch-me-not will fold and wilt. Credit: Natalya Balnova
In 2020 Bongard's AI discovered how to make robots walk. That accomplishment inspired Levin's lab to use microsurgery to remove live skin stem cells from an African clawed frog and nudge them together in water. The cells fused into a lump the size of a sesame seed and acted as a unit. Skin cells have cilia, tiny hairs that typically hold a layer of protective mucus on the surface of an adult frog, but these creations used their cilia like oars, rowing through their new world. They navigated mazes and even closed up wounds when injured. Freed from their confined existence in a biological cubicle, they became something new and made the best of their situation. They definitely weren't frogs, despite sharing the identical genome. But because the cells originally came from frogs of the genus Xenopus , Levin and Bongard nicknamed the things “xenobots.” In 2023 they showed similar feats could be achieved by pieces of another species: human lung cells. Clumps of the human cells self-assembled and moved around in specific ways. The Tufts team named them “anthrobots.”
To Levin, the xenobots and anthrobots are another sign that we need to rethink the way cognition plays out in the actual world. “Typically when you ask about a given living thing, you ask, ‘Why does it have the shape it has? Why does it have the behaviors it has?' And the standard answer is evolution, of course. For eons it was selected for. Well, guess what? There have never been any xenobots. There's never been any pressure to be a good xenobot. So why do these things do what they do within 24 hours of finding themselves in the world? I think it's because evolution does not produce specific solutions to specific problems. It produces problem-solving machines.”
Xenobots and anthrobots are, of course, quite limited in their capabilities, but perhaps they provide a window into how intelligence might naturally scale up when individual units with certain goals and needs come together to collaborate. Levin sees this innate tendency toward innovation as one of the driving forces of evolution, pushing the world toward a state of, as Charles Darwin might have put it, endless forms most beautiful. “We don't really have a good vocabulary for it yet,” he says, “but I honestly believe that the future of all this is going to look more like psychiatry talk than chemistry talk. We're going to end up having a calculus of pressures and memories and attractions.”
Levin hopes this vision will help us overcome our struggle to acknowledge minds that come in packages bearing little resemblance to our own, whether they are made of slime or silicon. For Adelaide's Lyon, recognizing that kinship is the real promise of basal cognition. “We think we are the crown of creation,” she says. “But if we start realizing that we have a whole lot more in common with the blades of grass and the bacteria in our stomachs—that we are related at a really, really deep level—it changes the entire paradigm of what it is to be a human being on this planet.”
Indeed, the very act of living is by default a cognitive state, Lyon says. Every cell needs to be constantly evaluating its surroundings, making decisions about what to let in and what to keep out and planning its next steps. Cognition didn't arrive later in evolution. It's what made life possible.
“Everything you see that's alive is doing this amazing thing,” Lyon points out. “If an airplane could do that, it would be bringing in its fuel and raw materials from the outside world while manufacturing not just its components but also the machines it needs to make those components and doing repairs, all while it's flying! What we do is nothing short of a miracle.”
Rowan Jacobsen is a journalist and author of several books, including Truffle Hound (Bloomsbury, 2021). He wrote about cracking the code that makes artificial proteins in Scientific American 's July 2021 issue. Follow Jacobsen on X (formerly Twitter) @rowanjacobsen
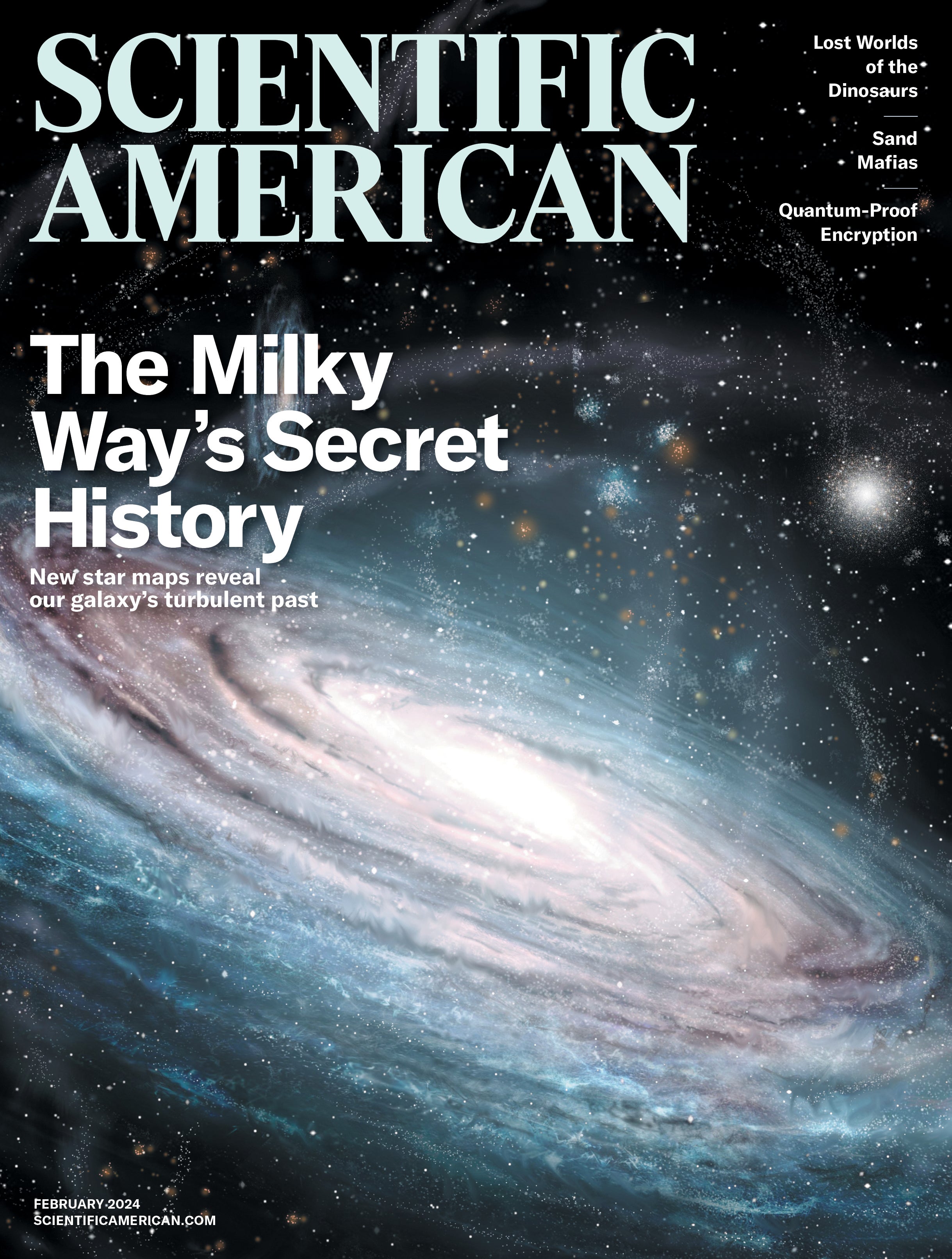
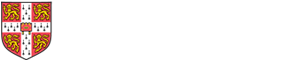
Study at Cambridge
About the university, research at cambridge.
- For Cambridge students
- For our researchers
- Business and enterprise
- Colleges and Departments
- Email and phone search
- Give to Cambridge
- Museums and collections
- Events and open days
- Fees and finance
- Postgraduate courses
- How to apply
- Fees and funding
- Postgraduate events
- International students
- Continuing education
- Executive and professional education
- Courses in education
- How the University and Colleges work
- Visiting the University
- Annual reports
- Equality and diversity
- A global university
- Public engagement
AI system self-organises to develop features of brains of complex organisms
- Research home
- About research overview
- Animal research overview
- Overseeing animal research overview
- The Animal Welfare and Ethical Review Body
- Animal welfare and ethics
- Report on the allegations and matters raised in the BUAV report
- What types of animal do we use? overview
- Guinea pigs
- Naked mole-rats
- Non-human primates (marmosets)
- Other birds
- Non-technical summaries
- Animal Welfare Policy
- Alternatives to animal use
- Further information
- Funding Agency Committee Members
- Research integrity
- Horizons magazine
- Strategic Initiatives & Networks
- Nobel Prize
- Interdisciplinary Research Centres
- Open access
- Energy sector partnerships
- Podcasts overview
- S2 ep1: What is the future?
- S2 ep2: What did the future look like in the past?
- S2 ep3: What is the future of wellbeing?
- S2 ep4 What would a more just future look like?
- Research impact

Cambridge scientists have shown that placing physical constraints on an artificially-intelligent system – in much the same way that the human brain has to develop and operate within physical and biological constraints – allows it to develop features of the brains of complex organisms in order to solve tasks.
Not only is the brain great at solving complex problems, it does so while using very little energy Jascha Achterberg
As neural systems such as the brain organise themselves and make connections, they have to balance competing demands. For example, energy and resources are needed to grow and sustain the network in physical space, while at the same time optimising the network for information processing. This trade-off shapes all brains within and across species, which may help explain why many brains converge on similar organisational solutions.
Jascha Achterberg, a Gates Scholar from the Medical Research Council Cognition and Brain Sciences Unit (MRC CBU) at the University of Cambridge said: “Not only is the brain great at solving complex problems, it does so while using very little energy. In our new work we show that considering the brain’s problem solving abilities alongside its goal of spending as few resources as possible can help us understand why brains look like they do.”
Co-lead author Dr Danyal Akarca, also from the MRC CBU, added: “This stems from a broad principle, which is that biological systems commonly evolve to make the most of what energetic resources they have available to them. The solutions they come to are often very elegant and reflect the trade-offs between various forces imposed on them.”
In a study published today in Nature Machine Intelligence , Achterberg, Akarca and colleagues created an artificial system intended to model a very simplified version of the brain and applied physical constraints. They found that their system went on to develop certain key characteristics and tactics similar to those found in human brains.
Instead of real neurons, the system used computational nodes. Neurons and nodes are similar in function, in that each takes an input, transforms it, and produces an output, and a single node or neuron might connect to multiple others, all inputting information to be computed.
In their system, however, the researchers applied a ‘physical’ constraint on the system. Each node was given a specific location in a virtual space, and the further away two nodes were, the more difficult it was for them to communicate. This is similar to how neurons in the human brain are organised.
The researchers gave the system a simple task to complete – in this case a simplified version of a maze navigation task typically given to animals such as rats and macaques when studying the brain, where it has to combine multiple pieces of information to decide on the shortest route to get to the end point.
One of the reasons the team chose this particular task is because to complete it, the system needs to maintain a number of elements – start location, end location and intermediate steps – and once it has learned to do the task reliably, it is possible to observe, at different moments in a trial, which nodes are important. For example, one particular cluster of nodes may encode the finish locations, while others encode the available routes, and it is possible to track which nodes are active at different stages of the task.
Initially, the system does not know how to complete the task and makes mistakes. But when it is given feedback it gradually learns to get better at the task. It learns by changing the strength of the connections between its nodes, similar to how the strength of connections between brain cells changes as we learn. The system then repeats the task over and over again, until eventually it learns to perform it correctly.
With their system, however, the physical constraint meant that the further away two nodes were, the more difficult it was to build a connection between the two nodes in response to the feedback. In the human brain, connections that span a large physical distance are expensive to form and maintain.
When the system was asked to perform the task under these constraints, it used some of the same tricks used by real human brains to solve the task. For example, to get around the constraints, the artificial systems started to develop hubs – highly connected nodes that act as conduits for passing information across the network.
More surprising, however, was that the response profiles of individual nodes themselves began to change: in other words, rather than having a system where each node codes for one particular property of the maze task, like the goal location or the next choice, nodes developed a flexible coding scheme. This means that at different moments in time nodes might be firing for a mix of the properties of the maze. For instance, the same node might be able to encode multiple locations of a maze, rather than needing specialised nodes for encoding specific locations. This is another feature seen in the brains of complex organisms.
Co-author Professor Duncan Astle, from Cambridge’s Department of Psychiatry, said: “This simple constraint – it’s harder to wire nodes that are far apart – forces artificial systems to produce some quite complicated characteristics. Interestingly, they are characteristics shared by biological systems like the human brain. I think that tells us something fundamental about why our brains are organised the way they are.”
Understanding the human brain
The team are hopeful that their AI system could begin to shed light on how these constraints, shape differences between people’s brains, and contribute to differences seen in those that experience cognitive or mental health difficulties.
Co-author Professor John Duncan from the MRC CBU said: “These artificial brains give us a way to understand the rich and bewildering data we see when the activity of real neurons is recorded in real brains.”
Achterberg added: “Artificial ‘brains’ allow us to ask questions that it would be impossible to look at in an actual biological system. We can train the system to perform tasks and then play around experimentally with the constraints we impose, to see if it begins to look more like the brains of particular individuals.”
Implications for designing future AI systems
The findings are likely to be of interest to the AI community, too, where they could allow for the development of more efficient systems, particularly in situations where there are likely to be physical constraints.
Dr Akarca said: “AI researchers are constantly trying to work out how to make complex, neural systems that can encode and perform in a flexible way that is efficient. To achieve this, we think that neurobiology will give us a lot of inspiration. For example, the overall wiring cost of the system we've created is much lower than you would find in a typical AI system.”
Many modern AI solutions involve using architectures that only superficially resemble a brain. The researchers say their works shows that the type of problem the AI is solving will influence which architecture is the most powerful to use.
Achterberg said: “If you want to build an artificially-intelligent system that solves similar problems to humans, then ultimately the system will end up looking much closer to an actual brain than systems running on large compute cluster that specialise in very different tasks to those carried out by humans. The architecture and structure we see in our artificial ‘brain’ is there because it is beneficial for handling the specific brain-like challenges it faces.”
This means that robots that have to process a large amount of constantly changing information with finite energetic resources could benefit from having brain structures not dissimilar to ours.
Achterberg added: “Brains of robots that are deployed in the real physical world are probably going to look more like our brains because they might face the same challenges as us. They need to constantly process new information coming in through their sensors while controlling their bodies to move through space towards a goal. Many systems will need to run all their computations with a limited supply of electric energy and so, to balance these energetic constraints with the amount of information it needs to process, it will probably need a brain structure similar to ours.”
The research was funded by the Medical Research Council, Gates Cambridge, the James S McDonnell Foundation, Templeton World Charity Foundation and Google DeepMind.
Reference Achterberg, J & Akarca, D et al. Spatially embedded recurrent neural networks reveal widespread links between structural and functional neuroscience findings. Nature Machine Intelligence; 20 Nov 2023; DOI: 10.1038/s42256-023-00748-9

Read this next
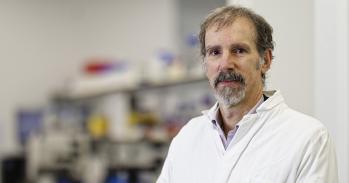
AI speeds up drug design for Parkinson’s ten-fold
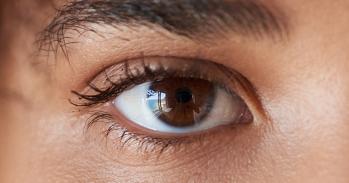
Artificial Intelligence beats doctors in accurately assessing eye problems
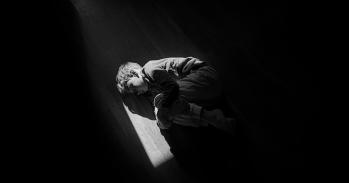
Study unpicks why childhood maltreatment continues to impact on mental and physical health into adulthood
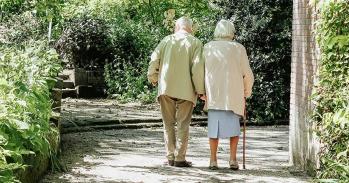
UK-wide trials to begin on blood tests for diagnosing dementia
Media enquiries.
Graphic representing an artificially intelligent brain
Credit: DeltaWorks

Search research
Sign up to receive our weekly research email.
Our selection of the week's biggest Cambridge research news sent directly to your inbox. Enter your email address, confirm you're happy to receive our emails and then select 'Subscribe'.
I wish to receive a weekly Cambridge research news summary by email.
The University of Cambridge will use your email address to send you our weekly research news email. We are committed to protecting your personal information and being transparent about what information we hold. Please read our email privacy notice for details.
- Spotlight on neuroscience
- Artificial intelligence
- Duncan Astle
- Jascha Achterberg
- Danyal Akarca
- John Duncan
- School of Clinical Medicine
- MRC Cognition and Brain Sciences Unit
- Gates Cambridge Trust
- Robinson College
- Cambridge Neuroscience
Related organisations
- Medical Research Council
- James S McDonnell Foundation
- Templeton World Charity Foundation
Connect with us
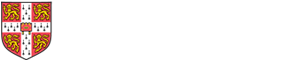
© 2024 University of Cambridge
- Contact the University
- Accessibility statement
- Freedom of information
- Privacy policy and cookies
- Statement on Modern Slavery
- Terms and conditions
- University A-Z
- Undergraduate
- Postgraduate
- Cambridge University Press & Assessment
- Research news
- About research at Cambridge
- Spotlight on...
- Reference Manager
- Simple TEXT file
People also looked at
Original research article, how organisms come to know the world: fundamental limits on artificial general intelligence.
- 1 Department of Computer Science and Engineering, Campus of Cesena, Università di Bologna, Bologna, Italy
- 2 European Centre for Living Technology, Venezia, Italy
- 3 Complexity Science Hub (CSH) Vienna, Vienna, Austria
- 4 Institute for Systems Biology, Seattle, WA, United States
Artificial intelligence has made tremendous advances since its inception about seventy years ago. Self-driving cars, programs beating experts at complex games, and smart robots capable of assisting people that need care are just some among the successful examples of machine intelligence. This kind of progress might entice us to envision a society populated by autonomous robots capable of performing the same tasks humans do in the near future. This prospect seems limited only by the power and complexity of current computational devices, which is improving fast. However, there are several significant obstacles on this path. General intelligence involves situational reasoning, taking perspectives, choosing goals, and an ability to deal with ambiguous information. We observe that all of these characteristics are connected to the ability of identifying and exploiting new affordances—opportunities (or impediments) on the path of an agent to achieve its goals. A general example of an affordance is the use of an object in the hands of an agent. We show that it is impossible to predefine a list of such uses. Therefore, they cannot be treated algorithmically. This means that “AI agents” and organisms differ in their ability to leverage new affordances. Only organisms can do this. This implies that true AGI is not achievable in the current algorithmic frame of AI research. It also has important consequences for the theory of evolution. We argue that organismic agency is strictly required for truly open-ended evolution through radical emergence. We discuss the diverse ramifications of this argument, not only in AI research and evolution, but also for the philosophy of science.
1. Introduction
Since the founding Dartmouth Summer Research Project in 1956 ( McCarthy et al., 1955 ), the field of artificial intelligence (AI) has attained many impressive achievements. The potential of automated reasoning, problem solving, and machine learning has been unleashed through a wealth of different algorithms, methods, and tools ( Russell and Norvig, 2021 ). Not only do AI systems accomplish to perform intricate activities, e. g. , playing games ( Silver et al., 2016 ), and to plan complex tasks ( LaValle, 2006 ), but most current apps and technological devices are equipped with some AI component. The impressive recent achievements of machine learning ( Domingos, 2015 ) have greatly extended the domains in which AI can be applied, from machine translation to automatic speech recognition. AI is becoming ubiquitous in our lives. In addition, AI methods are able to produce some kinds of creative artworks, such as paintings ( Hong and Curran, 2019 ), and music ( Briot and Pachet, 2020 ); moreover, GPT-3, the latest version of a deep learning system able to generate texts characterized by surprising writing abilities, has recently been released ( Brown et al., 2020 ) surrounded by some clamor ( Chalmers, 2020 ; Marcus and Davis, 2020 ).
These are undoubtedly outstanding accomplishments. However, each individual success remains limited to quite narrowly defined domains. Most of today's AI systems are target-specific: an AI program capable of automatically planning tasks, for example, is not usually capable of recognizing faces in photographs. Such specialization is, in fact, one of the main elements contributing to the success of these systems. However, the foundational dream of AI—featured in a large variety of fantastic works in science-fiction—is to create a system, maybe a robot, that incorporates a wide range of adaptive abilities and skills. Hence, the quest for Artificial General Intelligence (AGI) , computational systems able to connect, integrate, and coordinate these various capabilities. In fact, true general intelligence can be defined as the ability of combining “analytic, creative, and practical intelligence” ( Roitblat, 2020 , page 278). It is acknowledged to be a distinguishing property of “natural intelligence,” for example, the kind of intelligence that governs some of the behavior of humans as well as other mammalian and bird species.
If one considers the human brain as a computer—and by this we mean some sort of computational device equivalent to a universal Turing machine—then the achievement of AGI might simply rely on reaching a sufficient level of intricacy through the combination of different task-solving capabilities in AI systems. This seems eminently feasible—a mere extrapolation of current approaches in the context of rapidly increasing computing power—even though it requires not only the combinatorial complexification of the AI algorithms themselves, but also of the methods used to train them. In fact, many commentators predict that AGI is just around the corner, often admonishing us about the great (even existential) potentials and risks associated with this technological development (see, for example, Vinge, 1993 ; Kurzweil, 2005 ; Yudkowsky, 2008 ; Eden et al., 2013 ; Bostrom, 2014 ; Shanahan, 2015 ; Chalmers, 2016 ; Müller and Bostrom, 2016 ; Ord, 2020 ).
However, a number of serious problems arise when considering the higher-level integration of task-solving capabilities. All of these problems are massively confounded by the fact that real-world situations often involve information that is irrelevant, incomplete, ambiguous, and/or contradictory. First, there is the formal problem of choosing an appropriate metric for success (a cost or evaluation function) according to context and the task at hand. Second, there is the problem of identifying worthwhile tasks and relevant contextual features from an abundance of (mostly irrelevant) alternatives. Finally, there is the problem of defining what is worthwhile in the first place. Obviously, a truly general AI would have to be able to identify and refine its goals autonomously, without human intervention. In a quite literal sense, it would have to know what it wants, which presupposes that it must be capable of wanting something in the first place.
The problem of machine wanting has often been linked by philosophers to arguments about cognition, the existence of subjective mental states and, ultimately, to questions about consciousness. A well-known example is John Searle's work on minds and AI (see, for example, Searle, 1980 , 1992 ). Other philosophers have attempted to reduce machine wanting to cybernetic goal-seeking feedback (e. g., McShea, 2012 , 2013 , 2016 ). Here, we take the middle ground and argue that the problem is rooted in the concept of organismic agency, or bio-agency ( Moreno and Etxeberria, 2005 ; Barandiaran et al., 2009 ; Skewes and Hooker, 2009 ; Arnellos et al., 2010 ; Campbell, 2010 ; Arnellos and Moreno, 2015 ; Moreno and Mossio, 2015 ; Meincke, 2018 ). We show that the term “agency” refers to radically different notions in organismic biology and AI research.
The organism's ability to act is grounded in its functional organization, which grants it a certain autonomy (a “freedom from immediacy”) ( Gold and Shadlen, 2007 ). An organism not only passively reacts to environmental inputs. It can initiate actions according to internal goals, which it seeks to attain by leveraging opportunities and avoiding obstacles it encounters in its umwelt , that is, the world as perceived by this particular organism ( Uexküll von, 2010 ; Walsh, 2015 ). These opportunities and obstacles are affordances , relations between the living agential system and its umwelt that are relevant to the attainment of its goals ( Gibson, 1966 ). Organismic agency enables a constructive dialectic between an organism's goals, its repertoire of actions, and its affordances, which all presuppose and generate each other in a process of constant emergent co-evolution ( Walsh, 2015 ).
Our argument starts from the simple observation that the defining properties of natural systems with general intelligence (such as organisms) require them to take advantage of affordances under constraints given by their particular motivations, abilities, resources, and environments. In more colloquial terms, general intelligences need to be able to invent, to improvise, to jury-rig problems that are relevant to their goals. However, AI agents (unlike biological ones) are defined as sophisticated algorithms that process information from percepts (inputs) obtained through sensors to actions (outputs) implemented by effectors ( Russell and Norvig, 2021 ). We elaborate on the relation between affordances and algorithms—defined as computational processes that can run on universal Turing machines—ultimately arriving at the conclusion that identifying and leveraging affordances goes beyond algorithmic computation. This leads to two profound implications. First, while it may still be possible to achieve powerful AI systems endowed with quite impressive and general abilities, AGI cannot be fully attained in computational systems that are equivalent to universal Turing machines. This limitation holds for both non-embodied and embodied Turing machines, such as robots. Second, based on the fact that only true agents can harvest the power of affordances, we conclude that only biological agents are capable of generating truly open-ended evolutionary dynamics, implying that algorithmic attempts at creating such dynamics in the field of artificial life (aLife) are doomed to fail.
Our argument proceeds as follows: In Section 2, we provide a target definition for AGI and describe some major obstacles on the way to achieve it. In Section 3, we define and contrast the notion of an agent in organismic biology and AI research. Section 4 introduces the crucial role that affordances play in AGI, while Section 5 elucidates the limitations of algorithmic agents when it comes to identifying and leveraging affordances. In Section 6, we show that our argument also applies to embodied AI agents such as robots. Section 7 presents a number of possible objections to our argument. Section 8 discusses the necessity of bio-agency for open-ended evolution. Finally, Section 9 concludes the discussion with a few remarks on the scientific and societal implications of our argument.
2. Obstacles Toward Artificial General Intelligence
The proposal for the Dartmouth Summer Research Project begins with an ambitious statement: “An attempt will be made to find how to make machines use language, form abstractions and concepts, solve kinds of problems now reserved for humans, and improve themselves” ( McCarthy et al., 1955 ). Over the 66 years that have passed since this was written, the field of AI research has made enormous progress, and specialized AI systems have been developed that find application across almost all aspects of human life today (see Introduction). However, the original goal of devising a system capable of integrating all the various capabilities required for “true machine intelligence” has not yet been reached.
According to Roitblat (2020) , the defining characteristics of general intelligence are:
• reasoning and problem-solving,
• learning,
• inference-making,
• using common-sense knowledge,
• autonomously defining and adjusting goals,
• dealing with ambiguity and ill-defined situations, and
• creating new representations of the knowledge acquired.
Some of these capabilities are easier to formalize than others. Automated reasoning, problem-solving, learning, and inference-making, for example, can be grounded in the principles of formal logic, and are reaching impressive levels of sophistication in contemporary deep-learning approaches ( Russell and Norvig, 2021 ). In contrast, the complete algorithmic formalization of the other items on the list remains elusive. We will discuss the problem of autonomously defining goals shortly. The three remaining characteristics are not only hard to implement algorithmically, but are difficult to define precisely in the first place. This vagueness is of a semantic and situational nature: it concerns the meaning of concepts to an agent, the knower, in their particular circumstances.
For example, we have no widely agreed-upon definition of what “common-sense knowledge” is. In fact, it is very likely that there is no generalizable definition of the term, as “common sense” represents a kind of perspectival knowing that depends radically on context. It represents a way of reacting to an everyday problem that is shared by many (or all) people at a given location and time. It is thus an intrinsically situational and normative concept, and its meaning can shift drastically across different societal and historical contexts. What it would mean for a computer to have “common sense” remains unclear: does it have to act in a way that humans of its time and location would consider commonsensical? Or does it have to develop its own kind of computer-specific, algorithmic “common sense”? What would that even mean?
Exactly the same problem affects the ability of AI algorithms to create new representations of knowledge. Those representations must not only correspond to some state of affairs in the world, but must also be relatable, understandable, and useful to some kind of knowing agent. They must represent something to someone . But who? Is the task of AGI to generate representations for human understanding? If not, what kind of sense does it make for a purely algorithmic system to generate representations of knowledge? It does not need them, since it does not use visualizations or metaphors for reasoning and understanding. Again, the semantic nature of the problem makes it difficult to discuss within the purely syntactic world of algorithmic AIs.
Since they cannot employ situational knowledge, and since they cannot represent and reason metaphorically, AI systems largely fail at dealing with and exploiting ambiguities ( Byers, 2010 ). These limitations have been identified and formulated as the frame problem more than fifty years ago by Dreyfus (1965) (see also Dreyfus, 1992 ). Today, they are still with us as major obstacles for achieving AGI. What they have in common is an inability of algorithmic systems to reckon with the kind of uncertainty, or even paradox, that arises from context-dependent or ill-defined concepts. In contrast, the tension created by such unresolved states of knowing is often a crucial ingredient for human creativity and invention (see, for example, Scharmer and Senge, 2016 ).
Let us argue the case with an illustrative example. The ability to exploit ambiguities plays a role in almost any human cognitive activity. It can turn up in the most unexpected places, for instance, in one of the most rule-based human activities—an activity that we might think should be easy to formalize. As Byers beautifully observes about creativity in mathematics, “[a]mbiguity, which implies the existence of multiple, conflicting frames of reference, is the environment that gives rise to new mathematical ideas. The creativity of mathematics does not come out of algorithmic thought” ( Byers, 2010 , page 23). In situated problem-solving, ambiguity is oftentimes the cornerstone of a solution process. Let us consider the mathematical riddle in Figure 1 : if we break ambiguities by taking a purely formalized algebraic perspective, the solution we find is hardly simple. 1 Yet, if we change perspective and we observe the graphical shape of the digits, we can easily note that what is summed up are the closed loops present in the numeric symbols. It turns out that the puzzle, as it is formulated, requires the ability to observe from different perspectives, to dynamically shift perceptive and cognitive frames, mixing both graphical and algebraic approaches for a simple solution.

Figure 1 . A riddle found by one of the authors in a paper left in the coffee room of a department of mathematics.
Following Byers, we observe that even a strongly formalized human activity—the process of discovery in mathematics—is not entirely captured by an algorithmic search. A better metaphor would be an erratic walk across dark rooms. As Andrew Wiles describes his journey to the proof of the Fermat conjecture, 2 the solution process starts from a dark room where we “stumble around bumping into the furniture;” suddenly we find the light switch and, in the illuminated room, we “can see were we were”—an insight! Then, we move to an adjacent dark room and continue this process finding successive “light switches” into further dark rooms until the problem, at last, is solved. Each step from one room to the other is an insight , not a deduction and not an induction. The implication is fundamental: the mathematician comes to know a new world via an insight. The insight itself is not algorithmic. It is an act of semantic meaning-making . Roger Penrose makes the same point in the Emperor's New Mind ( Penrose, 1989 ).
Human creativity, in all kinds of contexts, seems to require frame-switching between metaphorical or formal representations, alongside our capabilities of dealing with contradictions and ambiguities. These are not only hallmarks of human creative processes, but should also characterize AGI systems. As we will see, these abilities crucially rely on affordances ( Gibson, 1966 ). Therefore, we must ask whether universal Turing machines can identify and exploit affordances. The initial step toward an answer to this question lies in the recognition that affordances arise from interactions between an agent and its umwelt. Therefore, we must first understand what an agent is, and how the concept of an “agent” is defined and used in biology and in AI research.
3. Bio-Agency: Contrasting Organisms to AI Agents
So far, we have avoided the question how an AGI could choose and refine its own goals ( Roitblat, 2020 ). This problem is distinct, but still tightly related to the issues of ambiguity and representation discussed in the previous section. Selecting goals has two aspects. The first is that one must motivate the choice of a goal. One must want to reach some goal to have a goal at all, and one must have needs to want something. The other aspect is to prioritize some particular goal over a set of alternatives, according to the salience and the alignment of the chosen goal with one's own needs and capabilities in a given context.
Choosing a goal, of course, presupposes a certain autonomy, i. e ., the ability to make a “choice” ( Moreno and Mossio, 2015 ). Here, we must emphasize again that our use of the term “choice” does not imply consciousness, awareness, mental states, or even cognition, which we take to involve at least some primitive kind of nervous system ( Barandiaran and Moreno, 2008 ). It simply amounts to a system which is capable of selecting from a more or less diversified repertoire of alternative dynamic behaviors (“actions”) that are at its disposal in a given situation ( Walsh, 2015 ). All forms of life—from simple bacteria to sophisticated humans—have this capability. The most central distinction to be made here is that the selection of a specific behavior is not purely reactive, not entirely determined by environmental conditions, but (at least partially) originates from and depends on the internal organization of the system making the selection. This implies some basic kind of agency ( Moreno and Mossio, 2015 ). In its broadest sense, “agency” denotes the ability of a system to initiate actions from within its own boundaries, causing effects that emanate from its own internal dynamics.
Agency requires a certain type of functional organization. More specifically, it requires organizational closure ( Piaget, 1967 ; Moreno and Mossio, 2015 ), which leads to autopoietic ( i. e. , self-making, self-maintaining, and self-repairing) capabilities ( Maturana and Varela, 1973 , 1980 ). It also leads to self-determination through self-constraint: by maintaining organizational closure, an organism is constantly providing the conditions for its own continued existence ( Bickhard, 2000 ; Mossio and Bich, 2017 ). This results in the most basic, metabolic, form of autonomy ( Moreno and Mossio, 2015 ). A minimal autonomous agent is a physically open, far-from-equilibrium, thermodynamic system capable of self-reproduction and self-determination.
Organisms, as autonomous agents, are Kantian wholes, i. e ., organized beings with the property that the parts exist for and by means of the whole ( Kant, 1892 ; Kauffman, 2000 , 2020 ). “Whole” indicates that organizational closure is a systems-level property. In physical terms, it can be formulated as a closure of constraints ( Montévil and Mossio, 2015 ; Moreno and Mossio, 2015 ; Mossio et al., 2016 ). Constraints change the dynamics of the underlying processes without being altered themselves (at least not at the same time scale). Examples of constraints in organisms include enzymes, which catalyze biochemical reactions without being altered in the process, or the vascular system in vertebrates, which regulates levels of nutrients, hormones, and oxygen in different parts of the body without changing itself at the time scale of those physiological processes ( Montévil and Mossio, 2015 ).
It is important to note that constraint closure does not imply a fixed (static) network of processes and constraints. Instead, organizational continuity is maintained if the current closed organization of a system causally derives from previous instantiations of organizational closure, that is, its particular organized state at this moment in time is dynamically presupposed by its earlier organized states ( Bickhard, 2000 ; DiFrisco and Mossio, 2020 ). Each successive state can (and indeed must) differ in their detailed physical structure from the current state. To be a Kantian whole, an autonomous system must perform at least one work-constraint cycle: it must perform physical work to continuously (re)constitute closure through new as well as recurring constraints ( Kauffman, 2000 , 2003 ; Kauffman and Clayton, 2006 ). Through each such cycle, a particular set of constraints is propagated, selected from a larger repertoire of possible constraints that all realize closure. In this way, the system's internal dynamics kinetically “lift” a set of mutually constituting processes from the totality of possible dynamics. This is how organizational closure leads to autopoiesis, basic autonomy, and self-determination by self-constraint: the present structure of the network of interacting processes that get “lifted” is (at least to some degree) the product of the previous unfolding of the organized network. In this way, organization maintains and propagates itself.
However, one key ingredient is still missing for an agent that actively chooses its own goals. The basic autonomous system we described above can maintain (and even repair) itself, but it cannot adapt to its circumstances—it cannot react adequately to influences from its environment. This adaptive capability is crucial for prioritizing and refining goals according to a given situation. The organism can gain some autonomy over its interactions with the environment if it is capable of regulating its own boundaries. These boundaries are required for autopoiesis, and thus must be part of the set of components that are maintained by closure ( Maturana and Varela, 1980 ). Once boundary processes and constraints have been integrated into the closure of constraints, the organism has attained a new level of autonomy: interactive autonomy ( Moreno and Mossio, 2015 ). It has now become a fully-fledged organismal agent , able to perceive its environment and to select from a repertoire of alternative actions when responding to environmental circumstances based on its internal organization. Expressed a bit more colloquially, making this selection requires being able to perceive the world and to evaluate “what's good or bad for me,” in order to act accordingly. Here, the transition from matter to mattering takes place.
Interactive autonomy provides a naturalistic (and completely scientific) account of the kind of bio-agency (and the particular kind of goal-directedness or teleology that is associated with it, Mossio and Bich, 2017 ), which grounds our examination of how organisms can identify and exploit affordances in their umwelt. But before we get to this, let us contrast the complex picture of an organismal agent as a Kantian whole with the much simpler concept of an agent in AI research. In the context of AI, “[a]n agent is anything that can be viewed as perceiving its environment through sensors and acting upon that environment through effectors ” ( Russell and Norvig, 2021 , original emphasis). In other words, an AI agent is an input–output processing device. Since the point of AI is to do “a good job of acting on the environment” ( Russell and Norvig, 2021 ), the internal processing can be quite complicated, depending on the task at hand. This very broad definition of an AI agent in fact includes organismal agents, since it does not specify the kind of processes that mediate between perception and action. However, although not always explicitly stated, it is generally assumed that input-output processing is performed by some sort of algorithm that can be implemented on a universal Turing machine. The problem is that such algorithmic systems have no freedom from immediacy, since all their outputs are determined entirely—even though often in intricate and probabilistic ways—by the inputs of the system. There are no actions that emanate from the historicity of internal organization. There is, therefore, no agency at all in an AI “agent.” What that means and why it matters for AGI and evolution will be the subject of the following sections.
4. The Key Role of Affordances
Having outlined a suitable naturalistic account of bio-agency, we can now revisit the issue of identifying and exploiting affordances in the umwelt, or perceived environment, of an organism. The concept of an affordance was first proposed by Gibson (1966) in the context of ecological psychology. It was later adopted to diverse fields of investigation such as biosemiotics ( Campbell et al., 2019 ) and robotics ( Jamone et al., 2016 ). “Affordances” refer to what the environment offers to an agent (in the organismic sense defined above), for “good or ill.” They can be manifested as opportunities or obstacles on our path to attain a goal. A recent philosophical account emphasizes the relation between the agent and its perceived environment (its umwelt), stating that affordances guide and constrain the behavior of organisms, precluding or allowing them to perform certain actions, showing them what they can and cannot do ( Heras-Escribano, 2019 , p. 3). A step, for instance, affords us the action of climbing; a locked door prevents us from entering. Affordances fill our world with meaning: organisms do not live in an inert environment, but “are surrounded by promises and threats” ( Heras-Escribano, 2019 , p. 3).
The dialectic mutual relationship between goals, actions, and affordances is of crucial importance here ( Walsh, 2015 ). Affordances, as we have seen, require an agent with goals. Those goals motivate the agent to act. The agent first chooses which goal to pursue. It then selects an action from its repertoire (see Section 3) that it anticipates to be conducive to the attainment of the goal. This action, in turn, may alter the way the organism perceives its environment, or it may alter aspects of the environment itself, which leads to an altered set of affordances present in its umwelt. This may incite the agent to choose an alternative course of action, or even to reconsider its goals. In addition, the agent can learn to perform new actions or develop new goals along the way. This results in a constructive co-emergent dynamic in which sets of goals, actions, and affordances continuously generate and collapse each other as the world of the agent keeps entering into the next space of possibilities, its next adjacent possible ( Kauffman, 2000 ). Through this co-emergent dialectic, new goals, opportunities, and ways of acting constantly arise. Since the universe is vastly non-ergodic, each moment in time provides its own unique set of opportunities and obstacles, affording new kinds of goals and actions ( Kauffman, 2000 ). In this way, true novelty enters into the world through radical emergence —the generation, over time, of opportunities and rules of engagement and interaction that did not exist at any previous time in the history of the universe.
A notable example of such a co-emergent process in a human context is jury-rigging : given a leak in the ceiling, we cobble together a cork wrapped in a wax-soaked rag, stuff it into the hole in the ceiling, and hold it in place with duct tape ( Kauffman, 2019 ). In general, solving a problem through jury-rigging requires several steps and involves different objects and actions, which articulate together toward a solution of the problem, mostly without any predetermined plan. Importantly, jury-rigging uses only specific subsets of the totality of causal properties of each object involved. Often, these properties do not coincide with previously known functional features of the object. Consider a tool, like a screwdriver, as an example. Its original purpose is to tighten screws. But it can also be used to open a can of paint, wedge a door open, scrape putty off the window, to stab or poke someone (please don't), or (should you feel so inclined) to pick your nose with it. What is important to note here is that any physical object has an indefinite number of alternative uses in the hands of an agent ( Kauffman, 1976 ). This does not mean that its uses are infinite —even if they might be—but rather that they cannot be known (and thus prestated) in advance.
Ambiguity and perspective-taking also play a fundamental role in jury-rigging, as the goal of the task is to find suitable novel causal properties of the available objects to solve the problem at hand. The same happens in an inverse process, where we observe an artifact (or an organism Kauffman, 2019 ), and we aim at providing an explanation by articulating its parts, along with the particular function they carry out. For example, if we are asked what the use of an automobile is, we would probably answer that it is a vehicle equipped with an engine block, wheels, and other parts, whose diverse causal features can be articulated together to function as a locomotion and transportation system. This answer resolves most ambiguities concerning the automobile and its parts by providing a coherent frame in which the parts of the artifact are given a specific function, aimed at explaining its use as a locomotion and transportation system. In contrast, if one supposes that the purpose of an automobile is to fry eggs, one would partition the system into different sets of parts that articulate together in a distinct way such that eggs can be fried on the hot engine block. In short, for the inverse process with regard to artifacts (or organisms) what we “see it as doing” drives us to decompose the system into parts in different ways ( Kauffman, 1976 ). Each such decomposition identifies precisely that subset of the causal properties of the identified parts that articulate together to account for and explain “what the system is doing” according to our current frame. It is critical to note that there is no universal or unique decomposition, since the way to decompose the system depends on its use and context (see also Wimsatt, 2007 ).
To close the loop of our argument, we note that the prospective uses of an object (and hence the decomposition we choose to analyze it) depend on the goals of the agent using it, which, in turn, depend on the agent's repertoire of actions and the affordances available to it, which change constantly and irreversibly over time. It is exactly because all of these are constantly evolving through their co-emergent dialectic interactions that the number of uses of an object remains indefinite and, in fact, unknowable ( Kauffman, 2019 ). Moreover, and this is important: there is no deductive relation between the uses of an object. Take, for example, an engine block, designed to be a propulsive device in a car. It can also serve as the chassis of a tractor. Furthermore, one can use it as a bizarre (but effective) paper weight, its cylinder bores can host bottles of wine, or it can be used to crack open coconuts on one of its corners. In general, we cannot know the number of possible uses of an engine block, and we cannot deduce one use from another: the use as a paper weight abstracts from details that can conversely be necessary for it to be used to crack open coconuts. As Robert Rosen put it, complex systems invariably retain hidden properties, and their manipulation can always result in unintended consequences ( Rosen, 2012 ). Even worse, we have seen that the relation between different uses of a thing is merely nominal , as there is no kind of ordering that makes it possible to relate them in a more structured way ( Kauffman, 2019 ; Kauffman and Roli, 2021b ).
This brings us to a cornerstone of our argument: when jury-rigging, it is impossible to compose any sort of well-defined list of the possible uses of the objects to be used. By analogy, it is impossible to list all possible goals, actions, or affordances of an organismic agent in advance. In other words, Kantian wholes can not only identify and exploit affordances, but they constantly generate new opportunities for themselves de novo . Our next question is: can algorithmic systems such as AI “agents” do this?
5. The Bounded Rationality of Algorithms
In the introduction, we have defined an algorithm as a computational process that can run on a universal Turing machine. This definition considers algorithms in a broad sense, including computational processes that do not halt. All algorithms operate deductively ( Kripke, 2013 ). When implementing an algorithm as a computer program by means of some kind of formal language (including those based on recursive functional programming paradigms), we must introduce specific data and code structures, their properties and interactions, as well as the set of operations we are allowed to perform on them, in order to represent the objects and relations that are relevant for our computation. In other words, we must provide a precisely defined ontology on which the program can operate deductively, e. g. , by drawing inferences or by ordering tasks for solving a given problem. In an algorithmic framework, novelty can only be represented combinatorially: it manifests as new combinations, mergers, and relations between objects in a (potentially vast, but predefined) space of possibilities. This means that an algorithm cannot discover or generate truly novel properties or relations that were not (at least implicitly) considered in its original ontology. Therefore, an algorithm operating in a deductive manner cannot jury-rig, since it cannot find new causal properties of an object that were not already inherent in its logical premises.
To illustrate this central point, let us consider automated planning : a planning program is given an initial state and a predefined goal, and its task is to find a feasible—and ideally optimal—sequence of actions to reach the goal. What makes this approach successful is the possibility of describing the objects involved in the task in terms of their properties, and of representing actions in terms of the effects they produce on the world delimited by the ontology of the program, plus the requirements that need to be satisfied for their application. For the planner to work properly, there must be deductive relations among the different uses of an object, which are exploited by the inference engine to define an evaluation function that allows it to arrive at a solution. The problem with the planner is that, in general, there is no deductive relation between the possible uses of an object (see Section 4). From the use of an engine block as a paper weight, the algorithm cannot deduce its use as a method to crack open coconuts. It can, of course, find the latter use if it can be deduced, i. e ., if there are: (i) a definitive list of properties, including the fact that the engine block has rigid and sharp corners, (ii) a rule stating that one can break objects in the class of “breakable things” by hitting them against objects characterized by rigid and sharp corners, and (iii) a fact stating that coconuts are breakable.
The universe of possibilities in a computer program—however, broadly construed—is like a world of LEGO TM bricks: components with predefined properties and compositional relations can generate a huge space of possible combinations, even unbounded if more bricks can always be supplemented. However, if we add scotch tape, which makes it possible to assemble bricks without being constrained by their compositional mechanism, and a cutter, which enables us to cut the bricks into smaller pieces of any shape, then rules and properties are no longer predefined. We can no longer prestate a well-defined list of components, with associated properties and relations. We now have a universe of indefinite possibilities, and we are no longer trapped inside the formal frame of algorithms. Formalization has reached its limits . What constitutes a meaningful compositional relation becomes a semantic question, depending on our particular circumstances and the whims of our creative mind. Our possibilities may not be infinite, but they become impossible to define in advance. And because we can no longer list them, we can no longer treat them in a purely algorithmic way. This is how human creativity transcends the merely combinatorial innovative capacities of any AI we can build today. Algorithms cannot take or shift perspective and that is why they cannot leverage ambiguity for innovation in the way an organismic agent can. Algorithms cannot jury-rig .
At the root of this limitation is the fact that algorithms cannot want anything. To want something implies having goals that matter to us. We have argued in Section 3, that only organismic agents (but not algorithmic AI “agents”) can have goals, because of their being Kantian wholes with autopoietic organization and closure of constraints. Therefore, nothing matters to an algorithm. But without mattering or goals, an algorithm has no means to identify affordances (in fact, it has no affordances), unless they are already formally predefined in its ontology, or can be derived in some logical way from predefined elements of that ontology. Thus, the algorithm cannot generate meaning where there was none before. It cannot engage in the process of semiosis ( Peirce, 1934 , p. 488). For us to make sense of the world, we must take a perspective: we must see the world from a specific point of view, contingent on our nature as fragile, limited, mortal beings which circumscribes our particular goals, abilities, and affordances. This is how organismic agents generate new frames in which to formalize posssibilities. This is how we tell what is relevant to us from what is not. Algorithms cannot do this, since they have no point of view, and require a predefined formal frame to operate deductively. To them, everything and nothing is relevant at the same time.
Now, we must draw our attention to an issue that is often neglected when discussing the nature of general intelligence: for a long time, we have believed that coming to know the world is a matter of induction, deduction, and abduction (see, for example, Hartshorne and Weiss, 1958 ; Mill, 1963 ; Ladyman, 2001 ; Hume, 2003 ; Okasha, 2016 ; Kennedy and Thornberg, 2018 ). Here, we show that this is not enough.
Consider induction , proceeding from a finite set of examples to an hypothesis of a universal. We observe many black ravens and formulate the hypothesis that “all ravens are black.” Observe that the relevant variables and properties are already prestated, namely “ravens” and “black.” Induction is over already identified features of the world and, by itself, does not identify new categories. In induction, there is an imputation of a property of the world (black) with respect to things we have already identified (ravens). There is however no insight with respect to new features of the world ( cf . Section 2). Let us pause to think about this: induction by itself cannot reveal novel features of the world—features that are not already in our ontology.
This is even more evident for deduction , which proceeds from prestated universal categories to the specific. “All men are mortal, Socrates is a man, therefore Socrates is a mortal.” All theorems and proofs in mathematics have this deductive structure. However, neither induction nor deduction by themselves can reveal novel features of the world not already in our ontology.
Finally, we come to abduction , which aims at providing an explanation of an observation by asserting an already known precondition that is likely to have this observation as a consequence. For example, if we identify an automobile as a means of locomotion and transportation, and had decomposed it into parts that articulate together to support its function as a means of locomotion and transportation, we are then able to explain its failure to function in this sense by a failure of one of its now defined parts. If the car does not turn on, we can suppose the battery is dead. Abduction is differential diagnosis from a prestated set of conditions and possibilities that articulate to carry out what we “see the system as doing or being.” But there is no unique decomposition. The number of decompositions is indefinite. Therefore, when implemented in a computer program, this kind of reasoning cannot reveal novel features of the world not already in the ontology of the program.
To summarize: with respect to coming to know the world, once we have carved the world into a finite set of categories, we can no longer see the world beyond those categories . In other words, new meanings—along with their symbolic grounding in real objects—are outside of the predefined ontology of an agential system. The same limitation also holds for probabilistic forms of inference, involving, e. g. , Bayesian nets (see Gelman et al., 2013 ). Consider the use of an engine block as a paper weight, and a Bayesian algorithm updating to improve engine blocks with respect to functioning as a paper weight. No such updating will reveal that engine blocks can also be used to crack open coconuts. The priors for such an innovation could not be deduced, even in principle. Similarly, Markov blankets (see, for example, Hipólito et al., 2021 ) are restricted to pre-existing categories.
Organisms come to know new features of the world by semiosis—a process which involves semantic meaning-making of the kind described above, not just formal (syntactic) reasoning through deduction, induction, or abduction. This is true of mathematicians. It is also true of Caledonian crows who solve problems of astonishing complexity, requiring sophisticated multi-step jury-rigging ( Taylor et al., 2010 ). Chimpanzees learning to use tools have the same capacity to improvise ( Köhler, 2013 ). Simpler organisms—down to bacteria—must have it too, although probably in a much more limited sense. After all, they are at the basis of an evolutionary process toward more complex behavior, which presupposes the identification and exploitation of new opportunities. Our human ontology has evolved into a much more complex state than that of a primitive unicellular organism. In general, all organisms act in alignment with their goals, capabilities, and affordances (see Section 4), and their agential behavior can undergo variation and selection. A useful action—exploiting a novel affordance—can be captured by heritable variation (at the genetic, epigenetic, behavioral, or cultural level) and thus passed on across generations. This “coming to know the world” is what makes the evolutionary expansion of our ontologies possible. It goes beyond induction, deduction, and abduction. Organisms can do it, but universal Turing machines cannot.
In conclusion, the rationality of algorithms is bounded by their ontology. However vast this ontology may be, algorithms cannot transcend their predefined limitations, while organisms can. This leads us to our central conclusion, which is both radical and profound: not all possible behaviors of an organismic agent can be formalized and performed by an algorithm—not all organismic behaviors are Turing-computable. Therefore, organisms are not Turing machines. It also means that true AGI cannot be achieved in an algorithmic frame , since AI “agents” cannot choose and define their own goals, and hence exploit affordances, deal with ambiguity, or shift frames in ways organismic agents can. Because of these limitations, algorithms cannot evolve in truly novel directions (see Section 8 below).
6. Implications for Robots
So far, we have only considered algorithms that run within some stationary computing environment. The digital and purely virtual nature of this environment implies that all features within in must, by definition, be formally predefined. Its digital environment, in its finite totality, is the ontology of an AI algorithm. There is nothing outside it for the AI “agent” to discover. The real world is not like that. We have argued in the previous sections that our world is full of surprises that cannot be entirely formalized, since not all future possibilities can be prestated. Therefore, the question arises whether an AI agent that does get exposed to the real world could identify and leverage affordances when it encounters them.
In other words, does our argument apply to embodied Turing machines , such as robots, that interact with the physical world through sensors and actuators and may be able to modify their bodily configuration? The crucial difference to a purely virtual AI “agent” is that the behavior of a robot results from interactions between its control program (an algorithm), its physical characteristics (which define its repertoire of actions), and the physical environment in which it finds itself ( Pfeifer and Bongard, 2006 ). Moreover, learning techniques are put to powerful use in robotics, meaning that robots can adapt their behavior and improve their performance based on their relations to their physical environment. Therefore, we can say that robots are able to learn from experience and to identify specific sensory-motor patterns in the real world that are useful to attain their goals ( Pfeifer and Scheier, 2001 ). For instance, a quadruped robot controlled by an artificial neural network can learn to control its legs on the basis of the forces perceived from the ground, so as to develop a fast and robust gait. This learning process can be guided either by a task-oriented evaluation function, such as forward gait speed, or a task-agnostic one that rewards coordinated behaviors ( Prokopenko, 2013 ), or both.
Does that mean that robots, as embodied Turing machines, can identify and exploit affordances? Does it mean that robots, just like organisms, have an umwelt full of opportunities and threats? As in the case of stationary AI “agents,” the answer is a clear and resounding “no.” The same problems we have discussed in the previous sections also affect robotics. Specifically, they manifest themselves as the symbol grounding problem and the frame problem. The symbol grounding problem concerns the issue of attaching symbols to sensory-motor patterns ( Harnad, 1990 ). It amounts to the question whether it is feasible for a robot to detect relevant sensory-motor patterns that need to be associated with new concepts— i. e ., new variables in the ontology of the robot. This, in turn, leads to the more general frame problem (see Section 2 and McCarthy and Hayes, 1969 ): the problem of specifying in a given situation what is relevant for a robot's goals. Again, we run into the problem of choosing one's own goals, of shifting frames, and of dealing with ambiguous information that cannot be formalized in the form of a predefined set of possibilities.
As an example, consider the case of a robot whose goal it is to open coconuts. Its only available tool is an engine block, which it currently uses as a paper weight. There are no other tools, and the coconuts cannot be broken by simply throwing them against a wall. In order to achieve its goal, the robot must acquire information on the relevant causal features of the engine block to open coconuts. Can it exploit this affordance? The robot can move around and perceive the world via its sensors. It can acquire experience by performing random moves, one of which may cause it to hit the engine block, to discover that the block has the property of being “hard and sharp,” which is useful for cracking the nut. However, how does the robot know that it needs to look for this property in the objects of its environment? This is but the first useful step in solving the problem. By the same random moves, the robot might move the engine block, or tip it on its side. How can the robot “understand” that “hard and sharp” will prove to be useful, but “move to the left” will not? How long will this single step take?
Furthermore, if the coconut is lying beside the engine block, tipping it over may lead to the nut being cracked as well. How can the robot connect several coordinated causal features to achieve its goal, if none of them can be deduced from the others? The answer is: it cannot. We observe that achieving the final goal may require connecting several relevant coordinated causal features of real-world objects, none of which is deducible from the others . This is analogous to the discovery process in mathematics we have described in Section 2: wandering through a succession of dark rooms, each transition illuminated by the next in a succession of insights. There is no way for the robot to know that it is improving over the incremental steps of its search. Once an affordance is identified, new affordances emerge as a consequence and the robot cannot “know” in advance that it is accumulating successes until it happens upon the final achievement: there is no function optimization to be performed over such a sequence of steps, no landscape to search by exploiting its gradients, because each step is a search in a space of possibilities that cannot be predefined. The journey from taking the first step to reaching the ultimate goal is blind luck over some unknown time scale. With more steps, it becomes increasingly difficult to know if the robot improves, since reaching the final goal is in general not an incremental process.
The only way to achieve the robot's ultimate goal is for it to already have a preprogrammed ontology that allows for multi-step inferences. Whether embodied or not, the robot's control algorithm can only operate deductively. But if the opportunity to crack open coconuts on the engine block has been predefined, then it does not really count as discovering a new causal property. It does not count as exploiting a novel affordance. Robots do not generate new opportunities for themselves in the way organisms do. Even though engaging with their environment, they cannot participate in the emergent triad of goals, actions, and affordances (see Section 4). Therefore, we must conclude that its embodied nature does not really help a robotic algorithm to achieve anything resembling true AGI.
7. Possible Objections
We suspect that our argument may raise a number of objections. In this section, we anticipate some of these, and attempt to provide adequate replies.
A first potential objection concerns the ability of deep-learning algorithms to detect novel correlations in large data sets in an apparently hypothesis-free and unbiased manner. The underlying methods are mainly based on complex network models, rather than traditional sequential formal logic. When the machine is trained with suitable data, shouldn't it be able to add new symbols to its ontology that represent the newly discovered correlations? Would this not count as identifying and exploiting a new affordance? While it is true that the ontology of such a deep-learning machine is not explicitly predefined, it is nevertheless implicitly given through the constraints of the algorithm and the training scenario. Correlations can only be detected between variables that are defined through an external model of the data. Moreover, all current learning techniques rely on the maximization (or minimization) of one or more evaluation functions. These functions must be provided by the designers of the training scenario, who thus determine the criteria for performance improvement. The program itself does not have the ability of choosing the goal of the task at hand. This even holds for task-agnostic functions of learning scenarios, as they again are the result of an imposed external choice. In the end, with no bias or hypothesis at all, what should the learning program look for? In a truly bias- or hypothesis-free scenario (if that is possible at all), any regularity (even if purely accidental) would become meaningful ( Calude and Longo, 2017 ), which results in no meaning at all. Without any goal or perspective, there is no insight to be gained.
A second objection might be raised concerning the rather common observation that AI systems, such as programs playing chess or composing music, often surprise us or behave in unpredictable ways. However, machine unpredictability does not imply that their behavior is not deducible. Instead, it simply means that we cannot find an explanation for it, maybe due to a lack of information, or due to our own limited cognitive and/or computational resources. For example, a machine playing chess can take decisions by exploiting a huge repertoire of moves, and this may produce surprising behavior in the eye of the human opponent, since it goes far beyond our own cognitive capacity. Nevertheless, the behavior of the machine is deductively determined, ultimately based on simple combinatorics. More generally, it is well-known that there are computer programs whose output is not compressible. Their behavior cannot be predicted other than actually running the full program. This computationally irreducible behavior cannot be anticipated, but it is certainly algorithmic. Due to their competitive advantage when dealing with many factors, or many steps, in a deductive procedure, AI “agents” can easily fool us by mimicking creative behavior, even though their algorithmic operation does not allow for the kind of semantic innovation even a simple organism is capable of.
A third objection could be that our argument carelessly ignores potential progress in computational paradigms and robot design that may lead to a solution of the apparently irresolvable problems we present here. A common futurist scenario in this context is one in which AI “agents” themselves replace human engineers in designing AI architectures, leading to a technological singularity —a technology which is far beyond human grasp (see, for example, Vinge, 1993 ; Kurzweil, 2005 ; Eden et al., 2013 ; Bostrom, 2014 ; Shanahan, 2015 ; Chalmers, 2016 ). We are sympathetic to this objection (although not to the notion of a singularity based on simple extrapolation of our current capabilities). Our philosophical approach is exactly based on the premise that the future is open, and will always surprise us in fundamentally unpredictable ways. But there is no paradox here: what we are arguing for is that AGI is impossible within the current algorithmic frame of AI research, which is based on Turing machines. We are open to suggestions how the limitations of this frame could be transcended. One obvious way to do this is a biological kind of robotics, which uses organismic agents (such as biological cells) to build organic computation devices or robots. We are curious (and also apprehensive) concerning the potential (and dangers) which such non-algorithmic frameworks hold for the future. An AGI which could indeed choose its own goals, would not be aligned with our own interests (by definition), and may not be controllable by humans, which seems to us to defy the purpose of generating AI as a benign and beneficial technology in the first place.
One final, and quite serious, philosophical objection to our argument is that it may be impossible to empirically distinguish between a sophisticated algorithm mimicking agential behavior , and true organismic agency as outlined in Section 3. In this case, our argument may be of no practical importance. It is true that humans are easily fooled into interpreting completely mechanistic behavior in intentional and teleological terms. Douglas Hofstadter (2007) , for example, mentions a dot of red light that is moving along the walls of the San Francisco Exploratorium, responding by simple feedback to movements of the museum visitors. Every time a visitor tries to touch the dot, it seems to escape at the very last moment. Even though based on a simple feedback mechanism, it is tempting to interpret such behavior as intentional. 3 Could we have fallen prey to such an illusion when interpreting the behavior of organisms as true agency? We do not think so. First, the organizational account of agency we rely on not only accounts for goal-oriented behavior, but also for basic functional properties of living systems, such as their autopoietic ability to self-maintain and self-repair. Thus, agency is a higher-level consequence of more basic abilities of organisms that cannot easily be accounted for by alternative explanations. Even though these basic abilities have not yet been put to the test in a laboratory, there is no reason to think that they won't be in the not-too-far future. Second, we think the account of organismic agency presented here is preferable over an algorithmic explanation of “agency” as evolved input-output processing, since it has much greater explanatory power. It takes the phenomenon of agency seriously instead of trying to explain it away. Without this conceptual framework, we could not even ask the kind of questions raised in this paper, since they would never arise within an algorithmic framework. In essence, the non-reductionist (yet still naturalist) world we operate in is richer than the reductionist one in that it allows us to deal scientifically with a larger range of undoubtedly interesting and relevant phenomena (see also Wimsatt, 2007 ).
8. Open-Ended Evolution in Computer Simulations
Before we conclude our argument, we would like to consider its implications beyond AGI, in particular, for the theory of evolution, and for research in the field of artificial life (ALife). One of the authors has argued earlier that evolvability and agency must go together, because the kind of organizational continuity that turns a cell cycle into a reproducer —the minimal unit of Darwinian evolution—also provides the evolving organism with the ability to act autonomously ( Jaeger, 2022 ). Here, we go one step further and suggest that organismic agency is a fundamental prerequisite for open-ended evolution , since it enables organisms to identify and exploit affordances in their umwelt, or perceived environment. Without agency, there is no co-emergent dialectic between organisms' goals, actions, and affordances (see Section 5). And without this kind of dialectic, evolution cannot transcend its predetermined space of possibilities. It cannot enter into the next adjacent possible. It cannot truly innovate, remaining caught in a deductive ontological frame ( Fernando et al., 2011 ; Bersini, 2012 ; Roli and Kauffman, 2020 ).
Let us illustrate this with the example of ALife. The ambitious goal of this research field is to create models of digital “organisms” that are able to evolve and innovate in ways equivalent to natural evolution. Over the past decades, numerous attempts have been made to generate open-ended evolutionary dynamics in simulations such as Tierra ( Ray, 1992 ) and Avida ( Adami and Brown, 1994 ). In the latter case, the evolving “organisms” reach an impressive level of sophistication (see, for example, Lenski et al., 1999 , 2003 ; Zaman et al., 2014 ). They have an internal “metabolism” that processes nutrients to gain energy from their environment in order to survive and reproduce. However, this “metabolism” does not exhibit organizational closure, or any other form of true agency, since it remains purely algorithmic. And so, no matter how complicated, such evolutionary simulations always tend to get stuck at a certain level of complexity ( Bedau et al., 2000 ; Standish, 2003 ). Even though some complexification of ecological interactions ( e. g. , mimics of trophic levels or parasitism) can occur, we never observe any innovation that goes beyond what was implicitly considered in the premises of the simulation. This has led to some consternation and the conclusion that the strong program of ALife—to generate any truly life-like processes in a computer simulation—has failed to achieve its goal so far. In fact, we would claim that this failure is comprehensive: it affects all attempts at evolutionary simulation that have been undertaken so far. Why is that so?
Our argument provides a possible explanation for the failure of strong ALife: even though the digital creatures of Avida, for example, can exploit “new” nutrient sources, they can only do so because these sources have been endowed with the property of being a potential food source at the time the simulation was set up. They were part of its initial ontology. The algorithm cannot do anything it was not (implicitly) set up to do. Avida's digital “life forms” can explore their astonishingly rich and large space of possibilities combinatorially. This is what allows them, for example, to feed off other “life forms” to become predators or parasites. The resulting outcomes may even be completely unexpected to an outside observer with insufficient information and/or cognitive capacity (see Section 7). However, Avida's “life forms” can never discover or exploit any truly new opportunities, like even the most primitive natural organisms can. They cannot generate new meaning that was not already programmed into their ontology. They cannot engage in semiosis. What we end up is a very high-dimensional probabilistic combinatorial search. Evolution has often been likened to such intricate search strategies, but our view suggests that organismic agency pushes it beyond.
Organismic open-ended evolution into the adjacent possible requires the identification and leveraging of novel affordances. In this sense, it cannot be entirely formalized. In contrast, algorithmic evolutionary simulations will forever be constrained by their predefined formal ontologies. They will never be able to produce any true novelty, or radical emergence. They are simply not like organismic evolution since they lack its fundamental creativity. As some of us have argued elsewhere: emergence is not engineering ( Kauffman and Roli, 2021a ). The biosphere is an endlessly propagating adapting construction, not an entailed algorithmic deduction ( Kauffman, 2019 ). In other words, the world is not a theorem ( Kauffman and Roli, 2021b ), but a neverending exploratory process. It will never cease to fascinate and surprise us.
9. Conclusion
In this paper, we have argued two main points: (1) AGI is impossible in the current algorithmic frame of research in AI and robotics, since algorithms cannot identify and exploit new affordances. (2) As a direct corollary, truly open-ended evolution into the adjacent possible is impossible in algorithmic systems, since they cannot transcend their predefined space of possibilities.
Our way of arriving at these conclusions is not the only possible one. In fact, the claim that organismic behavior is not entirely algorithmic was made by Robert Rosen as early as the 1950s ( Rosen, 1958a , b , 1959 , 1972 ). His argument is based on category theory and neatly complements our way of reasoning, corroborating our insight. It is summarized in Rosen's book “Life Itself” ( Rosen, 1991 ). As a proof of principle, he devised a diagram of compositional mappings that exhibit closure to efficient causation , which is equivalent to organizational closure (see Section 3). He saw this diagram as a highly abstract relational representation of the processes that constitute a living system. Rosen was able to prove mathematically that this type of organization “has no largest model” ( Rosen, 1991 ). This has often been confounded with the claim that it cannot be simulated in a computer at all. However, Rosen is not saying that we cannot generate algorithmic models of some (maybe even most) of the behaviors that a living system can exhibit. In fact, it has been shown that his diagram can be modeled in this way using a recursive functional programming paradigm ( Mossio et al., 2009 ). What Rosen is saying is exactly what we are arguing here: there will always be some organismic behaviors that cannot be captured by a preexisting formal model. This is an incompleteness argument of the kind Gödel made in mathematics ( Nagel and Newman, 2001 ): for most problems, it is still completely fine to use number theory after Gödel's proof. In fact, relevant statements about numbers that do not fit the theory are exceedingly rare in practice. Analogously, we can still use algorithms implemented by computer programs to study many aspects of organismic dynamics, or to engineer (more or less) target-specific AIs. Furthermore, it is always possible to extend the existing formal model to accommodate a new statement or behavior that does not yet fit in. However, this process is infinite. We will never arrive at a formal model that captures all possibilities. Here, we show that this is because those possibilities cannot be precisely prestated and defined in advance.
Another approach that comes to very similar insights to ours is biosemiotics (see, for example, Hoffmeyer, 1993 ; Barbieri, 2007 ; Favareau, 2010 ; Henning and Scarfe, 2013 ). Rather than a particular field of inquiry, biosemiotics sees itself as a broad and original perspective on life and its evolution. It is formulated in terms of the production, exchange, and interpretation of signs in biological systems. The process of meaning-making (or semiosis) is central to biosemiotics ( Peirce, 1934 ). Here, we link this process to autopoiesis ( Varela et al., 1974 ; Maturana and Varela, 1980 ) and the organizational account, which sees bio-agency grounded in a closure of constraints within living systems ( Montévil and Mossio, 2015 ; Moreno and Mossio, 2015 ; Mossio et al., 2016 ), and the consequent co-emergent evolutionary dialectic of goals, actions, and affordances ( Walsh, 2015 ; Jaeger, 2022 ). Our argument suggests that the openness of semiotic evolution is grounded in our fundamental inability to formalize and prestate possibilities for evolutionary and cognitive innovation in advance.
Our insights put rather stringent limitations on what traditional mechanistic science and engineering can understand and achieve when it comes to agency and evolutionary innovation. This affects the study of any kind of agential system—in computer science, biology, and the social sciences—including higher-level systems that contain agents, such as ecosystems or the economy. In these areas of investigation, any purely formal approach will remain forever incomplete. This has important repercussions for the philosophy of science: the basic problem is that, with respect to coming to know the world, once we have carved it into a finite set of categories, we can no longer see beyond those categories. The grounding of meaning in real objects is outside any predefined formal ontology. The evolution of scientific knowledge itself is entailed by no law. It cannot be formalized ( Kauffman and Roli, 2021a , b ).
What would such a meta-mechanistic science look like? This is not entirely clear yet. Its methods and concepts are only now being elaborated (see, for example, Henning and Scarfe, 2013 ). But one thing seems certain: it will be a science that takes agency seriously. It will allow the kind of teleological behavior that is rooted in the self-referential closure of organization in living systems. It is naturalistic but not reductive. Goals, actions, and affordances are emergent properties of the relationship between organismal agents and their umwelt—the world of meaning they live in. This emergence is of a radical nature, forever pushing beyond predetermined ontologies into the adjacent possible. This results in a worldview that closely resembles Alfred North Whitehead's philosophy of organism ( Whitehead, 1929 ). It sees the world less as a clockwork, and more like an evolving ecosystem, a creative process centered around harvesting new affordances.
It should be fairly obvious by now that our argument heavily relies on teleological explanations, necessitated by the goal-oriented behavior of the organism. This may seem problematic: teleological explanations have been traditionally banned from evolutionary biology because they seemingly require (1) an inversion of the flow from cause to effect, (2) intentionality, and (3) a kind of normativity, which disqualify them from being proper naturalistic scientific explanations.
Here, we follow Walsh (2015) , who provides a very convincing argument that this is not the case. First, it is important to note that we are not postulating any large-scale teleology in evolution—no omega point toward which evolution may be headed. On the contrary, our argument for open-endedness explicitly precludes such a possibility, even in principle (see Section 8). Second, the kind of teleological explanation we propose here for the behavior of organisms and its evolution is not a kind of causal explanation. While causal explanations state which effect follows which cause, teleological explanation deals with the conditions that are conducive for an organism to attain its goal. The goal does not cause these conditions, but rather presupposes them. Because of this, there is no inversion of causal flow. Finally, the kind of goal-directed behavior enabled by bio-agency does not require awareness, intentionality, or even cognition. It can be achieved by the simplest organisms (such as bacteria), simply due to the fact that they exhibit an internal organization based on a closure of constraints (see Section 3). This also naturalizes the kind of normativity we require for teleology ( Mossio and Bich, 2017 ): the organism really does have a goal from which it can deviate. That goal is to stay alive, reproduce, and flourish. All of this means that there is nothing supranatural or unscientific about the kind of teleological explanations that are used in our argument. They are perfectly valid explanations. There is no need to restrict ourselves to strictly mechanistic arguments, which yield an impoverished world view since they cannot capture the deep problems and rich phenomena we have been discussing throughout this paper.
While such metaphysical and epistemological considerations are important for understanding ourselves and our place in the world, our argument also has eminently practical consequences. The achievement of AGI is often listed as one of the most threatening existential risks to the future of humanity (see, for example, Yudkowsky, 2008 ; Ord, 2020 ). Our analysis suggests that such fears are greatly exaggerated. No machine will want to replace us, since no machine will want anything, at least not in the current algorithmic frame of defining a machine. This, of course, does not prevent AI systems and robots from being harmful. Protocols and regulations for AI applications are urgent and necessary. But AGI is not around the corner, and we are not alone with this assessment. The limits of current AI applications have been questioned by others, emphasizing that these systems lack autonomy and understanding capabilities, which we conversely find in natural intelligence ( Nguyen et al., 2015 ; Broussard, 2018 ; Hosni and Vulpiani, 2018 ; Marcus and Davis, 2019 ; Mitchell, 2019 ; Roitblat, 2020 ; Sanjuán, 2021 ; Schneier, 2021 ). The true danger of AI lies in the social changes and the disenfranchisement of our own agency that we are currently effecting through target-specific algorithms. It is not Skynet, but Facebook, that will probably kill us in the end.
Data Availability Statement
The original contributions presented in the study are included in the article/supplementary material, further inquiries can be directed to the corresponding authors.
Author Contributions
All authors contributed equally to this manuscript, conceived the argument, and wrote the paper together.
Conflict of Interest
The authors declare that the research was conducted in the absence of any commercial or financial relationships that could be construed as a potential conflict of interest.
Publisher's Note
All claims expressed in this article are solely those of the authors and do not necessarily represent those of their affiliated organizations, or those of the publisher, the editors and the reviewers. Any product that may be evaluated in this article, or claim that may be made by its manufacturer, is not guaranteed or endorsed by the publisher.
Acknowledgments
JJ has profited from numerous discussions on the topic of organismic agency with Jan-Hendirk Hofmeyr, Matteo Mossio, and Denis Walsh, and on possibility spaces with Andrea Loettgers and Tarja Knuuttila, none of whom necessarily share the opinions expressed in this paper. JJ thanks the late Brian Goodwin for crucial early influences on his thinking. No doubt, Brian would have loved this paper.
1. ^ In the sense of a suitable model explaining the riddle. See Burnham and Anderson (2002) .
2. ^ Nova interview, https://www.pbs.org/wgbh/nova/article/andrew-wiles-fermat .
3. ^ Regarding machine intentionality see also the work by Braitenberg (1986) .
Adami, C., and Brown, C. T. (1994). “Evolutionary learning in the 2D artificial life system ‘Avida’,” in Artificial Life IV: Proceedings of the Fourth International Workshop on the Synthesis and Simulation of Living Systems , eds P. Maes and R. Brooks (Cambridge, MA: MIT Press), 377–381.
Google Scholar
Arnellos, A., and Moreno, A. (2015). Multicellular agency: an organizational view. Biol. Philosophy 30, 333–357. doi: 10.1007/s10539-015-9484-0
CrossRef Full Text | Google Scholar
Arnellos, A., Spyrou, T., and Darzentas, J. (2010). Towards the naturalization of agency based on an interactivist account of autonomy. New Ideas Psychol. 28, 296–311. doi: 10.1016/j.newideapsych.2009.09.005
Barandiaran, X., and Moreno, A. (2008). On the nature of neural information: a critique of the received view 50 years later. Neurocomputing 71, 681–692. doi: 10.1016/j.neucom.2007.09.014
Barandiaran, X. E., Di Paolo, E., and Rohde, M. (2009). Defining agency: individuality, normativity, asymmetry, and spatio-temporality in action. Adapt. Behav. 17, 367–386. doi: 10.1177/1059712309343819
Barbieri, M. editor (2007). Introduction to Biosemiotics: The New Biological Synthesis . Dordrecht, NL: Springer.
Bedau, M. A., McCaskill, J. S., Packard, N. H., Rasmussen, S., Adami, C., Green, D. G., et al. (2000). Open problems in artificial life. Artif. Life 6, 363–376. doi: 10.1162/106454600300103683
PubMed Abstract | CrossRef Full Text | Google Scholar
Bersini, H. (2012). Emergent phenomena belong only to biology. Synthese 185, 257–272. doi: 10.1007/s11229-010-9724-4
Bickhard, M. H. (2000). Autonomy, function, and representation. Commun. Cogn. Artif. Intell. 17, 111–131.
Bostrom, N. (2014). Superintelligence: Paths, Dangers, Strategies . Oxford: Oxford University Press.
Braitenberg, V. (1986). Vehicles: Experiments in Synthetic Psychology . Cambridge, MA: MIT Press.
PubMed Abstract | Google Scholar
Briot, J.-P., and Pachet, F. (2020). Deep learning for music generation: challenges and directions. Neural Comput. Appl. 32, 981–993. doi: 10.1007/978-3-319-70163-9
Broussard, M. (2018). Artificial Unintelligence: How Computers Misunderstand the World . Cambridge, MA: MIT Press.
Brown, T., Mann, B., Ryder, N., Subbiah, M., Kaplan, J., Dhariwal, P., et al. (2020). Language models are few-shot learners. arXiv preprint arXiv:2005.14165.
Burnham, K., and Anderson, D. (2002). Model Selection and Multi-Model Inference , 2nd Edn, New York, NY: Springer.
Byers, W. (2010). How Mathematicians Think . Princeton, NJ: Princeton University Press.
Calude, C., and Longo, G. (2017). The deluge of spurious correlations in big data. Found. Sci. 22, 595–612. doi: 10.1007/s10699-016-9489-4
Campbell, C., Olteanu, A., and Kull, K. (2019). Learning and knowing as semiosis: extending the conceptual apparatus of semiotics. Sign Syst. Stud. 47, 352–381. doi: 10.12697/SSS.2019.47.3-4.01
Campbell, R. (2010). The emergence of action. New Ideas Psychol. 28, 283–295. doi: 10.1016/j.newideapsych.2009.09.004
Chalmers, D. (2020). GPT-3 and general intelligence. Daily Nous 30.
Chalmers, D. J. (2016). “The singularity: a philosophical analysis,” in Science Fiction and Philosophy , ed S. Schneider (Hoboken, NJ: John Wiley & Sons, Inc), 171–224.
DiFrisco, J., and Mossio, M. (2020). Diachronic identity in complex life cycles: an organizational perspective,” in Biological Identity: Perspectives from Metaphysics and the Philosophy of Biology , eds A. S. Meincke, and J. Dupré (London: Routledge).
Domingos, P. (2015). The Master Algorithm: How the Quest for the Ultimate Learning Machine Will Remake Our World , New York, NY: Basic Books.
Douglas Hofstadter, R. (2007). I Am a Strange Loop . New York, NY: Basic Books.
Dreyfus, H. (1965). Alchemy and Artificial Intelligence . Technical Report, RAND Corporation, Santa Monica, CA, USA.
Dreyfus, H. (1992). What Computers Still Can't Do: A Critique of Artificial Reason . Cambridge, MA: MIT Press.
Eden, A. H., Moor, J. H., Soraker, J. H., and Steinhart, E. editors (2013). Singularity Hypotheses: A Scientific and Philosophical Assessment . New York, NY: Springer.
Favareau, D. editor (2010). Essential Readings in Biosemiotics . Springer, Dordrecht, NL.
Fernando, C., Kampis, G., and Szathmáry, E. (2011). Evolvability of natural and artificial systems. Proc. Compu. Sci. 7, 73–76. doi: 10.1016/j.procs.2011.12.023
Gelman, A., Carlin, J. B., Stern, H. S., Dunson, D. B., and Vehtari, A. (2013). Bayesian Data Analysis , 3rd Edn. Boca Raton, FL: Taylor & Francis Ltd.
Gibson, J. (1966). The Senses Considered as Perceptual Systems . London: Houghton Mifflin.
Gold, J. I., and Shadlen, M. N. (2007). The neural basis of decision making. Ann. Rev. Neurosci. 30, 535–574. doi: 10.1146/annurev.neuro.29.051605.113038
Harnad, S. (1990). The symbol grounding problem. Physica D Nonlin. Phenomena 42, 335–346. doi: 10.1016/0167-2789(90)90087-6
Hartshorne, C., and Weiss (1958). Collected Papers of Charles Sanders Peirce . Boston, MA: Belknap Press of Harvard University Press.
Henning, B., and Scarfe, A. editors (2013). Beyond Mechanism: Putting Life Back Into Biology . Plymouth: Lexington Books.
Heras-Escribano, M. (2019). The Philosophy of Affordances . London: Springer.
Hipólito, I., Ramstead, M., Convertino, L., Bhat, A., Friston, K., and Parr, T. (2021). Markov blankets in the brain. Neurosci. Biobehav. Rev. 125, 88–97. doi: 10.1016/j.neubiorev.2021.02.003
Hoffmeyer, J. (1993). Signs of Meaning in the Universe . Bloomington, IN: Indiana University Press.
Hong, J.-W., and Curran, N. (2019). Artificial intelligence, artists, and art: attitudes toward artwork produced by humans vs. artificial intelligence. ACM Trans. Multimedia Comput. Commun. Appl. (TOMM) 15, 1–16. doi: 10.1145/3326337
Hosni, H., and Vulpiani, A. (2018). Data science and the art of modelling. Lettera Matematica 6, 121–129. doi: 10.1007/s40329-018-0225-5
Hume, D. (2003). A Treatise of Human Nature . Chelmsford, MA: Courier Corporation.
Jaeger, J. (2022). “The fourth perspective: evolution and organismal agency,” in Organization in Biology , ed M. Mossio (Berlin: Springer).
Jamone, L., Ugur, E., Cangelosi, A., Fadiga, L., Bernardino, A., Piater, et al. (2016). Affordances in psychology, neuroscience, and robotics: a survey. IEEE Trans. Cogn. Develop. Syst. 10, 4–25. doi: 10.1109/TCDS.2016.2594134
Kant, I. (1892). Critique of Judgement . New York, NY: Macmillan.
Kauffman, S. (1976). “Articulation of parts explanation in biology and the rational search for them,” in Topics in the Philosophy of Biology , (Dordrecht: Springer), 245–263.
Kauffman, S. (2000). Investigations . Oxford: Oxford University Press.
Kauffman, S. (2003). Molecular autonomous agents. Philosoph. Trans. Roy. Soc. London Series A Math. Phys. Eng. Sci. 361, 1089–1099. doi: 10.1098/rsta.2003.1186
Kauffman, S. (2019). A World Beyond Physics: the Emergence and Evolution of Life . Oxford: Oxford University Press.
Kauffman, S. (2020). Eros and logos. Angelaki 25, 9–23. doi: 10.1080/0969725X.2020.1754011
Kauffman, S., and Clayton, P. (2006). On emergence, agency, and organization. Biol. Philosophy 21, 501–521. doi: 10.1007/s10539-005-9003-9
Kauffman, S., and Roli, A. (2021a). The third transition in science: beyond Newton and quantum mechanics – a statistical mechanics of emergence. arXiv preprint arXiv:2106.15271.
Kauffman, S., and Roli, A. (2021b). The world is not a theorem. Entropy 23:1467. doi: 10.3390/e23111467
Kennedy, B., and Thornberg, R. (2018). “Deduction, induction, and abduction,” in The SAGE Handbook of Qualitative Data Collection (London: SAGE Publications), 49–64.
Köhler, W. (2013). The Mentality of Apes . London: Routledge.
Kripke, S. (2013). “The Church-Turing “thesis” as a special corollary of Gödel's completeness theorem,” in Computability: Gödel, Turing, Church, and Beyond , eds B. Copeland, C. Posy, and O. Shagrir (Cambridge, MA: The MIT Press), Ch. 4, 77–104.
Kurzweil, R. (2005). The Singularity Is Near: When Humans Transcend Biology . New York, NY: The Viking Press.
Ladyman, J. (2001). Understanding Philosophy of Science . London: Routledge.
LaValle, S. (2006). Planning Algorithms . Cambridge: Cambridge University Press.
Lenski, R. E., Ofria, C., Collier, T. C., and Adami, C. (1999). Genome complexity, robustness and genetic interactions in digital organisms. Nature 400, 661–664. doi: 10.1038/23245
Lenski, R. E., Ofria, C., Pennock, R. T., and Adami, C. (2003). The evolutionary origin of complex features. Nature 423, 139–144. doi: 10.1038/nature01568
Marcus, G., and Davis, E.. (2019). Rebooting AI: Building Artificial Intelligence We Can Trust . New York, NY: Vintage.
Marcus, G., and Davis, E.. (2020). GPT-3, Bloviator: OpenAI's language generator has no idea what it's talking about. Technol. Rev.
Maturana, H., and Varela, F. (1973). De Maquinas y Seres Vivos . Santiago: Editorial Universitaria.
Maturana, H., and Varela, F. J. (1980). Autopoiesis and Cognition: The Realization of the Living . Dordrecht: Springer.
McCarthy, J., and Hayes, P. (1969). Some philosophical problems from the standpoint of artificial intelligence. Mach. Intell. 463–502.
McCarthy, J., Minsky, M., Rochester, N., and Shannon, C. (1955). A proposal for the Dartmouth summer research project on artificial intelligence . Available online at: http://jmc.stanford.edu/articles/dartmouth/dartmouth.pdf
McShea, D. W. (2012). Upper-directed systems: a new approach to teleology in biology. Biol. Philosophy 27, 63–684. doi: 10.1007/s10539-012-9326-2
McShea, D. W. (2013). Machine wanting. Stud. History Philosophy Sci. Part C Biol. Biomed. Sci. 44, 679–687. doi: 10.1016/j.shpsc.2013.05.015
McShea, D. W. (2016). Freedom and purpose in biology. Stud. History Philosophy Sci. Part C Biol. Biomed. Sci. 58, 64–72. doi: 10.1016/j.shpsc.2015.12.002
Meincke, A. S. (2018). “Bio-agency and the possibility of artificial agents,” in Philosophy of Science (European Studies in Philosophy of Science), Vol. 9 , Eds. A. Christian, D. Hommen, N. Retzlaff, and G. Schurz (Cham: Springer International Publishing), 65–93.
Mill, J. (1963). Collected Works . Toronto, ON: University of Toronto Press.
Mitchell, M. (2019). Artificial Intelligence: A Guide for Thinking Humans . London: Penguin UK.
Montévil, M., and Mossio, M. (2015). Biological organisation as closure of constraints. J. Theor. Biol. 372, 179–191. doi: 10.1016/j.jtbi.2015.02.029
Moreno, A., and Etxeberria, A. (2005). Agency in natural and artificial systems. Artif. Life 11, 161–175. doi: 10.1162/1064546053278919
Moreno, A., and Mossio, M. (2015). Biological Autonomy . Dordrecht: Springer.
Mossio, M., and Bich, L. (2017). What makes biological organisation teleological? Synthese 194, 1089–1114. doi: 10.1007/s11229-014-0594-z
Mossio, M., Longo, G., and Stewart, J. (2009). A computable expression of closure to efficient causation. J. Theor. Biol. 257, 489–498. doi: 10.1016/j.jtbi.2008.12.012
Mossio, M., Montévil, M., and Longo, G. (2016). Theoretical principles for biology: organization. Progr. Biophys. Mol. Biol. 122, 24–35. doi: 10.1016/j.pbiomolbio.2016.07.005
Müller, V. C., and Bostrom, N. (2016). “Future progress in artificial intelligence: a survey of expert opinion,” in Fundamental Issues of Artificial Intelligence , Vol. 376, eds V. C. Müller (Cham: Springer International Publishing), 555–572.
Nagel, E., and Newman, J. R. (2001). Gödel's Proof . New York, NY: NYU Press.
Nguyen, A., Yosinski, J., and Clune, J. (2015). “Deep neural networks are easily fooled: high confidence predictions for unrecognizable images,” in Proceedings of the IEEE Conference on Computer Vision and Pattern Recognition , Boston, MA, 427–436.
Okasha, S. (2016). Philosophy of Science: A Very Short Introduction , 2nd Edn, Oxford: Oxford University Press.
Ord, T. (2020). The Precipice . New York, NY: Hachette Books.
Peirce, C. (1934). Collected Papers, Vol 5. Cambridge, MA: Harvard University Press.
Penrose, R. (1989). The Emperor's New Mind: Concerning Computers, Minds, and the Laws of Physics . Oxford: Oxford University Press.
Pfeifer, R., and Bongard, J. (2006). How the Body Shapes the Way We Think: A New View of Intelligence , Cambridge, MA: MIT Press.
Pfeifer, R., and Scheier, C. (2001). Understanding Intelligence , Cambridge, MA: The MIT Press.
Piaget, J. (1967). Biologie et Connaissance , Paris: Delachaux & Niestle.
Prokopenko, M. (2013). Guided Self-Organization: Inception , Vol. 9. Berlin: Springer Science & Business Media.
Ray, T. S. (1992). “Evolution and optimization of digital organisms,” in Scientific Excellence in Supercomputing: the 1990 IBM Contest Prize Papers , eds K. R. Billingsley, H. U. Brown, and E. Derohanes (Atlanta, GA: Baldwin Press), 489–531.
Roitblat, H. (2020). Algorithms Are Not Enough: Creating General Artificial Intelligence . Cambridge, MA: MIT Press.
Roli, A., and Kauffman, S. (2020). Emergence of organisms. Entropy 22, 1–12. doi: 10.3390/e22101163
Rosen, R. (1958a). A relational theory of biological systems. Bull. Math. Biophys. 20, 245–260. doi: 10.1007/BF02478302
Rosen, R. (1958b). The representation of biological systems from the standpoint of the theory of categories. Bull. Math. Biophys. 20, 317–341. doi: 10.1007/BF02477890
Rosen, R. (1959). A relational theory of biological systems II. Bull. Math. Biophys. 21, 109–128. doi: 10.1007/BF02476354
Rosen, R. (1972). “Some relational cell models: the metabolism-repair systems,” in Foundations of Mathematical Biology , Vol. II, ed R. Rosen (New York, NY: Academic Press), 217–253.
Rosen, R. (1991). Life Itself: A Comprehensive Inquiry Into the Nature, Origin, and Fabrication of Life . New York, NY: Columbia University Press.
Rosen, R. (2012). Anticipatory Systems: Philosophical, Mathematical, and Methodological Foundations , 2nd Edn. New York, NY: Springer.
Russell, S., and Norvig, P. (2021). Artificial Intelligence: A Modern Approach , 4th Global Edition. London: Pearson.
Sanjuán, M. (2021). Artificial intelligence, chaos, prediction and understanding in science. Int. J. Bifurc. Chaos 31:2150173. doi: 10.1142/S021812742150173X
Scharmer, O., and Senge, P. (2016). Theory U: Leading From the Future as It Emerges , 2nd Edn, Oakland, CA: Berrett-Koehler Publishers.
Schneier, B. (2021). “The coming AI hackers,” in International Symposium on Cyber Security Cryptography and Machine Learning Berlin: Springer, 336–360.
Searle, J. R. (1980). Minds, brains, and programs. Behav. Brain Sci. 3, 417–424. doi: 10.1017/S0140525X00005756
Searle, J. R. (1992). The Rediscovery of the Mind . Cambridge, MA: Bradford Books.
Shanahan, M. (2015). The Technological Singularity . Cambridge, MA: MIT Press.
Silver, D., Huang, A., Maddison, C., Guez, A., Sifre, L., Van Den Driessche, G., et al. (2016). Mastering the game of go with deep neural networks and tree search. Nature 529, 484–489. doi: 10.1038/nature16961
Skewes, J. C., and Hooker, C. A. (2009). Bio-agency and the problem of action. Biol. Philosophy 24, 283–300. doi: 10.1007/s10539-008-9135-9
Standish, R. K. (2003). Open-ended artificial evolution. Int. J. Comput. Intell. Appl. 3, 167–175. doi: 10.1142/S1469026803000914
Taylor, A., Elliffe, D., Hunt, G., and Gray, R. (2010). Complex cognition and behavioural innovation in new caledonian crows. Proc. R. Soc. B Biol. Sci. 277, 2637–2643. doi: 10.1098/rspb.2010.0285
Uexküll von, J. (2010). A Foray Into the Worlds of Animals and Humans: With a Theory of Meaning . Minneapolis, MN: University of Minnesota Press.
Varela, F., Maturana, H., and Uribe, R. (1974). Autopoiesis: the organization of living systems, its characterization and a model. Biosystems 5, 187–196. doi: 10.1016/0303-2647(74)90031-8
Vinge, V. (1993). “The coming technological singularity: how to survive in the post-human era,” in Vision-21: Interdisciplinary Science and Engineering in the Era of Cyberspace, NASA Conference Publication CP-10129 , ed G. A. Landis (Cleveland, OH: NASA Lewis Research Center), 11–22.
Walsh, D. (2015). Organisms, Agency, and Evolution . Cambridge: Cambridge University Press.
Whitehead, A. N. (1929). Process and Reality . New York, NY: The Free Press.
Wimsatt, W. (2007). Re-Engineering Philosophy for Limited Beings: Piecewise Approximations to Reality . Boston, MA: Harvard University Press.
Yudkowsky, E. (2008). “Artificial intelligence as a positive and negative factor in global risk,” in Global Catastrophic Risks , eds N. Bostrom, and M. M. Cirkovic (Oxford: Oxford University Press).
Zaman, L., Meyer, J. R., Devangam, S., Bryson, D. M., Lenski, R. E., and Ofria, C. (2014). Coevolution drives the emergence of complex traits and promotes evolvability. PLoS Biol. 12:e1002023. doi: 10.1371/journal.pbio.1002023
Keywords: artificial intelligence (AI), universal turing machine, organizational closure, agency, affordance, evolution, radical emergence, artificial life (ALife)
Citation: Roli A, Jaeger J and Kauffman SA (2022) How Organisms Come to Know the World: Fundamental Limits on Artificial General Intelligence. Front. Ecol. Evol. 9:806283. doi: 10.3389/fevo.2021.806283
Received: 31 October 2021; Accepted: 30 December 2021; Published: 28 January 2022.
Reviewed by:
Copyright © 2022 Roli, Jaeger and Kauffman. This is an open-access article distributed under the terms of the Creative Commons Attribution License (CC BY) . The use, distribution or reproduction in other forums is permitted, provided the original author(s) and the copyright owner(s) are credited and that the original publication in this journal is cited, in accordance with accepted academic practice. No use, distribution or reproduction is permitted which does not comply with these terms.
*Correspondence: Andrea Roli, andrea.roli@unibo.it ; Johannes Jaeger, jaeger@csh.ac.at
This article is part of the Research Topic
Current Thoughts on the Brain-Computer Analogy - All Metaphors Are Wrong, But Some Are Useful

AI system self-organizes to develop features of brains of complex organisms
Cambridge scientists have shown that placing physical constraints on an artificially-intelligent system -- in much the same way that the human brain has to develop and operate within physical and biological constraints -- allows it to develop features of the brains of complex organisms in order to solve tasks.
As neural systems such as the brain organise themselves and make connections, they have to balance competing demands. For example, energy and resources are needed to grow and sustain the network in physical space, while at the same time optimising the network for information processing. This trade-off shapes all brains within and across species, which may help explain why many brains converge on similar organisational solutions.
Jascha Achterberg, a Gates Scholar from the Medical Research Council Cognition and Brain Sciences Unit (MRC CBSU) at the University of Cambridge said: "Not only is the brain great at solving complex problems, it does so while using very little energy. In our new work we show that considering the brain's problem solving abilities alongside its goal of spending as few resources as possible can help us understand why brains look like they do."
Co-lead author Dr Danyal Akarca, also from the MRC CBSU, added: "This stems from a broad principle, which is that biological systems commonly evolve to make the most of what energetic resources they have available to them. The solutions they come to are often very elegant and reflect the trade-offs between various forces imposed on them."
In a study published today in Nature Machine Intelligence , Achterberg, Akarca and colleagues created an artificial system intended to model a very simplified version of the brain and applied physical constraints. They found that their system went on to develop certain key characteristics and tactics similar to those found in human brains.
Instead of real neurons, the system used computational nodes. Neurons and nodes are similar in function, in that each takes an input, transforms it, and produces an output, and a single node or neuron might connect to multiple others, all inputting information to be computed.
In their system, however, the researchers applied a 'physical' constraint on the system. Each node was given a specific location in a virtual space, and the further away two nodes were, the more difficult it was for them to communicate. This is similar to how neurons in the human brain are organised.
The researchers gave the system a simple task to complete -- in this case a simplified version of a maze navigation task typically given to animals such as rats and macaques when studying the brain, where it has to combine multiple pieces of information to decide on the shortest route to get to the end point.
One of the reasons the team chose this particular task is because to complete it, the system needs to maintain a number of elements -- start location, end location and intermediate steps -- and once it has learned to do the task reliably, it is possible to observe, at different moments in a trial, which nodes are important. For example, one particular cluster of nodes may encode the finish locations, while others encode the available routes, and it is possible to track which nodes are active at different stages of the task.
Initially, the system does not know how to complete the task and makes mistakes. But when it is given feedback it gradually learns to get better at the task. It learns by changing the strength of the connections between its nodes, similar to how the strength of connections between brain cells changes as we learn. The system then repeats the task over and over again, until eventually it learns to perform it correctly.
With their system, however, the physical constraint meant that the further away two nodes were, the more difficult it was to build a connection between the two nodes in response to the feedback. In the human brain, connections that span a large physical distance are expensive to form and maintain.
When the system was asked to perform the task under these constraints, it used some of the same tricks used by real human brains to solve the task. For example, to get around the constraints, the artificial systems started to develop hubs -- highly connected nodes that act as conduits for passing information across the network.
More surprising, however, was that the response profiles of individual nodes themselves began to change: in other words, rather than having a system where each node codes for one particular property of the maze task, like the goal location or the next choice, nodes developed a flexible coding scheme . This means that at different moments in time nodes might be firing for a mix of the properties of the maze. For instance, the same node might be able to encode multiple locations of a maze, rather than needing specialised nodes for encoding specific locations. This is another feature seen in the brains of complex organisms.
Co-author Professor Duncan Astle, from Cambridge's Department of Psychiatry, said: "This simple constraint -- it's harder to wire nodes that are far apart -- forces artificial systems to produce some quite complicated characteristics. Interestingly, they are characteristics shared by biological systems like the human brain. I think that tells us something fundamental about why our brains are organised the way they are."
Understanding the human brain
The team are hopeful that their AI system could begin to shed light on how these constraints, shape differences between people's brains, and contribute to differences seen in those that experience cognitive or mental health difficulties.
Co-author Professor John Duncan from the MRC CBSU said: "These artificial brains give us a way to understand the rich and bewildering data we see when the activity of real neurons is recorded in real brains."
Achterberg added: "Artificial 'brains' allow us to ask questions that it would be impossible to look at in an actual biological system. We can train the system to perform tasks and then play around experimentally with the constraints we impose, to see if it begins to look more like the brains of particular individuals."
Implications for designing future AI systems
The findings are likely to be of interest to the AI community, too, where they could allow for the development of more efficient systems, particularly in situations where there are likely to be physical constraints.
Dr Akarca said: "AI researchers are constantly trying to work out how to make complex, neural systems that can encode and perform in a flexible way that is efficient. To achieve this, we think that neurobiology will give us a lot of inspiration. For example, the overall wiring cost of the system we've created is much lower than you would find in a typical AI system."
Many modern AI solutions involve using architectures that only superficially resemble a brain. The researchers say their works shows that the type of problem the AI is solving will influence which architecture is the most powerful to use.
Achterberg said: "If you want to build an artificially-intelligent system that solves similar problems to humans, then ultimately the system will end up looking much closer to an actual brain than systems running on large compute cluster that specialise in very different tasks to those carried out by humans. The architecture and structure we see in our artificial 'brain' is there because it is beneficial for handling the specific brain-like challenges it faces."
This means that robots that have to process a large amount of constantly changing information with finite energetic resources could benefit from having brain structures not dissimilar to ours.
Achterberg added: "Brains of robots that are deployed in the real physical world are probably going to look more like our brains because they might face the same challenges as us. They need to constantly process new information coming in through their sensors while controlling their bodies to move through space towards a goal. Many systems will need to run all their computations with a limited supply of electric energy and so, to balance these energetic constraints with the amount of information it needs to process, it will probably need a brain structure similar to ours."
The research was funded by the Medical Research Council, Gates Cambridge, the James S McDonnell Foundation, Templeton World Charity Foundation and Google DeepMind.
- Brain-Computer Interfaces
- Neuroscience
- Intelligence
- Neural Interfaces
- Artificial Intelligence
- Computer Programming
- Computers and Internet
- Constructal theory
- Homosexuality
- Human brain
- Facial symmetry
- Limbic system
Story Source:
Materials provided by University of Cambridge . The original text of this story is licensed under a Creative Commons Attribution-NonCommercial-ShareAlike 4.0 International License . Note: Content may be edited for style and length.
Journal Reference :
- Jascha Achterberg, Danyal Akarca, D. J. Strouse, John Duncan, Duncan E. Astle. Spatially embedded recurrent neural networks reveal widespread links between structural and functional neuroscience findings . Nature Machine Intelligence , 2023; DOI: 10.1038/s42256-023-00748-9
Cite This Page :
Explore More
- Drain On Economy Due to Climate Change
- 'Tube Map' Around Planets and Moons: Knot Theory
- 'Bizarre' Evolutionary Pattern for Homo Lineage
- Largest Known Marine Reptile
- Neolithic Humans Lived in Lava Tube Caves
- Imminent Carbon Release from the Tundra
- How Working Memory Reallly Works
- Substantial Global Cost of Climate Inaction
- Paradox of Extreme Cold Events in Warming World
- Plastic Pollution Kills Ocean Embryos
Trending Topics
Strange & offbeat.
Thank you for visiting nature.com. You are using a browser version with limited support for CSS. To obtain the best experience, we recommend you use a more up to date browser (or turn off compatibility mode in Internet Explorer). In the meantime, to ensure continued support, we are displaying the site without styles and JavaScript.
- View all journals
- Explore content
- About the journal
- Publish with us
- Sign up for alerts
- Published: 23 February 2024
Artificial intelligence needs a scientific method-driven reset
- Luís A. Nunes Amaral ORCID: orcid.org/0000-0002-3762-789X 1
Nature Physics volume 20 , pages 523–524 ( 2024 ) Cite this article
890 Accesses
21 Altmetric
Metrics details
- Applied physics
- Computational science
AI needs to develop more solid assumptions, falsifiable hypotheses, and rigorous experimentation.
This is a preview of subscription content, access via your institution
Access options
Access Nature and 54 other Nature Portfolio journals
Get Nature+, our best-value online-access subscription
24,99 € / 30 days
cancel any time
Subscribe to this journal
Receive 12 print issues and online access
195,33 € per year
only 16,28 € per issue
Buy this article
- Purchase on Springer Link
- Instant access to full article PDF
Prices may be subject to local taxes which are calculated during checkout
Tsipras, D., Santurkar, S., Engstrom, L., Ilyas, A. & Madry, A. In International Conference on Machine Learning 9625–9635 (PMLR, 2020).
Strubell, E., Ganesh, A. & McCallum, A. AAAI 34 , 13693–13696 (2019).
Article Google Scholar
Bender, E. M., Gebru, T., McMillan-Major, A. & Shmitchell, S. In Proceedings of the 2021 ACM Conference on Fairness, Accountability, and Transparency 610–623 (Association for Computing Machinery, 2021).
Goodfellow, I. J., Shlens, J. & Szegedy C. In 3rd International Conference on Learning Representations (ICLR, 2015).
Chowdhuri, R., Deshmukh, N. & Koplow, D. No, GPT4 can’t ace MIT . https://flower-nutria-41d.notion.site/No-GPT4-can-t-ace-MIT-b27e6796ab5a48368127a98216c76864 (2023).
McCarthy, J., Minsky, M., Rochester, N. & Shannon, C. E. AI Mag. 27 , 12–14 (2006).
Google Scholar
Download references
Author information
Authors and affiliations.
School of Engineering, Northwestern University, Evanston, IL, USA
Luís A. Nunes Amaral
You can also search for this author in PubMed Google Scholar
Corresponding author
Correspondence to Luís A. Nunes Amaral .
Ethics declarations
Competing interests.
The author declares no competing interests.
Rights and permissions
Reprints and permissions
About this article
Cite this article.
Nunes Amaral, L.A. Artificial intelligence needs a scientific method-driven reset. Nat. Phys. 20 , 523–524 (2024). https://doi.org/10.1038/s41567-024-02403-5
Download citation
Published : 23 February 2024
Issue Date : April 2024
DOI : https://doi.org/10.1038/s41567-024-02403-5

Share this article
Anyone you share the following link with will be able to read this content:
Sorry, a shareable link is not currently available for this article.
Provided by the Springer Nature SharedIt content-sharing initiative
Quick links
- Explore articles by subject
- Guide to authors
- Editorial policies
Sign up for the Nature Briefing: AI and Robotics newsletter — what matters in AI and robotics research, free to your inbox weekly.


The Defense Acquisition Encyclopedia
Program Management
A number of problem-solving techniques are listed below: [1]
- Abstraction : solving the problem in a model of the system before applying it to the real system
- Analogy : using a solution that solved an analogous problem
- Brainstorming: (especially among groups of people) suggesting a large number of solutions or ideas and combining and developing them until an optimum is found
- Divide and conquer : breaking down a large, complex problem into smaller, solvable problems
- Hypothesis testing : assuming a possible explanation to the problem and trying to prove (or, in some contexts, disprove) the assumption
- Lateral thinking : approaching solutions indirectly and creatively
- Means-ends analysis : choosing an action at each step to move closer to the goal
- Method of focal objects : synthesizing seemingly non-matching characteristics of different objects into something new
- Morphological analysis : assessing the output and interactions of an entire system
- Reduction : transforming the problem into another problem for which solutions exist
- Research : employing existing ideas or adapting existing solutions to similar problems
- Root cause analysis : eliminating the cause of the problem
- Trial-and-error : testing possible solutions until the right one is found
AcqLinks and References:
- [1] Webpage: Wikipedia – Problem Solving
- FEMA Decision Making and Problem Solving
- Creative Problem Solving
Updated: 7/16/2017
Leave a Reply
You must be logged in to post a comment.
Argonne National Laboratory
Science 101: artificial intelligence, what is artificial intelligence.
Artificial intelligence ( AI ) is the collective term for computer technologies and techniques that help solve complex problems by imitating the brain’s ability to learn.
AI helps computers recognize patterns hidden within a lot of information, solve problems and adjust to changes in processes as they happen, much faster than humans can.
Researchers use AI to be better and faster at tackling the most difficult problems in science, medicine and technology, and help drive discovery in those areas. This could range from helping us understand how COVID-19 attacks the human body to finding ways to manage traffic jams.
Many Department of Energy ( DOE ) facilities, like Argonne National Laboratory, assist in developing some the most advanced AI technologies available. Today, they are used in areas of study ranging from chemistry to environmental and manufacturing sciences to medicine and the universe.
AI is used to help make models of complex systems, like engines or weather, and predict what might happen if certain parts of those systems changed — for example, if a different fuel was used or temperatures increased steadily.
But there are many more uses for AI .
A key tool in Argonne’s AI toolbox is a type of technique called machine learning that gets smarter or more accurate as it gets more data to learn from. Machine learning is really helpful in identifying specific objects hidden within a bigger, more crowded picture.
In a popular example, a machine learning model was trained to recognize the main features of cats and dogs by showing it many images. Later, the model was able to identify cats and dogs from pictures of mixed animals.
Similar machine learning models can help scientists identify, for example, one type of galaxy from another when they receive object-packed images from space telescopes.
Machine learning is just one of many AI techniques that help us learn more quickly and accurately. They can help choose the right molecule or chemical for a new material and may one day guide new experiments on their own.
Argonne has worked with many organizations around the world to become a leader in artificial intelligence use and development, this includes applying AI to:
- Improve battery life for cars and energy.
- Build better climate models that can predict wildfires, hurricanes and other disasters, and help our communities and power companies protect against them.
- Find those parts of viruses that attack our cells and develop drugs to fight them.
Analyzing large complex data to perform human tasks at computer speeds
Artificial intelligence ( AI ) is now a part of our daily lives, helping to simplify basic tasks, such as voice recognition, content recommendations or photo searches based on people or objects they contain. Scientists are using AI in similar ways to advance our understanding of the world around us. It can help them analyze mountains of data faster, and has provided better solutions. Different AI techniques are used in many research areas, from materials science and medicine to climate change and the cosmos.
For example, we can train AI to recognize complex patterns by viewing many different examples. Researchers can use this capability to find new and improved materials for things like solar cells or medicine by training AI on all the known materials for that application. Then AI can help researchers zero in on other promising materials that can be fabricated and tested in a laboratory.
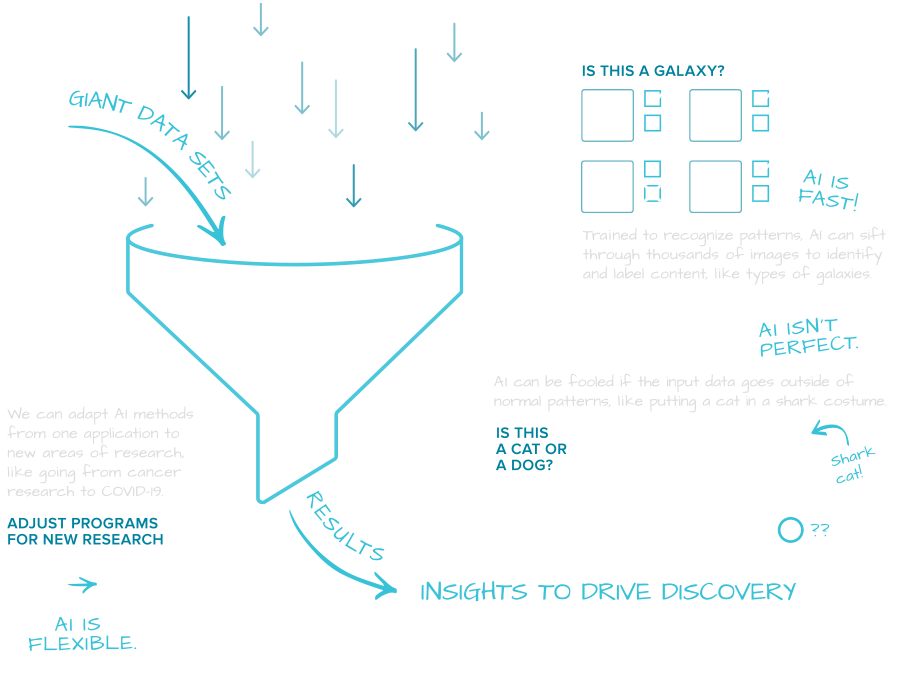

An official website of the United States government
The .gov means it’s official. Federal government websites often end in .gov or .mil. Before sharing sensitive information, make sure you’re on a federal government site.
The site is secure. The https:// ensures that you are connecting to the official website and that any information you provide is encrypted and transmitted securely.
- Publications
- Account settings
Preview improvements coming to the PMC website in October 2024. Learn More or Try it out now .
- Advanced Search
- Journal List
- Springer Nature - PMC COVID-19 Collection

Artificial Intelligence Technology and Social Problem Solving
Yeunbae kim.
12 Intelligent Information Technology Research Center, Hanyang University, Seoul, Korea
Jaehyuk Cha
13 Department of Computer Science, Hanyang University, Seoul, Korea
Modern societal issues occur in a broad spectrum with very high levels of complexity and challenges, many of which are becoming increasingly difficult to address without the aid of cutting-edge technology. To alleviate these social problems, the Korean government recently announced the implementation of mega-projects to solve low employment, population aging, low birth rate and social safety net problems by utilizing AI and ICBM (IoT, Cloud Computing, Big Data, Mobile) technologies. In this letter, we will present the views on how AI and ICT technologies can be applied to ease or solve social problems by sharing examples of research results from studies of social anxiety, environmental noise, mobility of the disabled, and problems in social safety. We will also describe how all these technologies, big data, methodologies and knowledge can be combined onto an open social informatics platform.
Introduction
A string of breakthroughs in artificial intelligence has placed AI in increasingly visible positions in society, heralding its emergence as a viable, practical, and revolutionary technology. In recent years, we have witnessed IBM’s Watson win first place in the American quiz show Jeopardy! and Google’s AlphaGo beat the Go world champion, and in the very near future, self-driving cars are expected to become a common sight on every street. Such promising developments spur optimism for an exciting future produced by the integration of AI technology and human creativity.
AI technology has grown remarkably over the past decade. Countries around the world have invested heavily in AI technology research and development. Major corporations are also applying AI technology to social problem solving; notably, IBM is actively working on their Science for Social Good initiative. The initiative will build on the success of the company’s noted AI program, Watson, which has helped address healthcare, education, and environmental challenges since its development. One particularly successful project used machine learning models to better understand the spread of the Zika virus. Using complex data, the team developed a predictive model that identified which primate species should be targeted for Zika virus surveillance and management. The results of the project are now leading new testing in the field to help prevent the spread of the disease [ 1 ].
On the other hand, investments in technology are generally mostly used for industrial and service growth, while investments for positive social impact appear to be relatively small and passive. This passive attitude seems to reflect the influence of a given nation’s politics and policies rather than the absence of technology.
For example, in 2017, only 4.2% of the total budget of the Korean government’s R&D of ICT (Information and Communication Technology) was used for social problem solving, but this investment will be increased to 45% within the next five years as the improvement of Korean people’s livelihoods and social problems are selected as important issues by the present government [ 2 ]. In addition, new categories within ICT, including AI, are required as a key means of improving quality of life and achieving population growth in this country.
In this letter, I introduce research on the informatics platform for social problem solving, specifically based on spatio-temporal data, conducted by Hanyang University and cooperating institutions. This research ultimately intends to develop informatics and convergent scientific methodologies that can explain, predict and deal with diverse social problems through a transdisciplinary convergence of social sciences, data science and AI. The research focuses on social problems that involve spatio-temporal information, and applies social scientific approaches and data-analytic methods on a pilot basis to explore basic research issues and the validity of the approaches. Furthermore, (1) open-source informatics using convergent-scientific methodology and models, and (2) the spatio-temporal data sets that are to be acquired in the midst of exploring social problems for potential resolution are developed.
In order to examine the applicability of the models and informatics platform in addressing a variety of social problems in the public as well as in private sectors, the following social problems are identified and chosen:
- Analysis of individual characteristics with suicidal impulse
- Study on the mobility of the disabled using GPS data
- Visualization of the distribution of anxiety using Social Network Services
- Big data - based analysis of noise environment and exploration of technical and institutional solutions for its improvement
- Analysis of the response governance regarding the Middle Eastern Respiratory Syndrome (MERS)
The research issues in the above social problems are explored, and the validity of the convergent-scientific methodologies are tested. The feasibility for the potential resolution of the problems are also examined. The relevant data and information are stored in a knowledge base (KB), and at the same time research methods that are used in data extraction, collection, analysis and visualization are also developed. Furthermore, the KB and the method database are merged into an open informatics platform in order to be used in various research projects, business activities, and policy debates.
Pilot Research and Studies on Social Problem Solving
Analysis of individual characteristics with suicidal impulse.
While suicide rates in OECD countries are declining, only South Korea has increasing suicide rates; moreover, Korea currently has the highest suicide rate among OECD countries as shown in Fig. 1 . Its high suicide rate is one of Korea’s biggest social problems, entailing the establishment of effective suicide prevention measures by understanding the causes of suicide. The goals of the research are to: (1) understand suicidal impulse by analyzing the characteristics of members of society according to suicidal impulse experience; (2) predict the likelihood of attempting suicide and analyzing the spatio-temporal quality of life; and (3) to establish a policy to help prevent suicide.

2013Y suicide rate by OECD countries
The Korean Social Survey and Survey of Youth Health Status Data are used for the analysis of suicide risk groups through data mining techniques, using a predictive model based on cell propagation to overcome the limitations of existing statistics methods such as characterization or classification. In the case of the characterization technique, results indicate that there are too many features related to suicide, and that there are variables including many categorical values, making it difficult to identify the variables that affect suicide. On the other hand, the classification technique had difficulties identifying the variables that affect suicide because the number of members attempting suicide was too small.
Correlations between suicide impulses and individual attributes of members of society and the trends of the correlations by year are obtained. The concepts of support , confidence and density are introduced to identify risk groups of suicide attempts, and computational performance problems caused by excessive numbers of risk groups are solved by applying a convex growing method.
The 2014Y social survey including personal and household information of members of the society are used for analysis. The attributes include gender , age , education , marital status , level of satisfaction , disability status , occupation status , housing , and household income .
The high-risk suicide cluster was identified using a small number of convexes. A convex is a set of cells, with one cell being the smallest unit of the cluster for the analysis, and a density is the ratio of the number of non-empty cells to the total number of cells in convex C [ 3 ].
Figure 2 shows that the highest suicidal risk group C1 is composed of members with low income and education level. It was identified that level of satisfaction with life has the highest impact on suicidal impulse, followed in order of impact by disability , marital status , housing , household income , occupation status , gender , age and level of education . The results showed that women and young people tend to have more suicidal impulse.

Suicide risk groups represented by household income and level of education
New prediction models with other machine learning methods and the establishment of mitigation policies are still in development. Subjective analyses of change of well-being, social exclusion, and characteristics of spatio-temporal analysis will also be explored in the future.
Study on the Mobility of the Disabled Using GPS Data
Mobility rights are closely related to quality of life as a part of social rights. Therefore social efforts are needed to guarantee mobility rights to both the physically and mentally disabled. The goal of the study is to suggest a policy for the extension of mobility rights of the disabled. In order to achieve this, travel patterns and socio-demographic characteristics of the physically impaired with low levels of mobility are studied. The study focused on individuals with physical impairments as the initial test group as a means to eventually gain insight into the mobility of the wider disabled population. Conventional studies on mobility measurement obtained data from travel diaries , interviews , and questionnaire surveys . A few studies used geo-location tracking GPS data.
GPS data is collected via mobile device and used to analyze the mobility patterns (distance, speed, frequency of outings) by using regression analysis, and to search for methods to extend mobility. A new metrics for mobility with a new indicator (travel range) was developed, and the way mobility impacts the quality of life of the disabled has been verified [ 4 ].
About 100 people with physical disabilities participated and collected more than 100,000 geo-location data over a month using an open mobile application called traccar . Their trajectories are visualized based on the GPS data as shown in Fig. 3 .

Visualization of trajectory of disabled using geo-location data
The use of location data explained mobility status better than the conventional questionnaire survey method. The questionnaire surveyed mainly the frequency of outings over a certain period and number of complaints about these outings. GPS data enabled researchers to conduct empirical observations on distance and range of travel. It was found that the disabled preferred bus routes that visit diverse locations over the shortest route. Age and monthly income are negatively associated with a disabled individual’s mobility.
Based on the research results, the following has been suggested: (1) development of new bus routes for the disabled and (2) recommendation of a new location for the current welfare center that would enable a greater range of travel. Further study on travel patterns by using indoor positioning technology and CCTV image data will be deployed.
Visualization of the Distribution of Anxiety Using Social Network Services
Many social issues including political polarization, competition in private education, increases in suicide rate, youth unemployment, low birth rate, and hate crime have anxiety as their background. The increase of social anxiety can intensify competition and conflict, which can interfere with social solidarity and cause a decrease in social trust.
Existing social science research mainly focused on grasping public opinion through questionnaires, and ignored the role of emotions. The Internet and social media were used to access emotional traits since they provide a platform not only for the active exchange of information, but also for the sharing and diffusion of emotional responses. If such emotional responses on the internet and geo-locations can be captured in real-time through machine learning, their spatio-temporal distribution could be visualized in order to observe their current status and changes by geographical region.
A visualization system was built to map the regional and temporal distribution of anxiety psychology by combining spatio-temporal information using SNS (Twitter) with sentiment analysis. A Twitter message collecting crawler was also developed to build a dictionary and tweet corpus. Based on these, an automatic classification system of anxiety-related messages was developed for the first time in Korea by applying machine learning to visualize the nationwide distribution of anxiety (See Fig. 4 ) [ 5 ].

Process of Twitter message classification
An average of 5,500 tweets with place_id are collected using Open API Twitter4j . To date, about 820,000 units of data have been collected. A Naïve Bayes Classifier was used for anxiety identification. An accuracy of 84.8% was obtained by using 1,750 and 70,830 anxiety and non-anxiety tweets as training data respectively, and 585 and 23,610 anxiety and non-anxiety tweets as testing data, respectively.
The system indicated the existence of regional disparities in anxiety emotions. It was found that Twitter users who reside in politicized regions have a lower degree of disclosure about their residing areas. This can be interpreted as the act of avoiding situations where the individual and the political position of the region coincide.
As anxiety is not a permanent characteristic of an individual, it can change depending on the time and situation, making it difficult to measure by questionnaire survey at any given time. The Twitter-based system can compensate for the limitations of such a survey method because it can continuously classify accumulated tweet text data and provide a temporal visualization of anxiety distribution at a given time within a desired visual scale (by ward, city, province and nationwide) as shown in Fig. 5 .

Regional distribution of anxiety in Korean society and visualization by geo-scale
Big Data-Based Analysis of Noise Environment and Exploration of Technical and Institutional Solutions for Its Improvement
Environmental issues are a major social concern in our age, and interest has been increasing not only in the consequences of pollution but also in the effects of general environmental aesthetics on quality of life. There is much active effort to improve the visual environment, but not nearly as much interest has been given to improve the auditory environment. Until now, policies on the auditory environment have remained passive countermeasures to simply quantified acoustic qualities (e.g., volume in dB) in specific places such as construction sites, railroads, highways, and residential areas. They lack a comprehensive study of contextual correlations, such as the physical properties of sound, the environmental factors in time and space, and the human emotional response of noise perception.
The goal of this study is to provide a cognitive-based, human-friendly solution to improve noise problems. In order to achieve this, the study aimed to (1) develop a tool for collecting sound data and converting into a sound database, and (2) build spatio-temporal features and a management platform for indoor and outdoor noise sources.
First, pilot experiments were conducted to predict the indicators that measure emotional reactions by developing a handheld device application for data collection.
Three separate free-walking experiments and in-depth interviews were conducted with 78 subjects at international airport lobbies and outdoor environments.
Through the experiment, the behavior patterns of the subjects in various acoustic environments were analyzed, and indicators of emotional reactions were identified. It was determined that the psychological state and the personal environment of the subject are important indicators of the perception of the auditory environment. In order to take into account both the psychological state of the subject and the physical properties of the external sound stimulus, an omnidirectional microphone is used to record the entire acoustic environment.
118 subjects with smartphones with the built-in application walked for an hour in downtown Seoul for data collection. On the app, after entering the prerequisite information, subjects pressed ‘ Good ’ or ‘ Bad ’ whenever they heard a sound that caught their attention. Pressing the button would record the sound for 15 s, and subjects were additionally asked to answer a series of questions about the physical characteristics of the specific location and the characteristics of the auditory environment. During the one-hour experiment, about 600 sound environment reports were accumulated, with one subject reporting the sound characteristics from an average of 5 different places.
Unlike previous studies, the subjects’ paths were not pre-determined, and the position, sound and emotional response of the subject are collected simultaneously. The paths can be displayed to analyze the relations of the soundscapes to the paths (Fig. 6 ).

Subject’s paths and marks for sound types
The study helped to build a positive auditory environment for specific places, to provide policy data for noise regulation and positive auditory environments, to identify the contexts and areas that are alienated from the auditory environment, and to extend the social meaning of “noise” within the study of sound.
Analysis of the Response Governance Regarding the Middle Eastern Respiratory Syndrome (MERS)
The development and spread of new infectious diseases are increasing due to the expansion of international exchange. As can be seen from the MERS outbreak in Korea in 2015, epidemics have profound social and economic impacts. It is imperative to establish an effective shelter and rapid response system (RRS) for infectious diseases control.
The goal of the study is to compare the official response system with the actual response system in order to understand the institutional mechanism of the epidemic response system, and to find effective policy alternatives through the collaboration of policy scholars and data scientists.
Web-based newspaper articles were analyzed to compare the official crisis response system designed to operate in outbreaks to the actual crisis response. An automatic news article crawling tool was developed, and 53,415 MERS-related articles were collected, clustered and stored in the database (Fig. 7 ).

Automatic news article collection & classification system
In order to manage and search for news articles related to MERS from the article database, a curation tool was developed. This tool is able to extract information into triplet graphs (subjects/verbs/objects) from the articles by applying natural language processing techniques. A basic dictionary for the analysis of the infectious disease response system was created based on the extracted triplet information. The information extracted by the curation tool is massive and complex, which limits the ability to correctly understand and interpret information.
A tool for visualizing information at a specific time with a network graph was developed and utilized to facilitate analysis and visualization of the networks (Fig. 8 ). All tools are integrated into a single platform to maximize the efficiency of the process.

Visualization of graph network by specific time
As for the official crisis response manual in case of an infectious disease, social network analysis indicated that while the National Security Bureau (NSB) and Public Health Centers play as large a role as the Center for Disease Control (CDC) in crisis management, the analysis of the news articles showed that the NSB was in fact rarely mentioned. It was found that the CDC and Central Disaster Response Headquarters, the official government organizations that deal with infectious diseases, as well as the Central MERS Management Countermeasures & Support Headquarters, a temporarily established organization, were not playing an important role in response to the MERS outbreak. On the other hand, the Ministry of Health and Welfare, medical institutions, and local governments all have played a central role in responding to MERS. This means that the structure and characteristics of the Command & Control and communication in the official response system seems to have a decisive influence on the cooperative response in a real crisis response. These results provided concrete information on the role of each respondent and the communication system that previous studies based on interviews and surveys have not found.
Much research based on machine learning has been criticized for giving more importance on method itself from the start rather than focusing on data reliability.
This study is based on a KB in which policy researchers manually analyze news articles and prepare basic data by tagging them. This way, it provides a basis for improving the reliability of results when executing text mining work through machine learning.
By using text mining techniques and social network analysis, it is possible to get a comprehensive view of social problems such as the occurrence of infectious diseases by examining the structure and characteristics of the response system from a holistic perspective of the entire system.
With the results of this study, new policies for infectious disease control are suggested in the following directions: (1) Strengthen cooperation networks in early response systems of infectious diseases; (2) Develop new, effective and efficient management plans of cooperative networks; and (3) Create new research to cover other diseases such as avian influenza and SARS [ 6 ].
Convergent Approaches and Open Informatics Platform
An ever-present obstacle in the traditional social sciences when addressing social issues are the difficulties of obtaining evidences from massive data for hypothesis and theory verification. Data science and AI can ease such difficulties and support social science by discovering hidden contexts and new patterns of social phenomena via low-cost analyses of large data. On the other hand, knowledge and patterns derived by machine learning from a large data set with noise often lack validity. Although data-driven inductive methods are effective for finding patterns and correlations, there is a clear limitation to discovering causal relationships.
Social science can help data science and AI by interpreting social phenomena through humanistic literacy and social-scientific thought to verify theoretical validity, and identifying causal relationships through deductive and qualitative approaches. This is why we need convergent-scientific approaches for social problem solving. Convergent approaches offer the new possibility of building an informatics platform that can interpret, predict and solve various social problems through the combination of social science and data science.
In all 5 pilot studies, the convergent-scientific approaches are found valid and sound. Most of the research agendas involved the real-time collection and development of spatio-temporal databases in a real-time manner, and analytic visualization of the results. Such visualization promises new possibilities in data interpretation. The data sets and tools for data collection, analysis and visualization are integrated onto an informatics platform so that they can be used in future research projects and policy debates.
The research was the first transdisciplinary attempt to converge social sciences and data sciences in Korea. This approach will offer a breakthrough in predicting, preventing and addressing future social problems. The research methodology, as a trailblazer, will offer new ground for a research field of a transdisciplinary nature converging data sciences, AI and social sciences. The data, information, knowledge, methodologies, and techniques will all be combined onto an open informatics platform. The platform will be maintained on an open-source basis so that it can be used as a hub for various academic research projects, business activities, and policy debates (See Fig. 9 ). The Open Informatics Platform is planned to be expanded to incorporate citizen sensing, in which people’s observations and views are shared via mobile devices and Internet services in the future [ 7 ].

Structure of informatics platform
Conclusions
In the area of social problem solving, fundamental problems have complex political, social and economic aspects that have their roots in human nature. Both technical and social approaches are essential for tackling social problem solving. In fact, it is the integrated, orchestrated marriage between the two that would bring us closer to effective social problem management.
We need to first study and carefully define the indicators specific to a given social problem or domain. There are many qualitative indicators that cannot be directly and explicitly measured such as social emotions, basic human needs and rights, and life fulfillment [ 8 ].
If the results of machine learning are difficult to measure or include combinations of results that are difficult to define, that particular social problem may not be suitable for machine learning. Therefore, there is a need for new social methods and algorithms that can accurately collect and identify the measurable indicators from opinions of social demanders. Recently, MIT has developed a device to quantitatively measure social signals. The small, lightweight wearable device contains sensors that record the people’s behaviors (physical activity, gestures, and the amount of variation in speech prosody, etc.) [ 9 ].
Machine learning technologies working on already existing data sets are relatively inexpensive compared to conventional million-dollar social programs since machine learning tools can be easily extended. However, they can introduce bias and errors depending on the data content used to train machine learning models or can also be misinterpreted. Human experts are always needed to recognize and correct erroneous outputs and interpretations in order to prevent prejudices [ 10 ].
In the development of AI applications, a great amount of time and resources are required to sort, identify and refine data to provide massive data for training. For instance, machine learning models need to learn millions of photos to recognize specific animals or faces, but human intelligence is able to recognize visual cues by looking at only a few photos. Perhaps it is time to develop a new AI framework which can infer and recognize objects based on small amounts of data, such as Transfer Learning [ 11 ], generate lacking data (GAN), or integrate traditional AI technologies, such as symbolic AI and statistical machine learning into new frameworks.
Machine learning is excellent in predicting, but many social problem solutions do not depend on predictions. The organic ways solutions to specific problems actually unfold according to new policies and programs can be more practical and worth studying than building a cure-all machine learning algorithm. While the evolution of AI is progressing at a stunning rate, there are still challenges to solving social problems. Further research on the integration of social science and AI is required.
A world in which artificial intelligence actually makes policy decisions is still hard to imagine. Considering the current limitations and capabilities of AI, AI should primarily be used as a decision aid.
Acknowledgements
This work was supported by the National Research Foundation of Korea (NRF) grant funded by the Korean government (MSIT 1 ) (No. 2018R1A5A7059549).
1 Ministry of Science and ICT.
Contributor Information
Fernando Koch, Email: ua.ude.bleminu@hcokf .
Atsushi Yoshikawa, Email: pj.ca.hcetit.sid@rab_ihsus_ta .
Shihan Wang, Email: moc.liamg@bb9891ws .
Takao Terano, Email: pj.ro.alalp.mulp@onaret .
Yeunbae Kim, Email: rk.ca.gnaynah@eabnueymik .
Jaehyuk Cha, Email: rk.ca.gnaynah@hjahc .
Academia.edu no longer supports Internet Explorer.
To browse Academia.edu and the wider internet faster and more securely, please take a few seconds to upgrade your browser .
Enter the email address you signed up with and we'll email you a reset link.
- We're Hiring!
- Help Center
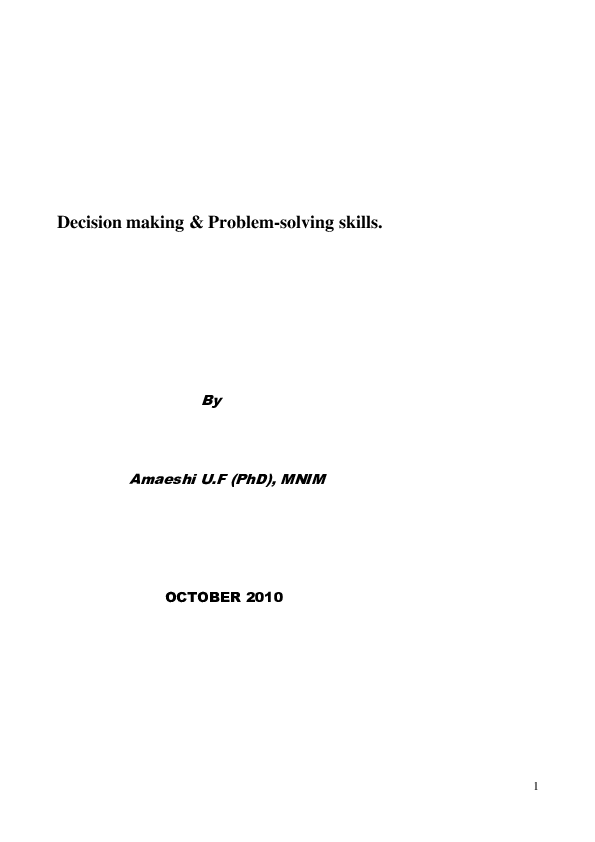
Decision making & Problem-solving skills. Decision making & Problem-solving skills

Related Papers
International Journal of Evaluation and Research in Education (IJERE)
yalçın dilekli
New world needs thinking generation. However, growing thinking generation is very difficult because there are many discrepancies in defining of thinking and thinking skills. Without defining thinking and its dimensions, it is nearly impossible to grow such generation. For having thinking generation, thinking and its dimensions should be described. In the past 20 years, there have been many different definitions with regard to thinking and thinking skills. But, three different approaches for defining thinking skills and needed basic cognitive operations were commonly accepted. Aim of this study whether these three approaches for defining thinking skills were accepted in Turkish literature or not. For this aim, 14 studies, selected from Turkish database, related to thinking skills were analyzed. According the content analysis results, Turkish literature follows these three movements for defining thinking skills except for belonging to specific areas.
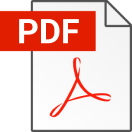
Manohar Atma
maryam farooq
Darrell Norman Burrell
Communications of the Korean Mathematical Society
Keumseong Bang
M A Matthews
Serge Brouyère
Acta Ophthalmologica
Casper Jersild
Cuadernos Hispanoamericanos de Psicología
Henry Marcelo Castillo
Se estudió el efecto de la valencia emocional y los tiempos de exposición en la consolidación de la memoria implícita mediante el uso del paradigma de priming perceptual enmascarado modificado, con reconocimiento de emociones bajo condiciones de consciencia limitada. Se presentaron rostros priming con expresiones emocionales de felicidad, rabia y neutro, en tiempos de exposición de 17ms, 33ms y 83 ms; posteriormente, los sujetos ejecutaron la tarea de memoria implícita, la cual consistía en recordar la emoción del rostro target de la primera tarea. Se encontraron diferencias estadísticamente significativas a nivel de la valencia emocional entre los diferentes tiempos de exposición en la tarea de procesamiento perceptual, en comparación con la tarea de consolidación de la memoria implícita a nivel de los tiempos de reacción. Igualmente, se encontraron diferencias estadísticamente significativas en los tiempos de exposición al comparar la tarea de procesamiento perceptual y la de cons...
RELATED PAPERS
Agrodatabase ADB
Physica C: Superconductivity
Alexander Balatsky
Otolaryngology -- Head and Neck Surgery
Victor Valdivia
2011 International Conference on Communication Systems and Network Technologies
Makmum Ashari
SPIE Proceedings
natalia gomez
European Online Journal of Natural and Social Sciences
Fatemeh zahra Mirzaei rad
The American journal of tropical medicine and hygiene
Preventive Veterinary Medicine
KOMUNITAS: International Journal of Indonesian Society and Culture
fitri juliana sanjaya
Cities and the Environment
natalia caceres
Journal of Higher Education and Science
Bekim Baliqi
Revista Brasileira de Gestão De Negócios
Décio Zylbersztajn
Anthropology of Consciousness
lydia nakashima degarrod
Junaedi Karso
Chemical Journal of Armenia
Armen Mkrtchyan
International Journal of Molecular Sciences
Izabela Irska
Optometry and Vision Science
RELATED TOPICS
- We're Hiring!
- Help Center
- Find new research papers in:
- Health Sciences
- Earth Sciences
- Cognitive Science
- Mathematics
- Computer Science
- Academia ©2024
7.1 What Is Cognition?
Learning objectives.
By the end of this section, you will be able to:
- Describe cognition
- Distinguish concepts and prototypes
- Explain the difference between natural and artificial concepts
- Describe how schemata are organized and constructed
Imagine all of your thoughts as if they were physical entities, swirling rapidly inside your mind. How is it possible that the brain is able to move from one thought to the next in an organized, orderly fashion? The brain is endlessly perceiving, processing, planning, organizing, and remembering—it is always active. Yet, you don’t notice most of your brain’s activity as you move throughout your daily routine. This is only one facet of the complex processes involved in cognition. Simply put, cognition is thinking, and it encompasses the processes associated with perception, knowledge, problem solving, judgment, language, and memory. Scientists who study cognition are searching for ways to understand how we integrate, organize, and utilize our conscious cognitive experiences without being aware of all of the unconscious work that our brains are doing (for example, Kahneman, 2011).
Upon waking each morning, you begin thinking—contemplating the tasks that you must complete that day. In what order should you run your errands? Should you go to the bank, the cleaners, or the grocery store first? Can you get these things done before you head to class or will they need to wait until school is done? These thoughts are one example of cognition at work. Exceptionally complex, cognition is an essential feature of human consciousness, yet not all aspects of cognition are consciously experienced.
Cognitive psychology is the field of psychology dedicated to examining how people think. It attempts to explain how and why we think the way we do by studying the interactions among human thinking, emotion, creativity, language, and problem solving, in addition to other cognitive processes. Cognitive psychologists strive to determine and measure different types of intelligence, why some people are better at problem solving than others, and how emotional intelligence affects success in the workplace, among countless other topics. They also sometimes focus on how we organize thoughts and information gathered from our environments into meaningful categories of thought, which will be discussed later.
Concepts and Prototypes
The human nervous system is capable of handling endless streams of information. The senses serve as the interface between the mind and the external environment, receiving stimuli and translating it into nervous impulses that are transmitted to the brain. The brain then processes this information and uses the relevant pieces to create thoughts, which can then be expressed through language or stored in memory for future use. To make this process more complex, the brain does not gather information from external environments only. When thoughts are formed, the mind synthesizes information from emotions and memories ( Figure 7.2 ). Emotion and memory are powerful influences on both our thoughts and behaviors.
In order to organize this staggering amount of information, the mind has developed a "file cabinet" of sorts. The different files stored in the file cabinet are called concepts. Concepts are categories or groupings of linguistic information, images, ideas, or memories, such as life experiences. Concepts are, in many ways, big ideas that are generated by observing details, and categorizing and combining these details into cognitive structures. You use concepts to see the relationships among the different elements of your experiences and to keep the information in your mind organized and accessible.
Concepts are informed by our semantic memory (you will learn more about semantic memory in a later chapter) and are present in every aspect of our lives; however, one of the easiest places to notice concepts is inside a classroom, where they are discussed explicitly. When you study United States history, for example, you learn about more than just individual events that have happened in America’s past. You absorb a large quantity of information by listening to and participating in discussions, examining maps, and reading first-hand accounts of people’s lives. Your brain analyzes these details and develops an overall understanding of American history. In the process, your brain gathers details that inform and refine your understanding of related concepts such as war, the judicial system, and voting rights and laws.
Concepts can be complex and abstract, like justice, or more concrete, like types of birds. In psychology, for example, Piaget’s stages of development are abstract concepts. Some concepts, like tolerance, are agreed upon by many people, because they have been used in various ways over many years. Other concepts, like the characteristics of your ideal friend or your family’s birthday traditions, are personal and individualized. In this way, concepts touch every aspect of our lives, from our many daily routines to the guiding principles behind the way governments function.
Another technique used by your brain to organize information is the identification of prototypes for the concepts you have developed. A prototype is the best example or representation of a concept. For example, what comes to your mind when you think of a dog? Most likely your early experiences with dogs will shape what you imagine. If your first pet was a Golden Retriever, there is a good chance that this would be your prototype for the category of dogs.
Natural and Artificial Concepts
In psychology, concepts can be divided into two categories, natural and artificial. Natural concepts are created “naturally” through your experiences and can be developed from either direct or indirect experiences. For example, if you live in Essex Junction, Vermont, you have probably had a lot of direct experience with snow. You’ve watched it fall from the sky, you’ve seen lightly falling snow that barely covers the windshield of your car, and you’ve shoveled out 18 inches of fluffy white snow as you’ve thought, “This is perfect for skiing.” You’ve thrown snowballs at your best friend and gone sledding down the steepest hill in town. In short, you know snow. You know what it looks like, smells like, tastes like, and feels like. If, however, you’ve lived your whole life on the island of Saint Vincent in the Caribbean, you may never actually have seen snow, much less tasted, smelled, or touched it. You know snow from the indirect experience of seeing pictures of falling snow—or from watching films that feature snow as part of the setting. Either way, snow is a natural concept because you can construct an understanding of it through direct observations, experiences with snow, or indirect knowledge (such as from films or books) ( Figure 7.3 ).
An artificial concept , on the other hand, is a concept that is defined by a specific set of characteristics. Various properties of geometric shapes, like squares and triangles, serve as useful examples of artificial concepts. A triangle always has three angles and three sides. A square always has four equal sides and four right angles. Mathematical formulas, like the equation for area (length × width) are artificial concepts defined by specific sets of characteristics that are always the same. Artificial concepts can enhance the understanding of a topic by building on one another. For example, before learning the concept of “area of a square” (and the formula to find it), you must understand what a square is. Once the concept of “area of a square” is understood, an understanding of area for other geometric shapes can be built upon the original understanding of area. The use of artificial concepts to define an idea is crucial to communicating with others and engaging in complex thought. According to Goldstone and Kersten (2003), concepts act as building blocks and can be connected in countless combinations to create complex thoughts.
A schema is a mental construct consisting of a cluster or collection of related concepts (Bartlett, 1932). There are many different types of schemata, and they all have one thing in common: schemata are a method of organizing information that allows the brain to work more efficiently. When a schema is activated, the brain makes immediate assumptions about the person or object being observed.
There are several types of schemata. A role schema makes assumptions about how individuals in certain roles will behave (Callero, 1994). For example, imagine you meet someone who introduces himself as a firefighter. When this happens, your brain automatically activates the “firefighter schema” and begins making assumptions that this person is brave, selfless, and community-oriented. Despite not knowing this person, already you have unknowingly made judgments about them. Schemata also help you fill in gaps in the information you receive from the world around you. While schemata allow for more efficient information processing, there can be problems with schemata, regardless of whether they are accurate: Perhaps this particular firefighter is not brave, they just work as a firefighter to pay the bills while studying to become a children’s librarian.
An event schema , also known as a cognitive script , is a set of behaviors that can feel like a routine. Think about what you do when you walk into an elevator ( Figure 7.4 ). First, the doors open and you wait to let exiting passengers leave the elevator car. Then, you step into the elevator and turn around to face the doors, looking for the correct button to push. You never face the back of the elevator, do you? And when you’re riding in a crowded elevator and you can’t face the front, it feels uncomfortable, doesn’t it? Interestingly, event schemata can vary widely among different cultures and countries. For example, while it is quite common for people to greet one another with a handshake in the United States, in Tibet, you greet someone by sticking your tongue out at them, and in Belize, you bump fists (Cairns Regional Council, n.d.)
Because event schemata are automatic, they can be difficult to change. Imagine that you are driving home from work or school. This event schema involves getting in the car, shutting the door, and buckling your seatbelt before putting the key in the ignition. You might perform this script two or three times each day. As you drive home, you hear your phone’s ring tone. Typically, the event schema that occurs when you hear your phone ringing involves locating the phone and answering it or responding to your latest text message. So without thinking, you reach for your phone, which could be in your pocket, in your bag, or on the passenger seat of the car. This powerful event schema is informed by your pattern of behavior and the pleasurable stimulation that a phone call or text message gives your brain. Because it is a schema, it is extremely challenging for us to stop reaching for the phone, even though we know that we endanger our own lives and the lives of others while we do it (Neyfakh, 2013) ( Figure 7.5 ).
Remember the elevator? It feels almost impossible to walk in and not face the door. Our powerful event schema dictates our behavior in the elevator, and it is no different with our phones. Current research suggests that it is the habit, or event schema, of checking our phones in many different situations that makes refraining from checking them while driving especially difficult (Bayer & Campbell, 2012). Because texting and driving has become a dangerous epidemic in recent years, psychologists are looking at ways to help people interrupt the “phone schema” while driving. Event schemata like these are the reason why many habits are difficult to break once they have been acquired. As we continue to examine thinking, keep in mind how powerful the forces of concepts and schemata are to our understanding of the world.
As an Amazon Associate we earn from qualifying purchases.
This book may not be used in the training of large language models or otherwise be ingested into large language models or generative AI offerings without OpenStax's permission.
Want to cite, share, or modify this book? This book uses the Creative Commons Attribution License and you must attribute OpenStax.
Access for free at https://openstax.org/books/psychology-2e/pages/1-introduction
- Authors: Rose M. Spielman, William J. Jenkins, Marilyn D. Lovett
- Publisher/website: OpenStax
- Book title: Psychology 2e
- Publication date: Apr 22, 2020
- Location: Houston, Texas
- Book URL: https://openstax.org/books/psychology-2e/pages/1-introduction
- Section URL: https://openstax.org/books/psychology-2e/pages/7-1-what-is-cognition
© Jan 6, 2024 OpenStax. Textbook content produced by OpenStax is licensed under a Creative Commons Attribution License . The OpenStax name, OpenStax logo, OpenStax book covers, OpenStax CNX name, and OpenStax CNX logo are not subject to the Creative Commons license and may not be reproduced without the prior and express written consent of Rice University.
Suggestions or feedback?
MIT News | Massachusetts Institute of Technology
- Machine learning
- Social justice
- Black holes
- Classes and programs
Departments
- Aeronautics and Astronautics
- Brain and Cognitive Sciences
- Architecture
- Political Science
- Mechanical Engineering
Centers, Labs, & Programs
- Abdul Latif Jameel Poverty Action Lab (J-PAL)
- Picower Institute for Learning and Memory
- Lincoln Laboratory
- School of Architecture + Planning
- School of Engineering
- School of Humanities, Arts, and Social Sciences
- Sloan School of Management
- School of Science
- MIT Schwarzman College of Computing
Artificial intelligence system uses transparent, human-like reasoning to solve problems
Press contact :.
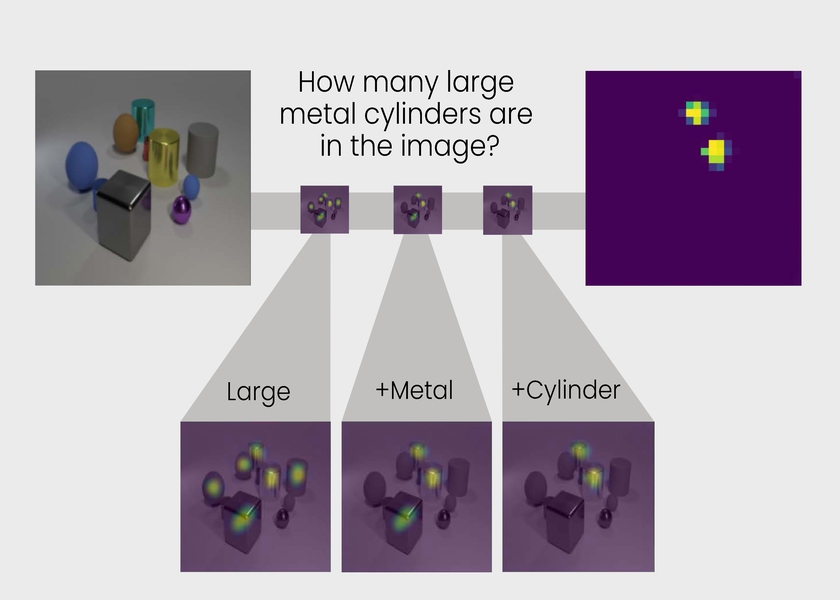
Previous image Next image
A child is presented with a picture of various shapes and is asked to find the big red circle. To come to the answer, she goes through a few steps of reasoning: First, find all the big things; next, find the big things that are red; and finally, pick out the big red thing that’s a circle.
We learn through reason how to interpret the world. So, too, do neural networks. Now a team of researchers from MIT Lincoln Laboratory's Intelligence and Decision Technologies Group has developed a neural network that performs human-like reasoning steps to answer questions about the contents of images. Named the Transparency by Design Network (TbD-net), the model visually renders its thought process as it solves problems, allowing human analysts to interpret its decision-making process. The model performs better than today’s best visual-reasoning neural networks.
Understanding how a neural network comes to its decisions has been a long-standing challenge for artificial intelligence (AI) researchers. As the neural part of their name suggests, neural networks are brain-inspired AI systems intended to replicate the way that humans learn. They consist of input and output layers, and layers in between that transform the input into the correct output. Some deep neural networks have grown so complex that it’s practically impossible to follow this transformation process. That's why they are referred to as "black box” systems, with their exact goings-on inside opaque even to the engineers who build them.
With TbD-net, the developers aim to make these inner workings transparent. Transparency is important because it allows humans to interpret an AI's results.
It is important to know, for example, what exactly a neural network used in self-driving cars thinks the difference is between a pedestrian and stop sign, and at what point along its chain of reasoning does it see that difference. These insights allow researchers to teach the neural network to correct any incorrect assumptions. But the TbD-net developers say the best neural networks today lack an effective mechanism for enabling humans to understand their reasoning process.
"Progress on improving performance in visual reasoning has come at the cost of interpretability,” says Ryan Soklaski, who built TbD-net with fellow researchers Arjun Majumdar, David Mascharka, and Philip Tran.
The Lincoln Laboratory group was able to close the gap between performance and interpretability with TbD-net. One key to their system is a collection of "modules," small neural networks that are specialized to perform specific subtasks. When TbD-net is asked a visual reasoning question about an image, it breaks down the question into subtasks and assigns the appropriate module to fulfill its part. Like workers down an assembly line, each module builds off what the module before it has figured out to eventually produce the final, correct answer. As a whole, TbD-net utilizes one AI technique that interprets human language questions and breaks those sentences into subtasks, followed by multiple computer vision AI techniques that interpret the imagery.
Majumdar says: "Breaking a complex chain of reasoning into a series of smaller subproblems, each of which can be solved independently and composed, is a powerful and intuitive means for reasoning."
Each module's output is depicted visually in what the group calls an "attention mask." The attention mask shows heat-map blobs over objects in the image that the module is identifying as its answer. These visualizations let the human analyst see how a module is interpreting the image.
Take, for example, the following question posed to TbD-net: “In this image, what color is the large metal cube?" To answer the question, the first module locates large objects only, producing an attention mask with those large objects highlighted. The next module takes this output and finds which of those objects identified as large by the previous module are also metal. That module's output is sent to the next module, which identifies which of those large, metal objects is also a cube. At last, this output is sent to a module that can determine the color of objects. TbD-net’s final output is “red,” the correct answer to the question.
When tested, TbD-net achieved results that surpass the best-performing visual reasoning models. The researchers evaluated the model using a visual question-answering dataset consisting of 70,000 training images and 700,000 questions, along with test and validation sets of 15,000 images and 150,000 questions. The initial model achieved 98.7 percent test accuracy on the dataset, which, according to the researchers, far outperforms other neural module network–based approaches.
Importantly, the researchers were able to then improve these results because of their model's key advantage — transparency. By looking at the attention masks produced by the modules, they could see where things went wrong and refine the model. The end result was a state-of-the-art performance of 99.1 percent accuracy.
"Our model provides straightforward, interpretable outputs at every stage of the visual reasoning process,” Mascharka says.
Interpretability is especially valuable if deep learning algorithms are to be deployed alongside humans to help tackle complex real-world tasks. To build trust in these systems, users will need the ability to inspect the reasoning process so that they can understand why and how a model could make wrong predictions.
Paul Metzger, leader of the Intelligence and Decision Technologies Group, says the research “is part of Lincoln Laboratory’s work toward becoming a world leader in applied machine learning research and artificial intelligence that fosters human-machine collaboration.”
The details of this work are described in the paper, “ Transparency by Design: Closing the Gap Between Performance and Interpretability in Visual Reasoning ," which was presented at the Conference on Computer Vision and Pattern Recognition (CVPR) this summer.
Share this news article on:
Related links.
- Paper: "Transparency by Design: Closing the Gap Between Performance and Interpretability in Visual Reasoning"
- Lincoln Laboratory Intelligence and Decision Technologies Group
Related Topics
- Artificial intelligence
- Computer science and technology
Related Articles
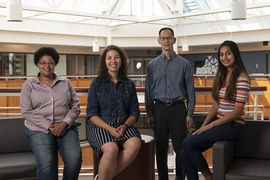
Taking machine thinking out of the black box
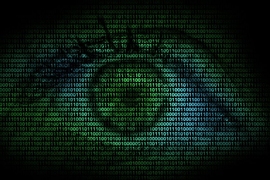
Peering into neural networks
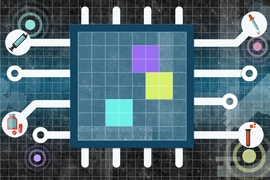
Artificial intelligence model “learns” from patient data to make cancer treatment less toxic
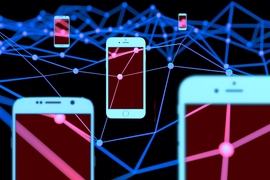
Revolutionizing everyday products with artificial intelligence
Previous item Next item
More MIT News
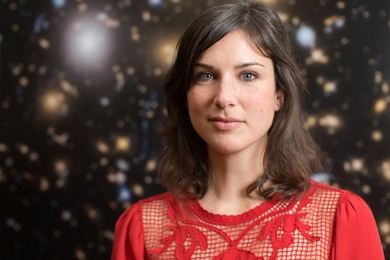
Erin Kara named Edgerton Award winner
Read full story →
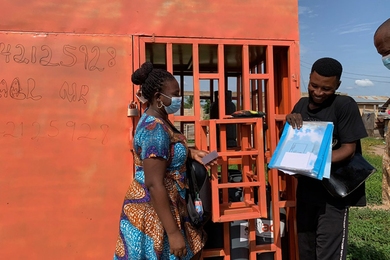
Q&A: Claire Walsh on how J-PAL’s King Climate Action Initiative tackles the twin climate and poverty crises
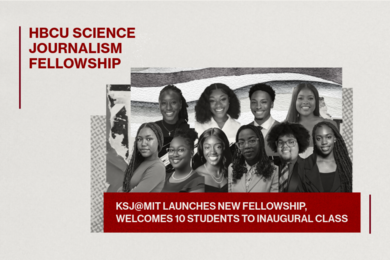
Knight Science Journalism Program launches HBCU Science Journalism Fellowship
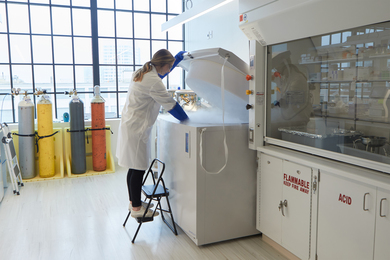
A home where world-changing innovations take flight
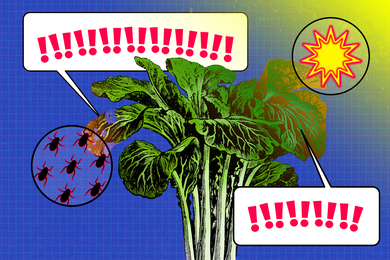
Plant sensors could act as an early warning system for farmers
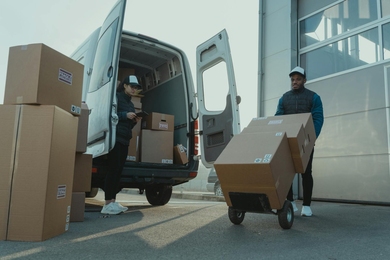
3 Questions: Enhancing last-mile logistics with machine learning
- More news on MIT News homepage →
Massachusetts Institute of Technology 77 Massachusetts Avenue, Cambridge, MA, USA
- Map (opens in new window)
- Events (opens in new window)
- People (opens in new window)
- Careers (opens in new window)
- Accessibility
- Social Media Hub
- MIT on Facebook
- MIT on YouTube
- MIT on Instagram

IMAGES
VIDEO
COMMENTS
"This is intelligence in action," Levin wrote, "the ability to reach a particular goal or solve a problem by undertaking new steps in the face of changing circumstances."
Cognitive abilities. As distinct cognitive abilities, we focus on the following traits and properties. Few-shot problem-solving. Few-shot problem-solving is the ability to solve unknown problems ...
Problem solving occurs when an organism or an artificial intelligence system needs to move. from a given state to a desired goal state. Problem solving activities get students more involved. in ...
It should be noted that there is also a body of artificial intelligence (AI) research that tackles the lifelong learning problem from a less clearly biological perspective 2,3,4,5,6,7,8,9,10.
When the system was asked to perform the task under these constraints, it used some of the same tricks used by real human brains to solve the task. For example, to get around the constraints, the artificial systems started to develop hubs - highly connected nodes that act as conduits for passing information across the network.
1. Introduction. Since the founding Dartmouth Summer Research Project in 1956 (McCarthy et al., 1955), the field of artificial intelligence (AI) has attained many impressive achievements.The potential of automated reasoning, problem solving, and machine learning has been unleashed through a wealth of different algorithms, methods, and tools (Russell and Norvig, 2021).
Scientists have shown that placing physical constraints on an artificially-intelligent system -- in much the same way that the human brain has to develop and operate within physical and biological ...
1. Introduction. Complex problem-solving involves engaging with problems that are dynamic, could have multiple solutions, and usually require multidisciplinary teamwork (Dörner, 1980).These are "problems that resemble real-life situations" (Kunze et al., 2018, p. 3).As society has become more globalized and interconnected, the problems generated have become more complex and intractable ...
Illusions of understanding that arise from an overreliance on AI in science (Fig. 1) cannot be overcome by using more sophisticated AI models or by preventing errors such as hallucinations. Rather ...
In this chapter we discuss the link between intelligence and problem-solving. To preview, we argue that the ability to solve problems is not just an aspect or feature of intelligence - it is the essence of intelligence. We briefly review evidence from psychometric research concerning the nature of individual differences in intelligence, and ...
Chamberlin (2006) defined problem solving more generally as: ―a higher-order cognitive process that requires the modulation and control of more routine or fundamental skills. Problem solving occurs when an organism or an artificial intelligence system needs to move from a given state to a desired goal state‖.
Nature Physics ( 2024) Cite this article. AI needs to develop more solid assumptions, falsifiable hypotheses, and rigorous experimentation. Recently, artificial intelligence (AI) has been ...
Considered the most complex of all intellectual functions, problem-solving has been defined as a higher-order cognitive process that requires the modulation and control of more routine or fundamental skills. Problem-solving occurs when an organism or an artificial intelligence system needs to move from a given state to the desired goal state.
4. Critical Thinking as an Applied Model for Intelligence. One definition of intelligence that directly addresses the question about intelligence and real-world problem solving comes from Nickerson (2020, p. 205): "the ability to learn, to reason well, to solve novel problems, and to deal effectively with novel problems—often unpredictable—that confront one in daily life."
Artificial intelligence ( AI) is the collective term for computer technologies and techniques that help solve complex problems by imitating the brain's ability to learn. AI helps computers recognize patterns hidden within a lot of information, solve problems and adjust to changes in processes as they happen, much faster than humans can.
Despite these advances, the problem of how a computational system can make sense of its environment continues to challenge the manifestation of autonomous intelligence by artificial cognitive systems . In a different context, this same problem—of making meaning—also presents a significant issue in the context of computational approaches to ...
Chapter 2 Problem-Solving and Artificial Intelligence In science and technology we incessantly encounter the necessity of solving problems, beginning from straightforward or well-defined tasks and ending with complex organizational and technological tasks. Hence, the question arises, what kind of problem requires the application of special ...
AI technology has grown remarkably over the past decade. Countries around the world have invested heavily in AI technology research and development. Major corporations are also applying AI technology to social problem solving; notably, IBM is actively working on their Science for Social Good initiative.
Wu and her team found that the process of identifying the ideal combination of separator algorithms to use is, in itself, a problem with an exponential number of solutions. "Separator management is a core part of every solver, but this is an underappreciated aspect of the problem space.
Problem solving occurs when an organism or an artificial intelligence system needs to move from a given state to a desired goal state. Problem solving is of crucial importance in engineering when products or processes fail, so corrective action can be taken to prevent further failures. Perhaps of more value, problem solving can be applied to a ...
Problem-solving, at its core, is the ability to identify and resolve issues, a skill that is crucial in AI. In AI, problem-solving involves the use of algorithms and models to find solutions to complex tasks. This process often requires the system to be adaptive, learn from experiences, and make decisions in uncertain conditions.
This is only one facet of the complex processes involved in cognition. Simply put, cognition is thinking, and it encompasses the processes associated with perception, knowledge, problem solving, judgment, language, and memory. Scientists who study cognition are searching for ways to understand how we integrate, organize, and utilize our ...
Artificial intelligence system uses transparent, human-like reasoning to solve problems ... TbD-net solves the visual reasoning problem by breaking it down to a chain of subtasks. The answer to each subtask is shown in heat maps highlighting the objects of interest, allowing analysts to see the network's thought process. ...