- Research article
- Open access
- Published: 15 February 2022

Determining intention, fast food consumption and their related factors among university students by using a behavior change theory
- Alireza Didarloo 1 ,
- Surur Khalili 2 ,
- Ahmad Ali Aghapour 2 ,
- Fatemeh Moghaddam-Tabrizi 3 &
- Seyed Mortaza Mousavi 4 , 5
BMC Public Health volume 22 , Article number: 314 ( 2022 ) Cite this article
19k Accesses
5 Citations
Metrics details
Today, with the advancement of science, technology and industry, people’s lifestyles such as the pattern of people’s food, have changed from traditional foods to fast foods. The aim of this survey was to examine and identify factors influencing intent to use fast foods and behavior of fast food intake among students based on the theory of planned behavior (TPB).
A cross-sectional study was conducted among 229 university students. The study sample was selected and entered to the study using stratified random sampling method. Data were collected using a four-part questionnaire including Participants’ characteristics, knowledge, the TPB variables, and fast food consumption behavior. The study data were analyzed in SPSS software (version 16.0) using descriptive statistics (frequencies, Means, and Standard Deviation) and inferential statistics (t-test, Chi-square, correlation coefficient and multiple regressions).
The monthly frequency of fast food consumption among students was reported 2.7 times. The TPB explained 35, 23% variance of intent to use fast food and behavior of fast food intake, respectively. Among the TPB variables, knowledge ( r = .340, p < 0.001) and subjective norm ( r = .318, p < 0.001) were known as important predictors of intention to consume fast foods - In addition, based on regression analyses, intention ( r = .215, p < 0.05), perceived behavioral control ( r = .205, p < 0.05), and knowledge ( r = .127, p < 0.05) were related to fast food consumption, and these relationships were statistically significant.
Conclusions
The current study showed that the TPB is a good theory in predicting intent to use fast food and the actual behavior. It is supposed that health educators use from the present study results in designing appropriate interventions to improve nutritional status of students.
Peer Review reports
Over the past few decades, non-communicable diseases such as eczema, asthma, cancer, type 2 diabetes, obesity, etc. have increased in developed countries [ 1 , 2 ]. Also, these diseases are more prevalent with increasing urbanization in developing countries [ 3 , 4 , 5 ]. The occurrence of many non-communicable diseases is related to diet [ 6 ]. Food habits are rooted from cultural, environmental, economic, social and religious factors. An effective factor in the development of chronic diseases is lifestyle, dietary patterns and habits. Inappropriate food habits and unhealthy environments have increased the incidence of non-communicable diseases in the world [ 7 , 8 ].
Many developing countries with a tendency towards Western dietary culture go away from traditional and local diets [ 6 ]. Healthy foods with nutrients have been replaced by new foods called fast foods [ 9 ]. Fast food is the food prepared and consumed outside and often in fast food restaurants [ 10 ]. Fast food is often highly processed and prepared in an industrial fashion, i.e., with standard ingredients and methodical and standardized cooking and production methods [ 10 ]. In fast food, vitamins, minerals, fiber and amino acids are low or absent but energy is high [ 9 ]. Fast food consumption has increased dramatically in the last 30 years in European and American countries [ 11 ].
Previous studies reported patterns of inappropriate and harmful food consumption in Iranian children and adolescents [ 12 , 13 ]. Most fast food customers are adolescents and youth, as these products are quickly and easily produced and relatively inexpensive [ 14 ]. One Iranian study shows that 51% of children eat inappropriate snacks and drinks over a week [ 15 ]. It is also reported that adults today consume fast food more than previous generations [ 16 ]. Faqih and Anousheh reported that 20% of adolescents and 10% of adults consumed sandwiches 3 or more times a week [ 17 ].
According to two studies, children and adolescents who consume fast food have received more energy, saturated fat, sodium, carbohydrates and more sugar than their peers, but they have less fiber, vitamin A and C, and less fruit and vegetables [ 18 , 19 ]. Also, because of the use of oils to fry these foods at high temperatures, these types of foods may contain toxic and inappropriate substances that threaten the health of consumers [ 20 ].
In a study in the United States on young people between 13 and 17 years old, it was found that there is a significant relationship between weight gain and obesity with pre-prepared foods [ 21 ]. According to the Center for Disease Control and Prevention (2007–2008), 17% of children aged 2 to 19 years and 34% of those aged 20 years and older were obese [ 22 ]. Many Health problems were caused by human health behavior(e.g. exercising regularly, eating a balanced diet, and obtaining necessary inoculations, etc.) and studying behavior change theories/models provides a good insight into the causes and ways of preventing these problems [ 23 ]. One of these theories is the Theory of Planned Behavior (TPB), which is a developed form of the Theory of reasoned action (TRA), and describes a healthy behavior that is not fully under the control of a person [ 24 ]. This theory can successfully predict eating habits and behaviors, and recently this theory has received considerable attention from researchers in identifying norms and beliefs related to the use of fast food [ 25 ].
Based on the TPB, intention to conduct a behavior with following three concepts is controlled: 1. Attitudes (positive and negative evaluation of a behavior), 2. Subjective norms (social pressure received from peers, family, health care providers for doing or not doing a given health behavior), 3. Perceived behavior control (This refers to a person’s perception of the ease or difficulty of performing the behavior of interest.) [ 26 , 27 , 28 ].
The TPB has been tested on different behaviors such as healthy food choice [ 24 ], physical activity [ 29 ], and fast food consumption [ 30 ]. For instance, the study conducted by Seo et al. showed that fast food consumption behavior was significantly associated with behavioral intention and perceived behavioral control. In addition, their findings highlighted that behavioral intention was significantly related to subjective norm and perceived behavioral control [ 28 ].
Given that our study population has cultural diversity and nutritional behaviors different from the societies of other countries and According to the mentioned materials, the researchers decided to test the study with the aim of investigating and explaining the intention and behavior of fast food consumption and their related factors based on the TPB among Urmia University of Medical Sciences students. The results of this study will increase the awareness and knowledge about fast food and, in addition, its results can be used in research, hospitals and healthcare settings.
This cross-sectional study was performed on students of Urmia University of Medical Sciences located in northwest Iran in academic year of 2018–2019. The inclusion criteria for the study are females and males who studied at Urmia University of Medical Sciences, and students’ voluntary participation in the study and obtaining written consent from the students and University principals for the students’ participation in the study. The lack of willingness to continue participating in the study and not signing the informed consent form were considered as exclusion criteria.
According to the results of the study of Yar Mohammadi and et al. [ 31 ], with a 95% confidence interval and an error of 0.05, using the formula for estimating the proportion in society, taking into account the 10% drop rate, sample size was estimated 330students. A randomized stratified sampling method was used to select the study samples. The study sample was randomly selected from each of the strata based on the share of the total sample.
Questionnaire
The data gathering tool in this study was a self-reported questionnaire (Additional file 1 ), which was designed according to the existing measures in scientific literature [ 32 , 33 , 34 ]. The study instrument was translated from English to Persian using a standard forward-backward translation technique [ 35 ]. The original instrument was translated by a bilingual specialist. The Persian version was then retranslated into English by two independent bilingual professionals to assess retention of the original meaning in the source language. Subsequently, translators worked separately in the translation process and then prepared the final version of the Persian translation. Content validity of The Persian version of questionnaire was evaluated by a panel of experts such as 3 nutrition specialists, 3 health education specialists, and 2 instrument designers. After receiving their comments, crucial revisions were conducted in the study tool. Finally, validity of the study instrument was confirmed. The present questionnaire including four following sections:
General characteristics
The first part contains personal information such as age, gender, weight, height, field of study, student education, father’s education, mother’s education, father job, mother’s job, ethnicity, marital status, participating in nutrition educational classes, students’ monthly income, family’s monthly income, housing status, information resource for healthy nutrition.
Constructs of the TPB
The second part contains questions about the constructs of the theory of planned behavior (attitude, subjective norms, perceived behavioral control and behavioral intention). In general, attitudes, subjective norm and perceived behavioral control of students were measured using indirect items. The internal reliability of all subscales of the TPB variables was good, with a Cronbach’s alpha of 0.852.
Attitude toward fast food use
The attitude of the people was evaluated using 28 indirect items (14 items of behavioral beliefs, 14 items of expectations evaluation) based on five-point the Likert scale (from strongly agree to strongly disagree) or (from very important to not at all important), and the score of each item varied from 1 to 5. The minimum and maximum score for the attitude subscale was 14 and 350, respectively. The internal reliability of attitude subscale was good, with a Cronbach’s alpha of 0.778.
Subjective norm
Subjective norms of students were measured by 10 indirect items (5 items of normative beliefs, 5 items of motivation to comply) based on five-point the Likert scale (from strongly agree to strongly disagree) or (from very important to not at all important), and the score of each item varied from 1 to 5. The minimum and maximum score for the subjective norm subscale was 5 and 125, respectively. The internal reliability of subjective norm subscale was good, with a Cronbach’s alpha of 0.726.
Perceived behavioral control
Perceived behavioral control were measured by 18 indirect items (9 items of control beliefs, 9 items of perceive power) based on five-point the Likert scale (from strongly agree to strongly disagree) or (from extremely difficult to extremely easy), and the score of each item varied from 1 to 5. The minimum and maximum score for the perceived behavioral control subscale was 9 and 225, respectively. The internal reliability of subscale of perceived behavioral control was good, with a Cronbach’s alpha of 0.815.
Behavioral intention
Behavioral intention was evaluated by 8 items based on five-point the Likert scale (from strongly agree to strongly disagree), and the score of each item varied from 1 to 5. The minimum and maximum score for the Behavioral intention subscale was 8 and 40, respectively. The internal reliability of behavioral intention subscale was good, with a Cronbach’s alpha of 0.821.
Knowledge of participants
And the third and fourth parts are items related to food knowledge and fast food behavior. Students’ knowledge of fast food was evaluated by 14 items, and the score of each item varied from 0 to 2. The minimum and maximum score for the knowledge subscale was 0 and 28, respectively. The internal reliability of students’ knowledge was good, with a Cronbach’s alpha of 0.783.
Fast food use
Students’ fast food consumption was assessed by frequency of use in a past month. The term “Fast food” was defined as hamburgers, doughnuts, hot dog, snack, pizza, fried chicken and fried potatoes. The frequency of fast food use was analyzed for each food category.
Statistical analyses
All statistical analyzes were performed using SPSS 16.0 software. Descriptive statistics methods such as frequencies, means and standard deviations were used along with independent t and χ2 tests. Pearson correlation test was used to investigate the relationship between TPB variables with intent to use fast food and the real use of fast food. Multiple regressions were used for further analysis.
Descriptives
A total of 330 students were selected and recruited to the study, but some subjects (31 samples) were excluded from the study due to incomplete questionnaires (21cases), and no return of questionnaires (10 cases). Statistical analyses were performed on 229 students. Of these, 28.4% of the students were males and 71.6% were females. The results of the study showed that the average age for all the students was 22.10 ± 3.30 (the average age for male and female sexes were 22.66 ± 4.47 and 21.84 ± 2.50, respectively). The two sexes differed in terms of BMI, so that the mean of BMI was higher in boy students than in girls, and this difference was statistically significant. Almost more than 72% of the students had normal weight, and 28% of subjects were in other weights. Approximately 20.51, 54.50, 79.77% of the students reported the professional doctoral degree, Azeri ethnicity and single.
In addition, findings revealed that 64.90% of the participants lived in the dormitory, and 35.10% of them lived in personal or rental housing. The most common level of education for father (37.10%) and mother (44.10%) of students was diploma. Nearly, 46.50% of students gained food information (especially fast food) from health care providers, while 53.50% of them received their food information from other sources. Most students had zero monthly income, but 61.61% of the students reported their family’s monthly income more than 50 million Rials and 38.39% of their family had income lower than the mentioned amount. Table 1 provides detailed information on students’ characteristics.
Main analysis
Table 2 presents the mean score of knowledge and variables of the study-related theoretical framework. As the mean score of subjective norm, perceived behavioral control and behavioral intention in male students compared to female students was high, but those were not significant statistically( p > 0.05).
Some variables of the TPB were significantly correlated with each other ( P < 0.01, Table 3 ). In particular, fast food consumption behavior was highly ( r = 0.382) correlated with behavioral intention. Multiple regression analyses were conducted to determine the relative importance of the variables of the TPB to behavioral intention and fast food consumption behavior (Tables 4 and 5 ). In these analyzes, when the attitude toward behavior, subjective norms, and perceived control was regressed to behavioral intention, the model was very significant ( P = 0.000) and explained 0.347 of variance of behavioral intention. While attitude and perceived behavioral control were not significant, the subjective norms and students’ knowledge were significantly related to the intention to eat fast food. It seems that subjective norms and students’ knowledge to be the most important predictors of behavioral intent. Table 4 shows more information about predictors of behavioral intention.
The second model, using fast food consumption as a dependent variable, was also very significant ( P = 0.000), and explained nearly a quarter of the variance (0.231) of fast food consumption. Both behavioral intention and perceived behavioral control were significantly associated with fast food consumption, of which behavioral intention appeared to be more important. Table 5 presents more information about predictors of fast food consumption.
This investigation was conducted on a sample of university students to assess the status of their fast-food consumption. It also examined the factors affecting behavioral intent and fast food consumption by applying the TPB. The results of the present study showed that students consumed fast food at an average of 2.7 times a month. Fast food in male students was often reported more than female students. A study on fast food consumption among students at Daejeon School reported monthly frequencies of fast food types: 2.7 for burgers, 2.1 for French fries, 1.8 for chicken [ 24 ]. Results of Kim study and other similar researches [ 31 , 36 ] approximately were in line with findings of the present study.
Given that most men do not have the time and skill to make traditional foods, and because of a lot of work, they prefer to turn to fast-foods, and so they are more likely to use fast foods. Meanwhile, the results of some studies indicate that most women are not very happy from high weight and are more likely to reduce their weight [ 37 ]. Therefore women do not have a positive attitude toward obesogenic foods compared to men [ 38 ], which can be a reason for consuming less fast food among women. Instead, the results of a study done by Seo et al. In Korea indicated that fast food consumption among high school students was 4.05 times a month and this consumption was reported among boys more than girls [ 28 ]. The results of the Korean study were contrary to the results of the study, meaning that fast food in Korean samples was more than Iranian. The reason for this difference can be traced to factors such as sample size, cultural, social, and economic characteristics of the samples.
Performing and not performing the behavior by a person is a function of several factors based on the theory of planned behavior. One of these factors is the person’s intention and desire to do the behavior. Behavioral intention itself is also affected by factors such as attitude, students’ knowledge, social pressure, and perceived behavioral control. In the present study, based on linear regression analysis, students’ knowledge and social pressure were both related to their intention and consume fast foods. That is, students who had the necessary information about nutrition, especially fast foods, had a high intent to choose and consume foods.
Several studies have examined the relationship between knowledge of foods and their contents and attitudes toward fast foods and processed foods or relationship between attitudes toward food additives and food choice behavior [ 39 , 40 , 41 , 42 ]. Aoki et al. [ 39 ] found that information about food and its contents positively or negatively affects attitudes and intentions towards food. They pointed out that food information was important for consumers in choosing food. Back and Lee [ 43 ] found that consumers had inadequate and incorrect information about foods, which could affect their attitudes or intent. These studies suggest that providing more information about foods and their compounds can help them to improve their attitude towards foods. Therefore, training on the performance, benefits and safety of foods, including positive and negative sides, should prevent misunderstandings about food supplements and reduce food safety concerns.
The findings of the present investigation showed that subjective norms of students were effective on intent to use fast foods. Friends had the most impact on the plan to eat fast foods, as expected. In addition, the normative beliefs of students were also more positive for friends than family and teachers. This conclusion suggests that most training programs should focus on their friends as a critical group that may affect intent to use fast foods.
Results of some previous studies were similar to findings of the current study. One study conducted by Mirkarimi et al. highlighted that subjective norms had the main role on students’ intent to use fast foods [ 44 ]. In the other words, they found that behavioral intention was affected by subjective norms. In addition, the study of Yarmohammadi and et al. showed that subjective norms predict intention and behavior [ 31 ].
In this study, TPB demonstrated to be a sound conceptual framework for explaining closely35% of the variance in students’ behavioral intention to consume fast-food. Among the TPB variables, subjective norm and knowledge of students were the most important predictors of intention to use fast foods. These findings are consistent with other results that identify that subjective norms have a significant effect on consuming fruits and vegetables [ 45 ]. In study of Lynn Fudge, Path analysis highlighted that TPB explained adolescent fast-food behavioral intention to consume fast food. The model identified subjective norms had the strongest relationship with adolescent behavioral intention to consume fast food [ 46 ].
The results of this study showed that the attitude toward fast food behavior did not predict intent and the behavior. However, some studies have reported contradictory findings with the study. For example, the findings of Stefanie and Chery’s study showed that attitude was a predictor for intent to use healthy nutrition [ 47 ]. Yarmohammadi and colleagues stated in their study that attitude was the most important predictor of behavioral intent [ 31 ]. In the study of determinants of fast food intake, Dunn et al. has identified attitude as a predictor of the intent of fast food consumption [ 32 ]. The results of studies by Seo et al., Ebadi et al., along with the findings of this study, showed that attitude toward fast food consumption is not significantly related to behavioral intention [ 28 , 48 ]. Based on the findings of the current study, fast-food consumption of students was also influenced by some the TPB variables. Multiple linear regression analyses revealed that the constructs of the TPB explained fast food use behaviors with R-squared (R 2 ) of 0.23. In these analyses, intention, perceived behavioral control, and knowledge were known as effective factors on fast-food consumption. Among the TPB constructs, behavioral intention was the most important predictor of fast-food consumption. The intention plays a fundamental role in the theory of planned behavior. The intentions include motivational factors that influence behavior and show how much people want to behave and how hard they try to do the behavior [ 49 ]. In study Ebadi et al., regression analysis showed the intention as a predictor of fast food consumption behavior [ 48 ]. In studies of Stefanie et al. and Seo et al., has reported intention as correlate of the behavior [ 28 , 47 ]. All these studies confirmed and supported this part of our study findings. In addition, the results indicated that perceived behavioral control directly influenced the behavior of fast-food consumption. Some investigations confirmed this portion of our results. For instance, the results of Dunn et al. showed that perceived behavioral control (PBC) and intent predicted the behavior of fast food consumption [ 32 ]. Also, in the study of Seo et al., regression analysis showed that fast food consumption behavior was correlated with perceived behavioral control [ 28 ]. Yarmohammadi et al. found that in predicting behavior, perceived behavioral control along with intention could predict 6% of behavior [ 31 ]. Although this study provides valuable knowledge regarding the relationships between behavioral intent and TPB variables, this study, like other studies, has a number of limitations. First, a cross-sectional study was used to examine the relationship between the variables. Due to the fact that in cross-sectional studies, all data are collected in a period of time, as a result, these studies do not have the necessary ability to examine the cause-and-effect relationships between variables. Second, the results of this type of study can only be generalized to populations with similar characteristics and have no generalizability beyond that. Third, since the data of this study were collected using the self-report questionnaire, the respondents may have errors and bias in completing the questionnaire and this can affect the results of the study.
In sum, this study was conducted to identify factors influencing intention and behavior of fast-food consumption among students by using the theory of planned behavior. The findings revealed that changeability of students’ intention to use fast food and their real behavior is dependent on the TPB variables. As this theoretical framework explained 35, 23% of intent to consume fast-foods and fast-food consumption, respectively. Among the TPB constructs, knowledge and subjective norm were known as the most important predictors of intention to use fast foods. In addition, the results indicated that intention and perceived behavioral control were the most important factors influencing consumption of fast foods among participants. It is imperative that health educators and promoters use these results in designing suitable educational interventions to improve people’s nutritional behavior.
Availability of data and materials
The datasets generated during and/or analyzed during the current study are not publicly available due to confidentiality of data and subsequent research, but are available from the corresponding author on reasonable request.
Abbreviations
Theory of Planned Behavior
Theory of Reasoned Action
Statistical Package for Social Sciences
Body Mass Index
ISAAC Steering Committee. Worldwide variation in prevalence of symptoms of asthma, allergic rhino conjunctivitis, and atopic eczema: ISAAC. Lancet. 1998;351:1225–32.
Google Scholar
Anonymous. Variations in the prevalence of respiratory symptoms, self-reported asthma attacks, and use of asthma medication in the European Community respiratory health survey (ECRHS). Eur Respir J. 1996;9:687–95.
Hijazi N, Abalkhail B, Seaton A. Diet and childhood asthma in a society in transition: a study in urban and rural Saudi Arabia. Thorax. 2000;55:775–9.
CAS PubMed PubMed Central Google Scholar
Asher MI, Montefort S, Björkstén B, et al. Worldwide time trends in the prevalence of symptoms of asthma, allergic rhinoconjunctivitis, and eczema in childhood: ISAAC phases one and three repeat multicountry cross-sectional surveys. Lancet. 2006;368:733–43.
PubMed Google Scholar
Beaglehole R, Bonita R, Horton R, et al. Priority actions for the non-communicable disease crisis. Lancet. 2011;377:1438–47.
Devereux G. The increase in the prevalence of asthma and allergy: food for thought. Nat Rev Immunol. 2006;6:869–74.
CAS PubMed Google Scholar
Nazari B, Asgari S, Sarrafzadegan N, et al. Evaluation and types of fatty acids in some of the most consumed foods in Iran. J Isfahan Med School. 2010;27(99):526–34.
Word Health Organization (WHO). Diet, nutrition and the prevention of chronic diseases report of a joint WHO/FAO expert consultation. Geneva: WHO.2003. Available at: http://whqlibdoc.who.int/publications/9241590416.pdf . [Accessed Jun 21, 2011].
Ashakiran S, Deepthi R. Fast foods and their impact on health. JKIMSU. 2012;1(2):7–15.
Vaida N. Prevalence of fast food intake among urban adolescent students. IJES. 2013;2(1):353–9.
Bowman SA, Vinyard BT. Fast food consumption of US adults: impact on energy and nutrient intakes and overweight status. J Am Coll Nutr. 2004;23(2):163–8.
Abdollahi M, Amini M, Kianfar H, et al. Qualitative study on nutritional knowledge of primary-school children and mothers in Tehran. EMHJ-Eastern Mediterranean Health Journal. 2008;14(1):82–9.
Shahanjarini A, Shojaezadeh D, Majdzadeh R, et al. Application of an integrative approach to identify determinants of junk food consumption among female adolescents. Iran J Nutr Sci Food Technol. 2009;4(2):61–70.
Lee JS. A comparative study on fast food consumption patterns classified by age in Busan. Korean J Commun Nutr. 2007;12(5):534–44.
Dehdari T, Mergen T. A survey of factors associated with soft drink consumption among secondary school students in Farooj city, 2010. J Jahrom Univ Med Sci. 2012;9(4):33–9.
Brownell KD. Does a" toxic" environment make obesity inevitable? Obssity Manage. 2005;1(2):52–5.
Faghih A, Anousheh M. Evaluating some of the feeding behaviors in obese patients visiting affiliating health centers. Hormozgan Med J. 2008;12(1):53–60.
Paeratakul S, Ferdinand DP, Champagne CM, et al. Fast-food consumption among US adults and children: dietary and nutrient intake profile. J Am Diet Assoc. 2003;103(10):1332–8.
Timperio AF, Ball K, Roberts R, et al. Children’s takeaway and fast-food intakes: associations with the neighbourhood food environment. Public Health Nutr. 2009;12(10):1960–4.
Pour Mahmoudi A, Akbar TabarTuri M, Pour Samad A, et al. Determination of peroxide in the oil consumed in restaurants and snack bar Yasuj. J Knowledge. 2008;13(1):116–23 [In Persian].
SadrizadehYeganeh H, AlaviNaein A, DorostiMotlagh A, et al. Obesity is associated with certain feeding behaviors in high school girls in Kerman. Payesh Quarterly Summer. 2007;6(3):193–9 [In Persian].
Greger N, Edwin CM. Obesity: a pediatric epidemic. Pediatr Ann. 2001;30(11):694–700.
Ghaffari M, Gharghani ZG, Mehrabi Y, et al. Premarital sexual intercourse-related individual factors among Iranian adolescents: a qualitative study. Iran Red Crescent Med J. 2016;18(2):e21220.
PubMed PubMed Central Google Scholar
Kim KW, Ahn Y, Kim HM. Fast food consumption and related factors among university students in Daejeon. Korean J Commun Nutr. 2004;9(1):47–57.
Harris KM, Gordon-Larsen P, Chantala K, et al. Longitudinal trends in race/ethnic disparities in leading health indicators from adolescence to young adulthood. Arch Pediatr Adolesc Med. 2006;160(1):74–81.
Ajzen I. The theory of planned behavior. Organ Behav Hum Decis Process. 1991;50(2):179–211.
Branscum P, Sharma M. Using the theory of planned behavior to predict two types of snack food consumption among Midwestern upper elementary children: implications for practice. Int Quarterly Commun Health Educ. 2011;32(1):41–55.
Seo H-s, Lee S-K, Nam S. Factors influencing fast food consumption behaviors of middle-school students in Seoul: an application of theory of planned behaviors. Nutr Res Pract. 2011;5(2):169–78.
Hewitt AM, Stephens C. Healthy eating among 10-13-year-old New Zealand children: understanding choice using the theory of planned behavior and the role of parental influence. Psychol Health Med. 2007;12:526–35.
Didarloo A, Shojaeizadeh D, EftekharArdebili H, et al. Factors influencing physical activity behavior among Iranian women with type 2 diabetes using the extended theory of reasoned action. Diabetes Metab J. 2011;35(5):513–22.
Yarmohammai P, Sharirad GH, Azadbakht L, et al. Assessing predictors of behavior of high school students in Isfahan on fast food consumption using theory of planned behavior. Journal of Health Syst Res. 2011;7(4):449–59.
Dunn K, Mohr P, Wilson C, et al. Determinants of fast food consumption: an application of the theory of planned behavior. Appetite. 2011;23(57):349–57.
Dunn KI, Mohr PB, Wilson CJ, Wittert GA. Beliefs about fast food in Australia: a qualitative analysis. Appetite. 2008;51(2):331–4.
Denney-Wilson E, Crawford D, Dobbins T, Hardy L, Okely AD. Influences on consumption of soft drinks and fast foods in adolescents. Asia Pac J Clin Nutr. 2009;18(3):447–52.
Brisling RW. The wording and translation of research instruments. In: Loner WJ, Berry JW, editors. Field Methods in Cross-cultural Research. Beverly Hills, CA: Sage; 1986. p. 134–64.
Sanaye S, Azarghashb A, Derisi M, et al. A survey on knowledge and attitude of students of ShahidBeheshti University of medical sciences toward fast food. Scientific J Med Council Islamic Republic Iran. 2016;34(1):23–30.
Driskell JA, Meckna BR, Scales NE. Differences exist in the eating habits of university men and women at fast-food restaurants. J Nutr. 2006;26(10):524–30.
CAS Google Scholar
Morse KL, Driskell JA. Observed sex differences in fast-food consumption and nutrition self-assessments and beliefs of college students. Sci Direct J Nutr Res. 2009;29(3):173–9.
Aoki K, Shen J, Saijo T. Consumer reaction to information on food additives: evidence from an eating experiment and a field survey. J Econ Behav Organ. 2010;73:433–8.
Stern T, Haas R, Meixner O. Consumer acceptance of wood-based food additives. Br Food J. 2009;11:179–95.
Kim H, Kim M. Consumers' awareness of the risk elements associated with foods and information search behavior regarding food safety. J East Asian Soc Diet Life. 2009;19:116–29.
Seo S, Kim OY, Shim S. Using the theory of planned behavior to determine factors influencing processed foods consumption behavior. Nutr Res Pract. 2014;8(3):327–35.
Back BS, Lee YH. Consumer's awareness and policies directions on food additives-focusing on consumer information. J Consum Stud. 2006;17:133–50.
Mirkarimi K, Mansourian M, Kabir MJ, et al. Fast food consumption behaviors in high-school students based on the theory of planned behavior (TPB). Int J Pediatr. 2016;4(7):2131–42.
Murnaghan DA, Blanchard CM, Rodgers WM, et al. Predictors of physical activity, healthy eating and being smoke-free in teens: a theory of planned behavior approach. Psychol Health. 2010;25:925–41. https://doi.org/10.1080/08870440902866894 .
Article PubMed Google Scholar
Julie Lynn Fudge. Explaining adolescent behavior intention to consume fast food using the theory of planned behavior. Dissertation Submitted to the Graduate Faculty Of the North Dakota State University Of Agriculture and Applied Science. lib.ndsu.nodak.edu. 2013.
Stefanie A, Chery S. Applying the theory of planned behavior to healthy eating behaviors in urban native American youth. Int J Behav Nutr Phys Act. 2006;30(3):1–10.
Ebadi L, Rakhshanderou S, Ghaffari M. Determinants of fast food consumption among students of Tehran: application of planned behavior theory. Int J Pediatr. 2018;6(10):8307–16.
Pender NJ, Murdaugh C, Parsons MA. Health promotion in nursing practice. 4th edition. Upper Saddle River, NJ: Prentice-Hall Health Inc; 2002. p. 250–5.
Download references
Acknowledgements
The article authors hereby express their gratitude to Vice Chancellors for Research of Urmia University of Medical Sciences and Education Department for supporting this study.
This study is supported by Urmia University of Medical Science, grant number(No: 2017–2323) .
Author information
Authors and affiliations.
Social Determinants of Health Research Center, Clinical Research Institute, Urmia University of Medical Sciences, The Province of Western Azarbaijan, Urmia, 5756115198, Iran
Alireza Didarloo
Faculty of Health, Urmia University of Medical Sciences, the Province of Western Azarbaijan, Urmia, 5756115198, Iran
Surur Khalili & Ahmad Ali Aghapour
Reproductive Health Research Center, Urmia University of Medical Sciences, the Province of Western Azarbaijan, Urmia, 5756115198, Iran
Fatemeh Moghaddam-Tabrizi
Faculty of Paramedical Sciences, Urmia University of Medical Sciences, the Province of Western Azarbaijan, Urmia, 5756115198, Iran
Seyed Mortaza Mousavi
Department of Paramedical Science, School of Paramedical Sciences, Urmia University of Medical Sciences, Urmia, Iran
You can also search for this author in PubMed Google Scholar
Contributions
All authors contribute in conceive, design of this study. A.D, S.K, A.A,FTM and S.M contributed to the design and implementation of the research, to the analysis of the results and to the writing of the manuscript. All authors revised the manuscript critically for important intellectual content and read and approved the final manuscript.
Corresponding author
Correspondence to Seyed Mortaza Mousavi .
Ethics declarations
Ethics approval and consent to participate.
Research has been presented in the ethics committee of Urmia University of Medical Sciences and has received the code of ethics (IR. UMSU.REC.1397.43). written informed consent was obtained from all participants in this study, and all provisions of the Helsinki Statement on Research Ethics were considered.
Consent for publication
Not applicable.
Competing interests
The authors declared no conflict of interest.
Additional information
Publisher’s note.
Springer Nature remains neutral with regard to jurisdictional claims in published maps and institutional affiliations.
Supplementary Information
Additional file 1..
The questionnire used in the study to collect the data. The first part of the questionnaire included General characteristics. The second part of the questionnaire consisted of the Constructs of TPB. The third part consisted of knowledge of participants. The fourth part consisted of Fast food use.
Rights and permissions
Open Access This article is licensed under a Creative Commons Attribution 4.0 International License, which permits use, sharing, adaptation, distribution and reproduction in any medium or format, as long as you give appropriate credit to the original author(s) and the source, provide a link to the Creative Commons licence, and indicate if changes were made. The images or other third party material in this article are included in the article's Creative Commons licence, unless indicated otherwise in a credit line to the material. If material is not included in the article's Creative Commons licence and your intended use is not permitted by statutory regulation or exceeds the permitted use, you will need to obtain permission directly from the copyright holder. To view a copy of this licence, visit http://creativecommons.org/licenses/by/4.0/ . The Creative Commons Public Domain Dedication waiver ( http://creativecommons.org/publicdomain/zero/1.0/ ) applies to the data made available in this article, unless otherwise stated in a credit line to the data.
Reprints and permissions
About this article
Cite this article.
Didarloo, A., Khalili, S., Aghapour, A.A. et al. Determining intention, fast food consumption and their related factors among university students by using a behavior change theory. BMC Public Health 22 , 314 (2022). https://doi.org/10.1186/s12889-022-12696-x
Download citation
Received : 07 December 2020
Accepted : 02 February 2022
Published : 15 February 2022
DOI : https://doi.org/10.1186/s12889-022-12696-x
Share this article
Anyone you share the following link with will be able to read this content:
Sorry, a shareable link is not currently available for this article.
Provided by the Springer Nature SharedIt content-sharing initiative
- Theory of planned behavior
BMC Public Health
ISSN: 1471-2458
- Submission enquiries: [email protected]
- General enquiries: [email protected]
Thank you for visiting nature.com. You are using a browser version with limited support for CSS. To obtain the best experience, we recommend you use a more up to date browser (or turn off compatibility mode in Internet Explorer). In the meantime, to ensure continued support, we are displaying the site without styles and JavaScript.
- View all journals
- My Account Login
- Explore content
- About the journal
- Publish with us
- Sign up for alerts
- Open access
- Published: 14 March 2024
Effect of mobile food environments on fast food visits
- Bernardo García Bulle Bueno 1 ,
- Abigail L. Horn 2 , 3 nAff9 ,
- Brooke M. Bell ORCID: orcid.org/0000-0001-8772-552X 2 , 4 ,
- Mohsen Bahrami ORCID: orcid.org/0000-0002-6665-3640 1 ,
- Burçin Bozkaya ORCID: orcid.org/0000-0002-3685-4791 5 ,
- Alex Pentland 1 ,
- Kayla de la Haye 6 &
- Esteban Moro ORCID: orcid.org/0000-0003-2894-1024 1 , 7 , 8
Nature Communications volume 15 , Article number: 2291 ( 2024 ) Cite this article
3403 Accesses
73 Altmetric
Metrics details
- Interdisciplinary studies
- Risk factors
Poor diets are a leading cause of morbidity and mortality. Exposure to low-quality food environments saturated with fast food outlets is hypothesized to negatively impact diet. However, food environment research has predominantly focused on static food environments around home neighborhoods and generated mixed findings. In this work, we leverage population-scale mobility data in the U.S. to examine 62M people’s visits to food outlets and evaluate how food choice is influenced by the food environments people are exposed to as they move through their daily routines. We find that a 10% increase in exposure to fast food outlets in mobile environments increases individuals’ odds of visitation by 20%. Using our results, we simulate multiple policy strategies for intervening on food environments to reduce fast-food outlet visits. This analysis suggests that optimal interventions are informed by spatial, temporal, and behavioral features and could have 2x to 4x larger effect than traditional interventions focused on home food environments.
Similar content being viewed by others
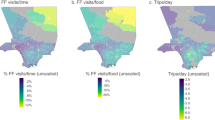
Population mobility data provides meaningful indicators of fast food intake and diet-related diseases in diverse populations
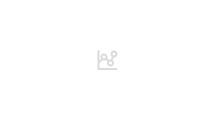
Fast food outlets, physical activity facilities, and obesity among adults: a nationwide longitudinal study from Sweden
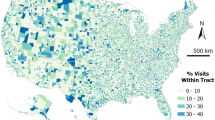
Integrating human activity into food environments can better predict cardiometabolic diseases in the United States
Introduction.
Poor diets, including the over-consumption of foods that are energy-dense but nutrient-poor, that have excess sugar and/or sodium, and that are ultra-processed, are a major cause of diet-related disease and mortality 1 , 2 , 3 . Poor diets led to 11 million deaths globally in 2017 (more than tobacco) 4 , largely due to their causal role in major chronic diseases, including obesity, type 2 diabetes, some cancers, and heart disease 5 . Exposure to, or spending time in, certain built food environments is hypothesized to impact diet and related diseases 6 , 7 . Low-quality built food environments are generally categorized into two types. Food deserts are defined as areas with low access to healthy foods (e.g., neighborhoods where a majority of residents live more than 0.5 or 1 mile from a supermarket, a key source of affordable, healthy food) 8 . Food swamps are areas saturated with food outlets selling unhealthy foods, often defined as neighborhoods that have a higher number of fast food outlets (FFO) and convenience stores, or a high ratio of these outlets relative to healthier food outlets 9 , 10 . Both of these types of low-quality food environments are frequently concentrated among low-income communities and communities of color in the US and contribute to inequities in nutritional health 11 , 12 . It is hypothesized that exposure to food swamps can nudge people to consume unhealthy food (e.g., fast food) due to a cost decrease via lower food prices or less time needed for transactions 13 , or through structural or social cues to behavior 14 . In contrast, food deserts are hypothesized to create barriers to accessing affordable healthy foods, which can lead people to make less healthy food choices that they would otherwise avoid.
To date, research into the relationship between food swamps or deserts and food choice has predominantly focused on predefined local and static food environments 15 , 16 , largely of the neighborhood around the home, with schools and workplaces to a lesser extent. While exposure to both types of food environment has been associated with increases in unhealthy eating and diet-related disease, overall, findings are mixed and predominantly null 17 , 18 , 19 , 20 . Furthermore, most of these studies have been cross-sectional and fail to establish a causal relationship between neighborhood food environments and unhealthy diets. Despite this limited evidence, there has been considerable interest from federal and local policymakers and private funders in supporting policy interventions to improve neighborhood food environments. These include investments by the U.S. Healthy Food Financing Initiative of $270 million plus $1 billion in leveraged financing to support healthy food retail in underserved neighborhoods since 2010 21 , and ‘fast food bans’ implemented in select Los Angeles neighborhoods by the city council using zoning regulation to restrict the opening of new FFO. 22 . Across numerous evaluations, these interventions have demonstrated no meaningful impact on diet quality or diet-related disease outcomes 22 , 23 , 24 , 25 , 26 , 27 . A better understanding of the relationship between food environment exposure and use, diet, and diet-related disease will be critical to designing more effective interventions to food environments.
The limited focus on residential and static food environments may be one explanation for these mixed results, given that a growing proportion of food acquisition and consumption occurs miles from our homes. For instance, Cooksey et al. 9 presented findings that food swamps predict higher rates of obesity at the neighborhood level. However, their results are weaker in neighborhoods where residents are more mobile (i.e., more residents who travel to work by car or public transport). Among US residents, food away from home (vs. foods prepared at home)–the vast majority coming from fast food and full-service restaurants–constitutes one-third of total energy intake, and one-half of food budgets 28 . Thus, a major source of exposure to and use of food environments is unlikely to be captured by existing research foci and methods. Additionally, these studies often test whether exposure to food swamps or deserts predicts nutritional health, without incorporating information on the food outlets that individuals actually visit. Given the well-documented biases of survey data to capture detailed human movement and dietary intake 29 , small studies (often <100 people) have begun to use tracking technologies to map how people move through their environment to acquire food over brief periods of time (e.g., one week) 30 , 31 , 32 , 33 , 34 . However, this has not been studied at scales large enough to capture habitual patterns of food environment exposure over extended time intervals or statistically significant effects of those food environments on peoples’ behavior. Overall, a major gap in the literature is detailed evidence of the food environments people are exposed to as they move around, both at and beyond where they sleep and work (i.e., mobile food environments), the food outlets they actually visit in these environments, and causal designs capable of investigating how mobile food environments influence diets and diet-related disease.
In this study, we use a large, privacy-preserving, population-scale mobility dataset spanning a 6-month period during 2016–2017 and 11 metropolitan areas in the US to examine peoples’ visits to food outlets (FO) and FFO in and beyond their home neighborhood and to investigate how these FFO visits are linked to features of the mobile food environments they are exposed to throughout their daily routines. Mobility data allow us to observe a diverse and heterogeneous population body 35 , 36 . They allow us to observe when and where FFO visits happen among this large, diverse population, and thus to understand the individual- and environment-level variables that condition that decision over other food choice alternatives. Moreover, they allow us to find structural, randomized shocks in people’s routines (e.g., moving, going to a government office), which we can leverage to investigate the causal effects of food environments on food outlet decisions. Our analyses focus on visits to FFOs as the key outcome because (i) greater intake of fast food, which is typically ultra-processed, low in nutrients, and energy-dense, is a well-established risk factor for poor diets, obesity, and cardiometabolic disease 2 , 37 ; and (ii) recent work has shown, using the same mobility dataset we utilize in this study, that visits to FFO are associated with self-reported fast food intake, obesity, and type 2 diabetes, thereby establishing the link between FFO visits observed in mobility data and nutritional health 38 .
Characterization of mobile food environments
Individuals in large urban areas travel or commute considerable distances 39 , indicating that for many people, the food environments they are exposed to throughout the day are not near their homes. In our dataset, we find that the median distance from home h to any place visited x is 7.83 km (Interquartile Range, IQR, [2.47–18.63 km]), see Fig. 1 . The median distance to any type of FO visited is 6.94 km (IQR [2.30 km–17.23 km]), but this varies by outlet type: the median distance to grocery stores/supermarkets is much smaller, 3.1 km (IQR [1.35 km–8.22 km]), while FFO is 6.74 km (IQR [2.50 km–16.62 km]) away (median, see Supplementary Note 1 for detailed statistical analysis of these differences). In fact, only 6.8% of the visits to FFO occur within a user’s home census tract. Thus, most fast food visits occur in food environments outside of a user’s home neighborhood.
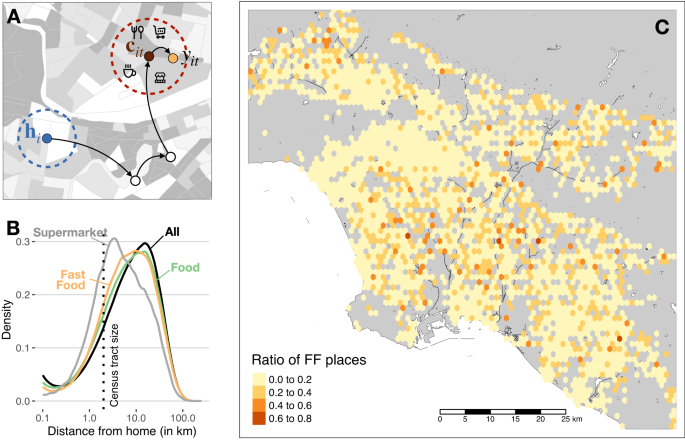
A in their daily life, users navigate the city from home h to different places until they arrive at a context c where they decide to have food in f . B Distribution of the distance from home to all visits in the city (black), all retail food outlets (green), and fast food outlets (orange). The distance traveled to food and fast food outlets is much larger than to supermarkets (gray) or the typical size of a census tract (dotted vertical line). C Heatmap of the ratio of fast food outlets ϕ in the Los Angeles metro area. The ratio is calculated within each hexagon of size ~ 1 km 2 . Icons designed by bqlqn/flaticon.com and maps were produced in R using the publicly available TIGER shapefiles from the U.S. Census Bureau 69 .
To characterize a user’s food environment at any given location x , we measure the ratio of FFO to FO within a 1 km radius, ϕ ( x ) (See “Methods” and Supplementary Note 4 for other definitions). As shown in Fig. 1 , most zones in the metro areas have small (average) values of ϕ ( x ). Because users move around the city, they are exposed to many different food environments: overall mobile exposure to food environments, the time-weighted ratio of FFO to FO that a user is exposed to in our 6-month entire period ( \({\phi }_{i}^{m}\) , see “Methods”) has a median of 14.0% (IQR [9.7–19.0%]. We also find that users’ mobile food environments are different from the food environments around their homes. Although home environments have a relatively low FFO to FO ratio (median of \({\phi }_{i}^{h}=\phi ({{{{{{{{\bf{h}}}}}}}}}_{i})\) for all users is 8.2%, IQR [0–17.5%]), we find that the correlation between mobile and home environments is small \(\rho ({\phi }_{i}^{m},\,{\phi }_{i}^{h})=0.213\pm 0.001\) across users. That correlation is slightly different across demographic groups, with residents in areas with low-income, high percentage of Black population, or large use of public transportation having a slightly stronger correlation between home and mobile environments. But in general, the correlation is small across groups ρ ≤ 0.29 (see Supplementary Fig. S19 ). In other words, the food environments that users are exposed to throughout the day are different from the ones around their homes.
The ratio of FFO to FO in users’ food environments is associated with various sociodemographic characteristics inferred from users’ home census block group. Using linear regression models for \({\phi }_{i}^{m}\) (see Fig. 2 , “Methods”, and Supplementary Table S3 for full statistical details of these models and their comparison), we find that users exposed to mobile food environments with a higher proportion of FFO (larger average \({\phi }_{i}^{m}\) ) reside in areas with a higher proportion of residents who are Black, who have long commutes, and who have lower skill jobs, and a lower proportion of residents with more educational attainment and who depend on public transportation. Similar findings have been obtained in small surveys on the use of different modes of transport to FO and FFO outlets 19 , 40 . Neighborhood-level household income is not significantly associated with any mobile food environment features. These relationships differ with users’ home food environments (see Fig. 2 ), where a greater proportion of FFO in the home neighborhood, larger \({\phi }_{i}^{h}\) , occurs in neighborhoods with lower income, higher levels of educational attainment, and shorter commuting patterns, similar to findings done by Powell et al. 41 . Despite that, we find a stronger relationship between the socio-demographic characteristics of users and their mobile food environments ( R 2 = 0.213 for \({\phi }_{i}^{m}\) ) than for their home food environments ( R 2 = 0.038 for \({\phi }_{i}^{h}\) ), see Supplementary Table S3 for more details. Similar results are found for more complex non-linear models (see Supplementary Note 7 ). This suggests that socio-demographic differences propagate slightly more strongly to people’s experienced mobile food environments than to their food spatial accessibility at home, possibly because those demographic traits slightly shape the differential access to different environments, targeted marketing, and other social and structural forces.
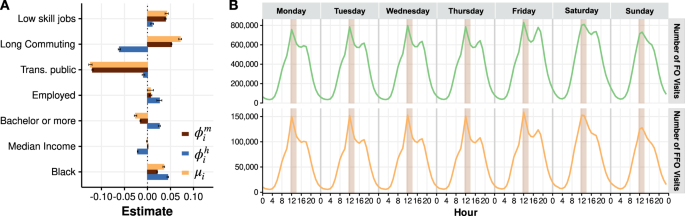
A Relationship between FF environments and socio-demographic traits. Bar shows the coefficient estimates for OLS regression models of overall mobile and home FFO environments ( \({\phi }_{i}^{m,h}\) ) and the fraction of visits ( μ i ) by user for the proportion of workers in low-skill jobs, the proportion of people with long (>45 min.) commute, the proportion of people taking public transportation for commuting, the proportion of people employed, the proportion of people with higher education level, median household income and proportion of Black people in their home census block group. Error bars are 95% confidence intervals for those coefficients. See Supplementary Table S3 for more details. B Daily patterns of the number of FO (top) and FFO (bottom) visits in our urban areas. The shaded area corresponds to the lunch observation period taken to determine the action y i t in our model ( 1 ).
Who, when, and how much people visit FFO
While \({\phi }_{i}^{h,m}\) describes the home and mobile exposure to fast food options at any given moment, we encode the actions of users at time t with the variable y i t . If individual i chooses to visit an FFO among the FO options, then we set y i t = 1. If they select a non-fast food option, then we set y i t = 0. The overall averaged fraction of FFO choices to FO options \({\mu }_{i}=\overline{{y}_{it}}\) (see “Methods”) in all environments over our observation period is heterogeneous across users with a median of 0.133, IQR [0.025, 0.273] (See Supplementary Note 1 ). That is, 13.3% of visits to FO are to an FFO (median). Our results also show that a significant proportion of users never visited FFOs (22.9%) during the 6-month period of observation. Using similar regression models as before, we find some statistically significant differences in the ratio of visits to FFO ( μ i ) across demographic groups. Similar to the results described above, individuals visit FFOs more often if they live in areas with less use of public transportation, with a higher proportion of Black residents, with longer commutes, and with less educational attainment. Similarly, income has a smaller association with the ratio of FFO visits when compared to the other demographic traits. Our results align well with the contradicting evidence of little variability in fast food intake across income levels despite consistent differences based on educational levels and race and ethnicity 42 , 43 , 44 . Additionally, we find that traditional demographic traits–race and ethnicity, type of job, income, and educational level–have a weaker association with fast food visits than characteristics related to mobility and time constraints–the use of public transportation and long commuting (see Supplementary Table S3 for full statistical details of these models and their comparison). However, it is important to note that the explanatory power of this association between μ i and socio-demographic variables is low, R 2 = 0.052. Thus, even though we find statistically significant differences, our results suggest that overall FFO visits do not meaningfully differ across different socio-demographic groups. Many types of people visit FFO in urban areas.
We also found that most food outings happen between midday (lunchtime) and the evening (dinner time), both during weekdays and weekends, see Fig. 2 . FFO visits have the same temporal pattern, with a peak of visits to fast food happening around lunchtime from Monday to Sunday.
Relationship between mobile food environments and fast food visits
To understand the effect of food environments on fast food visits, we first study the relationship between total average exposure to fast food \({\phi }_{i}^{h,m}\) and the overall observed ratio of FFO to FO visits, μ i . Many studies with small datasets have found null or contradicting results regarding the association between total exposure to FFO and fast food intake 32 , 45 . We find a positive relationship between a user’s average daily exposure to FFOs within their mobile food environment, \({\phi }_{i}^{m}\) , and overall observed ratio of visits of FO to FFO, μ i . Specifically, the correlation between these two variables is \(\rho ({\phi }_{i}^{m},\,{\mu }_{i})=0.268\pm 0.001\) . However, the correlation between FFOs within a user’s home food environment and overall ratio of visits to FFO is weaker, with \(\rho ({\phi }_{i}^{h},{\mu }_{i})=0.068\pm 0.001\) . These results are expected, given that most food outings happen far away from home, and suggest that an individual’s exposure to FFO across the day, rather than within their home environment, is a more important driver of the decision to get fast food.
To better understand what drives the association between FFO exposure and visits, we need to go beyond total exposure. Visits to FFO might be due to individual preferences, to structural or social cues received from frequent long-term exposure to environments high in FFO 14 , but also might be a direct response to the food environment where those decisions are made. A person may choose to visit different food outlets in food environments with different features. At the same time, different people exposed to the same food environment may make different decisions about which food outlet to visit. To identify the association between food environment features and FO decisions, we first design an individual analysis of each visit to a FFO. We restrict the data to FO visits during lunch hours (from 11 h30 to 14 h local time) because this time window has the highest FO and FFO visits (see Fig. 2 ). Most importantly, time constraints at this time of day could make the food environment options experienced before going to get lunch even more relevant. Indeed, we find that the relationship between action y i t and the food environment features is stronger around noon than at other times of the day (see Supplementary Note 7 ).
For decisions about food outlet visits y i t at time t , we define the context of that decision as the last place in the morning (until 11:30) where a user was observed (context c i t ), and characterize the food environment in that context by ϕ ( c i t ). Thus, the context for the food outing is the environment where the user was before the outing. To ensure that the context offered a choice between FFO and non-FFO, we focus exclusively on observations where the context c i t contains both types of outlets. We have extensively checked that our results do not depend on the precise definition of the context and of the food environment around it (see “Methods” and Supplementary Note 12 ). We use a logistic regression model to estimate the impact of user i ’s context environment on their decision to visit an FFO vs. a non-FFO at time t :
where logit −1 ( x ) = e x /(1 + e x ), α i is a fixed effect accounting for individual average preferences to visit FFO, δ t is a fixed effect accounting for daily variation, and ϕ ( c t ) is the ratio of FFO in the food environment a user was exposed to prior to the visit. By imposing a fixed factor by user, we separate the effect of individual preferences on visits to FFO from the effects of the food environment. For our regression, we only consider users that went to both fast and non-fast food outlets at lunch at least once during the 6 month observation period and where the food environment they were exposed to before lunch included both FFO and non-FFOs.
Our results, illustrated in Fig. 3 , show a large association between features of the mobile food environment and visiting an FFO. The model produces a log-odds of β = 1.87 ± 0.033 for all FO visits at lunchtime: when the context includes 10% more FFO, there is an increase in the odds to visit an FFO of ( e β ×0.1 − 1) × 100 ≃ 20%. This influence of the food environment one is exposed to before going to lunch was similar during weekdays and weekends and at different times of the day (not only during lunch hours) (see Fig. 3 and Supplementary Note 7 ). Additionally, the magnitude of the effect is largely independent of an individual’s income (see Fig. 3 ), other socio-demographic traits, distance from home (see Supplementary Note 7 ) or metro area (see Supplementary Note 10 ). Finally, as expected, we find that distance between context and lunch choice modulates this effect, although, for the majority of our data, we still get that the impact of context is positive and significant. We also see similar results for other food choices like Asian or Latin-American food (see Supplementary Note 11 ). Thus, individuals with different socio-demographic backgrounds appear to respond similarly to mobile food environments at different times.
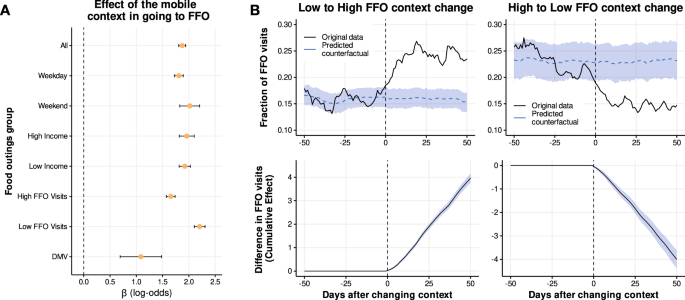
A Effect of the mobile food environment on visiting a FFO at different times, locations, or for different income or FFO visitation groups. Values show the coefficient β of the logistic regression in Eq. ( 1 ) for the food visits (outings) corresponding to the different groups. Error bars are 95% confidence intervals for those coefficients. See Supplementary Table 4 for more details. B Evolution of the fraction of FFO visits (top) and cumulative difference in FFO visits (bottom) for groups of users that change their contexts from Low to High FFO environments (left) and High to Low FFO environments. The dashed horizontal line is the predicted counterfactual for groups of users that changed their context. The shaded area corresponds to a 95% confidence interval for that predicted counterfactual.
Despite finding an effect of mobile food environments on visits to FFO, it could be that the lack of non-fast food options predominantly affects individuals when they are in a new place. This may be because they are less equipped to navigate the new food environment and identify food options they prefer. It is also plausible that a lack of options for different FO types constantly affects users who would otherwise visit FFO with a lower frequency, given their individual preferences. To address these questions, we propose a semi-causal framework using a natural experiment to investigate the relationship between habitual FFO context and FO decisions. In this experiment, we observe people who changed their quotidian context during the study. Using the time series of the different contexts before lunch and changepoint analysis, we were able to detect a small fraction of users (0.46%) that changed their habitual context before lunch within our observation period (see “Methods” and Supplementary Note 5 for details about this detection). Due to our large sample size, this small fraction had a large enough size (approx 8.5k users) to do statistical inference. Those users were split into four groups depending on whether they changed to a context with similar or different low ( ϕ < 0.13) or high ( ϕ > 0.13) exposure to fast food. We found that around 35% of the users who changed their context remained exposed to very similar food environment features before and after (High → High FFO exposure or Low → Low FFO exposure). Around 15% of the users changed their context, such that the FFO in their typical pre-lunch food environment went from Low → High exposure to FFO, and another 15% from High → Low FFO exposure. We construct the time series of the fraction of times that users visit an FFO relative to the day when they change contexts. We study the impact of the change on FFO visits using Bayesian structural time-series models (see “Methods”) by comparing the group that changed their FFO contexts (Low → High and High → Low) with the counterfactual of those that, despite changing their context, were exposed to similar FFO food environments (Low → Low and High → High, respectively, see “Methods”). Results are presented in Fig. 3 , which shows that the group that changed from Low → High FFO exposure increased their fraction of FFO visits from ~16% to ~25%. Similarly, users that changed their context from High to Low FFO exposure decreased from ~24% of FFO visits to ~15%. The counterfactual of users that changed contexts but remained exposed to food environments with similar FFO ratios maintained a similar fraction of FFO visits. We also note that this effect is statistically robust and persistent, remaining even 50 days after changing their context. In cumulative numbers, we find that users who changed their context to High (Low) FFO exposure visited FFO 4 times more (less) in 50 days than those who remained in food environments with similar FFO ratios. These results suggest that the effect of the food environment is strong even for the same users subject to different habitual contexts, and it is not only driven by visits to new places.
Finally, we sought to analyze a setting as close as possible to one where people are placed in random locations within the city. Studying food decisions under such circumstances would preclude potential estimation bias from omitted variables affecting both food preference and location in the city. For this, we propose a natural experiment looking at people who visited the Department of Motor Vehicles (DMV), the food environment they faced there, and the decision they took to get a meal. In particular, we repeat the analysis made for Eq. ( 1 ) but only when the context before lunch is the DMV. These locations are commonly visited for obtaining a driver’s license, government ID, voting, and other services. Moreover, the food environment around a DMV is unlikely to be a determining factor when choosing a DMV location, compared to other factors such as availability of appointments and distance from home/workplace. While distance to home/workplace can be influenced by latent factors such as income and even food preferences, the set of DMVs in our cities is small enough that many people need to exit their home and work neighborhoods to go there. Moreover, the time constraints caused by the scarcity of appointments help make the choice of DMV location less determined by spatial accessibility alone. Another important factor to consider was whether people going to the DMV will indeed look for lunch around it. It is possible that some of the customers of the DMV decide to eat before heading to it, or after returning to their work or home. We solved this problem by computing the probability of going to fast food given that the person went to a food outlet. This filtered out people who belong to the aforementioned group.
In our dataset, we detected 53k visits to the DMV across a 6-month period. The median distance traveled from home to the DMV was 7.62 km (IQR, [3.48–15.44 km]), greater than the median distance to FO. We consider the DMV as the context of a FO visit if such a visit occurs within 2 hours of the DMV visit. To investigate the effect of the DMV food environment on y i t , we use a logistic regression model \(\Pr ({y}_{it}=1)={{{{{{{{\rm{logit}}}}}}}}}^{-1}[{\beta }_{0}+{\hat{\alpha }}_{i}+\phi ({{{{{{{{\bf{c}}}}}}}}}_{it})]\) similar to Eq. ( 1 ) model. Since we typically have one visit to DMV per user, we model individual preference \({\hat{\alpha }}_{i}={\mu }_{i}\) as the fraction of visits to FFO of user i , and we do not include daily fixed factors. The effect of the DMV food environment is shown in Fig. 3 . We find a significant effect, although a little smaller in size than the effect of exposure to habitual contexts, with log-odds of β = 1.09 ± 0.20. This third analysis corroborates that features of food environments influence FFO visits.
Policy implications
The observed relationship between food environments with high ratios of FFOs and increased visits to FFO, specifically for mobile food environments, implies that more targeted interventions to reduce visits to FFO can be designed. Many intervention approaches have focused on improving food environment quality around the home neighborhood or in geographic regions with poor food environments, without accounting for where people more frequently visit food outlets. Notable examples include the over one billion dollars leveraged by the U.S. Healthy Food Financing Initiative to finance healthy food retail in under-served local neighborhoods 21 , and the ‘fast food ban’ implemented in 2008 in neighborhoods in South Los Angeles with a high prevalence of FFO 22 . Our findings highlight that FFO visits often take place well beyond the home neighborhood, and suggest that strategies that solely focus on geography and spatial access to food outlets in the home neighborhood (ignoring human behavior) are likely to lead to sub-optimal intervention effects. Indeed, evaluations of major policies and interventions to improve the quality of neighborhood food environments have demonstrated they have little impact on diet or diet-related diseases 25 , 46 . Here, we use the results of our observational study to identify the optimal locations to intervene in food environments to have the greatest impact on decreasing FFO visits. Specifically, these will be contexts demonstrating the highest ratios of FFO to FO, the highest frequencies of user exposure and FFO visits, and the largest observed impact of food environment features on a population’s FFO decisions. We investigate the likely effects of intervention strategies that change the ratio of FFO to FO in these optimal impact locations vs. interventions targeting locations such as neighborhood food deserts and food swamps, the traditional choice locations for intervention.
Changing the ratio of FFO to FO in an area can be accomplished by various strategies or interventions, including: decreasing the number of FFO relative to non-FFO, increasing the number of non-FFO relative to FFO, or converting a FFO into a non-FFO. Policy strategies in practice are more likely to involve one of the first two approaches, such as implemented interventions that have banned new FFO from opening in a specific area 22 , 47 , 48 , or supported non-FFO to obtain business licenses or opportunities in food retail 49 . The former has been implemented in Los Angeles 22 and in northeast England, where a blanket ban in a district led to a reduction of 15% in ϕ ( x ) 47 . The latter strategy was recently exemplified by a US White House nutrition initiative that invested in programs supporting local entrepreneurs to open healthy prepared-food outlets 50 . The last approach, converting a FFO to a non-FFO, could be seen as similar to approaches to shift the balance of the healthfulness of food items being sold at existing FFO 51 .
Assuming that our intervention I changes the context in an area Ω by δ ϕ / δ I and that users are still making their decisions according to the model in Eq. ( 1 ), the change in the number of FFO visits made immediately after being exposed to the food environment of Ω can be obtained as (see “Methods”):
where X i t = β 0 + δ t + α i + β ϕ ( c i t ). This expression shows that the effect of an intervention in an area Ω depends on three factors: (i) the susceptibility of FFO visits with respect to the availability of FO options in the area Ω, expressed through \({e}^{{X}_{it}}/{(1+{e}^{{X}_{it}})}^{2}\) and ultimately by the balance between individual preferences α i and the context ϕ ( c i t ), (ii) the number of decisions made in area Ω, expressed by the sum, and (iii) the effect of the intervention on the context ϕ , expressed by δ ϕ / δ I. For example, for the same intervention δ ϕ / δ I, we can have an area where many people go, but they have a large individual preference for FFO ( α i ≫ 0), causing the effect of the intervention to be small because they are not influenced by the food environment features. On the contrary, we can have an area that many people do not visit, but the people who do visit are highly influenced by that context’s food environment and the number of FFO around ( α i ≃ 0). An intervention in this latter area can meaningfully change a number of food outlet decisions.
To illustrate this, we consider a simple intervention in which we decrease the ratio of FFO to FO by one unit in a particular area. In this case, ϕ ( c i t ) changes by approximately δ ϕ / δ I ≃ − 1/ n Ω where n Ω is the number of FO in the area. We see this strategy as a theoretical illustration of the general concept of improving the food environment in an area by shifting the balance of FFO to healthier outlets using any of the approaches discussed above, by the same amount of effort per area. If the methodology we develop in the following were to be used, this could be implemented by setting the derivative of ϕ in our formula to be the expected derivative of the intervention. We have also extended the model to all times of the day and week to describe the full effect of the strategy (see “Methods”). Assuming that we have limited resources to change 100 food outlets, where are the areas in which our intervention maximizes its impact? Here, we compare four different strategies.
In the first strategy (Food Swamp intervention), we select the areas with the largest (average) values of ϕ , i.e., the areas where FFO predominate. For comparison and to resemble prior food desert interventions around home neighborhoods, in our second strategy (Low Food Access intervention), we select the areas that have the largest values of ϕ and are classified by the USDA as food deserts (both low-income and low-supermarket-access) 8 . The third strategy (Food Hotspots intervention) is implemented by selecting the areas where most FO visit decisions are made. However, these strategies do not incorporate individual preferences or susceptibility to food environments. Thus, in our fourth strategy (Behavior-Environment intervention), we select areas Ω as the top areas ranked by Δ FFO (Ω) in Eq. ( 2 ), which includes not only the context but also the individual preferences of people deciding in those contexts. Figure 4 A shows the relationship between the change in FFO visits Δ FFO (Ω) and the average context ϕ in the different areas (census tracts) in our cities. The figure illustrates the dependence between these two variables, but there is still significant variability. For the same average of ϕ , we have areas where the change in Δ FFO (Ω) spans two orders of magnitude. In this representation, our Food Swamp and Behavior-Environment interventions are very easy to interpret. They consist of choosing the rightmost (greatest change in context) or topmost (greatest change in FFO visits) areas, respectively. In the case of Food Swamps and Low Food Access interventions, we can see those strategies choose areas Ω in which Δ FFO (Ω) is small because not many decisions are made (Low Food Access) or because users are less affected by the FFO environment in those areas (Food Swamps). The Food Hotspots intervention chooses areas where the most FO decisions are made, but without considering whether users are affected by the FFO environment. As a result, the total effect of the four strategies is very different; see Fig. 4 B. Overall, the Behavior-Environment intervention would be 1.93 × to 3.85 × times more efficient in decreasing FFO visits than interventions that used only the FFO context where decisions are made or around the home neighborhood. In relative terms, by changing one hundred FFO (0.22% of the total), our Behavior-Environment intervention could avert around 0.56% of the visits to FFO, while other strategies could only affect 0.29% of those visits at most. If we scale these numbers to the total population, our Behavior-Environment could avert around 719k visits to FFO in 6 months, compared with only 442k at most in the other interventions. Furthermore, the impact of the interventions is predominantly independent of income (see Fig. 4 B), health conditions (see Supplementary Note 9 ), or particular city (see Supplementary Note 10 ), and thus its effect does not concentrate on particular groups or geographies. For example, we found that our Behavior-Environment intervention is still 2 × to 2.5 × more effective than the rest of the interventions at targeting decisions made by high obesity or diabetes prevalence groups (see Supplementary Note 9 ).
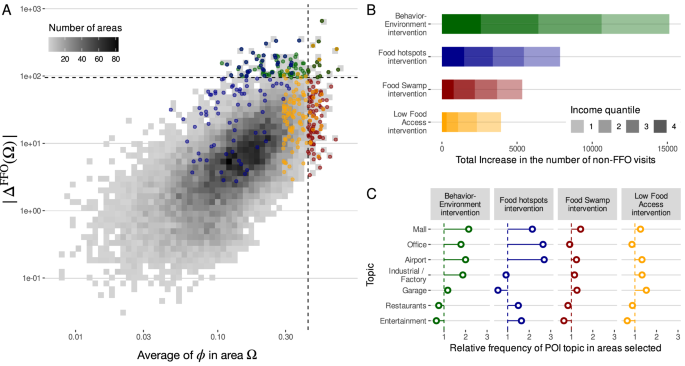
A Change in the number of visits to FFO in an area Ω after deploying an intervention I as a function of the ratio of FFO to FO, ϕ , in that area. Dashed lines marked the thresholds for the strategies to choose the top 100 areas by FFO ratio (vertical line, red points, Food Swamp intervention) or by the total change in the number of visits (horizontal line, green points, Behavior-Environment intervention). Orange points are those 100 areas chosen as the top home areas according to the FFO environment (Low Food Access intervention), and blue points correspond to those 100 areas chosen as the top areas containing more FO visits (Food Hotspots intervention). B Total effect of each intervention strategy in the different urban areas. Shades correspond to the number of actions changed by different income quantiles. C Relative frequency (to all areas) to find different groups of POIs (topics) in the areas selected for each intervention strategy.
Finally, to understand what types of areas Ω are targeted in our Behavior-Environment intervention, we use latent topic analysis to determine the groups of points of interest (POIs) that appear more frequently in those areas (see Supplementary Note 8 ). As shown in Fig. 4 , we find that the groups of POIs related to Malls, Industry/Factory, Airport or Office are more likely to appear in our targeted areas than in the rest of the areas in the city and the rest of interventions. Other groups of POIs, like Restaurants or Entertainment, are less likely to be areas selected in our Behavior-Environment intervention but more likely to be selected in the Food-Hotspots intervention. However, this intervention is less efficient, meaning that despite a lot of food visits happening around Restaurant and Entertainment areas, people making food decisions there are less affected by the environment, and thus the intervention is less successful. In summary, these large differences in our results suggest that more efficient interventions could be deployed to specific types of food environments away from residential areas, where FO decisions are most influenced (and likely constrained) by the environment, i.e., closer to work, travel, or shopping areas. Behavioral interventions in cities with different relative compositions of those areas might have slightly separated effects. For example, although behavioral interventions are always more effective than static interventions, the difference is slightly smaller in cities like Philadelphia, Seattle, or Los Angeles, suggesting that behavioral interventions depend somewhat on city-specific structural or commercial characteristics (see Supplementary Note 10 ).
The effect of food environment exposure on diet and related diseases has been studied extensively; however, in many cases, small, limited datasets have hampered the ability to understand this complex process in urban areas 18 , 19 , 20 , 22 , 23 , 25 , 27 , 30 , 31 , 32 , 33 , 45 . A major research limitation has been a focus on cross-sectional observations of static food environments around the home, and limited study of the mobile food environments people are exposed to and food outlets they visit as they navigate their day-to-day environments 20 , 30 , 31 , 32 , 45 . Our dataset and semi-causal study designs have allowed us to analyze the effect of exposure to food environments on food choice at a remarkable granularity and across diverse populations. This longitudinal, individual-level behavior data has also allowed us to analyze how food choice is motivated by features of food environments people are exposed to in their daily routines vs. by individual preference. We find that most visits to fast food outlets occur relatively far (a median of 6.74 km) from home and that exposure to low-quality food environments beyond the home is significantly linked to increased visits to FFO, across diverse sociodemographic groups. Furthermore, our results show that the composition of mobile food environments also affects the choice to visit other types of food options than fast food (e.g., Asian and Latin American cuisines; see Supplementary Note 11 ), reinforcing the role of mobile food environments beyond the home on multiple types of food decisions.
Previous policy interventions to food environments have been targeted at low-quality neighborhood food environments, such as ‘food swamps’ characterized by an abundance of fast food options. Interventions focused on static features of neighborhood environments do not reflect the complex intertwined process of human mobility, food environment exposure, and eating decisions in urban areas. This mischaracterization may partially explain the unsuccessful neighborhood interventions that aim to regulate the fast food environments near where people live 22 , 23 , 34 . To reduce visits to FFO and improve overall dietary quality, our results suggest that we may need to intervene in the mobile food environments that are not only characterized as low-quality, but also where most FFO decisions happen, and, importantly, where people have been demonstrated to be most influenced or constrained by the options available in that area - observations possible with this mobility data. The most efficient interventions may be further from people’s homes, in areas where food environments are more determinant to food decisions, like work, school, travel, or shopping areas. Finally, future policy-specific work should seek to investigate further details of the operationalization and implementation of the theoretical strategies we investigate here for shifting the balance of FFO to non-FFO in a food environment to optimize the dietary health of individuals frequenting that environment, including specifically how and what kinds of outlets should be involved in the interventions. Mobility data is increasingly available for researchers and policymakers, and could be used as part of ‘smart city’ initiatives to identify a community’s food hot-spots and tailor their food environment interventions by including behavior analysis of their actions when they move around the city.
While food environment interventions based on these factors were demonstrated to impact all groups equally, including lower income groups and those with higher rates of diet-related disease, these data also allow us to observe vast inequities in exposure to food environments of lower nutritional quality for historically marginalized communities. Based on these observations and innumerable studies demonstrating the structural inequities between socioeconomic groups food environment exposure and access 9 , 11 , 12 , 14 , future targeted interventions should be designed to account for the additional and complex dimensions of fairness and equity 52 , 53 , while accounting for individual preferences and projected decisions.
In addition to identifying locations for food environment intervention, our methodology could also be used to inform individual-level interventions promoting or encouraging visits to food outlets located in food environments that have more diverse, healthy food options; for example, through a mobile app. Our results show that the impact of mobile food environments is also quite strong for other types of foods than fast food. Thus, the design of holistic individual-level interventions would require a combination with other data about food intake (e.g., delivery, nutrients 54 , degree of food processing 55 ), healthiness of available menu options 56 , food preference and sentiment 57 , 58 , and price sensitivity 46 , 59 , 60 .
Population-scale mobility data provide useful, dynamic behavioral indicators of FF visits and consumption 35 , 38 , re-defining static notions of food deserts or food swamps to mobile food environments determined not only by the diversity of FO available in those environments but also by their frequency of use and people’s susceptibility to what they offer. We hope our results and our complex-systems methodology using large-scale mobility data can inform more efficient policies and interventions on food environments complementing and extending those around home neighborhoods 54 , 61 , 62 , 63 or efforts to increase the healthfulness of food items being sold at FFO 51 .
Our study has several limitations. Although it is well established that eating at FFOs is linked to poorer diet quality 28 , and there is a strong association between observed visits to FFO and FF intake 38 , foods of diverse nutritional quality are sold across FFO 56 . We have currently not examined the extent to which healthier options are offered at the FFO visited by our sample, nor how these options may impact purchase and consumption behavior. On the other hand, although it is likely that visits to FO that last more than 5 minutes lead to an eventual purchase, which is furthermore supported by the strong association between observed visits to a particular type of food outlet (fast food) and intake of food of that type 38 , there is no complete guarantee. Our results, therefore, serve as a proxy and are a lower bound for individuals’ potential FF intake. Also, since visits are attributed to the closest POI, there are limitations to the detection of visits to certain food outlets, such as those in multi-story or multi-purpose buildings (e.g., malls) where FFO are frequently found. Additionally, because we only detect visits greater than five minutes in duration, we may miss very brief FF outlet visits (e.g., drive-thrus). Finally, although our casual framework provides robust evidence about the impact of mobile food environments on people’s FFO visits, we believe our results may be further tested through carefully designed experiments and interventions. These experiments and interventions should also explore potentially different effects of food environments on other types of food outlet visits (e.g., visits to full-service restaurants or grocery stores). Finally, our mobility data sample from 2017 may not reflect changes in exposure to and impact of food environment on FO visit behavior following the pandemic and related changes that have occurred in the intervening years, including increased time spent in home neighborhoods and the great expansion of food delivery apps and their coverage. However, in settings where individuals are constrained by their environment, our findings and population-scale mobility framework likely still apply.
We use individual-level anonymized mobility data of 1.86 million anonymized users in 11 US metropolitan areas over a period of 6 months, from October 2016 to March 2017. The mobility data were collected with the informed consent of the users, who opted-in to anonymized data sharing for research purposes under a GDPR and CCPA-compliant framework. Our mobility data were obtained from Spectus, a location intelligence and measurement company. Since the data used was anonymized and spatially aggregated at places, categories, or census areas, we were granted an Exemption by the MIT Committee on the Use of Humans as Experimental Subjects (COUHES protocol #1812635935) and its extension #E-2962. To identify visits to FO and FFO, we extracted from the mobility data the stays (stops) of people around a large collection of points of interest (POI) obtained from Foursquare, see Supplementary Note 1 . FFOs are quick-service restaurants where patrons typically pay before eating and were defined using Foursquare’s taxonomy and a name search using a list of chain FFO; FOs, which represent all retail food outlets including grocery stores, supermarkets, big box stores, convenience stores, restaurants, were based on Foursquare’s existing taxonomy (see Supplementary Note 3 ). We have comprehensibly checked that our results do not depend on the choices made on the definition of stays, the categorization of the FFO, the POI database, or the definition of the environment and the population representativity of our data. A full description of those definitions and robustness checks is provided in the Supplementary Notes 1 , 2 , 3 , 4 , and 12 . Census demographic data come from the American Community Survey (5y) from the Census 64 . Census tract-level estimates for different health risk behaviors and outcomes were obtained from the 2017 edition of the PLACES Local data for Better Health dataset from the CDC 65 . Food desert data come from the Low-income and low-supermarket-access classification of census tracts done by the U.S. Department of Agriculture in their Food Environment Atlas 8 . Further details about those datasets can be found in the Supplementary Note 1 .
Definition of the home and mobile food environment and food context
To characterize the food environment users are exposed around a given place x we measure the ratio of FFO to any FO within a 1km radius, ϕ ( x ). We have extensively checked that our results do not depend on other definitions of the food environment. For example, in Supplementary Note 12 we show that similar results are obtained when we take ϕ ( x ) as the ratio of FFO to FO of the closest 25 FO to x , a definition that accounts for the different density of FO around the city. Home food environments are described by the value of ϕ around where people live \({\phi }_{i}^{h}=\phi ({{{{{{{{\bf{h}}}}}}}}}_{i})\) . While food environment exposure around place x is given by ϕ ( x ) we also computed the total exposure a user gets by moving around as \({\phi }_{i}^{m}={\sum }_{t}\tau ({{{{{{{{\bf{x}}}}}}}}}_{i,t})\phi ({{{{{{{{\bf{x}}}}}}}}}_{i,t})/{\sum }_{t}\tau ({{{{{{{{\bf{x}}}}}}}}}_{i,t})\) for all times t the user stops for more than five minutes (irrespectively of whether a FO is visited) and where τ ( x i , t ) is the duration of the stop of individual i at x i , t . The food context is the environment where the user was before going to visit a FFO. If the user was at c i t before lunch, the context is measured by ϕ ( c i t ). Finally, the overall averaged fraction of visits to fast food of individual i is computed as \({\mu }_{i}=\overline{{y}_{it}}={\sum }_{t}{y}_{it}/{N}_{i}\) for all times t the user visits a FO and where N i is the total number of FO visits of individual i .
Statistical models
To test the effect of mobile food environments, we have run a number of statistical models. For the main results in Fig. 2 , we used logistic regression to link the binary output y i t to the ratio of FFO options around the context ϕ ( c i t ), see Eq. ( 1 ). We control individual preferences and daily patterns by introducing a fixed effect by user ( α i ) and day ( δ t ). Regression was only performed for those individuals that have at least one FFO and non-FFO visit. To account for potential heterogeneity in our regression, we also cluster errors by day and user. Similarly, for the visits to the DMV, we used a simpler logistic regression. Since we typically have only one observation and day per user, the fixed factor α i was substituted by the actual observed fraction of visits to FFO of each individual, and we dropped the daily fixed factor. Finally, for the analysis of the different interventions, we have extended the model ( 1 ) to the rest of the day by considering each stay within our dataset as a context c i t , and we evaluate if there is a food visit y i t in the next two hours after that stay. A full description of those models, their predicting power (accuracy around 80% at individual level and 90% at area level), and the impact of distance between context and action are provided in the Supplementary Note 7 .
Detecting and analyzing the change of context
To identify those users that change their context before lunch, we have used a statistical methodology to detect change points in time series (see Supplementary Note 5 ). Using this method, we detected 7913 users in our dataset that changed context during our observation period. To provide a statistically robust estimation of the impact of that change in FFO visits at lunch, we define four groups of users depending on whether their contexts before/after the change have Low ( ϕ < 0.13) or High ( ϕ > 0.13) ratio of FFO in their contexts. We investigate the FFO ratio of visits of those groups of users that change from Low to High and from High to Low using those that change from Low to Low and from High to High as counterfactuals, respectively. Note that we did not use as counterfactual those users that stayed in the same geographical context, but only those that changed their geographical context. This was done to reduce the possibility of some endogeneity between changing contexts and the food environment in the previous context. To analyze the difference in response to the change, we use Bayesian Structural Time Series to predict how the response would have evolved after the change to a different context if the change had never happened 66 . Further details about this methodology can be found in the Supplementary Note 5 .
Interventions
To investigate the effect of an intervention strategy in an area Ω we evaluate the change in the probability P ( y i t = 1) for each action with context c i t in that area using the extension of the model in Eq. ( 1 ) for the full day with and without the intervention I. The total increase in the number of non-FFO visits can be approximated by the derivative of the model in Eq. ( 1 ):
where we are assuming that we only change the food environment ϕ in the intervention I. This expression is evaluated for a fixed amount (100) of areas Ω chosen by different criteria in each intervention strategy. See Supplementary Note 13 and 9 for further details about how interventions are defined and how we evaluate their impact on FFO visits.
Topic analysis of the areas
To identify the type of areas where the most efficient interventions happen, we use topic modeling to describe the different groups (topics) of POIs in each category that co-occur in the 18896 census tracts in our urban areas. Using Latent Dirichlet allocation (LDA), we found 20 groups of POIs and analyzed their composition. The topics are easily recognizable (see Supplementary Fig. S11 ), and we manually annotated them as Airports, Malls, Office, etc. Each census tract can be described then by the frequency of each of the 20 topics within it. Further details about this methodology can be found in the Supplementary Note 8 .
Reporting summary
Further information on research design is available in the Nature Portfolio Reporting Summary linked to this article.

Data availability
The data that support the findings of this study are available from Spectus through their Social Impact program https://spectus.ai/social-impact/ , but restrictions apply to the availability of these data, which were used under the license for the current study and are therefore not publicly available. Anonymized data to reproduce the results of our paper is available on request from https://doi.org/10.5281/zenodo.7798632 67 . Other data used come from the American Community Survey (5y) from the Census ( https://www.census.gov/programs-surveys/acs ), the PLACES Local data for Better Health from the CDC , or the Food Environment Atlas from the U.S. Department of Agriculture ( https://www.ers.usda.gov/data-products/food-environment-atlas ) which are publicly available on their websites. A description of these datasets is given in Supplementary Note 1 . Source data are provided with this paper.
Code availability
Code to run the analysis has been deposited on GitHub https://github.com/emoro/mobile_food_environments 68 .
Hall, K. D. et al. Ultra-processed diets cause excess calorie intake and weight gain: an inpatient randomized controlled trial of ad libitum food intake. Cell Metab. 30 , 67–77.e3 (2019).
Article PubMed PubMed Central Google Scholar
Popkin, B. M. et al. Towards unified and impactful policies to reduce ultra-processed food consumption and promote healthier eating. Lancet Diabetes Endocrinol. 9 , 462–470 (2021).
National Center for Chronic Disease Prevention and Health Promotion (NCCDPHP). Poor nutrition. https://www.cdc.gov/chronicdisease/resources/publications/factsheets/nutrition.htm . Accessed: 2022-06-04 (2022).
Afshin, A. et al. Health effects of dietary risks in 195 countries, 1990–2017: a systematic analysis for the Global Burden of Disease Study 2017. Lancet (Lond., Engl.) 393 , 1958–1972 (2019).
Article Google Scholar
Yach, D., Hawkes, C., Gould, C. L. & Hofman, K. J. The global burden of chronic diseases: overcoming impediments to prevention and control. Jama 291 , 2616–2622 (2004).
Article CAS PubMed Google Scholar
Story, M., Kaphingst, K. M., Robinson-O’Brien, R. & Glanz, K. Creating healthy food and eating environments: policy and environmental approaches. Annu. Rev. Public Health 29 , 253–272 (2008).
Article PubMed Google Scholar
De La Haye, K., Robins, G., Mohr, P. & Wilson, C. Adolescents’ intake of junk food: processes and mechanisms driving consumption similarities among friends. J. Res. Adolescence 23 , 524–536 (2013).
Economic Research Service (ERS), U.S. Department of Agriculture (USDA). Food environment atlas. https://www.ers.usda.gov/data-products/food-environment-atlas/ . Accessed: 2022-02-04 (2022).
Cooksey-Stowers, K., Schwartz, M. B. & Brownell, K. D. Food swamps predict obesity rates better than food deserts in the United States. Int. J. Environ. Res. Public Health 14 , 1366 (2017).
Luan, H., Law, J. & Quick, M. Identifying food deserts and swamps based on relative healthy food access: a spatio-temporal bayesian approach. Int. J. Health Geogr. 14 , 1–11 (2015).
Walker, R. E., Keane, C. R. & Burke, J. G. Disparities and access to healthy food in the united states: a review of food deserts literature. Health Place 16 , 876–884 (2010).
Fleischhacker, S. E., Evenson, K. R., Rodriguez, D. A. & Ammerman, A. S. A systematic review of fast food access studies. Obes. Rev. 12 , e460–e471 (2011).
Jekanowski, M. D., Binkley, J. K. & Eales, J. Convenience, accessibility, and the demand for fast food. J. Agric. Resour. Econ. 26 , 58–74 (2001).
Google Scholar
Rose, D., Bodor, J. N., Hutchinson, P. L. & Swalm, C. M. The importance of a multi-dimensional approach for studying the links between food access and consumption. J. Nutr. 140 , 1170–4 (2010).
Feng, J., Glass, T. A., Curriero, F. C., Stewart, W. F. & Schwartz, B. S. The built environment and obesity: a systematic review of the epidemiologic evidence. Health Place 16 , 175–190 (2010).
Leal, C. & Chaix, B. The influence of geographic life environments on cardiometabolic risk factors: a systematic review, a methodological assessment and a research agenda. Obes. Rev. 12 , 217–230 (2011).
Fraser, L. K., Edwards, K. L., Cade, J. & Clarke, G. P. The Geography of fast food outlets: a review. Int. J. Environ. Res. Public Health 7 , 2290–2308 (2010).
Sisnowski, J., Street, J. M. & Merlin, T. Improving food environments and tackling obesity: a realist systematic review of the policy success of regulatory interventions targeting population nutrition. PLoS ONE 12 , e0182581 (2017).
Dubowitz, T. et al. Healthy food access for urban food desert residents: an examination of the food environment, food purchasing practices, diet and bmi. Public health Nutr. 18 , 2220–2230 (2015).
Cobb, L. K. et al. The relationship of the local food environment with obesity: a systematic review of methods, study quality, and results. Obesity 23 , 1331–1344 (2015).
Reinvestment Fund. U.S.D.A. Healthy Food Financing Initiative. https://www.investinginfood.com/ . Accessed: 2022-08-13 (2022).
Sturm, R. & Hattori, A. Diet and obesity in Los Angeles County 2007–2012: Is there a measurable effect of the 2008 “Fast-Food Ban"? Soc. Sci. Med. 133 , 205–211 (2015).
Freedman, D. A. et al. Small improvements in an urban food environment resulted in no changes in diet among residents. J. Community Health 46 , 1–12 (2021).
Singleton, C. R., Li, Y., Odoms-Young, A., Zenk, S. N. & Powell, L. M. Change in food and beverage availability and marketing following the introduction of a healthy food financing initiative–supported supermarket. Am. J. Health Promotion 33 , 525–533 (2019).
Ghosh-Dastidar, M. et al. Does opening a supermarket in a food desert change the food environment? Health Place 46 , 249–256 (2017).
Ortega, A. N. et al. Substantial improvements not seen in health behaviors following corner store conversions in two latino food swamps. BMC Public Health 16 , 1–10 (2016).
Elbel, B. et al. Assessment of a government-subsidized supermarket in a high-need area on household food availability and children’s dietary intakes. Public Health Nutr. 18 , 2881–2890 (2015).
Saksena, M. J. et al. America’s eating habits: food away from home. Tech. Rep. United States Department of Agriculture, Economic Research Service (2018).
Subar, A. F. et al. Addressing current criticism regarding the value of self-report dietary data. J. Nutr. 145 , 2639–45 (2015).
Article CAS PubMed PubMed Central Google Scholar
Chaix, B. Mobile sensing in environmental health and neighborhood research. Annu. Rev. Public Health 39 , 367–384 (2018).
Burgoine, T. & Monsivais, P. Characterising food environment exposure at home, at work, and along commuting journeys using data on adults in the UK. Int. J. Behav. Nutr. Phys. Act. 10 , 85–85 (2013).
Cetateanu, A. & Jones, A. How can GPS technology help us better understand exposure to the food environment? A systematic review. SSM - Popul. Health 2 , 196–205 (2016).
Scully, J. Y., Moudon, A. V., Hurvitz, P. M., Aggarwal, A. & Drewnowski, A. A time-based objective measure of exposure to the food environment. Int. J. Environ. Res. Public Health 16 , 1180 (2019).
Li, J. & Kim, C. Exploring relationships of grocery shopping patterns and healthy food accessibility in residential neighborhoods and activity space. Appl. Geogr. 116 , 102169 (2020).
Moro, E., Calacci, D., Dong, X. & Pentland, A. Mobility patterns are associated with experienced income segregation in large US cities. Nat. Commun. 12 , 4633 (2021).
Article ADS CAS PubMed PubMed Central Google Scholar
Aleta, A. et al. Modelling the impact of testing, contact tracing and household quarantine on second waves of covid-19. Nat. Hum. Behav. 4 , 964–971 (2020).
Bahadoran, Z., Mirmiran, P. & Azizi, F. Fast food pattern and cardiometabolic disorders: a review of current studies. Health Promotion Perspect. 5 , 231 (2015).
Horn, A.L., et al. Population mobility data provides meaningful indicators of fast food intake and diet-related diseases in diverse populations. npj Digit. Med . 6 , 208 (2023).
Bureau of Transportation Statistics. U.S. Department of Transportation, Local Area Transportation Characteristics for Households (LATCH Survey). https://www.bts.gov/statistical-products/surveys/local-area-transportation-characteristics-households-latch-survey . Accessed: 2022-02-04 (2017).
Eckert, J. & Vojnovic, I. Fast food landscapes: exploring restaurant choice and travel behavior for residents living in lower eastside detroit neighborhoods. Appl. Geogr. 89 , 41–51 (2017).
Powell, L. M., Chaloupka, F. J. & Bao, Y. The availability of fast-food and full-service restaurants in the United States associations with neighborhood characteristics. Am. J. Prevent. Med. 33 , S240–S245 (2007).
Ogden, C. L. et al. Prevalence of obesity among adults, by household income and education - United States, 2011–2014. Mmwr. Morbidity Mortal. Wkly. Rep. 66 , 1369–1373 (2017).
Powell, L. M., Nguyen, B. T. & Han, E. Energy intake from restaurants: demographics and socioeconomics, 2003–2008. Am. J. Prevent. Med. 43 , 498–504 (2012).
Zagorsky, J. L. & Smith, P. K. The association between socioeconomic status and adult fast-food consumption in the us. Econ. Hum. Biol. 27 , 12–25 (2017).
Kestens, Y. et al. Association between activity space exposure to food establishments and individual risk of overweight. PLoS ONE 7 , e41418 (2012).
Allcott, H. et al. Food deserts and the causes of nutritional inequality. Q. J. Econ. 134 , 1793–1844 (2019).
Brown, H. et al. No new fast-food outlets allowed! evaluating the effect of planning policy on the local food environment in the north east of England. Soc. Sci. Med. 306 , 115126 (2022).
Nixon, L. et al. Fast-food fights: news coverage of local efforts to improve food environments through land-use regulations, 2000–2013. Am. J. Public Health 105 , 490–496 (2015).
Winne, M. Community food security: promoting food security and building healthy food systems. Venice, CA: Community Food Security Coalition https://www.hungercenter.org/wpcontent/uploads/2011/07/Community-Food-Security-Mark-Winne.pdf (2005).
The White House Briefing Room. Fact sheet: The biden-harris administration announces more that 8 billion in new commitments as part of call to action for white house conference on hunger, nutrition, and health. https://www.whitehouse.gov/briefing-room/statements-releases/2022/09/28/fact-sheet-the-biden-harris-administration-announces-more-than-8-billion-in-new-commitments-as-part-of-call-to-action-for-white-house-conference-on-hunger-nutrition-and-health/ . Accessed: 2023-03-20 (2022).
Fuster, M. et al. Facilitating healthier eating at restaurants: a multidisciplinary scoping review comparing strategies, barriers, motivators, and outcomes by restaurant type and initiator. Int. J. Environ. Res. Public Health 18 , 1479 (2021).
Rahmattalabi, A. et al. Fair influence maximization: a welfare optimization approach. Proc. AAAI Conf. Artif. Intell. 35 , 11630–11638 (2021).
He, Y., Burghardt, K., Guo, S. & Lerman, K. Learning fairer interventions. In Proceedings of the 2022 AAAI/ACM Conference on AI, Ethics, and Society , 317–323 https://doi.org/10.1145/3514094.3534172 (2022).
Aiello, L. M., Schifanella, R., Quercia, D. & Prete, L. D. Large-scale and high-resolution analysis of food purchases and health outcomes. EPJ Data Sci. 8 , 14 (2019).
Ravandi, B., Mehler, P., Barabasi, A.-L. & Menichetti, G. Grocerydb: Prevalence of processed food in grocery stores. medRxiv https://doi.org/10.1101/2022.04.23.22274217 (2022).
Liu, I. C., de la Haye, K., Abeliuk, A. & Horn, A. L. A continuous indicator of food environment nutritional quality. medRxiv. https://doi.org/10.1101/2021.11.24.21266841 . (2021).
De Choudhury, M., Sharma, S. & Kiciman, E. Characterizing dietary choices, nutrition, and language in food deserts via social media. In Proceedings of the 19th ACM conference on computer-supported cooperative work & social computing , 1157–1170 https://doi.org/10.1056/NEJMra052638 (2016).
Vydiswaran, V. V. et al. Uncovering the relationship between food-related discussion on twitter and neighborhood characteristics. J. Am. Med. Inform. Assoc. 27 , 254–264 (2020).
Afshin, A. et al. The prospective impact of food pricing on improving dietary consumption: a systematic review and meta-analysis. PloS One 12 , e0172277 (2017).
Meyer, K. A. et al. Sociodemographic differences in fast food price sensitivity. JAMA Intern. Med. 174 , 434–442 (2014).
Mozaffarian, D. Dietary and policy priorities to reduce the global crises of obesity and diabetes. Nat. Food 1 , 38–50 (2020).
Vermeulen, R., Schymanski, E. L., Barabási, A.-L. & Miller, G. W. The exposome and health: where chemistry meets biology. Science 367 , 392–396 (2020).
Aleta, A. et al. A need for a paradigm shift in healthy nutrition research. Front. Nutr. 9 , 881465 (2022).
United States Census Bureau. 2013–2017 American Community Survey 5-year Estimates. https://www.census.gov/programs-surveys/acs . Accessed: 2020-12-04 (2019).
Centers for Disease Control and Prevention. 500 Cities: local data for better health. https://www.cdc.gov/places/about/500-cities-2016-2019/index.html . Accessed: 08-01-2021 (2017).
Brodersen, K. H., Gallusser, F., Koehler, J., Remy, N. & Scott, S. L. Inferring causal impact using Bayesian structural time-series models. Ann. Appl. Stat. 9 , 247–274 (2015).
Article MathSciNet Google Scholar
Garcia-Bulle, B. et al. Data for the manuscript: effect of mobile food environments on fast food visits. https://doi.org/10.5281/zenodo.7798632 . (2024).
Garcia-Bulle, B. et al. Code for the manuscript: effect of mobile food environments on fast food visits. https://doi.org/10.5281/zenodo.10594056 . (2024).
U.S. Census Bureau. TIGER data products guide. https://www.census.gov/programs-surveys/geography/guidance/tiger-data-products-guide.html . Accessed: 2020-12-04 (2019).
Download references
Acknowledgements
E.M. acknowledges support by Ministerio de Ciencia e Innovación/Agencia Española de Investigación (MCIN/AEI/10.13039/501100011033) through grant PID2019-106811GB-C32 and the National Science Foundation under Grant No. 2218748. K. de la H. acknowledges support from the University of Southern California Keck School of Medicine Dean’s Pilot Funding Program, and the National Center for Advancing Translational Sciences under grant 1U54TR004279. B.M.B. acknowledges support from the Yale Cancer Prevention and Control Training Program, funded by the National Cancer Institute (T32 CA250803). A.L.H. acknowledges support from an NIH Ruth L Kirschstein National Research Service Award (NRSA) Institutional Training Grant (T32 5T32CA009492-35). The funders had no role in the study design, data collection and analysis, decision to publish, or preparation of the manuscript.
Author information
Abigail L. Horn
Present address: Information Sciences Institute, Viterbi School of Engineering, University of Southern California, Los Angeles, CA, 90292, USA
Authors and Affiliations
Institute for Data, Systems, and Society, Massachusetts Institute of Technology, Cambridge, MA, 02139, USA
Bernardo García Bulle Bueno, Mohsen Bahrami, Alex Pentland & Esteban Moro
Department of Population and Public Health Sciences, Keck School of Medicine, University of Southern California, Los Angeles, CA, 90089, USA
Abigail L. Horn & Brooke M. Bell
Department of Industrial and Systems Engineering, Viterbi School of Engineering, University of Southern California, Los Angeles, CA, 90089, USA
Department of Chronic Disease Epidemiology, Yale School of Public Health, Yale University, New Haven, CT, 06510, USA
Brooke M. Bell
Sabanci Business School, Sabanci University, 34956, Tuzla, Istanbul, Turkey
Burçin Bozkaya
Center for Economic and Social Research, University of Southern California, Los Angeles, CA, 90089, USA
Kayla de la Haye
Grupo Interdisciplinar de Sistemas Complejos (GISC), Department of Mathematics and GISC, Universidad Carlos III de Madrid, 28911, Leganés, Spain
Esteban Moro
Network Science Institute, Northeastern University, Boston, MA, 02115, USA
You can also search for this author in PubMed Google Scholar
Contributions
B.G.-B., A.L.H., K. de la H. and E.M. designed research; B.G.-B. and E.M. performed research and analyzed the results. B.G.-B. and E.M. wrote the first draft of the manuscript. B.G.-B., A.L.H., B.M.B., M.B., B.B., A.P., K. de la H. and E.M. discussed the results and edited the manuscript. All authors approved the final version.
Corresponding author
Correspondence to Esteban Moro .
Ethics declarations
Competing interests.
The authors declare no competing interests.
Peer review
Peer review information.
Nature Communications thanks the anonymous reviewers for their contribution to the peer review of this work. A peer review file is available.
Additional information
Publisher’s note Springer Nature remains neutral with regard to jurisdictional claims in published maps and institutional affiliations.
Supplementary information
Supplementary information, peer review file, reporting summary, source data, source data, rights and permissions.
Open Access This article is licensed under a Creative Commons Attribution 4.0 International License, which permits use, sharing, adaptation, distribution and reproduction in any medium or format, as long as you give appropriate credit to the original author(s) and the source, provide a link to the Creative Commons licence, and indicate if changes were made. The images or other third party material in this article are included in the article’s Creative Commons licence, unless indicated otherwise in a credit line to the material. If material is not included in the article’s Creative Commons licence and your intended use is not permitted by statutory regulation or exceeds the permitted use, you will need to obtain permission directly from the copyright holder. To view a copy of this licence, visit http://creativecommons.org/licenses/by/4.0/ .
Reprints and permissions
About this article
Cite this article.
García Bulle Bueno, B., Horn, A.L., Bell, B.M. et al. Effect of mobile food environments on fast food visits. Nat Commun 15 , 2291 (2024). https://doi.org/10.1038/s41467-024-46425-2
Download citation
Received : 15 August 2022
Accepted : 26 February 2024
Published : 14 March 2024
DOI : https://doi.org/10.1038/s41467-024-46425-2
Share this article
Anyone you share the following link with will be able to read this content:
Sorry, a shareable link is not currently available for this article.
Provided by the Springer Nature SharedIt content-sharing initiative
By submitting a comment you agree to abide by our Terms and Community Guidelines . If you find something abusive or that does not comply with our terms or guidelines please flag it as inappropriate.
Quick links
- Explore articles by subject
- Guide to authors
- Editorial policies
Sign up for the Nature Briefing newsletter — what matters in science, free to your inbox daily.

- Search Menu
- Advance articles
- Editor's Choice
- Supplement Archive
- Article Collection Archive
- Author Guidelines
- Submission Site
- Open Access
- Call for Papers
- Why Publish?
- About Nutrition Reviews
- About International Life Sciences Institute
- Editorial Board
- Early Career Editorial Board
- Advertising and Corporate Services
- Journals Career Network
- Self-Archiving Policy
- Journals on Oxford Academic
- Books on Oxford Academic
Article Contents
Introduction, energy and energy density, relationship between takeaway and fast foods and obesity, energy content and intake of takeaway and fast foods, total fat and saturated fatty acids, trans fatty acids.
- < Previous
Nutritional challenges and health implications of takeaway and fast food
- Article contents
- Figures & tables
- Supplementary Data
Agnieszka Jaworowska, Toni Blackham, Ian G Davies, Leonard Stevenson, Nutritional challenges and health implications of takeaway and fast food, Nutrition Reviews , Volume 71, Issue 5, 1 May 2013, Pages 310–318, https://doi.org/10.1111/nure.12031
- Permissions Icon Permissions
Consumption of takeaway and fast food continues to increase in Western societies and is particularly widespread among adolescents. Since food is known to play an important role in both the development and prevention of many diseases, there is no doubt that the observed changes in dietary patterns affect the quality of the diet as well as public health. The present review examines the nutritional characteristics of takeaway and fast food items, including their energy density, total fat, and saturated and trans fatty acid content. It also reports on the association between the consumption of such foods and health outcomes. While the available evidence suggests the nutrient profiles of takeaway and fast foods may contribute to a variety of negative health outcomes, findings on the specific effects of their consumption on health are currently limited and, in recent years, changes have been taking place that are designed to improve them. Therefore, more studies should be directed at gaining a firmer understanding of the nutrition and health consequences of eating takeaway and fast foods and determining the best strategy to reduce any negative impact their consumption may have on public health.
Lifestyle changes that have taken place in many countries worldwide over the last few decades have been shown to impact food consumption patterns. 1 , – 4 One of the most prominent trends is the increasing frequency with which meals are consumed outside of the home environment. 4 , – 6 In addition, even meals consumed at home are often purchased from catering outlets that offer takeaway or home delivery service. 5 , 7 The traditional family dinner is increasingly being replaced by eating “on the run” at various locations throughout the day. 1 As a result, less time is spent on food preparation, with an average woman and man in the United States spending 47 min and 19 min per day, respectively, carrying out food preparation and cleaning up. Moreover, among the 13,200 US citizens in one study, 59% of men and 32% of women did not spend any time on daily food preparation. 8
Food eaten outside of the home is becoming an important and regular component of the Western diet. 9 , 10 A number of studies have shown increased frequency of takeaway and fast food consumption worldwide, especially in Europe, the United States, and Australia. 9 , – 15 A governmental report in the United Kingdom indicated about 22% of residents were found to purchase foods from takeaway outlets at least once a week and 58% a few times a month. 11 A similar frequency of consumption of takeaway or fast food has also been observed in other countries, with approximately 28% of Australians 12 consuming takeaway meals at least twice a week and 37% of US residents 13 eating fast food at least once over two nonconsecutive days. Fast food is particularly popular among adolescents, with a report from 2001 indicating that 75% of US teenagers between the ages of 11 and 18 years eat at fast-food outlets at least once a week 14 and a 2010 report indicating that 70% of Brazilian students (9–18 years old) consume fast food four times or more per week. 15 Moreover, Guthrie et al. 10 reported that consumption of fast food among children has increased from 2% of total energy in the 1970s to 10% of energy in the 1990s. 10 That observed trend is continuing among children and adolescent populations, with data from the 2003–2006 National Health and Nutrition Examination Surveys showing a further increase to 13% of total daily energy intake. 16
It is well known that food plays an important role in the development and prevention of many diseases. 17 There is also no doubt that observed changes in dietary patterns affect the quality of the diet as well as public health. Consumption of takeaway and fast food has been shown to have adverse health effects, and while the majority of studies on this subject have focused on the relationship between fast food consumption and weight gain, 18 , – 20 more frequent consumption of meals prepared outside of the home has also been observed to correspond with increased risk of insulin resistance, type 2 diabetes, elevated total cholesterol, and low-density lipoprotein cholesterol (LDL-C) levels as well as decreased high-density lipoprotein cholesterol (HDL-C) concentrations. 18 , 21 , 22 Takeaway or fast food consumers are characterized by higher intakes of energy, fat, saturated fatty acids (SFAs), trans fatty acids (TFAs), added sugar and sodium, and lower intakes of fiber, macronutrients, and vitamins in comparison to those who do not eat food prepared outside the home. 13 , 19 , 23 , 24 Additionally, takeaway and fast-food consumption has been linked to poor dietary patterns including higher intakes of carbonated soft drinks and sweets and lower consumption of fruits, vegetables, whole grains, and dairy products. 12 , 13 , 19 , 24
This review focuses on the energy and fat content in takeaway and fast foods and their health implications. However, it should be pointed out that other components of takeaway and fast foods, such as salt and sugar, also have important effects on health.
Literature searches were performed using the following electronic databases: Medline, ScienceDirect, and Web of Science. The following key words were used: fast food, takeaway food, nutrient content, lifestyle, health, obesity, cardiovascular disease, blood lipids, fat, saturated fatty acids, trans fatty acids, energy density, food consumption patterns, diet quality. In addition, the reference list of each original and review article identified was searched for additional references. Searches were restricted to manuscripts in the English-language literature and included all available data until March 2011. Articles were limited to human participants only.
Humans possess a weak initial ability to recognize the energy density of consumed food and to appropriately regulate the bulk of food eaten to maintain energy balance; thus, people generally tend to consume a similar amount of food every day regardless of variations in energy density. 25 , – 27 This tendency to consume a constant amount of food was confirmed by Seagle et al., 28 who analyzed the 4-day food records of normal-weight adults. Daily variations in the weight of consumed food were significantly smaller than variations in the intake of either energy or fat. 28 Similarly, a retrospective investigation of three community studies from the United Kingdom (the Cambridge Family Food Survey, n = 195); the MRC National Survey of Health and Development [NSHD], n = 343; and the Leeds Nutritional Survey, n = 2,086) showed that the weight of food consumed remained relatively constant over a 7-day period. 29 However, when food with a low energy density is eaten, a greater amount of food needs to be consumed for a given level of energy intake in comparison to food with a high energy density. Therefore, increasing the energy density of the diet may result in a passive increase in energy intake, because people are generally habituated to eat a relatively constant weight of food.
Bell et al. 26 conducted a study of normal-weight women ( n = 18) who consumed all of their meals in the laboratory over three 2-day periods. During lunch, dinner, and an evening snack, participants consumed ad libitum main entrees, which were similar in macronutrient composition but varying in energy density (low, medium, or high). The women consumed similar amounts of food independent of the energy density of the meal served. Thus, energy intake was about 25% lower with meals of low energy density in comparison with those of high energy density. Results showed no differences across conditions in hunger or fullness before meals, after meals, or over each 2-day period. 26 These findings were confirmed by several other studies that tested the effect of variations in the fat content of the diet while maintaining a constant energy density. 30 , 31 Stubbs et al., 30 in a 14-day intervention study, reported that men who were offered a diet varying in fat content (20, 40, and 60% of total energy) but with a constant energy density, ate a constant weight of food; therefore, they had similar energy intakes despite different proportions of fat content in the diet. Similarly, in a randomized crossover study performed over an 11-day period, Saltzman et al. 31 found that seven pairs of male twins who consumed, ad libitum , a low- or a high-fat diet matched for energy density (20% or 40% of total energy) had similar daily energy intakes (10.3 and 10.7 MJ/d, respectively) regardless of the condition of the diet. These findings support the hypothesis that the energy density of consumed food is a crucial determinant of energy intake. Therefore, the weight or volume of food consumed, and, thus, the energy density, may increase or decrease energy intake independent of the macronutrient content of the diet.
The relationship between fast or takeaway food consumption and increased body mass index (BMI) and obesity has been reported in many epidemiological studies. 18 , – 20 , 32 Among a Spanish population ( n = 3,054), Schröder et al. 33 found that consumption of fast food more frequently than once a week increased the risk of being obese by 129%. These results are consistent with the findings of Kjøllesdal et al., 34 who reported from a group of working Oslo citizens ( n = 8,943) that the likelihood of being obese increased significantly with frequent eating in staff canteens, after demographic and socioeconomic variables were taken into account. Similar trends have also been observed in developing countries; Rouhani et al. 35 found that high intakes of fast foods were significantly associated with increased incidences of overweight and obesity among Isfahani (Iranian) girls aged 11–13 years.
Furthermore, consumption of fast foods two times or more per week has been independently associated with a 31% higher prevalence of moderate abdominal obesity in men and a 25% higher prevalence in women. 19 According to a theoretical model, an energy increase of 17 kcal/day for men and 19 kcal/day for women would lead to a weight increase of 1 kg per year independent of baseline body weight. 36 On average, regular consumption of fast-food meals was related to increases in energy intake of 56 kcal/day 37 and 187 kcal/day 19 among adults and children, respectively. Thus, a higher frequency of fast-food consumption was associated with a weight gain of 0.72 kg over 3 years, 37 and of 4.5 kg over a 15-year period, 18 above the average weight gain. Moreover, women who reported eating takeaway food once a week were 15% less likely to be weight maintainers than those who rarely (once a month or less) or never ate takeaway food. 38
It has been shown that a typical meal purchased from fast-food restaurants tends to be energy dense and contains approximately 236 kcal/100 g, which is twice the recommended energy density of a healthy diet. 39 Considering the large portion sizes of meals eaten out of the home, one meal can provide approximately 1,400 kcal. 40 Bauer et al. 41 found that despite the increasing attention to the role of fast food in the American diet, including legislation and public health campaigns addressing the healthfulness of fast food, the median energy content across all menu items remained relatively stable over a 14-year study period (1997–2010).
Mancino et al. 42 based on the dietary recall data collected over2 nonconsecutive days from the 2003–2004 National Health and Nutrition Examination Survey (NHANES) and the 1994–1996 Continuing Survey of Food Intakes by Individuals, and with the use of a first-difference estimator, found that each meal eaten away from home added, on average, 130 kcal to total daily energy intake, with lunch and dinner having the greatest effect on total daily energy. French et al., 14 in a study conducted among 11–18-year-old American teenagers ( n = 4,746), reported that energy intake was 40% higher among male and 37% higher among female adolescents who reported eating fast food three times or more during the studied week in comparison with those who had not eaten fast foods. Additionally, a dose-response pattern was observed with energy intake directly increasing with increased frequency of fast food consumption. 14 Similarly, a follow-up study including African American women aged 30–69 years ( n = 44,072) indicated that, compared to women who have never eaten Chinese food, pizzas, fried fish, fried chicken, or burgers, women who reported eating such foods at least once a week had significantly higher daily energy intakes. 21 Furthermore, a study by Bowman and Vinyard 19 that included 9,872 adults aged 20 years and older showed a positive relationship between the energy density of the diet and fast food consumption. Their evaluation of the quality of the diet of American adults showed increased dietary energy density among men and women who reported eating fast food (95 and 102 kcal/100 g, respectively) compared to those who did not (89 and 98 kcal/100 g among men and women, respectively). 19
The high levels of fat intake commonly associated with takeaway or fast food consumption may be a factor leading to obesity development that is independent of total energy intake. Findings of a study carried out by Alfieri et al. 43 among 150 adults in the United Kingdom found a strong positive correlation between BMI and total fat consumption but no association with energy intake. These results were in line with findings of a cross-sectional study of 15,266 men (55–79 years) performed by Satia-Abouta et al., 44 which showed that fat intake has a higher adipogenic effect than total energy intake. In a multivariate linear regression model after adjustment for demographic and health-related characteristics, BMI increased by 0.14 and 0.53 kg/m 2 for every 500 kcal of total energy intake and 500 kcal energy derived from fat, respectively. Additionally, only energy provided from fat, but not energy from other macronutrients (carbohydrate and protein), increased linearly with increasing BMI. In contrast, Larson et al. 45 suggested that dietary fat plays a very minor role in increasing adiposity, and explained only 2% of variation in body fat after controlling for other obesity risk factors.
There are several possible explanations for why dietary fat intake may be associated with body weight gain. A number of studies have shown that fat exerts a less satiating effect than either carbohydrate or protein. Cotton et al. 46 found that a carbohydrate-supplemented breakfast (173.4 g of carbohydrate, 11.2 g of fat, 12.7 g of protein, and 803 kcal of total energy) suppressed intake of food with the next meal, in contrast to the breakfast supplemented by fat (77.8 g of carbohydrate, 50.9 g of fat, 12.7 g of protein, and 803 kcal total energy), which produced no detectable effect on appetite expression. Furthermore, fat is utilized with very high energy efficiency; thus, the diet-induced thermogenesis following fat consumption is much lower than after protein or carbohydrate intakes. Also, a high-fat meal does not enhance lipid oxidation, and may, therefore, promote dietary fat accumulation in adipose tissue. In a study by Bennett et al., 47 the addition of 50 g of fat to a standard breakfast (55% energy from carbohydrate, 30% from fat, and 15% from protein) did not increase fat oxidation or energy expenditure either during the immediate 6 h postprandial period or over the following 18 h. Similarly, Horton et al. 48 found that in a group of 16 men who were offered 14 days of isoenergetic overfeeding (50% above energy recruitment) of fat and carbohydrate, overfeeding with fat did not produce an increase in fat oxidation and total energy expenditure, and led to storage of 90–95% of excess energy. In contrast, carbohydrate overfeeding was associated with increased carbohydrate oxidation and total energy expenditure and resulted in 75–85% of excess energy being stored. 48 Furthermore, Raben et al. 49 in a study of 19 healthy participants who were provided with meals similar in energy density but rich in protein, fat, carbohydrate, or alcohol observed that postprandial lipid oxidation was suppressed after protein-, carbohydrate-, and alcohol-rich meals and was almost unchanged after the fat-rich meal. Griffiths et al. 50 reported that lipid oxidation was higher after a high-fat meal (80 g of carbohydrate, 80 g of fat, and 18 g of protein) than after a low-fat meal (80 g of carbohydrate, 0.8 g of fat, and 18 g of protein), but the difference in oxidation level reached 10 g only (20.7 vs. 10.6 g, P < 0.01), despite the high-fat meal providing 79.2 g more fat than the low-fat meal. It should also be mentioned that fat is more effectively absorbed from the gastrointestinal tract in comparison to carbohydrate. Lammert et al. 51 showed that a high-fat diet produced significantly lower fecal loss of energy than a high-carbohydrate diet. In addition, fat is known to improve the taste and texture of many food products, which may also promote active overconsumption associated with enhanced appetite due to sensory stimulation. 52 Increased food intake occurring with increased food palatability has been observed in many previous studies. 53 , – 56 However, other studies that investigated the sensory properties of food involved in sensory-specific satiety found that increased sensory stimulation may reduce food consumption. 57
A diet high in fat, particularly one rich in SFAs, may not only lead to a higher risk of obesity development, it may also have other adverse health effects. Generally, SFAs increase total and HDL-C levels, although not all SFAs affect plasma lipid and lipoprotein concentrations in the same manner. 58 For example, stearic acid, in comparison with other SFAs, has little effect on plasma lipids; this has been proposed to be a result of the rapid conversion of stearic acid in the body to oleic acid. 59 On the other hand, SFAs with 12–16 carbon atoms are considered to be hypercholesterolemic, and lauric acid (C12:0) appears to be more potent than myristic acid (C14:0) or palmitic acid (C16:0). 59 However, it has been found that despite increasing serum total and LDL-C levels, C12:0, C14:0, and C16:0 acids also increase the concentration of HDL-C; as a result, they do not increase the ratio of total cholesterol to HDL-C. 60 Whether a diet high in SFAs is associated with an increased risk of coronary heart disease is still controversial. 60 , 61 A number of epidemiological and dietary intervention studies have found that a diet rich in SFAs is associated with a higher risk of impaired glucose tolerance, insulin resistance, and type 2 diabetes, 62 , – 64 but there is no evidence of a direct causal relationship with CVD. 65 Thanopoulou et al., 62 in a multinational survey, found that participants with recently diagnosed and undiagnosed type 2 diabetes had higher intakes of SFAs compared with healthy controls. These findings were similar to those of Wang et al. 64 who, in a 9-year follow-up study of 2,909 American participants (45–64 years of age), showed a positive association between diabetes incidence and the proportion of total SFAs in plasma cholesterol esters and phospholipids, which reflects dietary intake of fatty acids. In addition, higher intake of SFAs may increase the risk of several cancers. Kurahashi et al. 66 in a 7.5-year follow-up study among 43,435 Japanese men aged 45–74 years found that myristic and palmitic acids increased the risk of prostate cancer in a dose-dependent manner. Multivariable relative risk on comparison of the highest with the lowest quartiles of myristic acid and palmitic acid intake were 1.62 (1.15–2.29) and 1.53 (1.07–2.20), respectively. There is also evidence suggesting a possible relationship between SFA intake and a modest increase in breast cancer risk. 67
One of the main sources of SFAs in takeaway or fast foods worldwide is palm oil, which is widely used as a frying medium due to excellent frying performance together with production of a highly desirable fried food flavor; it is especially popular in Southeast Asian countries, as well as in small, independent takeaway outlets in the United Kingdom. 68 , 69 Palm oil is suggested as an acceptable alternative to PHVO in the deep fat frying process, but unhydrogenated vegetable oils are recommended as they produce a much more favorable plasma/serum lipid profile than either palm oil or partially hydrogenated oils. 59 , 70 In a dietary intervention study by Vega-López et al., 70 15 participants were provided for 5 weeks with food varying in the type of fat (partially hydrogenated soybean oil, soybean oil, palm oil, or canola oil; at two-thirds of total fat, or 20% of total energy). It was found that both partially hydrogenated soybean and palm oil resulted in higher LDL-C concentrations than other investigated fats. No significant differences in the total cholesterol to HDL-C ratio were observed among the diets enriched with palm, canola, and soybean oils. Vessby et al., 71 in the KANWU study, included 162 healthy participants who received an isoenergetic diet for 3 months containing either a high proportion of saturated or monounsaturated fatty acids, and found that replacement of SFAs with monounsaturated fatty acids was associated with improved insulin sensitivity.
On average, food eaten out of the home is characterized by a high total fat and SFA content. Stender et al., 72 after analyzing meals containing french fries and fried chicken (nuggets or hot wings) purchased from McDonald's and KFC outlets in 35 countries worldwide, found that the total fat content varied from 41 to 74 g depending on the country. These results were supported by later findings of Dunford et al., 73 who reported that food items (burgers, chicken products, sides, or pizzas) purchased from fast-food chains contained between 10 and 13 g of total fat and between 3.9 and 4.9 g of SFAs per 100 g.
The intake of fat and SFAs increases along with higher frequency of out-of-home eating. 13 , 19 , 21 A study involving a large sample ( n = 44,072) of adult African American women showed that total fat intake was significantly higher among women who reported eating out of the home at least once a week, regardless of the type of meals consumed (burgers, fried chicken, fried fish, Chinese food, pizzas, or Mexican food) when compared to those who had never eaten food prepared outside the home. 21 This is consistent with previous findings of Paeratakul et al., 13 which indicated that, among 9,063 adults and 8,307 children and adolescents, on the day when fast food was eaten, the intake of total fat, SFAs, and percentage of energy provided by fat was higher compared to the day without fast food consumption.
Trans fatty acids are formed during the commercial partial hydrogenation of unsaturated fats. Small amounts of TFAs are also produced by ruminants during the biohydrogenation of unsaturated fatty acids from feed by hydrogen produced during the oxidation of substrates with bacterial enzymes in the rumen. These two sources of TFAs contain similar species of TFA isomers, but in different amounts and proportions; thus, their consumption may have different biological effects. 74 The concentration of TFAs in partially hydrogenated vegetable oils (PHVO) may be as high as 30–50%, compared with only around 5% in dairy and ruminant meat products. 74 Ruminant and industrially produced TFAs have been shown to have a detrimental effect on blood lipids when consumed in high doses; however, moderate intakes of ruminant TFAs, such as those seen with normal dietary consumption, have neutral effects on plasma lipids and other risk factors for cardiovascular disease. 75 Hulshof et al. 76 reported that TFA intake from ruminant products was under 2 g/day (<1% of total energy intake) in all Western European countries investigated in the TRANSFAIR study, and the main source of TFAs in the diet was PHVO. A more recent study estimated that average US consumption of industrially produced TFAs has significantly decreased from 4.6 g/per day (2003) to 1.3 g/per day (2010) as a result of food labelling and legislation. 77 However, individuals with certain dietary habits may still consume high levels of industrially produced TFAs. 77 Indeed, very high TFA intake levels (3.5–12.5% of total energy intake) have been shown in males aged 12–19 years, and the types of foods consumed included fast food such as french fries, pies, and pastries. 78 In the United Kingdom, the National Diet Nutrition Survey reported an intake of less than 2 g per day of TFA, 79 but this survey did not assess intake from takeaway food from independent outlets.
Trans fatty acids, due to their physiological effects, are undesirable components of the diet. A growing body of evidence has demonstrated numerous adverse effects associated with consumption of TFAs, including systemic inflammation, diabetes, insulin resistance, endothelial dysfunction, obesity, decreased LDL particle size, decreased HDL-C and apolipoprotein A1 concentrations, and increased total cholesterol, lipoprotein (a) and apolipoprotein B levels. 80 , 81 A recent meta-analysis of the effects of TFA consumption on blood lipids and lipoproteins showed that each 1% energy replacement of TFAs with SFAs, monounsaturated fatty acids, or polyunsaturated fatty acids, respectively, decreased total cholesterol/HDL-C ratio by 0.31, 0.54, and 0.67; apolipoprotein B/apolipoprotein A1 ratio by 0.007, 0.010, and 0.011; and Lp(a) concentration by 3.76, 1.39, and 1.11 mg/L. 82 Esmaillzadeh et al. 83 in a cross-sectional study of 486 apparently healthy women aged 40–60 years found that greater consumption of PHVO was associated with increased circulating concentrations of several markers of endothelial dysfunction and systemic inflammation. C-reactive protein, interleukin 6, and soluble tumor necrosis factor 2 levels were, respectively, 73%, 17%, and 5% higher among women in the highest quintile of TFA intake compared with the lowest quintile. 84 Many studies that investigated an association between habitual intakes of or exposure to TFAs, assessed using tissue biomarkers (for example, erythrocyte membrane TFA concentrations, serum phospholipids, and adipose tissue fatty acid composition) have demonstrated a significantly increased risk of coronary heart disease among individuals with greater TFA consumption or exposure. 85 The majority of these studies have focused on PHVO and, until recently, no positive association between ruminant TFA consumption and cardiovascular risk was found 86 ; however, a recent study by Laake et al. 87 found ruminant TFAs increased this risk, including for coronary heart disease, among women but not among men. Furthermore, TFA consumption may be associated with weight gain and visceral fat accumulation. A large prospective study conducted among 16,587 men, after controlling for potential confounders, found that substitution of each 2% of energy intake from TFAs by energy from polyunsaturated fatty acids was independently associated with a 2.7 cm increase in waist circumference over 9 years. 88 It should also be mentioned that TFAs are transferred from the mother to the fetus across the placenta and are present in breast milk. 80 , 89 Because humans do not synthesize TFA isomers, the concentration of these isomers in human milk is directly related to the maternal diet. The content of TFAs in human milk varies among countries, from 0.5% in Africa, through 1.40–2.80% in Poland, to 6–7% of total fatty acids in Canada. 90 A recent cross-sectional study found that infants of mothers who consumed 4.5 g or more of TFAs daily while breastfeeding were over two times more likely to have body fat higher than 24% in comparison to the offspring of mothers consuming less TFAs. 91
Takeaway and fast food, particularly french fries and deep-fried meats may contain a large amount of TFAs from PHVO, which are used for deep frying. It has been reported that a single meal of french fries (171 g) and fried chicken (160 g) purchased from fast food outlets provided from 0.3 to 24 g of TFAs. 72 Similarly, Wagner et al. 92 found that the TFA content in burgers may vary between 0.1 and 1.05 g per 100 g and in french fries between 0.1 and 1.6 g per 100 g. This is in agreement with Australian data reporting that the level of TFAs in takeaway meals may be between 0.1 and 1.4 g/100 g depending on the type of meal. 93 Also, recent data from the United States showed that the content of TFAs in 32 representative fast-food samples ranged from 0.1 to 3.1 g per serving. 94
It has been assessed that individuals who frequently consume fast-food meals could be receiving between 6 and 12% of their dietary energy from TFAs, 95 and a single meal of fried chicken with chips may deliver four times more TFAs than the daily recommended allowance in the United Kingdom (i.e., no more than 2% of total recommended daily energy intake). 96
In New York City, legal requirements regarding the use of PHVO in the preparation of foods sold by chain and nonchain restaurants have been implemented. Phase one of the initiative (2007) obligated all food outlets to use oils, shortenings, and margarines containing less than 0.5 g of TFAs per serving. Phase two (2008) involved reformulating all food items to contain less than 0.5 g of TFAs per serving. These restrictions have resulted in significant decreases in the TFA content of foods purchased, and between 2007 and 2009, the mean TFA content per purchase decreased by 2.4 g. However, the existing regulations in New York City apply only to restaurants that are required to hold a permit from the New York City Health Department 97 ; thus, the level of TFAs in food products from independent outlets may be higher. 98
It should be pointed out that most of the accessible studies regarding TFA levels in fast foods or other takeaway fried meal options did not distinguish between naturally occurring TFAs in food products and TFAs from PHVO, or they evaluated the levels of specific species of TFA isomers.
In summary, a growing body of evidence suggests that, even though positive changes are being made to improve the nutrient profiles of takeaway and fast foods, 77 some of these frequently consumed foods may contribute to a variety of negative health outcomes, including cardiovascular disease, insulin resistance, type 2 diabetes, and obesity. 12 , 18 , – 22 Simultaneously, food prepared outside of the home is making up an increasing portion of the Western diet and there is no expectation that this trend will reverse or stop. However, most of the studies performed to date have only investigated the nutritional quality of food from fast-food restaurant chains, and there is still a lack of data regarding the nutrient content in takeaway meals from small independent outlets, including those serving such foods as ethnic cuisines, deli foods, fish and chips, and pizza. Furthermore, there is a lack of good-quality data on the consumption of different takeaway food options. To the best of our knowledge, no studies published to date have differentiated between consumption of fast foods and other types of takeaway meals, and the majority of previous studies have concentrated on foods from fast-food chain restaurants or have investigated food prepared outside of the home without considering the source, i.e., fast-food chain restaurants or independent takeaway outlets. However, recent work indicates there are significant differences in the nutrient composition of different types of takeaway meals (e.g., Indian, Chinese, English, pizzas, kebabs) 99 , 100 as well as between takeaway meals and ready-to-eat meal options of a similar type. 101 , 102 To date, only one study has examined the relationship between the frequency of consumption of specific types of meals eaten out of the home (i.e., burgers, pizzas, fried chicken, fried fish, Chinese food, and Mexican food) and the incidence of type 2 diabetes, but this study was limited to restaurant food only. 21 Furthermore, most studies have only investigated the frequency of out-of-home eating and have not taken into account the amount of food consumed, the overall diet quality, and other lifestyle factors. Therefore, more studies should be directed at furthering understanding of the nutrition and health consequences of both takeaway and fast food consumption and to finding the best strategies to reduce any negative impact their consumption may have on public health. Such strategies may require governmental regulation. In Finland, for example, legislation on food labelling, such as the mandatory warning of “high salt product” on products in which the salt concentration exceeds set limits, has been shown to be a useful tool to reduce salt intake in the population. 103 Similarly, in Denmark, the restriction of industrially produced TFA levels of all food products to a maximum of 2% of the total fat content showed it is possible to reduce or remove TFAs from the content of food products. 104
The cooperation of food technologists, nutritionists, and chefs is needed in order to alter the food preparation processes at fast-food and takeaway outlets with the goal of improving the nutritional quality of prepared meals. However, this may not be easy to achieve, as chefs and other decision makers may be reluctant to change recipes, especially due to concerns about adverse effects on palatability, which can potentially affect profits. Furthermore, voluntary guidelines do not always result in adequate changes to the nutritional quality of takeaway foods; thus, governmental regulations may be a more powerful means of effecting change.
Declaration of interest
The authors have no relevant interests to declare.
Jabs JT Devine CM . Time scarcity and food choices: an overview . Appetite. 2006 ; 47 : 196 – 204 .
Google Scholar
Naska A Fouskakis D Oikonomou E , et al. Dietary patterns and their socio-demographic determinants in 10 European countries: data from the DAFNE databank . Eur J Clin Nutr. 2006 ; 60 : 181 – 190 .
Uauy R Monteiro CA . The challenge of improving food and nutrition in Latin America . Food Nutr Bull. 2004 ; 25 : 175 – 182 .
Kearney J . Food consumption trends and drivers . Philos Trans R Soc Lond B Biol Sci. 2010 ; 365 : 2793 – 2807 .
Bowers DE . Cooking trends echo changing roles of women . Food Rev. 2000 ; 23 : 23 – 29 .
Hawkes C . Marketing activities of global soft drink and fast food companies in emerging markets: a review . In: Globalization, Diets and Noncommunicable Diseases . Geneva, Switzerland : World Health Organization ; 2002 . Available at: http://whqlibdoc.who.int/publications/9241590416.pdf . Accessed 27 November 2011.
Google Preview
Jekanowski MD . Causes and consequences of fast food sales growth . Food Rev. 1999 ; 22 : 11 – 16 .
Denton SL. Adding eldercare questions to the American Time Use Survey. Monthly Labor Review Online. November 2012. Available at: http://www.bls.gov/opub/mlr/2012/11/art3full.pdf . Accessed 21 March 2013.
Orfanos P Naska A Trichopoulos D , et al. Eating out of home and its correlates in 10 European countries. The European Prospective Investigation into Cancer and Nutrition (EPIC) study . Public Health Nutr. 2007 ; 10 : 1515 – 1525 .
Guthrie JF Lin BH Frazao E . Role of food prepared away from home in the American diet, 1977–78 versus 1994–96: changes and consequences . J Nutr Educ Behav. 2002 ; 34 : 140 – 150 .
Food Standards Agency . Consumer Attitudes to Food Standards: Wave 8 . January 2008 ; Available at: http://tna.europarchive.org/20111116080332/http://www.food.gov.uk/multimedia/pdfs/cas2007ukreport.pdf . Accessed 23 March 2013.
Smith KJ McNaughton SA Gall SL , et al. Takeaway food consumption and its associations with diet quality and abdominal obesity: a cross-sectional study of young adults . Int J Behav Nutr Phys Act. 2009 ; 6 : 29 .
Paeratakul S Ferdinand DP Champagne CM , et al. Fast-food consumption among US adults and children: dietary and nutrient intake profile . J Am Diet Assoc. 2003 ; 103 : 1332 – 1338 .
French SA Story M Neumark-Sztainer D , et al. Fast food restaurant use among adolescents: associations with nutrient intake, food choices and behavioural and psychosocial variables . Int J Obes. 2001 ; 25 : 1823 – 1833 .
Cimadon HM Geremia R Pellanda LC . Dietary habits and risk factors for atherosclerosis in students from Bento Gonçalves (state of Rio Grande do Sul) . Arq Bras Cardiol. 2010 ; 95 : 166 – 172 .
Poti JM Popkin BM . Trends in energy intake among US children by eating location and food source, 1977–2006 . J Am Diet Assoc. 2011 ; 111 : 1156 – 1164 .
World Health Organization . Diet, Nutrition, and the Prevention of Chronic Diseases . Technical Report Series 916. Geneva, Switzerland: World Health Organization; 2003 .
Pereira MA Kartashov AI Ebbeling CB , et al. Fast-food habits, weight gain, and insulin resistance (the CARDIA study): 15-year prospective analysis . Lancet. 2005 ; 365 : 36 – 42 .
Bowman SA Vinyard BT . Fast food consumption of U.S. adults: impact on energy and nutrient intakes and overweight status . J Am Coll Nutr. 2004 ; 23 : 163 – 168 .
Duffey KJ Gordon-Larsen P Jacobs DR Jr , et al. Differential associations of fast food and restaurant food consumption with 3-y change in body mass index. The Coronary Artery Risk Development in Young Adults Study . Am J Clin Nutr. 2007 ; 85 : 201 – 208 .
Krishnan S Coogan PF Boggs DA , et al. Consumption of restaurant foods and incidence of type 2 diabetes in African American women . Am J Clin Nutr. 2010 ; 91 : 465 – 471 .
Duffey KJ Gordon-Larsen P Steffen LM , et al. Regular consumption from fast food establishments relative to other restaurants is differentially associated with metabolic outcomes in young adults . J Nutr. 2009 ; 139 : 2113 – 2118 .
Clemens LH Slawson DL Klesges RC . The effect of eating out on quality of diet in premenopausal women . J Am Diet Assoc. 1999 ; 99 : 442 – 444 .
Bowman SA Gortmaker SL Ebbeling CB , et al. Effects of fast-food consumption on energy intake and diet quality among children in a national household survey . Pediatrics. 2004 ; 113 : 112 – 118 .
Rolls BJ Bell EA Castellanos VH , et al. Energy density but not fat content of foods affected energy intake in lean and obese women . Am J Clin Nutr. 1999 ; 69 : 863 – 871 .
Bell EA Castellanos VH Pelkman CL , et al. Energy density of foods affects energy intake in normal-weight women . Am J Clin Nutr. 1998 ; 67 : 412 – 420 .
Rolls BJ . The role of energy density in the overconsumption of fat . J Nutr. 2000 ; 130 ( 2S Suppl ): S268 – S271 .
Seagle H Davy B Grunwald G , et al. Energy density of self-reported food intake: variation and relationship to other food components [Abstract] . Obes Res. 1997 ; 5 : 78S .
Poppitt SD Prentice AM . Energy density and its role in the control of food intake: evidence from metabolic and community studies . Appetite. 1996 ; 26 : 153 – 174 .
Stubbs RJ Harbron CG Prentice AM . Covert manipulation of the dietary fat to carbohydrate ratio of isoenergetically dense diets: effect on food intake in feeding men ad libitum . Int J Obes. 1996 ; 20 : 651 – 660 .
Saltzman E Dallal GE Roberts SB . Effect of high-fat and low-fat diets on voluntary energy intake and substrate oxidation: studies in identical twins consuming diets matched for energy density, fiber and palatability . Am J Clin Nutr. 1997 ; 66 : 1332 – 1339 .
Burns C Jackson M Gibbons C , et al. Foods prepared outside the home: association with selected nutrients and body mass index in adult Australians . Public Health Nutr. 2002 ; 5 : 441 – 448 .
Schröder H Fïto M Covas MI . Association of fast food consumption with energy intake, diet quality, body mass index and the risk of obesity in a representative Mediterranean population . Br J Nutr. 2007 ; 98 : 1274 – 1280 .
Kjøllesdal MR Holmboe-Ottesen G Wandel M . Frequent use of staff canteens is associated with unhealthy dietary habits and obesity in a Norwegian adult population . Public Health Nutr. 2010 ; 14 : 133 – 141 .
Rouhani MH Mirseifinezhad M Omrani N , et al. Fast food consumption, quality of diet, and obesity among Isfahanian adolescent girls . J Obes. 2012 ; No. 2012 : doi: 10.1155/2012/597924 .
French SA Harnack L Jeffery RW . Fast food restaurant use among women in the Pound of Prevention study: dietary, behavioral and demographic correlates . Int J Obes. 2000 ; 24 : 1353 – 1359 .
Christiansen E Garby L Sorensen TI . Quantitative analysis of the energy requirements for development of obesity . J Theor Biol. 2005 ; 234 : 99 – 106 .
Ball K Brown W Crawford D . Who does not gain weight? Prevalence and predictors of weight maintenance in young women . Int J Obes. 2002 ; 26 : 1570 – 1578 .
Prentice AM Jebb SA . Fast foods, energy density and obesity: a possible mechanistic link . Obes Rev. 2003 ; 4 : 187 – 194 .
Isganaitis E Lustig RH . Fast food, central nervous system insulin resistance, and obesity . Arterioscler Thromb Vasc Biol. 2005 ; 25 : 2451 – 2462 .
Bauer KW Hearst MO Earnest AA , et al. Energy content of U.S. fast-food restaurant offerings: 14-year trends . Am J Prev Med. 2012 ; 43 : 490 – 497 .
Mancino L Todd J Lin BH . Separating what we eat from where: measuring the effect of food away from home on diet quality . Food Policy. 2009 ; 34 : 557 – 562 .
Alfieri M Pomerleau J Grace DM . A comparison of fat intake of normal weight, moderately obese and severely obese subjects . Obes Surg. 1997 ; 7 : 9 – 15 .
Satia-Abouta J Patterson RE Schiller RN , et al. Energy from fat is associated with obesity in U.S. men: results from the Prostate Cancer Prevention Trial . Prev Med. 2002 ; 34 : 493 – 501 .
Larson DE Hunter GR Williams MJ , et al. Dietary fat in relation to body fat and intraabdominal adipose tissue: a cross-sectional analysis . Am J Clin Nutr. 1996 ; 64 : 677 – 684 .
Cotton JR Burley VJ Weststrate JA , et al. Dietary fat and appetite: similarities and differences in the satiating effect of meals supplemented with either fat or carbohydrate . J Hum Nutr Diet. 2007 ; 20 : 186 – 199 .
Bennett C Reed GW Peters JC , et al. Short-term effects of dietary-fat ingestion on energy expenditure and nutrient balance . Am J Clin Nutr. 1992 ; 55 : 1071 – 1077 .
Horton TJ Drougas H Brachey A , et al. Fat and carbohydrate overfeeding in humans: different effects on energy storage . Am J Clin Nutr. 1995 ; 62 : 19 – 29 .
Raben A Agerholm-Larsen L Flint A , et al. Meals with similar energy densities but rich in protein, fat, carbohydrate, or alcohol have different effects on energy expenditure and substrate metabolism but not on appetite and energy intake . Am J Clin Nutr. 2003 ; 77 : 91 – 100 .
Griffiths AJ Humphreys SM Clark ML , et al. Immediate metabolic availability of dietary fat in combination with carbohydrate . Am J Clin Nutr. 1994 ; 59 : 53 – 59 .
Lammert O Grunnet N Faber P , et al. Effects of isoenergetic overfeeding of either carbohydrate or fat in young men . Br J Nutr. 2000 ; 84 : 233 – 245 .
Warwick ZS Schiffman SS . Role of dietary fat in calorie intake and weight gain . Neurosci Biobehav Rev. 1992 ; 16 : 585 – 596 .
Zandstra EH De Graaf C Meal DJ , et al. Short- and long-term effects of changes in pleasantness on food intake . Appetite. 2000 ; 34 : 253 – 260 .
De Castro JM Bellisle F Dalix A , et al. Palatability and intake relationship in free-living humans: characterization and independence of influence in North Americans . Physiol Behav. 2000 ; 70 : 343 – 350 .
De Castro JM Bellisle F Dalix A . Palatability and intake relationships in free-living humans: measurement and characterization in the French . Physiol Behav. 2000 ; 68 : 271 – 277 .
Yeomans MR Gray RW Mitchell CJ , et al. Independent effects of palatability and within-meal pauses on intake and appetite ratings in human volunteers . Appetite. 1997 ; 29 : 61 – 76 .
Sørensen LB Møller P Flint A , et al. Effect of sensory perception of foods on appetite and food intake: a review of studies on humans . Int J Obes. 2003 ; 27 : 1152 – 1166 .
Hunter JE Zhang J Kris-Etherton PM . Cardiovascular disease risk of dietary stearic acid compared with trans, other saturated, and unsaturated fatty acids: a systematic review . Am J Clin Nutr. 2010 ; 91 : 46 – 63 .
Mensink RP Zock PL Kester AD , et al. Effects of dietary fatty acids and carbohydrates on the ratio of serum total to HDL cholesterol and on serum lipids and apolipoproteins: a meta-analysis of 60 controlled trials . Am J Clin Nutr. 2003 ; 77 : 1146 – 1155 .
Parodi PW . Has the association between saturated fatty acids, serum cholesterol and coronary heart disease been over emphasized? Int Dairy J. 2009 ; 19 : 345 – 361 .
Astrup A Dyerberg J Elwood P , et al. The role of reducing intakes of saturated fat in the prevention of cardiovascular disease: where does the evidence stand in 2010? Am J Clin Nutr. 2011 ; 93 : 684 – 688 .
Risérus U Willett WC Hu FB . Dietary fats and prevention of type 2 diabetes . Prog Lipid Res. 2009 ; 48 : 44 – 51 .
Thanopoulou AC Karamanos BG Angelico FV , et al. Dietary fat intake as risk factor for the development of diabetes: multinational, multicenter study of the Mediterranean Group for the Study of Diabetes (MGSD) . Diabetes Care. 2003 ; 26 : 302 – 307 .
Wang L Folsom AR Zheng ZJ , et al. Plasma fatty acid composition and incidence of diabetes in middle-aged adults: the Atherosclerosis Risk in Communities (ARIC) study . Am J Clin Nutr. 2003 ; 78 : 91 – 98 .
Siri-Tarino PW Sun Q Hu FB , et al. Meta-analysis of prospective cohort studies evaluating the association of saturated fat with cardiovascular disease . Am J Clin Nutr. 2010 ; 91 : 535 – 546 .
Kurahashi N Inoue M Iwasaki M , et al. Dairy product, saturated fatty acid, and calcium intake and prostate cancer in a prospective cohort of Japanese men . Cancer Epidemiol Biomarkers Prev. 2008 ; 17 : 930 – 937 .
Gerber M . Background review paper on total fat, fatty acid intake and cancers . Ann Nutr Metab. 2009 ; 55 : 140 – 161 .
Matthäus B . Use of palm oil for frying in comparison with other high-stability oils . Eur J Lipid Sci Technol. 2007 ; 109 : 400 – 409 .
Berger KG . The use of palm oil in frying . In: Frying Oil Series . Selangor, Malaysia ; Malaysian Palm Oil Promotion Council. Available at: http://www.americanpalmoil.com/publications/Palm%20Oil%20in%20Frying.pdf . Accessed 22 March 2013 .
Vega-López S Ausman LM Jalbert SM , et al. Palm and partially hydrogenated soybean oils adversely alter lipoprotein profiles compared with soybean and canola oils in moderately hyperlipidemic subjects . Am J Clin Nutr. 2006 ; 84 : 54 – 62 .
Vessby B Uusitupa M Hermansen K , et al. Substituting dietary saturated for monounsaturated fat impairs insulin sensitivity in healthy men and women: the KANWU Study . Diabetologia. 2001 ; 44 : 312 – 319 .
Stender S Dyerberg J Astrup A . Fast food: unfriendly and unhealthy . Int J Obes. 2007 ; 31 : 887 – 890 .
Dunford E Webster J Barzi F , et al. Nutrient content of products served by leading Australian fast food chains . Appetite. 2010 ; 55 : 484 – 489 .
Mendis S Cruz-Hernandez C Ratnayake WM . Fatty acid profile of Canadian dairy products with special attention to the trans-octadecenoic acid and conjugated linoleic acid isomers . J AOAC Int. 2008 ; 91 : 811 – 819 .
Motard-Bélanger A Charest A Grenier G , et al. Study of the effect of trans fatty acids from ruminants on blood lipids and other risk factors for cardiovascular disease . Am J Clin Nutr. 2008 ; 87 : 593 – 599 .
Hulshof KF van Erp-Baart MA Anttolainen M , et al. Intake of fatty acids in Western Europe with emphasis on trans fatty acids: the TRANSFAIR Study . Eur J Clin Nutr. 1999 ; 53 : 143 – 157 .
Doell D Folmer D Lee H , et al. Updated estimate of trans fat intake by the US population . Food Addit Contam Part A Chem Anal Control Expo Risk Assess. 2012 ; 29 : 861 – 874 .
Kris-Etherton PM Lefevre M Mensink RP , et al. Trans fatty acid intakes and food sources in the U.S. population: NHANES 1999–2002 . Lipids. 2012 ; 47 : 931 – 940 .
Pot GK Prynne CJ Roberts C , et al. National Diet and Nutrition Survey: fat and fatty acid intake from the first year of the rolling programme and comparison with previous surveys . Br J Nutr. 2012 ; 107 : 405 – 415 .
Teegala SM Willett WC Mozaffarian D . Consumption and health effects of trans fatty acids: a review . J AOAC Int. 2009 ; 92 : 1250 – 1257 .
Mozaffarian D Aro A Willett WC . Health effects of trans-fatty acids: experimental and observational evidence . Eur J Clin Nutr. 2009 ; 63 ( Suppl 2 ): S5 – S21 .
Mozaffarian D Clarke R . Quantitative effects on cardiovascular risk factors and coronary heart disease risk of replacing partially hydrogenated vegetable oils with other fats and oils . Eur J Clin Nutr. 2009 ; 63 ( Suppl 2 ): S22 – S33 .
Esmaillzadeh A Azadbakht L . Home use of vegetable oils, markers of systemic inflammation, and endothelial dysfunction among women . Am J Clin Nutr. 2008 ; 88 : 913 – 921 .
Lopez-Garcia E Schulze MB Meigs JB , et al. Consumption of trans fatty acids is related to plasma biomarkers of inflammation and endothelial dysfunction . J Nutr. 2005 ; 135 : 562 – 566 .
Ganguly R Pierce GN . Trans fat involvement in cardiovascular disease . Mol Nutr Food Res. 2012 ; 56 : 1090 – 1096 .
Micha R Mozaffarian D . Trans fatty acids: effects on cardiometabolic health and implications for policy . Prostaglandins Leukot Essent Fatty Acids. 2008 ; 79 : 147 – 152 .
Laake I Pedersen JI Selmer R , et al. A prospective study of intake of trans-fatty acids from ruminant fat, partially hydrogenated vegetable oils, and marine oils and mortality from CVD . Br J Nutr. 2012 ; 108 : 743 – 754 .
Koh-Banerjee P Chu NF Spiegelman D , et al. Prospective study of the association of changes in dietary intake, physical activity, alcohol consumption, and smoking with 9-y gain in waist circumference among 16 587 US men . Am J Clin Nutr. 2003 ; 78 : 719 – 727 .
Mojska H . Influence of trans fatty acids on infant and fetus development . Acta Microbiol Pol. 2003 ; 52 ( Suppl ): 67 – 74 .
Mojska H Socha P Socha J , et al. Trans fatty acids in human milk in Poland and their association with breastfeeding mothers' diets . Acta Paediatr. 2003 ; 92 : 1381 – 1387 .
Anderson AK McDougald DM Steiner-Asiedu M . Dietary trans fatty acid intake and maternal and infant adiposity . Eur J Clin Nutr. 2010 ; 64 : 1308 – 1315 .
Wagner KH Plasser E Proell C , et al. Comprehensive studies on the trans fatty acid content of Austrian foods: convenience products, fast food and fats . Food Chem. 2008 ; 108 : 1054 – 1060 .
New South Wales Food Authority . Report on 2009 Trans Fatty Acid Survey – Analytical Results . A survey conducted under the Coordinated Food Survey Plan with participation from food regulatory jurisdiction in NSW, SA, WA and NZ. Food Standards Australia New Zealand, 2009 ; Available at: http://www.foodstandards.gov.au/_srcfiles/TFA_survey_analytical_2009.pdf . Accessed 19 March 2013.
Tyburczy C Delmonte P Fardin-Kia AR , et al. Profile of trans fatty acids (FAs) including trans polyunsaturated FAs in representative fast food samples . J Agric Food Chem. 2012 ; 60 : 4567 – 4577 .
Department of Health . Dietary Reference Values for Food Energy and Nutrients for the United Kingdom . Report of the Panel on DRVs of the Committee on Medical Aspects of Food Policy (COMA), Report of Health and Social Subjects No 41. London: The Stationary Office; 1991 .
National Institute for Health and Clinical Excellence . The Prevention of Cardiovascular Disease at a Population Level . 2010 ; Available at: http://www.nice.org.uk/nicemedia/pdf/CVDEP9TransFats.pdf . Accessed 19 March 2013.
Lichtenstein AH . New York City trans fat ban: improving the default option when purchasing foods prepared outside of the home . Ann Intern Med. 2012 ; 157 : 144 – 145 .
Angell SY Cobb LK Curtis CJ , et al. Change in trans fatty acid content of fast-food purchases associated with New York City's restaurant regulation: a pre-post study . Ann Intern Med. 2012 ; 157 : 81 – 86 .
Jaworowska A Blackham T Stevenson L . Nutritional composition of takeaway meals served by independent small outlets. [Abstract] . Proc Nutr Soc. 2011 ; 70 ( OCE4 ): E166 .
Jaworowska A Blackham T Stevenson L , et al. Determination of salt content in hot takeaway meals in the United Kingdom . Appetite. 2012 ; 59 : 517 – 522 .
Blackham T Abayomi JC Davies IG . Comparison of the nutritional quality of Indian takeaway and supermarket ready meals. [Abstract] . Proc Nutr Soc. 2012 ; 71 ( OCE2 ): E159 .
Stevenson L Jaworowska A Blackham T . Comparison of the nutritional quality of takeaway and ready to eat meals. [Abstract] . Proc Nutr Soc. 2011 ; 70 ( OCE4 ): E160 .
Pietinen P Valsta LM Hirvonen T , et al. Labelling the salt content in foods: a useful tool in reducing sodium intake in Finland . Public Health Nutr. 2007 ; 11 : 335 – 340 .
Leth T Jensen HG Mikkelsen AA , et al. The effect of the regulation on trans fatty acid content in Danish food . Atheroscler Suppl. 2006 ; 7 : 53 – 56 .
- trans fatty acids
Email alerts
Citing articles via.
- Recommend to your Library
Affiliations
- Online ISSN 1753-4887
- Print ISSN 0029-6643
- Copyright © 2024 International Life Sciences Institute
- About Oxford Academic
- Publish journals with us
- University press partners
- What we publish
- New features
- Open access
- Institutional account management
- Rights and permissions
- Get help with access
- Accessibility
- Advertising
- Media enquiries
- Oxford University Press
- Oxford Languages
- University of Oxford
Oxford University Press is a department of the University of Oxford. It furthers the University's objective of excellence in research, scholarship, and education by publishing worldwide
- Copyright © 2024 Oxford University Press
- Cookie settings
- Cookie policy
- Privacy policy
- Legal notice
This Feature Is Available To Subscribers Only
Sign In or Create an Account
This PDF is available to Subscribers Only
For full access to this pdf, sign in to an existing account, or purchase an annual subscription.
Americans' Perceptions about Fast Food and How They Associate with Its Consumption and Obesity Risk
Affiliations.
- 1 Systems-Oriented Global Childhood Obesity Intervention Program, Fisher Institute of Health and Well-being, College of Health, Ball State University, Muncie, IN.
- 2 Grand Forks Human Nutrition Research Center, US Department of Agriculture-Agricultural Research Service, Grand Forks, ND.
- 3 Department of Health Behavior and Policy, School of Medicine, Virginia Commonwealth University, Richmond, VA.
- 4 Department of Nutrition and Health Sciences, College of Health, Ball State University, Muncie, IN.
- PMID: 30084879
- PMCID: PMC6140449
- DOI: 10.1093/advances/nmy032
We aimed to systematically examine Americans' perceptions of fast food (FF) and how these perceptions might affect fast food consumption (FFC) and obesity risk. We searched PubMed and Google for studies published in English until February 17, 2017 that reported on Americans' perceptions (defined as their beliefs, attitudes, and knowledge) regarding FF as well as those on their associations with FFC and obesity risk. Thirteen articles met inclusion criteria. Limited research has been conducted on these topics, and most studies were based on convenience samples. A 2013 nationally representative phone survey of about 2000 subjects showed that one-fifth of Americans thought FF was good for health, whereas two-thirds considered FF not good. Even over two-thirds of weekly FF consumers (47% of the total population) thought FF not good. Americans seem to have limited knowledge of calories in FF. Negative and positive FF perceptions were associated with FFC. Those who consumed less FF seemed more likely to view FF negatively. When Americans valued the convenience and taste of FF and preferred FF restaurants with kid's menus and play areas, they were likely to purchase more FF. Available research indicates neither perceived availability of FF nor Geographical Information System (GIS)-based FF presence in the neighborhood has significant associations with weekly FFC. No studies examined potential links between FF perceptions and obesity risk. Americans' perceptions of FF and how they might associate with FFC and obesity risk are understudied. Considerable variation was observed in Americans' perceptions and FFC.
Publication types
- Systematic Review
- Consumer Behavior
- Cross-Sectional Studies
- Eating / psychology*
- Fast Foods / adverse effects*
- Feeding Behavior / psychology*
- Health Knowledge, Attitudes, Practice*
- Longitudinal Studies
- Obesity / etiology
- Obesity / psychology*
- Risk Assessment
- United States
- Young Adult
Grants and funding
- U54 HD070725/HD/NICHD NIH HHS/United States
The Effect of Fast Food Restaurants on Obesity and Weight Gain
We investigate the health consequences of changes in the supply of fast food using the exact geographical location of fast food restaurants. Specifically, we ask how the supply of fast food affects the obesity rates of 3 million school children and the weight gain of over 3 million pregnant women. We find that among 9th grade children, a fast food restaurant within a tenth of a mile of a school is associated with at least a 5.2 percent increase in obesity rates. There is no discernable effect at .25 miles and at .5 miles. Among pregnant women, models with mother fixed effects indicate that a fast food restaurant within a half mile of her residence results in a 1.6 percent increase in the probability of gaining over 20 kilos, with a larger effect at .1 miles. The effect is significantly larger for African-American and less educated women. For both school children and mothers, the presence of non-fast food restaurants is uncorrelated with weight outcomes. Moreover, proximity to future fast food restaurants is uncorrelated with current obesity and weight gain, conditional on current proximity to fast food. The implied effects of fast-food on caloric intake are at least one order of magnitude larger for students than for mothers, consistent with smaller travel cost for adults.
The authors thank John Cawley, the editor, two anonymous referees and participants in seminars at the NBER Summer Institute, the 2009 AEA Meetings, the ASSA 2009 Meetings, the Federal Reserve Banks of New York and Chicago, the FTC, the New School, the Tinbergen Institute, UC Davis, the Rady School at UCSD, and Williams College for helpful comments. We thank Joshua Goodman, Cecilia Machado, Emilia Simeonova, Johannes Schmeider, and Xiaoyu Xia for excellent research assistance. We thank Glenn Copeland of the Michigan Dept. of Community Health, Katherine Hempstead and Matthew Weinberg of the New Jersey Department of Health and Senior Services, and Rachelle Moore of the Texas Dept. of State Health Services for their help in accessing the data. The authors are solely responsible for the use that has been made of the data and for the contents of this article. The views expressed herein are those of the author(s) and do not necessarily reflect the views of the National Bureau of Economic Research.
MARC RIS BibTeΧ
Download Citation Data
- February 10, 2009
Non-Technical Summaries
- Do Fast Food Restaurants Contribute to Obesity? Author(s): Janet Currie Stefano DellaVigna Enrico Moretti Vikram Pathania Over the past thirty years, the prevalence of obesity and obesity-related diseases in the U.S. has risen sharply. Since the early 1970s,...
Published Versions
Mentioned in the news, more from nber.
In addition to working papers , the NBER disseminates affiliates’ latest findings through a range of free periodicals — the NBER Reporter , the NBER Digest , the Bulletin on Retirement and Disability , the Bulletin on Health , and the Bulletin on Entrepreneurship — as well as online conference reports , video lectures , and interviews .
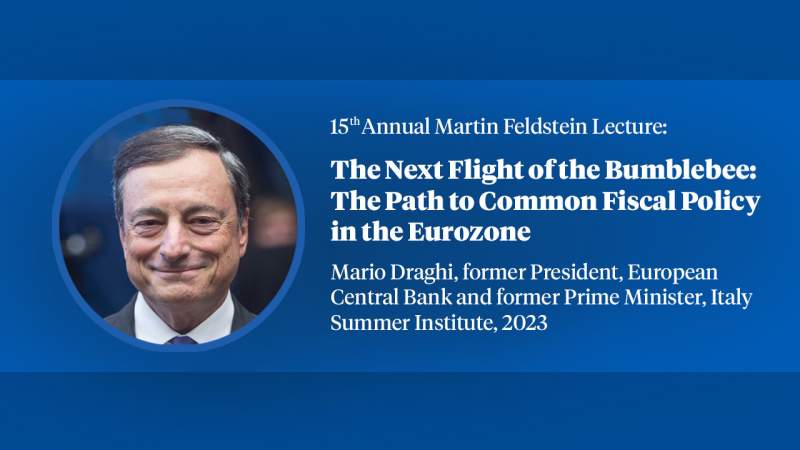
Academia.edu no longer supports Internet Explorer.
To browse Academia.edu and the wider internet faster and more securely, please take a few seconds to upgrade your browser .
Enter the email address you signed up with and we'll email you a reset link.
- We're Hiring!
- Help Center
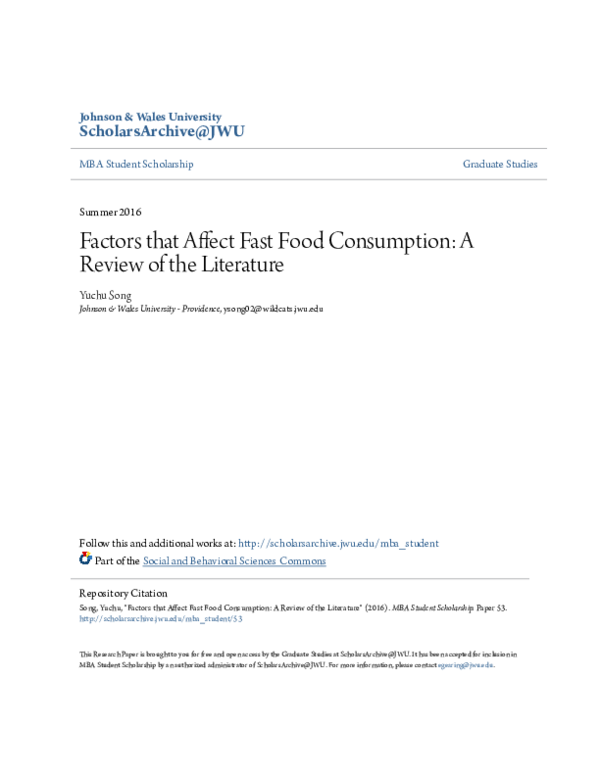
Factors that Affect Fast Food Consumption: A Review of the Literature

Related Papers
American Journal of Health Promotion
Emily Greenberg
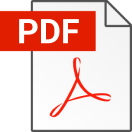
Consumption of takeaway and fast food by young adolescents is no longer confined to the developed countries; it has spread to the developing countries as well. The culture of fast food consumption has replaced the traditional meal among university students and is a great public health concern. Excessive consumption of fast food is responsible for obesity epidemics and the cause of a dramatic increase of obesity-related diseases. A cross-sectional study was carried out from March to December, 2015 among students attending in five. The aim of the present study was to examine the preference, prevalence and pattern of fast food consumption among the students. The prevalence of fast food consumption among those students was 98.5%, and 43.3% of their pocket money was spent on its purchase. The important factors for the preference of fast food include good taste, easy accessibility, increased convenience, and pocket friendly in nature. Approximately 22% of the respondents mentioned that they consumed fast food 4 days per week and more than one-fifth had the meal every day. Fifty four percent of the respondents skipped their breakfast due to a variety of reasons including class pressure and had fast food after finishing their classes, either from varsity canteens or other fast-food outlets. Though 98% of the students were well informed about the negative effects associated with excessive fast food consumption, they were still profoundly addicted to it. Specific health education programs, dietary guidelines and effective public awareness campaigns could be initiated to address the unhealthy lifestyle of university students and improve their health.
Jason A Gilliland
This investigation sought to gain an understanding of how youth perceive neighborhood environmental influences on their physical activity and eating behaviors. This qualitative study targeted a heterogeneous sample of 12- to 14-year-olds in London, Ontario, Canada. Using a semi-structured interview guide, we conducted nine focus groups (n = 60) and used inductive content analysis to investigate their discussions. Most participants discussed their school, parks, and opportunity structures around their homes as influencing their physical activity, and overwhelmingly reported the availability of fast-food restaurants, convenience stores, and slow-food restaurants in their neighborhoods as influencing their eating practices. The descriptive information collected through this study is potentially significant for the efforts of city planners, policy makers, health professionals, school officials, and parents to promote and support healthier youth behaviors.
This study examined the relationship between the neighborhood food environment and the food purchasing behaviors among adolescents. Grade 7 and 8 students (n = 810) at 21 elementary schools in London, Ontario, Canada completed a questionnaire assessing their food purchasing behaviors. Parents of participants also completed a brief questionnaire providing residential address and demographic information. A Geographic Information System (GIS) was used to assess students’ home and school neighborhood food environment and land use characteristics. Logistic regression analysis was conducted to assess the influence of the home neighborhood food environment on students’ food purchasing behaviors, while two-level Hierarchical Non-Linear Regression Models were used to examine the effects of school neighborhood food environment factors on students’ food purchasing behaviors. The study showed that approximately 65% of participants reported self-purchasing foods from fast-food outlets or convenience stores. Close proximity (i.e., less than 1 km) to the nearest fast-food outlet or convenience store in the home neighborhood increased the likelihood of food purchasing from these food establishments at least once per week by adolescents (p < 0.05). High fast-food outlet density in both home and school neighborhoods was associated with increased fast-food purchasing by adolescents (i.e., at least once per week; p < 0.05). In conclusion, macro-level regulations and policies are required to amend the health-detracting neighborhood food environment surrounding children and youth’s home and school
Caribbean Public Health Agency
Caroline Allen
Ecology of Food and Nutrition
Ashley Walvoord
Obesity Reviews
Natalie Parletta
Marla Reicks , Jinan Banna , Siew Wong
Among early adolescents (10–14 years), poor diet quality along with physical inactivity can contribute to an increased risk of obesity and associated biomarkers for chronic disease. Approximately one-third of United States (USA) children in this age group are overweight or obese. Therefore, attention to factors affecting dietary intake as one of the primary contributors to obesity is important. Early adolescents consume foods and beverages during eating occasions that occur with and without parental supervision. Parents may influence eating behaviors of early adolescents during eating occasions when they are present or during independent eating occasions by engaging in practices that affect availability of foods and beverages, and through perceived normative beliefs and expectations for intake. Therefore, the purpose of this article was to describe the influence of parenting practices on eating behaviors in general and when specifically applied to independent eating occasions of early adolescents. This information may be helpful to inform parenting interventions targeting obesity prevention among early adolescents focusing on independent eating occasions.
Vivica Kraak , Tessa Englund
This study identified and adapted choice-architecture frameworks to develop a novel framework that restaurant owners could use to promote healthy food environments for customers who currently overconsume products high in fat, sugar and sodium that increase their risk of obesity and diet-related non-communicable diseases (NCDs). This review was conducted in three steps and presented as a narrative summary to demonstrate a proof of concept. Step one was a systematic review of nudge or choice-architecture frameworks used to categorize strategies that cue healthy behaviors in microenvironments. We searched nine electronic databases between January 2000 and December 2016 and identified 1,244 records. Inclusion criteria led to the selection of five choice-architecture frameworks, of which three were adapted and combined with marketing-mix principles to highlight eight strategies (i.e., place, profile, portion, pricing, promotion, healthy default picks, prompting or priming and proximity). Step two involved conducting a comprehensive evidence review between January 2006 and December 2016 to identify U.S. recommendations for the restaurant sector organized by strategy. Step three entailed developing 12 performance metrics for the eight strategies. This framework should be tested to determine its value to assist restaurant owners to promote and socially normalize healthy food environments to reduce obesity and NCDs.
Health education & behavior : the official publication of the Society for Public Health Education
This study tested the relationships among child executive function (EF), child-perceived parent fast food intake, and child self-reported subsequent consumption of high-calorie, low-nutrient (HCLN) food. One year and 6-month longitudinal observation from a larger randomized controlled trial. Southern California elementary schools. Fourth- and fifth-grade children (N = 1,005) participating in the Pathways to Health obesity prevention program. Child EF problems were associated with higher concurrent HCLN intake (B = 0.29, SE = 0.10, p < .001) and had a significant indirect effect through higher perceived frequency of parent fast food intake (indirect effect = 0.17, 95% confidence interval [CI] = [0.11, 0.25], p < .001). Longitudinally, child EF problems did not significantly predict higher HCLN intake a year and a half later (B = 0.01, SE = 0.10, p = .92, n = 848) but did have a significant indirect effect through higher perceived parent fast food intake (indirect effect = 0.05,...
RELATED PAPERS
Rafael Monge-Rojas
Dr. Sadguna Anasuri
Vanessa Smith-Castro , Rafael Monge-Rojas
Carlos Penilla , Elena Flores
Disha katyan
Turkish Pizza
Nutrition Reviews
Richard R . Rosenkranz
Nutrition reviews
ozge sensoy bahar
International Food …
Argelia Lorence
Ahmed Yasir
Journal of Public Policy & Marketing
Sonya Grier
Geographies of Children and Young People
Kate Cairns
Edward Orehek
Journal of Consumer Affairs
Marie-Eve Laporte , Géraldine Michel
The Future of Children
Marianne McGarry Wolf
Nalini Ranjit
Health Promotion Practice
Cheryl Perry , Leslie Lytle
Joanne Guthrie , Lisa Mancino , Biing-hwan Lin
Consumer & Nutrition
SUZANNE M PISCOPO
Monique Raats
Mallory Koenings , Colleen Delaney , C. Byrd-bredbenner
verzhine duryan
BMC Public Health
Heather Lillico
Koen Lemmink
Applied physiology, nutrition, and metabolism = Physiologie appliquée, nutrition et métabolisme
Ian Janssen
IJSRP Journal
Noemi Mejia
Atanda Projectmaster
Health & Place
Marilyn Tseng
Health and Place
Ann Forsyth
Journal of Business Research
Elizabeth Howlett
Annual Review of Public Health
Catalina Velasquez
Juliana Kain
Johnrich Misa
Preventive Medicine
Rebecca Roberts
Annual Review of Clinical Psychology
Natasha Schvey
RELATED TOPICS
- We're Hiring!
- Help Center
- Find new research papers in:
- Health Sciences
- Earth Sciences
- Cognitive Science
- Mathematics
- Computer Science
- Academia ©2024
fast food consumption Recently Published Documents
Total documents.
- Latest Documents
- Most Cited Documents
- Contributed Authors
- Related Sources
- Related Keywords
Factors influencing fast food consumption: a case study of University of Venda Students, Limpopo, South Africa
Eating fast foods more than twice per week is associated with more weight gain and insulin resistance in otherwise healthy young adults. However many consumers see fast foods as symbols of wealth, progress and well-ordered openness of western society. Studies reveal that fast food consumption is the dominant dietary pattern among children and young adults today. However there is little data on the factors influencing fast food consumption in South Africa. Therefore this research paper focuses on factors influencing fast food consumption in South Africa. This is an empirical data which was obtained using exploratory and descriptive research design. Data was collected through unstructured interview of focus groups. The interview involved a direct interaction between the researcher and a group. Participants reported to use fast foods due to the perceived lack of time to prepare one’s own meal. Fast food consumption was found to be more frequent among participants especially during lunch times. The following findings were recorded as factors influencing fast food: Lack of time, change in living conditions, lack of cooking skills & cooking utensils, fun & cravings, socializing and convenience.
“Two quid, chicken and chips, done”: understanding what makes for young people’s sense of living well in the city through the lens of fast food consumption
Determinants of healthy diet choices during austerity in greece.
PurposeThe purpose of this paper is to investigate the key determinants of dietary choices of the Greek population during a period of financial austerity.Design/methodology/approachData from the 2016 “Health and Welfare” Greek national cross-sectional survey, in a representative sample of 2,003 individuals, were examined. The survey was conducted via computer-assisted telephone interviews. Sociodemographic characteristics and diet knowledge were examined as potential determinants of four dietary behaviours (fruit, fish, red meat and fast food consumption).FindingsFindings showed significant gender differences against men (64.4% were overweight and obese; 57.6% and 18.4% reported red meat and fast food consumption more than twice a week, respectively). Age and financial affordability were the most significant determinants of fruit consumption. Fish consumption was determined by age, financial affordability, and family status (unmarried, living with the parents). Gender, age, family status (unmarried, living alone), employment status (unemployed) and social support affected red meat consumption. Finally, factors influencing fast food consumption were gender, age and employment status (unemployed).Originality/valueThis research incorporates unique and original insight in the determinants of healthy dietary choices during the austerity measures in Greece. Findings could contribute to a better understanding of the main factors that influence healthy eating and help develop policies to encourage healthy dietary lifestyles for the general public.
HUBUNGAN PENGARUH TEMAN DENGAN FREKUENSI KONSUMSI FAST FOOD MODERN PADA MAHASISWA UNIVERSITAS XYZ DI KOTA DEPOK
ABSTRACTExcessive consumption of fast food can increase the incidence of obesity and other health problems in adolescents. Eating fast food more than twice per week can increase BMI z-score significantly, compared to eating fast food once a week or not eating fast food at all. This study aims to determine the relationship between the influence of friends with the frequency of modern fast food consumption among students XYZ University in Depok. The research method used is quantitative with cross-sectional study design conducted to 148 students XYZ University Depok selected by systematic random sampling. It used questionnaire about individual characteristics, food characteristics, and environmental characteristics, while data of frequency fast food consumption from FFQ. Result showed that 51,4% of respondents consumed fast food often. Furthermore, there is a difference proportion in the influence of friends (p = 0.001) in determining the frequency of fast food consumption. Students with strong friend influences are 3.6 times more likely to eat fast food more often compared to poor friend influences. ABSTRAKKonsumsi fast food yang berlebihan dapat meningkatkan kejadian obesitas dan masalah kesehatan lainnya pada remaja. Mengonsumsi fast food lebih dari dua kali per minggu dapat meningkatkan BMI z-score secara signifikan, dibandingkan dengan yang mengonsumsi fast food seminggu sekali atau tidak mengonsumsi fast food sama sekali. Penelitian ini bertujuan untuk mengetahui hubungan faktor pengaruh teman dengan frekuensi konsumsi fast food modern pada mahasiswa Universitas XYZ di Kota Depok. Metode penelitian yang digunakan adalah kuantitatif dengan desain studi cross-sectional yang dilakukan kepada 148 mahasiswa Universitas XYZ yang dipilih dengan systematic random sampling. Data karakteristik diperoleh dari kuesioner, sedangkan data frekuensi konsumsi fast food diperoleh dari FFQ. Hasil penelitian ini menunjukkan sebanyak 51,4% responden mengonsumsi fast food dengan frekuensi sering. Terdapat perbedaan proporsi pada pengaruh teman (p=0,001) dalam menentukan frekuensi konsumsi fast food. Mahasiswa dengan pengaruh teman yang kuat berpeluang 3,6 kali lebih sering mengonsumsi fast food dibandingkan mahasiswa dengan pengaruh teman lemah.
Stakeholder perceptions of strategies to reduce fast food consumption in Cambodian adults
Background: Fast food consumption is one of the major contributing factors effecting overweightness and obesity, leading to many non-communicable diseases. Therefore, the purpose of this study was to determine strategies for reducing the fast-food consumption of Cambodian adults. Methods: This qualitative study was conducted among adults in Phnom Penh city, Cambodia, in 2018. 10 stakeholders were included from different institutions in Cambodia, mostly health institutions. The tools used in this study were a multidisciplinary meeting with stakeholders and the completion of observation forms. Using a semi-structured questionnaire, data were collected, and a thematic analysis was used. Results: Stakeholders’ viewpoints followed three identifiable themes with regard to approaches to reduce fast-food consumption among Cambodian adults. These comprised: (1) health education and health promotion (focusing on educational institutions), (2) reducing the availability and marketing impact of fast-food, and (3) implementing government policy. Conclusions: Knowing the important contributors to reduce the consumption of the fast food among Cambodia adults was the first priorities for all policy makers and other stakeholders to take action. This study provided essential findings for improving the decision-making abilities of those preparing strategy and policy for reducing fast-food consumption.
Eating Out: Fast Food Consumption and its Impact on Health of Young Generation of Karachi
This paper has measured the impacts of fast food on the health of the young generation. In today’s age where both the genders are working as bread earners of the family, people prefer fast foods because of the convenience factor and eating fast food is affecting and influencing the health of the young generation. The core population, who consume fast food the most frequently is teenage youth. The study is based on value expectancy theory and attempts to develop and test a theory-based questionnaire that identifies factor relating to fast food consumption and impact on health. In this qualitative study, data is collected by interviews with seven persons. The predetermine open-ended questions had been made to investigate the thoughts of respondents and indicate the key meanings that each participant had described his or her experiences. After conducting interviews from participants then identified relevant themes and are further discussed in the results. All findings are supported by the literature review that is related to this study. Moreover, the qualitative study ends with some recommendations to the young generation.
Factors Influencing the Consumption of Fast Food among Young Adults
Background: The habit of consuming the fast- foods and packed foods are increased among the people in developing countries. Besides, several studies showed that the fast- food eating brings higher risks for developing the physiological imbalances such as obesity, over – weight even in younger generation. The fast foods ensure for important features such as easy availability, tastes good, convenient, and has friendly- packing than the traditional food items. Due to such kind of life style modifications, the ratio of fast – food habitats in younger and adolescents are highly increasing in compare to the older and middle aged people. The increase is inevitable in different age groups in recent years. These food habit modifications make a turn in traditional systems and attract the great attention against the public health concern. Objective: The present study aimed to investigate the factors that influence the fast-food consumption in Tamil Nadu and Puducherry Methods: A cross-sectional study was carried out to examine the preference, prevalence and pattern of fast food consumption among the young adults. Results and Discussion: The study showed that many of the students unavoidably skipping the morning foods due to study pressure, morning – hurry and they had them at late- morning hours from the fast- foods outlets such as canteens or other hotels. Though, 90.7% of the students know the importance and negative consequences of break- fast skipping that are related to consumption of excessive fast- food habits, they have been severely addicted with the fast- foods. Conclusion: As a conclusion, the present study showed the importance of initiating the specific health programs, dietary guidelines and effective awareness for addressing the unhealthy issues related with the fast- food consumption among the student community.
Consumption of Sugary Drinks and Alcohol, and Factors Associated with Increased Fast-Food Consumption Among Adults, During Panama’s COVID-19 Lockdown
1500determinants of dietary behaviour and physical activity in australian adolescents.
Abstract Background The increased prevalence of obesity, unhealthy diet and sedentary lifestyles among Australian adolescents has become a public health concern. This study investigated the associations between socio-economic status, screen-time, social networks, sleep, and wellbeing and subsequent health behaviours. Methods A cohort of 582 adolescents aged 13-19 years from Sydney were prospectively followed for 22-weeks (8 November 2019 – 19 April 2020). Study factors were collected via mobile phone app using weekly ecological momentary assessments (EMAs). Multi-level mixed effects logistic regression model assessed associations between baseline characteristics of participants, socio-economic status, screen-time, sleep, and wellbeing and subsequent dietary behaviour and physical activity. Results TV watching (odds ratio [OR]=1.22, 95% CI: 0.98-1.5), social media and internet use (OR = 1.22, 95% CI: 0.97-1.53) were associated with fast food consumption. Participants who spent more than four hours a day on social media were also less likely to be physically active for ³60 minutes (OR = 0.75, 95% CI: 0.6-0.94). Fast food consumption was associated with lower vegetable (OR = 0.61, 95% CI: 0.43-0.85) and fruit intake (OR = 0.78, 95% CI: 0.61-1.01), and was also more prevalent in participants with a job (OR = 1.42, 95% CI: 1.09-1.86). There was no substantial effect of psychological distress, positive emotions, social relationships and sleep on any health behaviour. Conclusions The present study has shown that lifestyle behaviours had a stronger effect on dietary behaviour and physical activity than some of the socio-demographic factors. Key messages Future studies with a balanced sample in terms of sex, age and cultural background are needed.
Internal Influences on Consumer Behavior of Burgarooh Restaurant: A Case in the Fast Food Industry of Oman
Internal Influences on Consumer Behavior of Burgarooh Restaurant: A Case in the Fast Food Industry of Oman Nasser Juma Al Harthi MBA, Middle East College Email: [email protected] Dr. Maria Teresa Matriano Assistant Professor, Department of Post-Graduate Studies, Middle East College, Oman Email: [email protected] Abstract Purpose:- The Sultanate of Oman is one of the countries where the restaurant and fast food sectors are active. The study aims to analyze the factors affecting customers' choice of fast food. This research measures both personal and psychological elements on buying fast food in the Sultanate of Oman. This project is concerned with the internal influences of personal factors and factors that interact with the individual consumer. Design / methodology / approach:- The research has attempted to sample 486 customers of the restaurant from its various branches and used survey questionnaire and online interviews for data collection. Interviews were facilitated with group of customers in the restaurant branches in the Governorate of Muscat. Findings:- The study concluded that the most critical factor in choosing a burger for the consumer is that it is a means for family and friends' participation by 44%. In comparison, 43% of the participants considered that the preparation speed is one of the main factors in choosing burger meals. The study also indicated that the product's price is one of the least influencing factors for selecting the product. Moreover, the study has also concluded that fast food consumption is based on community participation and accessibility rather than direct personal choices. Research limitation / Implications:- The study indicated that product's price is the least influencing factors for selecting the product and concluded that fast food consumption is based on community participation and accessibility rather than direct personal choices. Originality / value:- There are no previous studies conducted on internal influences on consumer behavior. The study places psychological factors, which represent convictions and beliefs, at the forefront of the factors affecting the consumer's buying decision.
Export Citation Format
Share document.

An official website of the United States government
The .gov means it’s official. Federal government websites often end in .gov or .mil. Before sharing sensitive information, make sure you’re on a federal government site.
The site is secure. The https:// ensures that you are connecting to the official website and that any information you provide is encrypted and transmitted securely.
- Publications
- Account settings
Preview improvements coming to the PMC website in October 2024. Learn More or Try it out now .
- Advanced Search
- Journal List
- Iran J Med Sci
- v.46(6); 2021 Nov
Fast Food and Fast Research: Life-threatening Phenomena
Mojtaba kafi.
1 Department of Animal Reproduction, School of Veterinary Medicine, Shiraz University, Shiraz, Iran
Seyed Ali Enjoo
2 Medical Ethics Department, School of Traditional Medicine, Shahid Beheshti University of Medical Sciences, Tehran, Iran
Dear Editor
The surge in fast food consumption in recent years is considered a threat to human health. This change in the life habit has raised serious concerns among health policy-makers and medical nutrition researchers. Environmental stress, multitasking, low physical activity, and low academic achievement have been shown to influence the predilection for consuming fast food. 1 We have now faced with one similarly threatening phenomenon among university students and academics, which can be termed “fast research”.
Social pressures, as well as the fear of losing job opportunities, are the two main factors that propel university students to urgently seek a thesis supervisor, so that they can perform their research project in a short time, get published, and then secure a position. The occurrence of the social crisis associated with the emergence of the COVID-19 pandemic has caused depression and anxiety spikes in many postgraduate students as reported by Chirikov and colleagues. 2 This, in turn, may result in conducting fast research programs by students and academics to enable them to publish their papers in a short time. One best example of fast research and publication is certainly the crisis of the COVID-19 pandemic. Web of Science and Scopus indexed more than 23,600 articles on the COVID-19 pandemic published in the first half of 2020. This number included a plethora of research articles, reviews, notes, letters, and editorials related to COVID-19, covering every subject from virology to epidemiology. 3 A rush in, or lack of, the peer-review process, publishing COVID-19 papers in non-specialized journals, and detection of duplicate published images are all telltale signs of fast research. 3 , 4 More than half of the medical research has been reported as useless due to poor framing of the study questions, poor study design, scientific misconduct, and poor reporting of results. 5 Many of these problems are thought to be exacerbated in COVID-19 research. 4 , 6 Furthermore, it has been reported that most early publications on COVID-19 did not contain new information as compared to the H1N1 swine influenza pandemic that occurred in 2009. 7 This is another facet of the fast research and publication phenomena, which hampers the acquisition of valid applied knowledge, but might serve the researcher’s career interests. These issues and the trying conditions of the COVID-19 era can push the researchers and other people towards a point of no return, akin to the event horizon of a black hole. Fast research may lead university students and academics to underthinking and irresponsibility.
Fast food and fast research are both easy to prepare and relatively inexpensive and are favored by people across age and gender divides. Even though they are sometimes stale, they can save people’s lives in an emergency; however, if resorted to frequently, both would deleteriously influence our life in the end.
Conflict of Interest
None declared.

IMAGES
VIDEO
COMMENTS
Because of the limited access to supermarkets, they eat more unhealthy fast and processed foods and end up having 7 times the risk of early-life stroke (before age 45), putting people in nursing homes in their 30s, 40s, and 50s. 4 - 7. The vulnerable poor in these areas also have double the risk of heart attack, double the risk of diabetes, and ...
Fast food has become a significant portion of the world's diet. For example, Table 1 shows the rapid increase in consumption in the United States across all age groups. In the 1970s, an average US adult (aged 18-65 y) consumed fast food on <10% of days, but this had risen to 40.7% of days in 2017-2018. Among US survey participants aged 12 ...
Consumption of fast foods t wo times or more per. week has been associa ted with 31% highe r. prevalen ce of moderate abdominal obesity in men. and 25% higher preval ence in women 70. Obesity is ...
To determine whether fast food consumption is associated with consuming a Western dietary pattern for the remainder of intake, we used logistic regression to estimate the odds of consuming a Western dietary pattern for low and high fast food consumers compared with fast food nonconsumers. We examined effect measure modification of this ...
Introduction. Consumption of fast food (FF, food being mass-produced and served quickly) is common in the United States and many other industrialized countries, and it has been increasing steadily in some developing countries as well owing to factors such as its convenience, low cost, consistent taste, easy access through a variety of restaurant chains, and the FF industry's marketing effort ...
Previous research has identified a strong positive association between the availability of fast food and its consumption as well as fast food consumption and obesity outcomes [5, 8, 10, 14, 15]. However, some studies assessed the fast food consumption on the general obesity based on Body Mass Index (BMI) [ 5 , 8 , 10 , 16 ].
The sample was divided into two groups according to their fast food consumption. Group 1 included those who consumed fast food 3 times per week or less (n = 35; men = 30, women = 5), and the participants' mean age was 21.23 years. Group 2 included those who consumed fast food more than 3 times per week (n = 25; men = 21, women = 4).
Background Today, with the advancement of science, technology and industry, people's lifestyles such as the pattern of people's food, have changed from traditional foods to fast foods. The aim of this survey was to examine and identify factors influencing intent to use fast foods and behavior of fast food intake among students based on the theory of planned behavior (TPB). Methods A cross ...
We find that a 10% increase in exposure to fast food outlets in mobile environments increases individuals' odds of visitation by 20%. Using our results, we simulate multiple policy strategies ...
REVIEW ARTICLE. Fast Foods and their Impact on Health. Ashakiran 1* & Deepthi R. 1 Department of Biochemistry, 2Department of Community Medicine, Sri Devaraj Urs Medical College, Kolar- 563101 ...
Abstract. Consumption of takeaway and fast food continues to increase in Western societies and is particularly widespread among adolescents. Since food is known to play an important role in both the development and prevention of many diseases, there is no doubt that the observed changes in dietary patterns affect the quality of the diet as well as public health.
Americans seem to have limited knowledge of calories in FF. Negative and positive FF perceptions were associated with FFC. Those who consumed less FF seemed more likely to view FF negatively. When Americans valued the convenience and taste of FF and preferred FF restaurants with kid's menus and play areas, they were likely to purchase more FF.
We aimed to systematically examine Americans' perceptions of fast food (FF) and how these perceptions might affect fast food consumption (FFC) and obesity risk. We searched PubMed and Google for studies published in English until February 17, 2017 that reported on Americans' perceptions (defined as their beliefs, attitudes, and knowledge) regarding FF as well as those on their associations ...
Fast food tends to be high in salt, sugar, saturated fats, trans fats, calories, and processed preservatives and ingredients. A wealth of well-conducted research has proven the negative health ...
nutritional value", "obesity" and "additives". Now it is a common sense that fast food. is harmful to body fitness. To be more specific, a study named "Local Concentration of. Fast-Food Outlets Is Associated with Poor Nutrition and Obesity" was conducted in. May/ June 2014 by Daniel J. Kruger and his colleagues.
Specifically, we ask how the supply of fast food affects the obesity rates of 3 million school children and the weight gain of over 3 million pregnant women. We find that among 9th grade children, a fast food restaurant within a tenth of a mile of a school is associated with at least a 5.2 percent increase in obesity rates.
Fast food chains seem to keep up with the increasing demands of the public for inexpensive and accessible food. The ASPBI reports that fast-food chains led second in the formal sector with a total of 4,477 establishments. That is 16.6% of the formal sector. The fast food industry has been growing over the past few decades and
There is an absence of public health social science research that examines chicken shops as a discrete sub-type of fast food outlet. Research and commentary that directly addresses the ... was to conflate 'health' with food hygiene. In effect, reframing a healthy diet to include fast food as long as the fast food places are clean or ...
food" mainly refers to the ability to provide customers with convenient use of food delivery services.2.2"Characteristics of "take-. out fast food. 3.2.1 Convenient and fast. Convenience and speed ...
Therefore, the objective of this study was to test the effect of the presence of health information and its integration into a fast food menu context on young adults' healthy food choices. An experimental between-subjects design consisting of three conditions—subtle, explicit, and no health information—was conducted among 142 participants ...
While many studies concerning people's fast food consumption have been conducted and are based on different stand points, existing research has so far rarely provided a comprehensive view of fast food phenomenon. Furthermore, this paper provides a brief review of motives for people's fast food consumption, based on this, more research on ...
Eating fast foods more than twice per week is associated with more weight gain and insulin resistance in otherwise healthy young adults. However many consumers see fast foods as symbols of wealth, progress and well-ordered openness of western society. Studies reveal that fast food consumption is the dominant dietary pattern among children and ...
Dear Editor. The surge in fast food consumption in recent years is considered a threat to human health. This change in the life habit has raised serious concerns among health policy-makers and medical nutrition researchers. Environmental stress, multitasking, low physical activity, and low academic achievement have been shown to influence the ...