Online ordering is currently unavailable due to technical issues. We apologise for any delays responding to customers while we resolve this. For further updates please visit our website: https://www.cambridge.org/news-and-insights/technical-incident
We use cookies to distinguish you from other users and to provide you with a better experience on our websites. Close this message to accept cookies or find out how to manage your cookie settings .

Login Alert

- > Journals
- > Epidemiology & Infection
- > Volume 148
- > Application of ordinal logistic regression analysis...
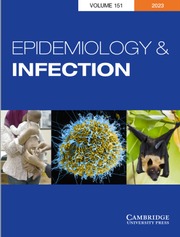
Article contents
Introduction, application of ordinal logistic regression analysis to identify the determinants of illness severity of covid-19 in china.
Published online by Cambridge University Press: 07 July 2020
- Supplementary materials
Corona Virus Disease 2019 (COVID-19) has presented an unprecedented challenge to the health-care system across the world. The current study aims to identify the determinants of illness severity of COVID-19 based on ordinal responses. A retrospective cohort of COVID-19 patients from four hospitals in three provinces in China was established, and 598 patients were included from 1 January to 8 March 2020, and divided into moderate, severe and critical illness group. Relative variables were retrieved from electronic medical records. The univariate and multivariate ordinal logistic regression models were fitted to identify the independent predictors of illness severity. The cohort included 400 (66.89%) moderate cases, 85 (14.21%) severe and 113 (18.90%) critical cases, of whom 79 died during hospitalisation as of 28 April. Patients in the age group of 70+ years (OR = 3.419, 95% CI: 1.596–7.323), age of 40–69 years (OR = 1.586, 95% CI: 0.824–3.053), hypertension (OR = 3.372, 95% CI: 2.185–5.202), ALT >50 μ/l (OR = 3.304, 95% CI: 2.107–5.180), cTnI >0.04 ng/ml (OR = 7.464, 95% CI: 4.292–12.980), myohaemoglobin>48.8 ng/ml (OR = 2.214, 95% CI: 1.42–3.453) had greater risk of developing worse severity of illness. The interval between illness onset and diagnosis (OR = 1.056, 95% CI: 1.012–1.101) and interval between illness onset and admission (OR = 1.048, 95% CI: 1.009–1.087) were independent significant predictors of illness severity. Patients of critical illness suffered from inferior survival, as compared with patients in the severe group (HR = 14.309, 95% CI: 5.585–36.659) and in the moderate group (HR = 41.021, 95% CI: 17.588–95.678). Our findings highlight that the identified determinants may help to predict the risk of developing more severe illness among COVID-19 patients and contribute to optimising arrangement of health resources.
The pandemic of the novel corona virus disease 2019 (COVID-19), which originally emerged in Wuhan, China in December 2019 has spread around the world [ Reference Huang 1 , Reference Chen 2 ]. As of 5 June 2020, the WHO has reported a total of 6 535 354 COVID-19 cases and 387 155 deaths globally, with an average mortality of 5.92% and the person-to-person transmission is still continuing [ 3 ]. The clinical spectrum of COVID-19 appears to be wide, ranging from asymptomatic infection to mildly, severely, critically ill cases. Mild patients present only upper respiratory tract symptoms like cough and fever, however, respiratory failure, acute respiratory distress syndrome, heart failure, septic shock and even death can be observed in patients with critical conditions [ Reference Zhou 4 ]. Although most confirmed patients (81%) were classified as mild or moderate, 14% were severe and 5% were critical according to the largest investigation of 72 314 cases to date [ Reference Wu 5 ]. Accumulated evidences have indicated that older age, male, smoking, comorbidity, neutrophilia, coagulopathy, elevated D-dimer level and organ dysfunction were associated with increased risk of death from COVID-19 [ Reference Wu 5 – Reference Wang 10 ]. However, investigations of determinants of severity of COVID-19 are scarce. Early detecting cases with the potential deterioration of disease will contribute to optimising the use of limited health resources and allocating the proper care. Liang et al . developed a clinical risk score to predict the occurrence of critical COVID-19 based on severe or non-severe [ Reference Liang 11 ]. To our knowledge, no previous studies have been conducted to investigate the risk factors of severity of COVID-19 based on ordinal response, namely moderate, severe and critical illness. The estimation of risk factors of disease severity is therefore not very robust.
Here, we conducted a retrospective study based on COVID-19 patients from four designated hospitals in Shanghai, Hubei and Anhui provinces to describe the clinical features of COVID-19, and aimed to identify the predictors of multi-level response of severity from moderate, severe to critical illness.
Study design and participants
This multi-centre retrospective study encompassed COVID-19 patients classified as being moderately, severely and critically ill. The illness severity of COVID-19 was defined according to the Guideline on the Diagnosis and Treatment of COVID-19 by the National Health Commission (V.5) as described previously [ Reference Feng 12 ]. Patients were admitted to Shanghai Public Health Clinical Center, Wuhan Jinyintan Hospital and Tongji Hospital of Tongji Medical College HUST in Hubei province and Tongling Municipal People's Hospital in Anhui province from 1 January 2020 to 8 March 2020. All patients recruited in this study were laboratory-confirmed COVID-19. The study was approved by the Ethics Committees of these four hospitals, respectively. Written informed consent was waived owing to the need of rapid emergency response to this infectious disease.
Data collection
Medical records of COVID-19 patients were reviewed by the research team, and demographic, epidemiological, clinical, laboratory, treatment and outcome data were retrieved from electronic medical records using a standardised case report form. All data were cross-checked by two experienced doctors. To ascertain the medical histories or epidemiological data, which were unavailable from electronic medical records, the patients or their close relatives were interviewed by researchers. Data from the medical records were adopted if there was a discrepancy between the subjective description and the medical records.
Laboratory procedures
Method of laboratory confirmation of severe acute respiratory syndrome coronavirus 2 (SARS-CoV-2) has been described elsewhere [ Reference Huang 1 ]. Simply, the Chinese Center for Disease Control and Prevention (CDC) and local CDC were in charge of detecting SARS-CoV-2 in throat-swab specimens from the upper respiratory tract by real-time reverse transcription polymerase chain reaction assay (RT-PCR). The criteria of discharge included absence of fever for at least 3 days, remission of respiratory symptoms, complete improvement in bilateral lungs in chest CT, together with negative for 2 times in throat-swab samples for SARS-CoV-2 RNA at least 24 h apart.
Initial clinical laboratory examinations involved complete blood count, serum biochemical tests (including liver and kidney functions, creatine kinase, lactate dehydrogenase (LDH) and electrolytes), myocardial enzymes, D-dimer and procalcitonin (PCT). Frequency of examinations was under the discretion of treating physicians. Chest computed tomographic (CT) scans were carried out for all COVID-19 patients. Two radiologists were invited to interpret chest CT scans independently and were blinded to the severity of the patient. When disagreement arose, a third radiologist was consulted to reach a final decision.
Statistical analysis
Continuous variables were presented as median with interquartile range (IQR) and the analysis of variance (ANOVA) or Kruskal−Wallis H test were used to compare the difference among three groups as appropriate. Categorical variables were expressed as frequency with percentages, and were analysed by Pearson's χ 2 test or Fisher's exact test. Bonferroni's correction was used for pairwise comparison. All patients were divided into moderate, severe and critical illness groups. Potential predictive variables included the following case characteristics on admission: demographic and epidemiological features, comorbidity, clinical signs and symptoms, laboratory findings and chest imaging results. To explore the risk factors associated with illness severity of COVID-19, namely moderately, severely and critically ill, which means the response variable was ordinally scaled, a cumulative logit model was used to investigate the effect of predictors of COVID-19 severity. Imputation for missing variables of some patients at hospital admission was considered if missing values were less than 20%, and imputation based on the expectation−maximisation algorithm method was used to replace missing values. Before ordinal logistic regression model was fitted, continuous variables of laboratory findings were transformed into categorical variables according to their reference values. The univariate and multivariate cumulative logit models were fitted with moderate illness as the reference level. Potential predictors of severity were investigated using univariate ordinal logistic regression firstly. We further conducted a backward stepwise multivariate ordinal logistic regression analysis excluding variables which were not significant in univariate cumulative logit model. Since missing rate of 34.6% occurred in the lung imaging results and over 40% existed in urine protein and urine glucose, these variables were excluded from multivariate ordinal logistic model. The overall survival (OS) was estimated using the method of Kaplan−Meier and the log-rank test was applied to compare the survival difference among different severity illness groups. The hazard ratio with 95% confidence interval (CI) was estimated with Cox proportional hazard model. A two-sided α of less than 0.05 was considered statistically significant. All statistical analyses were conducted using SAS software (V. 9.4) (SAS Institute Inc., USA).
Demographics, laboratory findings and clinical course
As of 28 April 2020, data from 598 COVID-19 cases admitted to these four hospitals, including 400 (66.89%) moderate cases, 85 (14.21%) severe cases and 113 (18.90%) critical cases, had been collected to be incorporated into this study, of whom 79 cases had died during hospitalisation, with an average mortality of 13.21%, and 457 cases had recovered and been discharged. The remaining 62 cases were still in hospitals. The median age of the 598 patients was 57 years (IQR 42–66), ranging from 11 to 89 years, and 58.03% patients were male ( Table 1 ). At least one comorbidity was present in 51.54% of patients, with hypertension being the most frequent comorbidity (33.90%), followed by diabetes (13.18%) and cardiovascular disease (6.51%). Few cases had a current (7.53%) or former (2.57%) smoking habit. The most common symptoms on admission were fever (81.22%) and dry cough (33.63%), followed by sputum production (30.77%) and shortness of breath (26.65%). Overall, the median interval between illness onset and confirmed diagnosis was 4 days (IQR 2–7), whereas the median interval between illness onset and admission was 7 days (IQR 3–11).
Table 1. Clinical characteristics and comorbidities of 598 COVID-19 patients
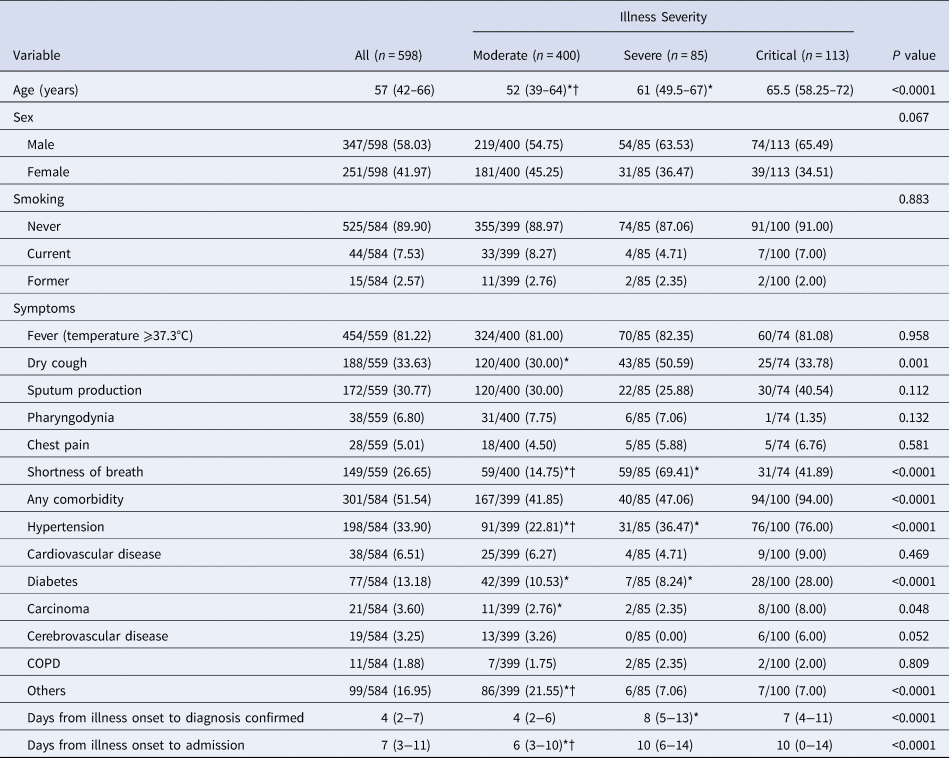
COPD, chronic obstructive pulmonary disease.
Data are median (IQR) or n / total (%). P value denotes the comparison among moderate, severe and critical illness group. * and †Signify P < 0.05 for pos-hoc comparison. *Refers to comparison between the critical group and the severe group or the moderate group. †Refers to comparison between the severe group and the moderate group.
The substantial differences of laboratory findings on admission among three groups of patients were observed ( Table 2 ). The results indicated that significantly higher proportion of patients showing abnormal alanine aminotransferase (ALT) and aspartate aminotransferase (AST), and significantly higher levels of C-reactive protein, neutrophil count, serum potassium, cardiac troponin I (cTnI), myohaemoglobin, PCT, brain natriuretic peptide (BNP) and D-dimer were observed in the critically ill group and the severely ill group than in the moderately ill group, whereas, the levels of haemoglobin and serum albumin were significantly lower in critically ill group and severely ill group as compared with moderately ill group ( P < 0.05). The critical group showed a significantly higher level of platelet count, fibrinogen and serum calcium as compared with severe group and moderate group. The severe group showed a significantly lower level of lymphocyte count than the moderate group. The normal ranges of laboratory indicators are shown in Supplementary Table S1. Abnormalities on chest radiographs on admission were seen in most patients ( Table 2 ). Overall, typical findings on chest CT images were ground-glass opacity (94.37%), followed by pleural thickening (47.06%) and consolidation (35.74%). Chest CT scans showed significantly higher percentage of bilateral lungs involvement in critical group (92.45%) and severe group (95.24%) as compared with moderate group (80.07%). The median lung lobes involved in critical group (5, IQR 5–5) and severe group (5, IQR 2.75–5) were greater than those in moderate group (4, IQR 2–5).
Table 2. Laboratory and chest CT findings on admission of 598 COVID-19 patients
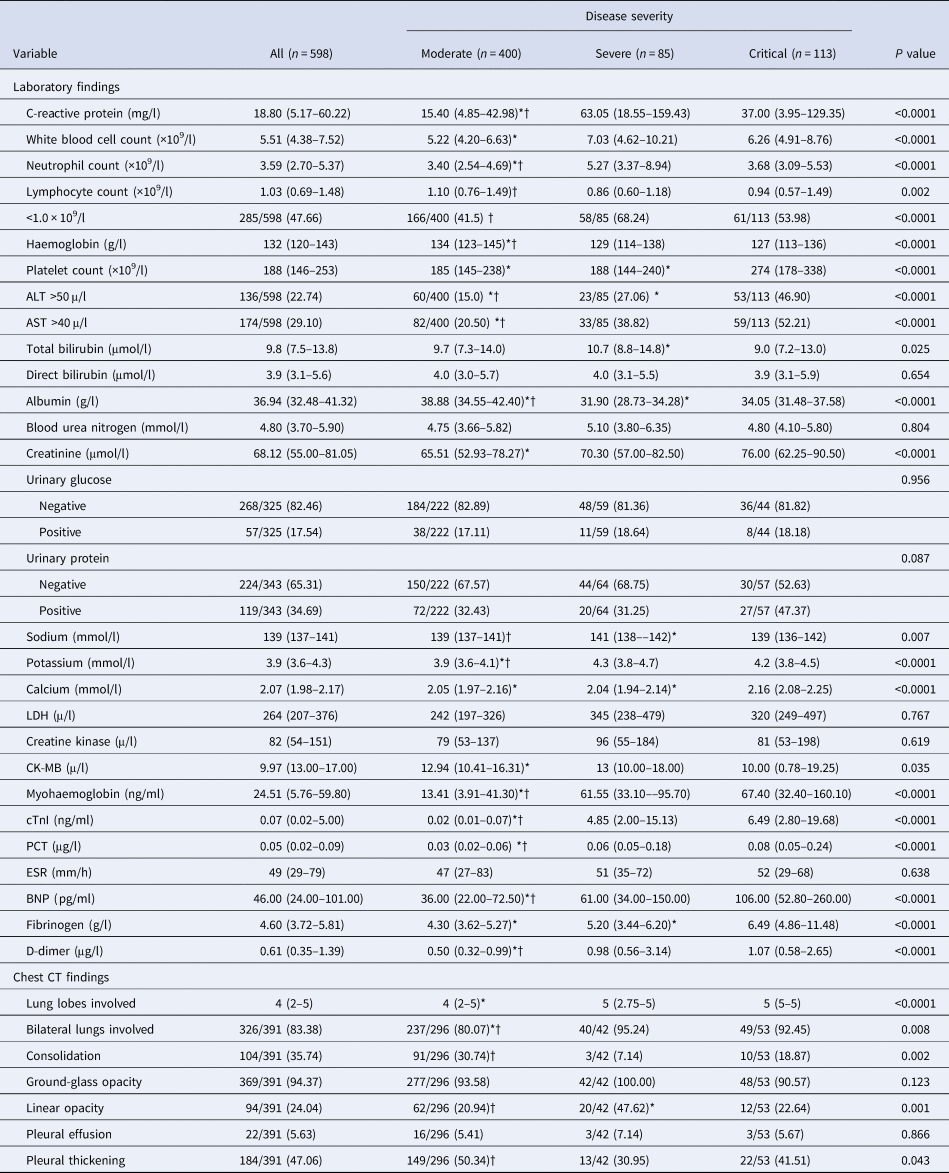
ALT, alanine aminotransferase; AST, aspartate aminotransferase. LDH, lactate dehydrogenase; CK-MB, creatine kinase isoenzymes; cTnI, cardiac troponin I; PCT, procalcitonin; ESR, erythrocyte sedimentation rate; BNP, brain natriuretic pepti.
Data are median (IQR) or n / total (%). P value denotes the comparison among moderate, severe and critical illness group. * and †Signify P < 0.05 for post-hoc comparison. *Refers to comparison between the critical group and the severe group or the moderate group. †Refers to comparison between the severe group and the moderate group.
Totally, 320 (61.07%) patients were given antivirals within 2 days after admission including lopinavir/ritonavir, arbidol, darunavir and chloroquine. In all, 369 (70.83%) patients received antibiotics and 117 (23.08%) received corticosteroids. More patients received corticosteroids in critical group and severe group as compared with the moderate group ( P < 0.05). The proportions of patients accepting high-flow nasal cannula oxygen therapy and non-invasive mechanical ventilation, respectively, in critical and severe groups were significantly higher than in mode rate group ( P < 0.05). Compared with the moderate group, the critical group and the severe group had a significantly lower rate of discharge and a higher mortality rate ( P < 0.05), as shown in Table 3 . The comparison of demographic and baseline characteristics, symptoms, laboratory parameters, lung image features, treatment and prognosis among moderately, severely and critically ill patients were shown in Tables 1 – 3 .
Table 3. Treatment and prognosis of 598 COVID-19 patients
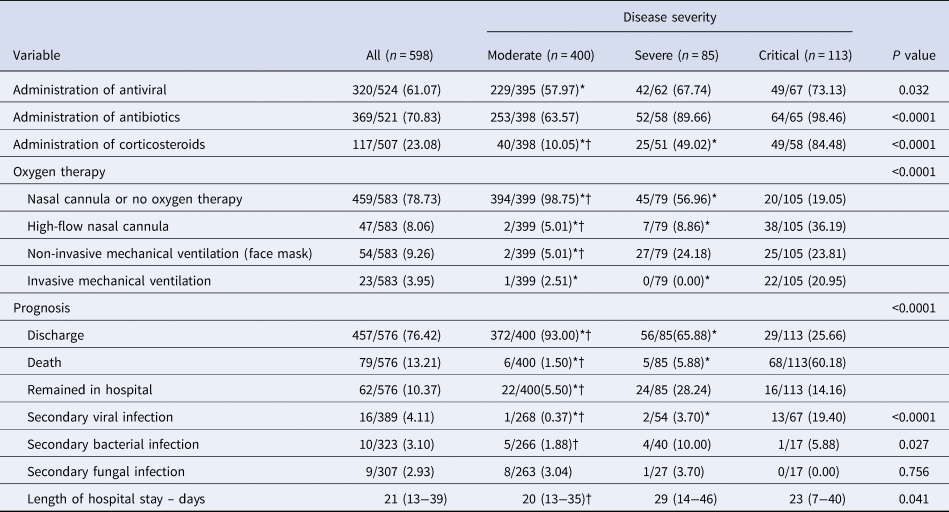
Determinants of illness severity
Fifty-three variables on admission were successively included in the univariate ordinal logistic regression, and 35 variables were found to be associated with illness severity, including age, gender, hypertension, diabetes, interval between illness onset and diagnosis, interval between illness onset and admission, pharyngodynia, shortness of breath, early administration of antiviral, C-reactive protein, white blood cell (WBC) count, neutrophil count, lymphocyte count, haemoglobin, platelet count, ALT, AST, albumin, blood urea nitrogen, creatinine, potassium, LDH, creatine kinase, myohaemoglobin, troponin I (cTnI), PCT, erythrocyte sedimentation rate (ESR), BNP, fibrinogen, D-dimer, bilateral lungs involved, consolidation, linear opacity, pleural thickening and lung lobes involved ( Table 4 ).
Table 4. Results of univariate ordinal logistic model using three levels of severity as response
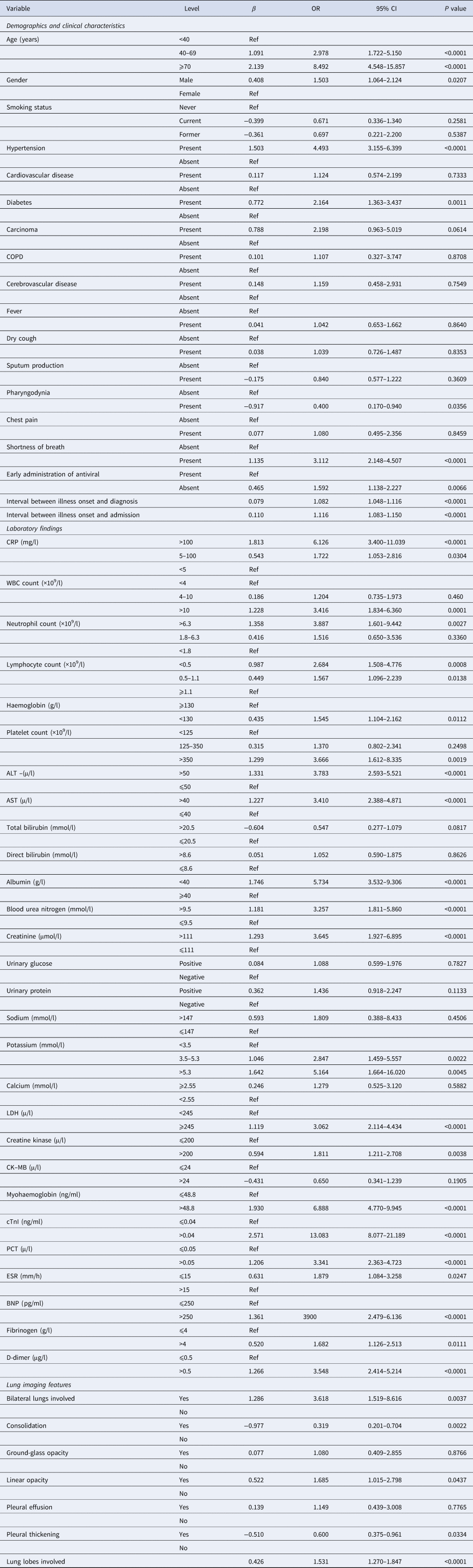
COPD, chronic obstructive pulmonary disease; CRP, C-reactive protein; WBC, white blood cell; ALT, alanine aminotransferase; AST, aspartate aminotransferase; LDH, lactate dehydrogenase; CK-MB, creatine kinase isoenzymes; cTnI, cardiac troponin I; PCT, procalcitonin; ESR, erythrocyte sedimentation rate; BNP, brain natriuretic peptid.
Except five lung image variables, 30 significant predictors of severity in univariable analysis were included in a multivariable stepwise cumulative logit model, and seven variables retained in the final model which were statistically significant independent determinants of COVID-19 illness severity ( Table 5 ). The results of multivariate model revealed that the risks of having more severe illness were 1.586 (95% CI: 0.824–3.053) and 3.419 (95% CI: 1.596–7.323) times higher among patients belonging to the age group 40–69 and 70+ years, respectively, when compared with patients of less than 40 years. Patients with hypertension had 3.372 (95% CI: 2.185–5.202) times greater risk of having worse severity of illness compared with patients without hypertension. The risk of having worse severity of illness was found higher for patients with ALT>50 μ/l (OR = 3.304; 95% CI: 2.107–5.180) when compared with those having ALT⩽50 μ/l. The risk of having worse severity of illness was found significantly higher for patients with higher cTnI (>0.04 ng/ml) than those with normal cTnI (⩽0.04 ng/ml) with OR being 7.464 (95% CI: 4.292–12.980). COVID-19 patients with myohaemoglobin>48.8 ng/ml at admission had a 2.214 (95% CI: 1.42–3.453)-fold greater risk of having worse severity of illness when comparison was made with patients having normal myohaemoglobin level. Table 5 also shows that interval between illness onset and diagnosis and interval between illness onset and admission were independent significant predictors of illness severity with OR being 1.056 (95% CI: 1.012–1.101) and 1.048 (95% CI: 1.009–1.087), respectively.
Table 5. Results of multiple ordinal logistic model using three levels of severity as response
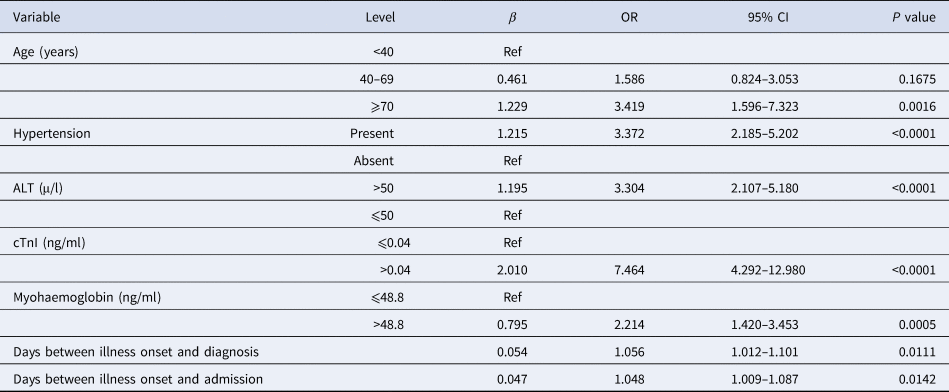
ALT, alanine aminotransferase; cTnI, cardiac troponin I.
Post-hoc comparison of survival among patient groups with different risk factors
In the critical illness group, 68 of 113 patients (60.18%) died, as compared with five of 85 (5.88%) in the severe illness group and six out of 400 (1.50%) in the moderate illness group. The median OS was 29 days in critical group, as compared with not attainable in severe group and moderate group. The 30-day OS rates were 97.7% (95% CI: 95.5–100%), 95.3% (95% CI: 90.2–100%) and 46.4% (95% CI: 37.4–57.6%) in moderate, severe and critical groups, respectively ( P < 0.001), as shown in Figure 1 . Patients of critical illness suffered from inferior survival, as compared with patients in severe group (HR = 14.309, 95% CI: 5.585–36.659) and those in moderate group (HR = 41.021, 95% CI: 17.588–95.678), representing 14.309 times greater risk of death and 41.021 times greater risk of death when compared with severe and moderate groups, respectively. As shown in Supplementary Table S2, COVID-19 patients of older age (HR = 9.823 for ⩾70 vs. <40; HR = 3.361 for 40–69 vs. <40), comorbidity of hypertension (HR = 3.161), abnormal ALT (HR = 1.657), abnormal cTnI (HR = 2.513) or abnormal myohaemoglobin (HR = 2.671) suffered from inferior survival. Also, the Kaplan−Meier survival curves (Supplementary Figs S1–S5) demonstrated that the differences of OS with respect to stratification by these risk factors were all statistically significant ( P < 0.05).
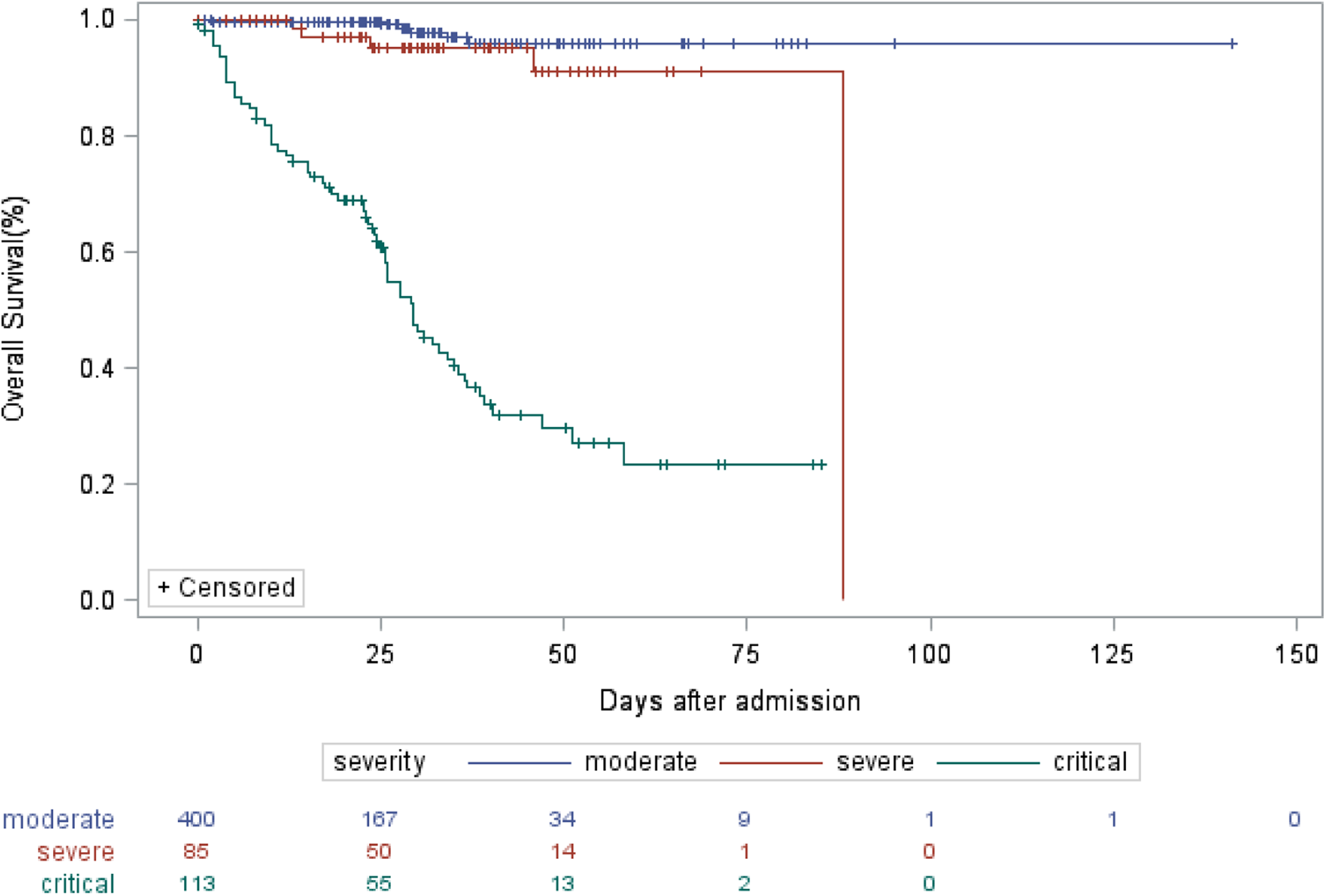
Fig. 1. Kaplan−Meier estimate of OS of COVID-19 patients according to severity of illness.
COVID-19 has presented an unprecedented challenge to the health-care system across the world. With the increasingly scarce health resources, mortality is the most important issue when dealing with epidemics. Early identifying potential of severe and critical patients becomes the priority in minimising the mortality and contributes to allocation of limited critical care. Previously, a risk forecasting model to predict the occurrence of critical illness among hospitalised COVID-19 patients in China has been reported by Liang et al . [ Reference Liang 11 ]. The response variable in Liang's model had just two levels of severe and non-severe. Actually, the mortalities of COVID-19 patients with different severity are variant. This study demonstrated the mortality of 60.18% in critical cases, followed by 5.88% in severe cases and 1.50% in moderate cases. Kaplan−Meier analysis also showed that patients of critical illness suffered from inferior survival, as compared with patients of severe illness and moderate illness, indicating that illness severity is related to the prognosis. In our opinion, more precise classification of illness severity into three levels suits the clinical spectrum of COVID-19 and the predicting model based on multi-level ordinal response variables can be more practical. The current multi-centre retrospective study identified the risk factors of illness severity among COVID-19 inpatients from four hospitals of Hubei, Anhui and Shanghai. According to the Guideline on the Diagnosis and Treatment of COVID-19 by the National Health Commission, inpatients of COVID-19 in this study were divided into moderately ill, severely ill and critically ill groups, and the ordinal logistic regression model was fitted to identify the predicators of severity of illness. Based on current data, older age, comorbidity of hypertension, elevated levels of ALT, elevated cTnI, elevated myohaemoglobin, together with prolonged interval between illness onset and diagnosis and interval between illness onset and admission were independent determinants of severity of COVID-19 and represented higher odds of worse severity of illness.
Evidence is gradually accumulating with regard to the risk factors associated with severity of COVID-19. As described previously, older age has been reported as an important independent predictor of severity in COVID-19 patients [ Reference Wu 5 , Reference Wu 6 , Reference Liang 11 ], which is proved by this study. Comorbidity of hypertension was found to be associated with an increased risk of death. A meta-analysis including 46 248 patients with confirmed COVID-19 indicated that those with the most severe illness were more likely to have hypertension with OR of 2.36 (95% CI: 1.46–3.83). Hypertension was reported to increase the OR for death by 3.05 (95% CI: 1.57–5.92) in patients with COVID-19 [ Reference Zhou 4 ]. Similarly, patients with hypertension had 3.372 (95% CI: 2.185–5.202) times greater risk of developing worse severity of illness in comparison with those without hypertension in our study. The relationship between hypertension and COVID-19 may relate to the role of angiotensin converting enzyme (ACE2) [ Reference Kreutz 13 ]. As a key element in the renin–angiotensin–aldosterone system (RAAS), ACE2 is critically involved in the pathophysiology of hypertension. Studies demonstrated that inhibition of the RAAS with ACE inhibitors (ACEIs) or angiotensin II receptor blockers (ARBs) may result in a compensatory increase in tissue levels of ACE2 and poorer clinical course and prognosis, leading to suggestions that these drugs may be detrimental to COVID-19 patients [ Reference Vaduganathan 14 ].
It has been reported that COVID-19 had significant impact on the liver function [ Reference Zhang 15 , Reference Lei 16 ]. A meta-analysis including 20 retrospective studies with 3428 COVID-19 patients revealed that higher serum levels of AST (mean difference = 8.84 U/l, 95% CI: 5.97–11.71, P < 0.001) and ALT (mean difference = 7.35 U/l, 95% CI: 4.77–9.93, P < 0.001) and lower serum levels of albumin (mean difference = −4.24 g/l, 95% CI: −6.20 to −2.28, P < 0.001) were associated with a significant increase in the severity of COVID-19 [ Reference Parohan 17 ]. Our univariate logistic regression results also showed patients with elevated AST, ALT and decreased albumin had 3.410-, 3.783- and 5.734-fold greater risk of worse severity, which was consistent with this meta-analysis. Particularly, our multivariate ordinal regression demonstrated that ALT is an independent predictor of severity of COVID-19 patients. Since elevated liver injury indicators are strongly associated with the severity risk and subsequent death risk, the liver function should be monitored during hospitalisation. Acute myocardial injury is the most commonly described cardiovascular complication in COVID-19 [ Reference Bansal 18 ]. The overall incidence of acute myocardial injury has been variable but roughly 8–12% of COVID-19 patients are found to develop significant elevation of cTnI [ Reference Lippi 19 ]. The patients admitted to ICU or having severe/fatal illness have several-fold higher likelihood of cTnI elevation. Several recent studies indicated that higher concentration of cTnI and myohaemoglobin were associated with the severity and case fatality rate of COVID-19 [ Reference Chen 20 – Reference Gaze 22 ]. Chen et al . reported that elevated cTnI (OR = 26.909, 95%CI: 4.086–177.226, P = 0.001) were the independent risk factors of critical disease status [ Reference Chen 20 ]. Han et al ., found there were statistically significant differences in the level and positive rate of cTnI and myohaemoglobin among the mild, severe and critical COVID-19 case groups [ Reference Han 23 ]. Our results are in agreement with the previous studies that the elevated myocardial injury markers such as cTnI and myohaemoglobin are independent determinants of illness severity in COVID-19 patients representing negative clinical course and potentially life-threatening prognosis. In particular, cTnI is the strongest predictor of worse severity with OR being 7.464 (95% CI: 4.292–12.980). Direct myocardial injury due to viral myocarditis or the effect of systemic inflammation appears to be the most common mechanisms of acute cardiac injury. Our study also found that interval between illness onset and diagnosis and interval between illness onset and admission were independently associated with illness severity. The risk of developing worse illness severity increased by 1.056-fold and 1.048-fold for each day delay of interval between illness onset and diagnosis and interval between illness onset and admission, respectively. Chen et al . [ Reference Chen 24 ] found the median time from symptom onset to admission was 10 days among deceased COVID-19 patients, one day longer than that of discharged patients. Considering the limited health resources and high case volume in some regions currently, adjusted tactics and strategies should be taken to maximise the availability of and accessibility to medical service to shorten the diagnosis delay or admission delay.
Our study had several limitations. First, this is a retrospective study design, which could be subject to recall bias and selection bias. Second, not all laboratory parameters were tested in all patients, including LDH and D-dimer. Although imputation technique was used to replace missing values, their role might be underestimated in predicting illness severity. Third, due to massive loss of chest CT results, the predicting role of chest CT abnormalities could not be evaluated in this study. Its role of predicting critical illness of COVID-19 has been demonstrated by other studies. Last but not least, generalisability of our findings might be limited by the sample size, and the results need to be validated based on a much larger patient population.
In this study, we identified older age, presence of hypertension, elevated ALT, cTnI and myohaemoglobin, prolonged interval between illness onset and diagnosis and admission as the independent determinants to predict the risk of developing more severe illness among COVID-19 patients. Given the ongoing global pandemic of COVID-19, this study will contribute to early identifications of patients with high risk of developing critical illness and optimising the arrangement of health resources.
Supplementary material
The supplementary material for this article can be found at https://doi.org/10.1017/S0950268820001533 .
Acknowledgements
We acknowledge all health-care workers involved in the diagnosis and treatment of patients in four sites and we thank Dr Qiqi Cao and Dr Weixia Li for assistance in the analysis and interpretation of the lung image data.
Author contributions
Dr Jian Li had full access to all the data in the study and takes responsibility for the integrity of the data and the accuracy of the data analysis. Study design: Jian Li; acquisition of data: Kandi Xu, Dexiang Yang, Yun Ling, Kui Liu, Tao Bai; analysis and interpretation of data: Jian LI, Kandi Xu, Min Zhou, Zenghui Chen; funding acquisition: Min Zhou; drafting of the manuscript: Jian Li; statistical analysis: Jian Li, Kandi Xu; critical revision of the manuscript: all authors.
Financial support
This study was funded by grants from the National R&D Program of China, Shanghai Key Discipline for Respiratory Diseases, Shanghai Shenkang Hospital Development Center Clinical Science and Technology Innovation Project and Zhejiang University Special Scientific Research Fund for COVID-19 Prevention and Control.
Conflict of interests
We declare no competing interests.
Data availability statements
The data that support the findings of this study are available from Clinical Research Center, Ruijin Hospital, Shanghai Jiao Tong University School of Medicine. Restrictions apply to the availability of these data, which were used under license for this study.
Contributed equally.
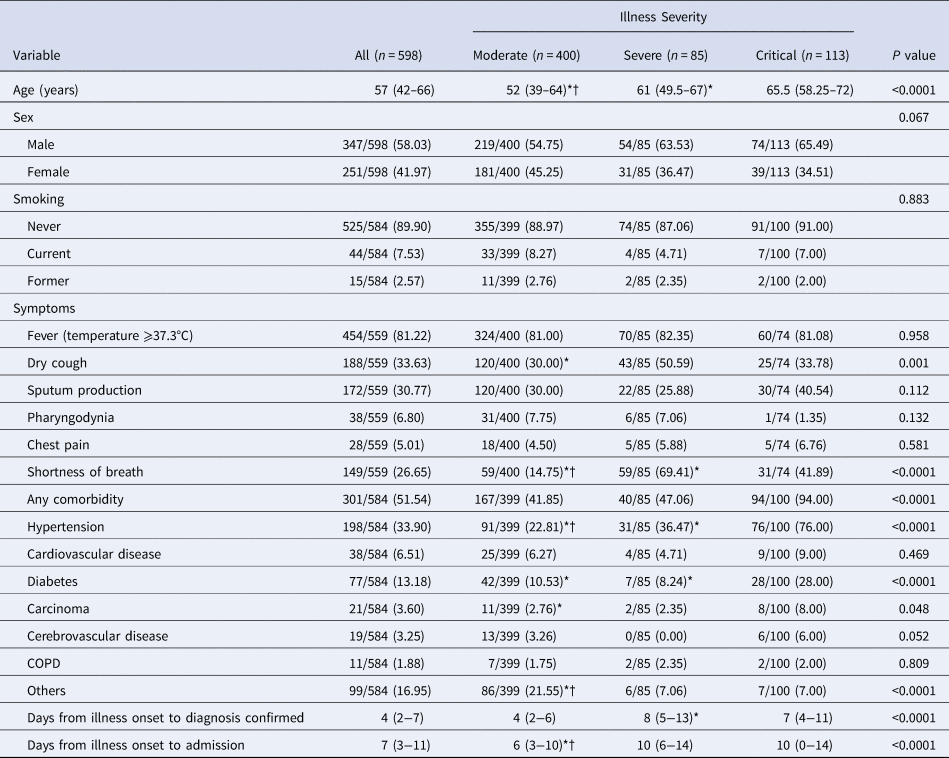
Xu et al. Supplementary Materials
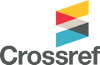
This article has been cited by the following publications. This list is generated based on data provided by Crossref .
- Google Scholar
View all Google Scholar citations for this article.
Save article to Kindle
To save this article to your Kindle, first ensure [email protected] is added to your Approved Personal Document E-mail List under your Personal Document Settings on the Manage Your Content and Devices page of your Amazon account. Then enter the ‘name’ part of your Kindle email address below. Find out more about saving to your Kindle .
Note you can select to save to either the @free.kindle.com or @kindle.com variations. ‘@free.kindle.com’ emails are free but can only be saved to your device when it is connected to wi-fi. ‘@kindle.com’ emails can be delivered even when you are not connected to wi-fi, but note that service fees apply.
Find out more about the Kindle Personal Document Service.
- Kandi Xu (a1) (a2) , Min Zhou (a1) (a2) , Dexiang Yang (a3) , Yun Ling (a4) , Kui Liu (a5) , Tao Bai (a6) , Zenghui Cheng (a7) and Jian Li (a8)
- DOI: https://doi.org/10.1017/S0950268820001533
Save article to Dropbox
To save this article to your Dropbox account, please select one or more formats and confirm that you agree to abide by our usage policies. If this is the first time you used this feature, you will be asked to authorise Cambridge Core to connect with your Dropbox account. Find out more about saving content to Dropbox .
Save article to Google Drive
To save this article to your Google Drive account, please select one or more formats and confirm that you agree to abide by our usage policies. If this is the first time you used this feature, you will be asked to authorise Cambridge Core to connect with your Google Drive account. Find out more about saving content to Google Drive .
Reply to: Submit a response
- No HTML tags allowed - Web page URLs will display as text only - Lines and paragraphs break automatically - Attachments, images or tables are not permitted
Your details
Your email address will be used in order to notify you when your comment has been reviewed by the moderator and in case the author(s) of the article or the moderator need to contact you directly.
You have entered the maximum number of contributors
Conflicting interests.
Please list any fees and grants from, employment by, consultancy for, shared ownership in or any close relationship with, at any time over the preceding 36 months, any organisation whose interests may be affected by the publication of the response. Please also list any non-financial associations or interests (personal, professional, political, institutional, religious or other) that a reasonable reader would want to know about in relation to the submitted work. This pertains to all the authors of the piece, their spouses or partners.
Ordinal logistic regression models: Application in quality of life studies
- February 2008
- Cadernos de saúde pública / Ministério da Saúde, Fundação Oswaldo Cruz, Escola Nacional de Saúde Pública 24 Suppl 4:s581-91
- 24 Suppl 4:s581-91
- CC BY-NC 4.0
- This person is not on ResearchGate, or hasn't claimed this research yet.

- Federal University of São João del-Rei

- Federal University of Minas Gerais
Discover the world's research
- 25+ million members
- 160+ million publication pages
- 2.3+ billion citations
- Jane Acquaye
- Joseph Kwasi Brenyah
- Isaac Asenso Brobbey-Kyei
- Emmanuel Brobbey-Kyei

- Tasya Aguilera
- Yogo Aryo Jatmiko
- BMC MED INFORM DECIS

- Anna Månsdotter
- Chris J. Selman
- Katherine J Lee
- Kristin N. Ferguson

- Solomon Kalayu Mengesha
- Yared Tbebu Gebru

- J CLIN NURS

- W. Teixeira Caiaffa

- J.O.P. Fonseca

- B. Peterson

- W.A. Santos
- M.R. Quaresma
- W. A. Thompson
- P. McCullagh
- J. A. Nelder
- Annette J. Dobson

- Stanley Lemeshow
- Stephen E. Fienberg
- PSYCHOL MED
- THE WHOQOL GROUP
- J. A. Anderson
- Recruit researchers
- Join for free
- Login Email Tip: Most researchers use their institutional email address as their ResearchGate login Password Forgot password? Keep me logged in Log in or Continue with Google Welcome back! Please log in. Email · Hint Tip: Most researchers use their institutional email address as their ResearchGate login Password Forgot password? Keep me logged in Log in or Continue with Google No account? Sign up
- Search Menu
Sign in through your institution
- Browse content in Arts and Humanities
- Browse content in Archaeology
- Anglo-Saxon and Medieval Archaeology
- Archaeological Methodology and Techniques
- Archaeology by Region
- Archaeology of Religion
- Archaeology of Trade and Exchange
- Biblical Archaeology
- Contemporary and Public Archaeology
- Environmental Archaeology
- Historical Archaeology
- History and Theory of Archaeology
- Industrial Archaeology
- Landscape Archaeology
- Mortuary Archaeology
- Prehistoric Archaeology
- Underwater Archaeology
- Urban Archaeology
- Zooarchaeology
- Browse content in Architecture
- Architectural Structure and Design
- History of Architecture
- Residential and Domestic Buildings
- Theory of Architecture
- Browse content in Art
- Art Subjects and Themes
- History of Art
- Industrial and Commercial Art
- Theory of Art
- Biographical Studies
- Byzantine Studies
- Browse content in Classical Studies
- Classical Literature
- Classical Reception
- Classical History
- Classical Philosophy
- Classical Mythology
- Classical Art and Architecture
- Classical Oratory and Rhetoric
- Greek and Roman Papyrology
- Greek and Roman Archaeology
- Greek and Roman Epigraphy
- Greek and Roman Law
- Late Antiquity
- Religion in the Ancient World
- Digital Humanities
- Browse content in History
- Colonialism and Imperialism
- Diplomatic History
- Environmental History
- Genealogy, Heraldry, Names, and Honours
- Genocide and Ethnic Cleansing
- Historical Geography
- History by Period
- History of Emotions
- History of Agriculture
- History of Education
- History of Gender and Sexuality
- Industrial History
- Intellectual History
- International History
- Labour History
- Legal and Constitutional History
- Local and Family History
- Maritime History
- Military History
- National Liberation and Post-Colonialism
- Oral History
- Political History
- Public History
- Regional and National History
- Revolutions and Rebellions
- Slavery and Abolition of Slavery
- Social and Cultural History
- Theory, Methods, and Historiography
- Urban History
- World History
- Browse content in Language Teaching and Learning
- Language Learning (Specific Skills)
- Language Teaching Theory and Methods
- Browse content in Linguistics
- Applied Linguistics
- Cognitive Linguistics
- Computational Linguistics
- Forensic Linguistics
- Grammar, Syntax and Morphology
- Historical and Diachronic Linguistics
- History of English
- Language Evolution
- Language Reference
- Language Variation
- Language Families
- Language Acquisition
- Lexicography
- Linguistic Anthropology
- Linguistic Theories
- Linguistic Typology
- Phonetics and Phonology
- Psycholinguistics
- Sociolinguistics
- Translation and Interpretation
- Writing Systems
- Browse content in Literature
- Bibliography
- Children's Literature Studies
- Literary Studies (Romanticism)
- Literary Studies (American)
- Literary Studies (Modernism)
- Literary Studies (Asian)
- Literary Studies (European)
- Literary Studies (Eco-criticism)
- Literary Studies - World
- Literary Studies (1500 to 1800)
- Literary Studies (19th Century)
- Literary Studies (20th Century onwards)
- Literary Studies (African American Literature)
- Literary Studies (British and Irish)
- Literary Studies (Early and Medieval)
- Literary Studies (Fiction, Novelists, and Prose Writers)
- Literary Studies (Gender Studies)
- Literary Studies (Graphic Novels)
- Literary Studies (History of the Book)
- Literary Studies (Plays and Playwrights)
- Literary Studies (Poetry and Poets)
- Literary Studies (Postcolonial Literature)
- Literary Studies (Queer Studies)
- Literary Studies (Science Fiction)
- Literary Studies (Travel Literature)
- Literary Studies (War Literature)
- Literary Studies (Women's Writing)
- Literary Theory and Cultural Studies
- Mythology and Folklore
- Shakespeare Studies and Criticism
- Browse content in Media Studies
- Browse content in Music
- Applied Music
- Dance and Music
- Ethics in Music
- Ethnomusicology
- Gender and Sexuality in Music
- Medicine and Music
- Music Cultures
- Music and Media
- Music and Culture
- Music and Religion
- Music Education and Pedagogy
- Music Theory and Analysis
- Musical Scores, Lyrics, and Libretti
- Musical Structures, Styles, and Techniques
- Musicology and Music History
- Performance Practice and Studies
- Race and Ethnicity in Music
- Sound Studies
- Browse content in Performing Arts
- Browse content in Philosophy
- Aesthetics and Philosophy of Art
- Epistemology
- Feminist Philosophy
- History of Western Philosophy
- Metaphysics
- Moral Philosophy
- Non-Western Philosophy
- Philosophy of Language
- Philosophy of Mind
- Philosophy of Perception
- Philosophy of Action
- Philosophy of Law
- Philosophy of Religion
- Philosophy of Science
- Philosophy of Mathematics and Logic
- Practical Ethics
- Social and Political Philosophy
- Browse content in Religion
- Biblical Studies
- Christianity
- East Asian Religions
- History of Religion
- Judaism and Jewish Studies
- Qumran Studies
- Religion and Education
- Religion and Health
- Religion and Politics
- Religion and Science
- Religion and Law
- Religion and Art, Literature, and Music
- Religious Studies
- Browse content in Society and Culture
- Cookery, Food, and Drink
- Cultural Studies
- Customs and Traditions
- Ethical Issues and Debates
- Hobbies, Games, Arts and Crafts
- Natural world, Country Life, and Pets
- Popular Beliefs and Controversial Knowledge
- Sports and Outdoor Recreation
- Technology and Society
- Travel and Holiday
- Visual Culture
- Browse content in Law
- Arbitration
- Browse content in Company and Commercial Law
- Commercial Law
- Company Law
- Browse content in Comparative Law
- Systems of Law
- Competition Law
- Browse content in Constitutional and Administrative Law
- Government Powers
- Judicial Review
- Local Government Law
- Military and Defence Law
- Parliamentary and Legislative Practice
- Construction Law
- Contract Law
- Browse content in Criminal Law
- Criminal Procedure
- Criminal Evidence Law
- Sentencing and Punishment
- Employment and Labour Law
- Environment and Energy Law
- Browse content in Financial Law
- Banking Law
- Insolvency Law
- History of Law
- Human Rights and Immigration
- Intellectual Property Law
- Browse content in International Law
- Private International Law and Conflict of Laws
- Public International Law
- IT and Communications Law
- Jurisprudence and Philosophy of Law
- Law and Society
- Law and Politics
- Browse content in Legal System and Practice
- Courts and Procedure
- Legal Skills and Practice
- Primary Sources of Law
- Regulation of Legal Profession
- Medical and Healthcare Law
- Browse content in Policing
- Criminal Investigation and Detection
- Police and Security Services
- Police Procedure and Law
- Police Regional Planning
- Browse content in Property Law
- Personal Property Law
- Study and Revision
- Terrorism and National Security Law
- Browse content in Trusts Law
- Wills and Probate or Succession
- Browse content in Medicine and Health
- Browse content in Allied Health Professions
- Arts Therapies
- Clinical Science
- Dietetics and Nutrition
- Occupational Therapy
- Operating Department Practice
- Physiotherapy
- Radiography
- Speech and Language Therapy
- Browse content in Anaesthetics
- General Anaesthesia
- Neuroanaesthesia
- Clinical Neuroscience
- Browse content in Clinical Medicine
- Acute Medicine
- Cardiovascular Medicine
- Clinical Genetics
- Clinical Pharmacology and Therapeutics
- Dermatology
- Endocrinology and Diabetes
- Gastroenterology
- Genito-urinary Medicine
- Geriatric Medicine
- Infectious Diseases
- Medical Toxicology
- Medical Oncology
- Pain Medicine
- Palliative Medicine
- Rehabilitation Medicine
- Respiratory Medicine and Pulmonology
- Rheumatology
- Sleep Medicine
- Sports and Exercise Medicine
- Community Medical Services
- Critical Care
- Emergency Medicine
- Forensic Medicine
- Haematology
- History of Medicine
- Browse content in Medical Skills
- Clinical Skills
- Communication Skills
- Nursing Skills
- Surgical Skills
- Medical Ethics
- Browse content in Medical Dentistry
- Oral and Maxillofacial Surgery
- Paediatric Dentistry
- Restorative Dentistry and Orthodontics
- Surgical Dentistry
- Medical Statistics and Methodology
- Browse content in Neurology
- Clinical Neurophysiology
- Neuropathology
- Nursing Studies
- Browse content in Obstetrics and Gynaecology
- Gynaecology
- Occupational Medicine
- Ophthalmology
- Otolaryngology (ENT)
- Browse content in Paediatrics
- Neonatology
- Browse content in Pathology
- Chemical Pathology
- Clinical Cytogenetics and Molecular Genetics
- Histopathology
- Medical Microbiology and Virology
- Patient Education and Information
- Browse content in Pharmacology
- Psychopharmacology
- Browse content in Popular Health
- Caring for Others
- Complementary and Alternative Medicine
- Self-help and Personal Development
- Browse content in Preclinical Medicine
- Cell Biology
- Molecular Biology and Genetics
- Reproduction, Growth and Development
- Primary Care
- Professional Development in Medicine
- Browse content in Psychiatry
- Addiction Medicine
- Child and Adolescent Psychiatry
- Forensic Psychiatry
- Learning Disabilities
- Old Age Psychiatry
- Psychotherapy
- Browse content in Public Health and Epidemiology
- Epidemiology
- Public Health
- Browse content in Radiology
- Clinical Radiology
- Interventional Radiology
- Nuclear Medicine
- Radiation Oncology
- Reproductive Medicine
- Browse content in Surgery
- Cardiothoracic Surgery
- Gastro-intestinal and Colorectal Surgery
- General Surgery
- Neurosurgery
- Paediatric Surgery
- Peri-operative Care
- Plastic and Reconstructive Surgery
- Surgical Oncology
- Transplant Surgery
- Trauma and Orthopaedic Surgery
- Vascular Surgery
- Browse content in Science and Mathematics
- Browse content in Biological Sciences
- Aquatic Biology
- Biochemistry
- Bioinformatics and Computational Biology
- Developmental Biology
- Ecology and Conservation
- Evolutionary Biology
- Genetics and Genomics
- Microbiology
- Molecular and Cell Biology
- Natural History
- Plant Sciences and Forestry
- Research Methods in Life Sciences
- Structural Biology
- Systems Biology
- Zoology and Animal Sciences
- Browse content in Chemistry
- Analytical Chemistry
- Computational Chemistry
- Crystallography
- Environmental Chemistry
- Industrial Chemistry
- Inorganic Chemistry
- Materials Chemistry
- Medicinal Chemistry
- Mineralogy and Gems
- Organic Chemistry
- Physical Chemistry
- Polymer Chemistry
- Study and Communication Skills in Chemistry
- Theoretical Chemistry
- Browse content in Computer Science
- Artificial Intelligence
- Computer Architecture and Logic Design
- Game Studies
- Human-Computer Interaction
- Mathematical Theory of Computation
- Programming Languages
- Software Engineering
- Systems Analysis and Design
- Virtual Reality
- Browse content in Computing
- Business Applications
- Computer Games
- Computer Security
- Computer Networking and Communications
- Digital Lifestyle
- Graphical and Digital Media Applications
- Operating Systems
- Browse content in Earth Sciences and Geography
- Atmospheric Sciences
- Environmental Geography
- Geology and the Lithosphere
- Maps and Map-making
- Meteorology and Climatology
- Oceanography and Hydrology
- Palaeontology
- Physical Geography and Topography
- Regional Geography
- Soil Science
- Urban Geography
- Browse content in Engineering and Technology
- Agriculture and Farming
- Biological Engineering
- Civil Engineering, Surveying, and Building
- Electronics and Communications Engineering
- Energy Technology
- Engineering (General)
- Environmental Science, Engineering, and Technology
- History of Engineering and Technology
- Mechanical Engineering and Materials
- Technology of Industrial Chemistry
- Transport Technology and Trades
- Browse content in Environmental Science
- Applied Ecology (Environmental Science)
- Conservation of the Environment (Environmental Science)
- Environmental Sustainability
- Environmentalist Thought and Ideology (Environmental Science)
- Management of Land and Natural Resources (Environmental Science)
- Natural Disasters (Environmental Science)
- Nuclear Issues (Environmental Science)
- Pollution and Threats to the Environment (Environmental Science)
- Social Impact of Environmental Issues (Environmental Science)
- History of Science and Technology
- Browse content in Materials Science
- Ceramics and Glasses
- Composite Materials
- Metals, Alloying, and Corrosion
- Nanotechnology
- Browse content in Mathematics
- Applied Mathematics
- Biomathematics and Statistics
- History of Mathematics
- Mathematical Education
- Mathematical Finance
- Mathematical Analysis
- Numerical and Computational Mathematics
- Probability and Statistics
- Pure Mathematics
- Browse content in Neuroscience
- Cognition and Behavioural Neuroscience
- Development of the Nervous System
- Disorders of the Nervous System
- History of Neuroscience
- Invertebrate Neurobiology
- Molecular and Cellular Systems
- Neuroendocrinology and Autonomic Nervous System
- Neuroscientific Techniques
- Sensory and Motor Systems
- Browse content in Physics
- Astronomy and Astrophysics
- Atomic, Molecular, and Optical Physics
- Biological and Medical Physics
- Classical Mechanics
- Computational Physics
- Condensed Matter Physics
- Electromagnetism, Optics, and Acoustics
- History of Physics
- Mathematical and Statistical Physics
- Measurement Science
- Nuclear Physics
- Particles and Fields
- Plasma Physics
- Quantum Physics
- Relativity and Gravitation
- Semiconductor and Mesoscopic Physics
- Browse content in Psychology
- Affective Sciences
- Clinical Psychology
- Cognitive Psychology
- Cognitive Neuroscience
- Criminal and Forensic Psychology
- Developmental Psychology
- Educational Psychology
- Evolutionary Psychology
- Health Psychology
- History and Systems in Psychology
- Music Psychology
- Neuropsychology
- Organizational Psychology
- Psychological Assessment and Testing
- Psychology of Human-Technology Interaction
- Psychology Professional Development and Training
- Research Methods in Psychology
- Social Psychology
- Browse content in Social Sciences
- Browse content in Anthropology
- Anthropology of Religion
- Human Evolution
- Medical Anthropology
- Physical Anthropology
- Regional Anthropology
- Social and Cultural Anthropology
- Theory and Practice of Anthropology
- Browse content in Business and Management
- Business Ethics
- Business History
- Business Strategy
- Business and Technology
- Business and Government
- Business and the Environment
- Comparative Management
- Corporate Governance
- Corporate Social Responsibility
- Entrepreneurship
- Health Management
- Human Resource Management
- Industrial and Employment Relations
- Industry Studies
- Information and Communication Technologies
- International Business
- Knowledge Management
- Management and Management Techniques
- Operations Management
- Organizational Theory and Behaviour
- Pensions and Pension Management
- Public and Nonprofit Management
- Strategic Management
- Supply Chain Management
- Browse content in Criminology and Criminal Justice
- Criminal Justice
- Criminology
- Forms of Crime
- International and Comparative Criminology
- Youth Violence and Juvenile Justice
- Development Studies
- Browse content in Economics
- Agricultural, Environmental, and Natural Resource Economics
- Asian Economics
- Behavioural Finance
- Behavioural Economics and Neuroeconomics
- Econometrics and Mathematical Economics
- Economic History
- Economic Methodology
- Economic Systems
- Economic Development and Growth
- Financial Markets
- Financial Institutions and Services
- General Economics and Teaching
- Health, Education, and Welfare
- History of Economic Thought
- International Economics
- Labour and Demographic Economics
- Law and Economics
- Macroeconomics and Monetary Economics
- Microeconomics
- Public Economics
- Urban, Rural, and Regional Economics
- Welfare Economics
- Browse content in Education
- Adult Education and Continuous Learning
- Care and Counselling of Students
- Early Childhood and Elementary Education
- Educational Equipment and Technology
- Educational Strategies and Policy
- Higher and Further Education
- Organization and Management of Education
- Philosophy and Theory of Education
- Schools Studies
- Secondary Education
- Teaching of a Specific Subject
- Teaching of Specific Groups and Special Educational Needs
- Teaching Skills and Techniques
- Browse content in Environment
- Applied Ecology (Social Science)
- Climate Change
- Conservation of the Environment (Social Science)
- Environmentalist Thought and Ideology (Social Science)
- Natural Disasters (Environment)
- Social Impact of Environmental Issues (Social Science)
- Browse content in Human Geography
- Cultural Geography
- Economic Geography
- Political Geography
- Browse content in Interdisciplinary Studies
- Communication Studies
- Museums, Libraries, and Information Sciences
- Browse content in Politics
- African Politics
- Asian Politics
- Chinese Politics
- Comparative Politics
- Conflict Politics
- Elections and Electoral Studies
- Environmental Politics
- Ethnic Politics
- European Union
- Foreign Policy
- Gender and Politics
- Human Rights and Politics
- Indian Politics
- International Relations
- International Organization (Politics)
- International Political Economy
- Irish Politics
- Latin American Politics
- Middle Eastern Politics
- Political Behaviour
- Political Economy
- Political Institutions
- Political Theory
- Political Methodology
- Political Communication
- Political Philosophy
- Political Sociology
- Politics and Law
- Politics of Development
- Public Policy
- Public Administration
- Quantitative Political Methodology
- Regional Political Studies
- Russian Politics
- Security Studies
- State and Local Government
- UK Politics
- US Politics
- Browse content in Regional and Area Studies
- African Studies
- Asian Studies
- East Asian Studies
- Japanese Studies
- Latin American Studies
- Middle Eastern Studies
- Native American Studies
- Scottish Studies
- Browse content in Research and Information
- Research Methods
- Browse content in Social Work
- Addictions and Substance Misuse
- Adoption and Fostering
- Care of the Elderly
- Child and Adolescent Social Work
- Couple and Family Social Work
- Direct Practice and Clinical Social Work
- Emergency Services
- Human Behaviour and the Social Environment
- International and Global Issues in Social Work
- Mental and Behavioural Health
- Social Justice and Human Rights
- Social Policy and Advocacy
- Social Work and Crime and Justice
- Social Work Macro Practice
- Social Work Practice Settings
- Social Work Research and Evidence-based Practice
- Welfare and Benefit Systems
- Browse content in Sociology
- Childhood Studies
- Community Development
- Comparative and Historical Sociology
- Economic Sociology
- Gender and Sexuality
- Gerontology and Ageing
- Health, Illness, and Medicine
- Marriage and the Family
- Migration Studies
- Occupations, Professions, and Work
- Organizations
- Population and Demography
- Race and Ethnicity
- Social Theory
- Social Movements and Social Change
- Social Research and Statistics
- Social Stratification, Inequality, and Mobility
- Sociology of Religion
- Sociology of Education
- Sport and Leisure
- Urban and Rural Studies
- Browse content in Warfare and Defence
- Defence Strategy, Planning, and Research
- Land Forces and Warfare
- Military Administration
- Military Life and Institutions
- Naval Forces and Warfare
- Other Warfare and Defence Issues
- Peace Studies and Conflict Resolution
- Weapons and Equipment

- < Previous chapter
- Next chapter >

4 Regression with an Ordinal Dependent Variable
- Published: May 2009
- Cite Icon Cite
- Permissions Icon Permissions
This chapter discusses ordinal logistic regression (also known as the ordinal logit, ordered polytomous logit, constrained cumulative logit, proportional odds, parallel regression, or grouped continuous model), for modeling relationships between an ordinal dependent variable and multiple independent variables. Ordinal variables have three or more ordered categories, and ordinal logistic regression focuses on cumulative probabilities of the dependent variable and odds and odds ratios based on those cumulative probabilities, estimating a single common odds ratio. The chapter discusses the proportional odds or parallel regression assumption; this is the assumption that the odds ratios for each cumulative level are equal in the population (although they might be different in a sample due to sampling error). The concepts of threshold , sometimes called a cut - point , proportional odds or parallel regression assumption , are also discussed.
Personal account
- Sign in with email/username & password
- Get email alerts
- Save searches
- Purchase content
- Activate your purchase/trial code
- Add your ORCID iD
Institutional access
Sign in with a library card.
- Sign in with username/password
- Recommend to your librarian
- Institutional account management
- Get help with access
Access to content on Oxford Academic is often provided through institutional subscriptions and purchases. If you are a member of an institution with an active account, you may be able to access content in one of the following ways:
IP based access
Typically, access is provided across an institutional network to a range of IP addresses. This authentication occurs automatically, and it is not possible to sign out of an IP authenticated account.
Choose this option to get remote access when outside your institution. Shibboleth/Open Athens technology is used to provide single sign-on between your institution’s website and Oxford Academic.
- Click Sign in through your institution.
- Select your institution from the list provided, which will take you to your institution's website to sign in.
- When on the institution site, please use the credentials provided by your institution. Do not use an Oxford Academic personal account.
- Following successful sign in, you will be returned to Oxford Academic.
If your institution is not listed or you cannot sign in to your institution’s website, please contact your librarian or administrator.
Enter your library card number to sign in. If you cannot sign in, please contact your librarian.
Society Members
Society member access to a journal is achieved in one of the following ways:
Sign in through society site
Many societies offer single sign-on between the society website and Oxford Academic. If you see ‘Sign in through society site’ in the sign in pane within a journal:
- Click Sign in through society site.
- When on the society site, please use the credentials provided by that society. Do not use an Oxford Academic personal account.
If you do not have a society account or have forgotten your username or password, please contact your society.
Sign in using a personal account
Some societies use Oxford Academic personal accounts to provide access to their members. See below.
A personal account can be used to get email alerts, save searches, purchase content, and activate subscriptions.
Some societies use Oxford Academic personal accounts to provide access to their members.
Viewing your signed in accounts
Click the account icon in the top right to:
- View your signed in personal account and access account management features.
- View the institutional accounts that are providing access.
Signed in but can't access content
Oxford Academic is home to a wide variety of products. The institutional subscription may not cover the content that you are trying to access. If you believe you should have access to that content, please contact your librarian.
For librarians and administrators, your personal account also provides access to institutional account management. Here you will find options to view and activate subscriptions, manage institutional settings and access options, access usage statistics, and more.
Our books are available by subscription or purchase to libraries and institutions.
Month: | Total Views: |
---|---|
October 2022 | 16 |
November 2022 | 3 |
December 2022 | 5 |
February 2023 | 7 |
March 2023 | 17 |
April 2023 | 2 |
May 2023 | 7 |
June 2023 | 4 |
July 2023 | 8 |
August 2023 | 2 |
September 2023 | 8 |
October 2023 | 6 |
November 2023 | 20 |
December 2023 | 17 |
January 2024 | 9 |
February 2024 | 18 |
March 2024 | 22 |
April 2024 | 24 |
May 2024 | 18 |
June 2024 | 5 |
- About Oxford Academic
- Publish journals with us
- University press partners
- What we publish
- New features
- Open access
- Rights and permissions
- Accessibility
- Advertising
- Media enquiries
- Oxford University Press
- Oxford Languages
- University of Oxford
Oxford University Press is a department of the University of Oxford. It furthers the University's objective of excellence in research, scholarship, and education by publishing worldwide
- Copyright © 2024 Oxford University Press
- Cookie settings
- Cookie policy
- Privacy policy
- Legal notice
This Feature Is Available To Subscribers Only
Sign In or Create an Account
This PDF is available to Subscribers Only
For full access to this pdf, sign in to an existing account, or purchase an annual subscription.
Ordinal Logistic Regression
Cite this chapter.
Part of the book series: Statistics for Biology and Health ((SBH))
1467 Accesses
1 Citations
This is a preview of subscription content, log in via an institution to check access.
Access this chapter
- Available as PDF
- Read on any device
- Instant download
- Own it forever
Tax calculation will be finalised at checkout
Purchases are for personal use only
Institutional subscriptions
Unable to display preview. Download preview PDF.
Rights and permissions
Reprints and permissions
Copyright information
© 2002 Springer-Verlag New York, Inc.
About this chapter
(2002). Ordinal Logistic Regression. In: Logistic Regression. Statistics for Biology and Health. Springer, New York, NY. https://doi.org/10.1007/0-387-21647-2_10
Download citation
DOI : https://doi.org/10.1007/0-387-21647-2_10
Publisher Name : Springer, New York, NY
Print ISBN : 978-0-387-95397-7
Online ISBN : 978-0-387-21647-8
eBook Packages : Springer Book Archive
Share this chapter
Anyone you share the following link with will be able to read this content:
Sorry, a shareable link is not currently available for this article.
Provided by the Springer Nature SharedIt content-sharing initiative
- Publish with us
Policies and ethics
- Find a journal
- Track your research
- DOI: 10.1590/S0102-311X2008001600010
- Corpus ID: 18342220
Ordinal logistic regression models: application in quality of life studies.
- M. Abreu , A. L. Siqueira , +1 author W. Caiaffa
- Published in Cadernos de Saúde Pública 2008
Ask This Paper
By using this feature, you agree to AI2's terms and conditions and that you will not submit any sensitive or confidential info.
AI2 may include your prompts and inputs in a public dataset for future AI research and development. Please check the box to opt-out.
Ask a question about " "
Supporting statements, tables from this paper.
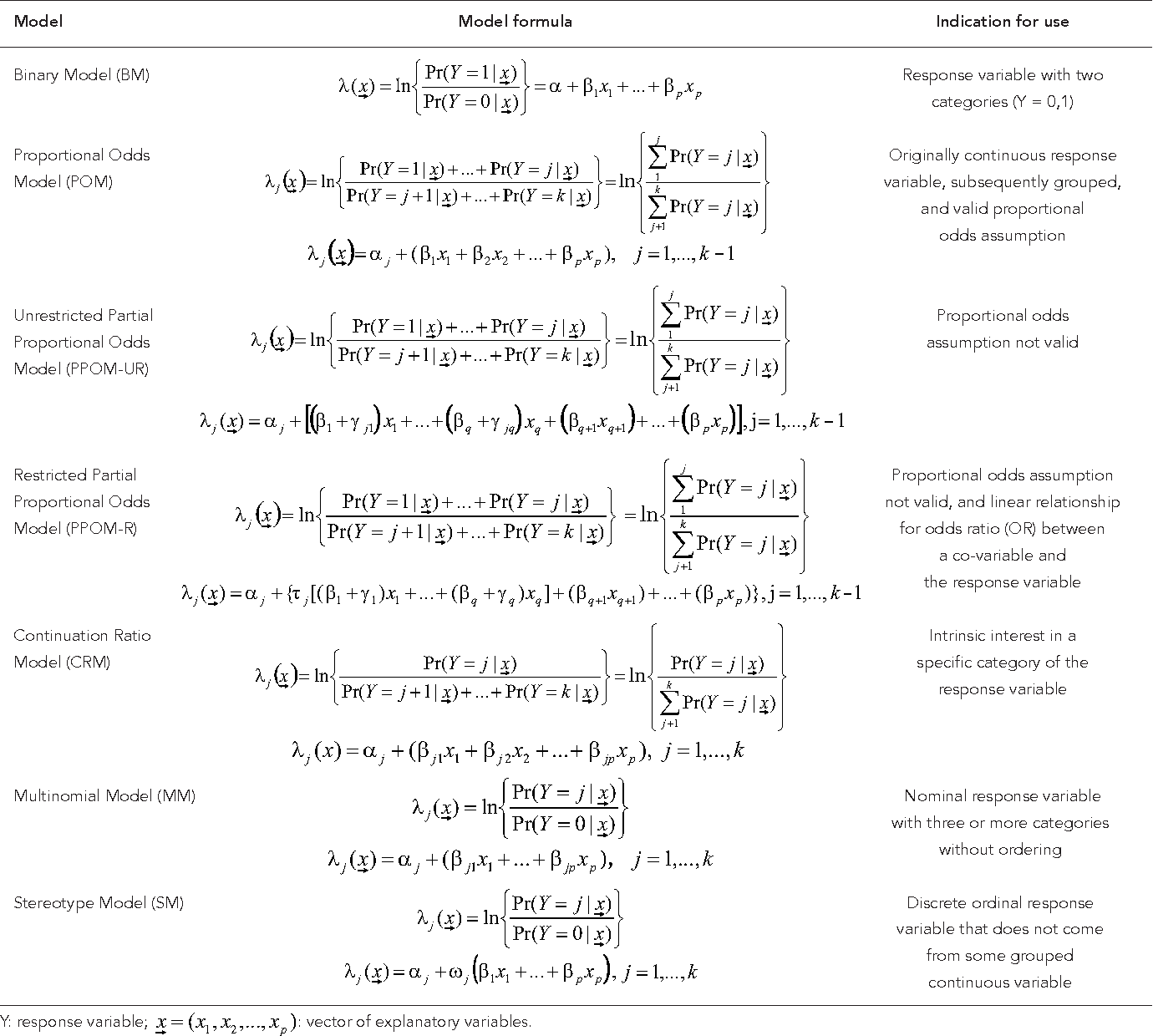
82 Citations
Modeling ordinal longitudinal outcomes : an applied perspective of marginal and conditional approaches, how are bmi, nutrition, and physical exercise related an application of ordinal logistic regression, a method for ordinal outcomes: the ordered stereotype model, ordered logistic regression on the mental health of undergraduate students, ordinary least squares regression of ordered categorical data: inferential implications for practice.
- Highly Influenced
Statistical analyses of ordinal outcomes in randomised controlled trials: a scoping review
A utility‐based design for randomized comparative trials with ordinal outcomes and prognostic subgroups, proportional odds model for health states analysis, application of ordinal logistic regression analysis in determining risk factors of child malnutrition in bangladesh, investigating the power of goodness-of-fit tests for multinomial logistic regression, 25 references, a review of ordinal regression models applied on health-related quality of life assessments, statistical assessment of ordinal outcomes in comparative studies., regression models for ordinal responses: a review of methods and applications., design and analysis of trials with quality of life as an outcome: a practical guide, applied logistic regression: hosmer/applied logistic regression, assessing proportionality in the proportional odds model for ordinal logistic regression., factors associated with low quality of life in schizophrenia., partial proportional odds models for ordinal response variables, ordinal logistic regression in medical research, regression models for ordinal data, related papers.
Showing 1 through 3 of 0 Related Papers
- Technical Support
- Find My Rep
You are here
Logistic Regression Models for Ordinal Response Variables
- Ann A. O'Connell - Ohio State University, USA
- Description
ISBN: 9780761929895 | Paperback | Suggested Retail Price: $42.00 | Bookstore Price: $33.60 |
ISBN: 9781452210834 | Electronic Version | Suggested Retail Price: $36.00 | Bookstore Price: $28.80 |
See what’s new to this edition by selecting the Features tab on this page. Should you need additional information or have questions regarding the HEOA information provided for this title, including what is new to this edition, please email [email protected] . Please include your name, contact information, and the name of the title for which you would like more information. For information on the HEOA, please go to http://ed.gov/policy/highered/leg/hea08/index.html .
For assistance with your order: Please email us at [email protected] or connect with your SAGE representative.
SAGE 2455 Teller Road Thousand Oaks, CA 91320 www.sagepub.com
- Explores model fit statistics and provides information on how to run these models within the major statistics packages
- Provides comparative interpretations among the models using current data from the Early Childhood Longitudinal Study (ECLS) to provide worked out examples of the concepts
- Gives an example of the cumulative odds model within a multilevel context (children within schools)
- Provides worked out examples from public health, education, management, and psychology
Sage College Publishing
You can purchase this book and request an instructor sample on our US College site:
Related Products

This title is also available on SAGE Research Methods , the ultimate digital methods library. If your library doesn’t have access, ask your librarian to start a trial .
- Research article
- Open access
- Published: 26 April 2020
Ordinal logistic regression model describing factors associated with extent of nodal involvement in oral cancer patients and its prospective validation
- Vishwajeet Singh 1 ,
- Sada Nand Dwivedi ORCID: orcid.org/0000-0003-4262-6143 1 &
- S. V. S. Deo 2
BMC Medical Research Methodology volume 20 , Article number: 95 ( 2020 ) Cite this article
15k Accesses
11 Citations
Metrics details
Oral cancer is the most common cancer among Indian men, and has strong tendency of metastatic spread to neck lymph node which strongly influences prognosis especially 5 year survival-rate and also guides the related managements more effectively. Therefore, a reliable and accurate means of preoperative evaluation of extent of nodal involvement becomes crucial. However, earlier researchers have preferred to address mainly its dichotomous form (involved/not-involved) instead of ordinal form while dealing with epidemiology of nodal involvement. As a matter of fact, consideration of ordinal form appropriately may increase not only the efficiency of the developed model but also accuracy in the results and related implications. Hence, to develop a model describing factors associated with ordinal form of nodal involvement was major focus of this study.
The data for model building were taken from the Department of Surgical Oncology, Dr.BRA-IRCH, AIIMS, New Delhi, India. All the OSCC patients (duly operated including neck dissection) and confirmed histopathologically from 1995 to 2013 were included. Further, another data of 204 patients collected prospectively from 2014 to 2015 was considered for the validation of the developed model. To assess the factors associated with extent of nodal involvement, as a first attempt in the field of OSCC, stepwise multivariable regression procedure was used and results are presented as odds-ratio and corresponding 95% confidence interval (CI). For appropriate accounting of ordinal form, the ordinal models were assessed and compared. Also, performance of the developed model was validated on a prospectively collected another data.
Under multivariable proportional odds model, pain at the time of presentation, sub mucous fibrosis, palpable neck node, oral site and degree of differentiation were found to be significantly associated factors with extent of nodal involvement. In addition, tumor size also emerged to be significant under partial-proportional odds model.
Conclusions
The analytical results under the present study reveal that in case of ordinal form of the outcome, appropriate ordinal regression may be a preferred choice. Present data suggest that, pain, sub mucous fibrosis, palpable neck node, oral site, degree of differentiation and tumor size are the most probable associated factors with extent of nodal involvement.
Peer Review reports
In India, in terms of prevalence and incidence, oral cancer ranks first among men and overall at rank three, however regarding related mortality it is at third position in men (GLOBOCAN 2012) [ 1 ]. The prognosis of oral cancer including 5 year survival rate may get highly affected due to its metastatic spread to neck lymph node [ 2 ]. As a result, the regional control and overall survival may also get affected [ 3 , 4 , 5 , 6 , 7 ]. In other words, the outcome of oral cancer may significantly change due to the nodal involvement. Hence, appropriate management of the cervical lymph nodes is an important part of oral cancer therapy [ 8 , 9 , 10 , 11 , 12 , 13 ]. However, there has been controversy over the indication, timing and methods of neck dissection [ 12 , 13 ]. Difficulties in early diagnosing thus restrict the treatment carried out by surgeons [ 14 ]. A reliable and accurate means of preoperative evaluation of cervical lymph node metastasis is therefore crucial for the correct management of oral cancer [ 8 , 10 , 13 , 15 , 16 ]. Also, understanding of its associated factors may provide clues to the clinicians for better management.
There are negligible numbers of studies dealing with analysis of nodal involvement among oral cancer patients. Most of them have focused either on occult nodal metastasis only or a specific oral site i.e., tongue, lip or buccal mucosa or stages [ 2 , 17 , 18 , 19 , 20 , 21 , 22 , 23 , 24 , 25 , 26 , 27 ]. Further, they have dealt with only presence/absence of nodal involvement, not in the ordinal form. It is well known that, ignoring the ordering has its own disadvantage mainly because it does not fully utilize the available information [ 28 ]. For example, Armstrong and Sloan [ 29 ] have reported that compared to a cumulative logit model for a five level ordered response, use of logistic model resulted into a loss of 25–50% of efficiency. To the best of our knowledge, this is the first such study dealing with the ordinal form of extent of involved nodes among oral cancer patients.
Keeping in view of the above points, objective of this study was to develop an ordinal logistic regression model to find out the factors associated with ordinal form of involved nodes and validate it on temporal data.
The utilized dataset under the present study is same as that considered while assessing the factors associated with nodal involvement (yes/no) among oral squamous cell carcinoma patients [ 30 , 31 ]. As such, the data maintained at Department of Surgical Oncology, Institute Rotary Cancer Hospital (IRCH), AIIMS, New Delhi, India for patients with histopathologically proven oral squamous cell carcinoma who went under surgery including neck dissection was considered. Out of data of 1123 oral cancer patients available during 1995 to 2013, 945 fulfilled the inclusion criteria. Further, prospectively collected data of 204 patients from January 2014 to December 2015 was used for the temporal validation of the developed ordinal model. The number of involved nodes of each patient was collected from their histopathological reports. In view to utilize maximum available information for more accurate results, ordinal categories mainly relied on desired varying management strategies being adopted by the oncologists according to cut-off values of involved nodes. Accordingly it was considered as 0, 1, 2–4 and > 4 involved nodes in ordinal categories. The covariates in this study remain same as those under dichotomous model [ 30 , 31 ]. Statistical software, STATA/SE version 14.2 (StataCorp LP, College Station, TX, USA), was used for the analysis.
Statistical analysis
Categorical variables were described using absolute/relative frequency distribution and quantitative variables using measures of central tendency/location like mean (standard deviation)/median (quartile range). The association between qualitative independent variables, was assessed using Chi-square test/ Fisher’s exact test. To assess association between two quantitative variables, Pearson/Spearman’s correlation coefficient was used. Collinearity between the covariates was assessed by Cramer’s V as 0.7 and more. To find out the factors associated with ordinal form of nodal involvement, stepwise ordinal logistic regression procedure was used. Variables which were found to be significant at the level of 25% under crude association analysis (univariable analysis) and/or on the basis of their clinical relevance were taken as a sub-set of covariates for stepwise regression. Results are presented in the form of odds ratio and corresponding 95% confidence interval (CI). Brant test was used for proportionality assumption [ 32 ] . The model performance was assessed using measure of discrimination and calibration (i.e. the accuracy of the prediction probability of nodal involvement). Discrimination performance was evaluated using Average Dichotomous C-index, Generalized C-index and Set wise C-index (ORC) [ 33 ]. Calibration of the predicted probabilities under the developed model was investigated using M.W. Fagerland and D.W. Hosmer test for goodness of fit [ 34 ] and specification error by linktest [ 35 ]. The equal spaced integer weight score was used to discriminate the individuals regarding calibration and discrimination ability of the developed model [ 36 ].
Statistical models
There are different ordinal logistic regression models to tack care of ordinal form of outcomes. These different ordinal regression models have different ways to form their logits. For example, proportional odds model (considered as cumulative higher category(s) verses cumulative remaining lower category(s)); continuation ratio model (considered as cumulative higher category(s) verses just lower category alone); and adjacent category model (between any of two consecutive categories). Accordingly every form of the logit has its own benefits or limitation, one can use the models as per specific need. To be more specific, continuation ratio model and adjacent category model do not relay on complete data set. In epidemiological and biomedical applications, proportional odd model (POM) is often used. However, sometime continuation ratio model is also used [ 37 , 38 ]. Further, choice between the POM and CRM models relies on the goals of the statistical analysis. As obvious, in case of nodal involvement, interpretation under POM will remain more logical and meaningful. However, if the proportionality assumption does not fulfill, partial proportional odds model may be a better choice [ 35 , 39 ]. Further, choice between proportional odds and partial proportional odds models was assessed using likelihood ratio test, LR, AIC and BIC.
Proportional odds model (POM)
If log odds ratio across the cut points is identical, i.e. proportional odds assumption is satisfied, the proportional odds model is used. The proportional odds model was initially proposed by Walker and Duncan [ 40 ] as cumulative logit model, but later it was named as proportional odds model by McCullagh [ 37 ]. In case of the present study, independent observations on 945 patients were available. As described earlier, observation on the nodal involvement (Y) for each patient is classified into either of four categories. Likewise, covariates ( x i ) denote the p-dimensional vector of covariates (i = 1,2….p), containing the observation on the full set of p explanatory variables. Accordingly, the dependency of Y on x i may be expressed as:
Where, Pr ( Y ≥ y j ) is the cumulative probability of the event ( Y ≥ y j ); α j are the respective intercept parameters; β is a (p by 1) vector of regression coefficients corresponding to x i covariates.
Partial proportional odds model (PPOM)
If identical log odds ratio assumption under POM is not fulfilled for some of the covariates, partial proportional odds model may be used [ 41 ]. Out of the two approaches available in this regard, unconstrained partial proportional odds model and constrained partial proportional odds model, unconstrained PPOM model was used due to unavailability of prior knowledge or beliefs regarding constraints and also availability of computational facility [ 42 ]. The PPOM permits non proportional odds for a subset of q of the p-predictors (q ≤ p). The unconstrained PPOM cumulative probability may be defined as:
Where x i is a (p by 1) vector containing the values of observation i on the full set of p explanatory variables, β is a (p by 1) vector of regression coefficients associated with p variables. Further, t ′ is a (1 by q) vector of q-covariates, containing the values of observation i on that subset of the p explanatory variables for which proportionality assumption is either not fulfilled or is to be tested; and γ j is a (q by 1) vector of regression coefficients associated with the q covariates. Accordingly, t ′ γ j is the increment associated with jth cumulative logit (1 < = j < =3),where γ 1 is equal to 0. If γ j = 0 for all j, then this model reduces to proportional odds model. Accordingly, the proportional odds assumption for the q variables in t is a test of the null hypothesis that γ j = 0 for all j = 2, 3.
The ordinal logistic regression model was developed using data on 945 patients, where as the data of another 204 patients was used for temporal validation of the developed model. Out of 945 patients, females were 212 (22.4%). Majority of the patients were in the age group 40 to 60 years 549 (58.1%) and in lower/lower middle socio-economic class 751 (79.5%). The distribution of number of involved nodes were as; no node involved: 569 (60.21%); 1 node involved: 149 (15.77%); 2–4 nodes involved: 162 (17.14%) and > 4 nodes involved: 65 (6.88%).
Out of 39 available variables, a set of 19 covariates were considered for stepwise regression procedure, among which 9 variables were selected on the basis of its crude association at 25% level of significance and 10 variables on its clinical relevance. Collinearity and first order effect modifier were assessed before developing the multivariable model. They were absent in the present dataset. The distribution of nodal involvement and its associations with covariates are presented in Table 1 .
Under multivariable regression analysis, proportional odds assumption was found to be satisfactory for each of the considered covariates selected for developing final model except histopathological tumor size. Moreover, overall proportionality assumption was not violated ( p = 0.97). Accordingly, both the models (POM and PPOM) were developed and compared.
Interestingly, the results under proportional odds multivariable regression analysis emerged to be similar to those reported under binary form of nodal involvement [ 30 ]. To be more specific, pain at presentation, SMF, palpable neck node, oral site and degree of differentiation were retained to be significantly associated factors also with higher extent of nodal involvement. The patients presenting with pain were 37% more likely to have higher order of nodal positivity as compared to lower [1.37 (1.05 to 1.78)], whereas SMF was protective and patients with SMF had 57% less chance for higher frequency of node involvement [0.43 (0.21 to 0.90)]. Patients other than well differentiated tumors were more likely for presence of positive node [1.41 (1.07 to 1.86)] (Table 2 ).
Like proportional odds model, under multivariable partial proportional odds model, pain at the time of presentation, SMF, palpable neck node, oral site and degree of differentiation were found to be significantly associated factors. In addition, tumor size also emerged to be significant. For patients with large tumor size, the chance of involving higher number of positive nodes went up. The patients with tumor size more than 4 cm had more than two fold chance to be involved with more than 4 numbers of positive nodes. The detailed results under multivariable regression analysis are presented in (Table 3 ).
The comparative appraisal of both the models (Table 2 , Table 3 ) revealed that partial proportional odds model stands to be preferred as indicated by LR and AIC (Table 4 ). Likelihood-ratio test also suggests the same ( p = 0.004).
Assessment of the model
Fagerland and Hosmer test [ 34 ] for goodness of fit suggests ( p = 0.88) that our developed model describes the distribution of nodal involvement satisfactorily. Further, link test suggest ( p = 0.65) that there was no specification error in the developed partial proportional odds model. For discrimination ability of the developed model, Average Dichotomous C-index, Generalized C-index and Set wise C-index (ORC) were used [ 33 ]. The Average Dichotomous C-index values of the developed model was found to be 0.67, which suggests that probability to correctly discriminate a case with a lower outcome level from a case with higher outcome level was 0.67. However, Generalized C-index value was observed as 0.635, which means that probability to discriminate two cases from different categories was 0.635. Further, set wise C-index (ORC) value of the model was 0.634. It implies that the probability of correct discrimination in a pair of cases from two randomly chosen categories was 0.634.

Validation of the developed model
As described earlier, temporal validation [ 43 ] of the developed model was evaluated on another dataset of 204 patients (validation data), collected prospectively from the same centre. The goodness of fit test on validation data also suggests ( p = 0.35) that the developed model sustains its ability to describe the distribution of nodal involvement satisfactorily. In this case, the probability values of all the three indices (i.e. Average Dichotomous C-index, Generalized C-index and Set wise C-index (ORC)) providing discrimination ability of the ordinal logistic regression were 0.66, 0.63 and 0.64 respectively, which again indicates that the developed model performs equally on validation data. The analytical results amply reveal that the developed model remains to be generalizable and acceptable.
In biomedical research, in addition to frequent emergence of ordinal categorical data, sometime ordinal categories are the result of grouping of quantitative data [ 44 ]. However, as known in case of change in origin and scale, dichotomization or ignoring the order has its own disadvantage. Armstrong and Sloan (1989) found that compared to a cumulative logit model of a five level ordered response, logistic model attains only 50–75% of efficiency [ 29 ].
In this study, ordinal categories mainly relied on desired varying management strategies being adopted by the oncologists according to cut-off values. Accordingly, number of involved cervical nodes were categorized as 0, 1, 2–4 and > 4 involved nodes. Until now, there is no study considering the ordinal form of nodal involvement while assessing its associated factors. The available studies have dealt with only binary form of nodal involvement [ 2 , 14 , 24 , 30 , 45 , 46 , 47 ].
In the present study, POM seems to be appropriate as overall model did not violate the proportional odds assumption significantly. However, one of the covariates was found to violate this assumption. Theoretical ground or empirical tests do not provide clear guidelines about when to relax the proportional odds assumption [ 48 ]. Under exploration of this possibility, either to use POM or PPOM, log likelihood, AIC and likelihood ratio test supported the PPOM. Also, a clinically relevant covariate, tumor size emerged to be significant under PPOM. The availability of GOLOGIT2 with AUTOFIT syntax in STATA makes the things easier to select the appropriate model between POM and PPOM [ 35 , 42 ].
Results under binary form of nodal involvement on the same dataset and considering same set of covariates are already reported elsewhere [ 30 , 31 ]. Under appropriate consideration of the nodal involvement in the ordinal form, one of the clinically more relevant covariates i.e., histopathological tumor size, was also found to be significantly associated with extent of nodal involvement, which was missed in its binary consideration. However, other significant covariates were similar and also the effect size was in same direction. Under model assessment, model for binary as well as ordinal form of nodal involvement described the distribution satisfactorily.
The analytical results under the present study reveal that in case of ordinal form of the outcome, ordinal regression may be a preferred choice. Further, in case of violation of proportionality assumption in any of the covariates, PPOM may be a better choice. This is likely to ensure accuracy not only in results but also in related inferences and their implications. In summary, pain at the time of presentation, sub mucous fibrosis, clinically palpable neck node, oral site, degree of differentiation and tumor size are the most probable associated factors with extent of nodal involvement in oral squamous cell carcinoma patients.
As mentioned earlier, the ordinal categories mainly relied on desired varying management strategies being adopted by the oncologists according to cut-off values of involved nodes. Otherwise, as true in case of any such study, change in cut-off values of nodal involvement is bound to change the results. Further, as true under such modeling, finite sample bias may remain a concern in these models as well.
Availability of data and materials
All the data are from the Department of Surgical Oncology, Dr. BRA-IRCH, All India Institute of Medical Sciences, New Delhi, India. The datasets are available upon request from SVS Deo.
Abbreviations
Proportional odds model
Partial proportional odds model OSCC: Oral squamous cell carcinoma
Retromolar triangle
Sub mucous fibrosis
95% Confidence interval
Lower class
Lower middle class
Upper class
Upper middle class
Ulceroinfiltrative
Ulceroproliferative
Well Differentiated
Degree of differentiation
Clinical skin involvement
Clinical bone involvement
Unadjusted odds ratio
Adjusted odds ratio
World Health Organization. International agency for research on cancer. GLOBOCAN 2012. <globocan.iarc.fr/pages/fact_sheets_cancer.aspx> [accessed 13-01-2015].
Karino M, Nakatani E, Hideshima K, et al. Applicability of preoperative nuclear morphometry to evaluating risk for cervical lymph node metastasis in oral squamous cell carcinoma. PLoS One. 2014;9:1–15.
Article Google Scholar
De Matos LL, Manfro G, Dos Santos RV, et al. Tumor thickness as a predictive factor of lymph node metastasis and disease recurrence in T1N0 and T2N0 squamous cell carcinoma of the oral tongue. Oral Surg Oral Med Oral Pathol Oral Radiol. 2014;118:209–17.
Warburton G, Nikitakis NG, Roberson P, et al. Histopathological and Lymphangiogenic parameters in relation to lymph node metastasis in early stage Oral squamous cell carcinoma. J Oral Maxillofac Surg. 2007:475–84.
Mendez E, Fan W, Choi P, et al. Tumor-Specific Genetic Expression Profile Of Metastatic Oral Squamous Cell Carcinoma. Head Neck. 2007:803–14.
Massano J, Regateiro FS, Juanario G, et al. Oral squamous cell carcinoma: review of prognostic and predictive factors. Oral Surg Oral Med Oral Pathol Oral Radiol Endod. 2006;102(1):67–76.
Okura M, Iida S, Aikawa T, Adachi T, et al. Tumor thickness and Paralingual distance of coronal MR imaging predicts cervical node. Am J Neuroradiol. 2008;29(1):45–50.
Article CAS Google Scholar
Deo SVS, Shukla NK, Jha D. Are we over-treating neck in Buccal & Alveolo-buccal Cancers: experience from a tertiary Cancer care center. Ind J Surg Oncol. 2012;3:272–5.
Hoch S, Fasunla J, Eivazi B, et al. Delayed lymph node metastases after elective neck dissection in patients with oral and oropharyngeal cancer and pN0 neck. Am J Otolaryngol Neck Med Surg. 2012;33:505–9.
Google Scholar
Kim K-Y, Cha I-H. A novel algorithm for lymph node status prediction of oral cancer before surgery. Oral Oncol. 2011;47:1069–73.
Lim YC, Koo BS, Lee JS, et al. Level V lymph node dissection in Oral and Oropharyngeal carcinoma patients with clinically node-positive neck: is it absolutely necessary? Laryngoscope. 2006:1232–5.
Montes DM, Carlson ER, Fernandes R, et al. Oral maxillary squamous carcinoma: an indication for neck dissection in the clinically negative neck. Head Neck. 2011;33(11):1581–5.
Liao C, Hsueh C, Lee L, et al. Neck dissection field and lymph node density predict prognosis in patients with oral cavity cancer and pathological node metastases treated with adjuvant therapy. Oral Oncol. 2012;48:329–36.
Wu K, Yang X, Li L, et al. Neurovascular invasion and histological grade serve as the risk factors of cervical lymph node metastases in early tongue squamous cell carcinoma. Mol Neurobiol. 2015;53(5):2920–6.
Kang C, Liao C, Hsueh C, et al. Outcome analysis of patients with well-differentiated oral cavity squamous cell carcinoma. Oral Oncol. 2011;47:1085–91.
Sekine J, Uehara M, Hideshima K, et al. Predictability of lymph node metastases by preoperative nuclear morphometry in squamous cell carcinoma of the tongue. Cancer Detect Prev. 2003;27:427–33.
Pimenta Amaral TM, Da Silva Freire AR, Carvalho AL, et al. Predictive factors of occult metastasis and prognosis of clinical stages I and II squamous cell carcinoma of the tongue and floor of the mouth. Oral Oncol. 2004;40:780–6.
Sekine J, Uehara M, Hideshima K, et al. Predictability of lymph node metastases by preoperative nuclear morphometry in squamous cell carcinoma of the tongue. Cancer Detect Prev. 2003;27(6):427–33.
Briggs RJS, Pienta KJ, Hruban H, et al. Nuclear Morphometry for prediction of metastatic potential in early squamous cell carcinoma of the floor of the mouth squamous. Archives of Otolaryngology. Head and Neck Surgery. 1992;118(5):531–3.
Chone CT, Aniteli MB, Magalhães RS, et al. Impact of immunohistochemistry in sentinel lymph node biopsy in head and neck cancer. Eur Arch Otorhinolaryngol. 2013:313–7.
Lim S, Zhang S, Ishii G, et al. Predictive markers for late cervical metastasis in stage I and II invasive squamous cell carcinoma of the Oral tongue. Clin Cancer Res. 2004;10:166–72.
Kawano K, Yanagisawa S. Original article predictive value of Laminin-5 and membrane type 1-matrix metalloproteinase expression for cervical lymph node metastasis in T1 and T2 squamous cell carcinomas of the tongue. Head Neck. 2006;28(6):525–33.
Harada H, Medical T, Omura K, et al. Cyclin B1 is useful to predict occult cervical lymph node metastases in tongue carcinoma Cyclin B1 is useful to predict occult cervical lymph node metastases in tongue carcinoma. J Exp Clin Cancer Res. 2006;25(3):351–6.
CAS PubMed Google Scholar
Byers RM, El-Naggar AK, Lee YY, et al. Can we detect or predict the presence of occult nodal metastases in patients with squamous carcinoma of the oral tongue? Head Neck. 1998;20:138–44.
d’Alessandro AF, Pinto FR, Lin CS, et al. Oral cavity squamous cell carcinoma: factors related to occult lymph node metastasis. Braz J Otorhinolaryngol. 2015;81:248–54.
Kowalski LP, Sanabria A. Elective neck dissection in oral carcinoma: a critical review of the evidence. Acta Otorhinolaryngol. 2007;27:113–7.
CAS Google Scholar
Suzuki M, Suzuki T, Asai M, et al. Clinicopathological factors related to cervical lymph node metastasis in a patient with carcinoma of the oral floor. Acta Otolaryngol. 2007;127:129–35.
Cande V Ananth, and David G Kleinbaum. Regression models for ordinal responces a review of methods and application. International journal of epidemiology. 1997; Vol. 26, No. 6.
Armstrong BB, Sloan M. Ordinal regression models for epidemiological data. Am J Epidemiol. 1989;129(1):191–204.
Singh V, Deo SVS, Dwivedi SN, et al. An Epidemiological Model to Find out Factors Associated with Nodal Involvement among. Ind Oral Cancer Patients Open J Epidemiol. 2018;8:117–29.
Singh V, Dwivedi AK, Dwivedi SN, et al. Comparative Appraisal of Estimated Odds Ratio and Risk Ratio Using Binary Regression Models: Analysis of Nodal Involvement Among Oral-Cancer Patients. Biostatistics Biometrics Open Access Journal. 2018;8(4).
Brant R. Assessing proportionality in the proportional odds model for ordinal logistic regression. Biometrics. 1990;46(4):1171–8.
Van Calster B, Van Belle V, Vergouwe Y, et al. Discrimination ability of prediction models for ordinal outcomes: relationships between existing measures and a new measure. Biom J. 2012;54(5):674–85.
Fagerlanda MW, Hosmer DW. A goodness-of-fit test for the proportional odds regression model Statistics in medicine. 2013;32(13):2235–49.
Scott Long J, Freese J. Regression models for categorical dependent variablesusing Stata. Third Edition. College Station: Stata Press; 2014.
Lipsitz SR, Fitzmaurice GM, Molenberghs G. Goodness-of-fit tests for ordinal response regression models. Appl Stat. 1996;45(2):175–90.
McCullagh P. Regression models for ordinal data (with discussion). J R Statis Soc Series B. 1980;42:109–42.
Cox C, Chuang C. A comparison of chi-square partitioning and two logit analyses of ordinal pain data from a pharmaceutical study. Stat Med. 1984;3:273–85.
Ananth CV, Kleinbaum DG. Regression models for ordinal responses: a review of methods and applications. International Journal of Epidemiology. 1997; Vol. 26, No. 6.
Walker SH, Duncan DB. Estimation of the probability of an event as a function of several independent variables. Biometrika. 1967;54:167–79.
Peterson BL, Harrell FE. Partial proportional odds models for ordinal response variables. Appl Stat. 1990;39:205–17.
Richard Willianms. Generalized ordered logit/ partial proportional odds modes for ordinal dependent variables. The Stata Journal. 2006; Number 1, pp. 58–82.
Altman DG, Royston P. What do we mean by validating a prognostic model? Stat Med. 2000;19:453–73.
Sundaram KR, Dwivedi SN, Sreenivas V. Medical statistics principles & methods. Wolter Kluwer. 2015; 2nd edition. Alphen Aan Den Rijn.
Vartanian JG, Carvalho AL, Filho d A, et al. Predictive factors and distribution of lymph node metastasis in lip Cancer patients and their implications on the treatment of the neck. Oral Oncol. 2004;40:223–7.
Angadi PV, Patil PV, Hallikeri K, et al. Tumor budding is an independent prognostic factor for prediction of lymph node metastasis in Oral squamous cell carcinoma. Int J Surg Pathol. 2015;23:102–10.
Samarakoon B, Samadarani M, Mudiynaselage R. Predictive model of Oral Cancer metastasis for different Cancer sites and age groups. J Investig Clin Dent. 2016;7:127–31.
Fullerton AS, Jun X. The proportional odds with partial proportionality constraints model for ordinal response variables. Soc Sci Res. 2012:182–98.
Download references
Acknowledgements
Thanks to All India Institute of Medical Sciences, New Delhi, for providing the required facilities. Also, thanks to the reviewers, and editor of the journal, for their valuable suggestions to improve the article further.
Nil. Present study is not funded by any external funding agency. It is part Ph.D. work of the first author.
Author information
Authors and affiliations.
Department of Biostatistics, All India Institute of Medical Sciences, New Delhi, 110029, India
Vishwajeet Singh & Sada Nand Dwivedi
Department of Surgical Oncology, Dr BRA-IRCH, All India Institute of Medical Sciences, New Delhi, India
S. V. S. Deo
You can also search for this author in PubMed Google Scholar
Contributions
VS, SND and SVSD conceived and designed the study. VS did the analysis under supervision of SND. VS, SND and SVSD interpreted the results. The final draft of the manuscript was prepared by VS under supervision of SND and SVSD. Each of the three authors read and approved the final manuscript.
Corresponding author
Correspondence to Sada Nand Dwivedi .
Ethics declarations
Ethics approval and consent to participate.
The present study was approved by Ethics Committee for Post graduate research, All India Institute of Medical Sciences, New Delhi, India. Consent to participate is not applicable.
Consent for publication
Not applicable.
Competing interests
The authors declare they have no competing interests.
Additional information
Publisher’s note.
Springer Nature remains neutral with regard to jurisdictional claims in published maps and institutional affiliations.
Rights and permissions
Open Access This article is licensed under a Creative Commons Attribution 4.0 International License, which permits use, sharing, adaptation, distribution and reproduction in any medium or format, as long as you give appropriate credit to the original author(s) and the source, provide a link to the Creative Commons licence, and indicate if changes were made. The images or other third party material in this article are included in the article's Creative Commons licence, unless indicated otherwise in a credit line to the material. If material is not included in the article's Creative Commons licence and your intended use is not permitted by statutory regulation or exceeds the permitted use, you will need to obtain permission directly from the copyright holder. To view a copy of this licence, visit http://creativecommons.org/licenses/by/4.0/ . The Creative Commons Public Domain Dedication waiver ( http://creativecommons.org/publicdomain/zero/1.0/ ) applies to the data made available in this article, unless otherwise stated in a credit line to the data.
Reprints and permissions
About this article
Cite this article.
Singh, V., Dwivedi, S.N. & Deo, S.V.S. Ordinal logistic regression model describing factors associated with extent of nodal involvement in oral cancer patients and its prospective validation. BMC Med Res Methodol 20 , 95 (2020). https://doi.org/10.1186/s12874-020-00985-1
Download citation
Received : 27 November 2019
Accepted : 20 April 2020
Published : 26 April 2020
DOI : https://doi.org/10.1186/s12874-020-00985-1
Share this article
Anyone you share the following link with will be able to read this content:
Sorry, a shareable link is not currently available for this article.
Provided by the Springer Nature SharedIt content-sharing initiative
- Ordinal logistic regression
- Oral cancer
- Nodal involvement
- Squamous cell carcinoma
BMC Medical Research Methodology
ISSN: 1471-2288
- General enquiries: [email protected]

An official website of the United States government
The .gov means it’s official. Federal government websites often end in .gov or .mil. Before sharing sensitive information, make sure you’re on a federal government site.
The site is secure. The https:// ensures that you are connecting to the official website and that any information you provide is encrypted and transmitted securely.
- Publications
- Account settings
Preview improvements coming to the PMC website in October 2024. Learn More or Try it out now .
- Advanced Search
- Journal List
- Cambridge University Press - PMC COVID-19 Collection

Application of ordinal logistic regression analysis to identify the determinants of illness severity of COVID-19 in China
1 Department of Respiration and Critical Care Diseases, Ruijin Hospital, School of Medicine, Shanghai Jiao Tong University, Shanghai, China
2 Institute of Respiratory Diseases, School of Medicine, Shanghai Jiaotong University, Shanghai, China
Dexiang Yang
3 Department of Respiratory Diseases, Tongling People's Hospital, Tongling, China
4 Department of Infectious Disease, Shanghai Public Health Clinical Center, Shanghai, China
5 Department of Respiratory and Critical Care Medicine, Tongji Medical College of Huazhong University of Science and Technology, Wuhan, China
6 Department of Infectious Disease, Wuhan Jinyintan Hospital, Wuhan, China
Zenghui Cheng
7 Department of Radiology, Ruijin Hospital, School of Medicine, Shanghai Jiao Tong University, Shanghai, China
8 Clinical Research Center, Ruijin Hospial, School of Medicine, Shanghai Jiao Tong University, Shanghai, China
Associated Data
For supplementary material accompanying this paper visit http://dx.doi.org/10.1017/S0950268820001533.
The data that support the findings of this study are available from Clinical Research Center, Ruijin Hospital, Shanghai Jiao Tong University School of Medicine. Restrictions apply to the availability of these data, which were used under license for this study.
Corona Virus Disease 2019 (COVID-19) has presented an unprecedented challenge to the health-care system across the world. The current study aims to identify the determinants of illness severity of COVID-19 based on ordinal responses. A retrospective cohort of COVID-19 patients from four hospitals in three provinces in China was established, and 598 patients were included from 1 January to 8 March 2020, and divided into moderate, severe and critical illness group. Relative variables were retrieved from electronic medical records. The univariate and multivariate ordinal logistic regression models were fitted to identify the independent predictors of illness severity. The cohort included 400 (66.89%) moderate cases, 85 (14.21%) severe and 113 (18.90%) critical cases, of whom 79 died during hospitalisation as of 28 April. Patients in the age group of 70+ years (OR = 3.419, 95% CI: 1.596–7.323), age of 40–69 years (OR = 1.586, 95% CI: 0.824–3.053), hypertension (OR = 3.372, 95% CI: 2.185–5.202), ALT >50 μ/l (OR = 3.304, 95% CI: 2.107–5.180), cTnI >0.04 ng/ml (OR = 7.464, 95% CI: 4.292–12.980), myohaemoglobin>48.8 ng/ml (OR = 2.214, 95% CI: 1.42–3.453) had greater risk of developing worse severity of illness. The interval between illness onset and diagnosis (OR = 1.056, 95% CI: 1.012–1.101) and interval between illness onset and admission (OR = 1.048, 95% CI: 1.009–1.087) were independent significant predictors of illness severity. Patients of critical illness suffered from inferior survival, as compared with patients in the severe group (HR = 14.309, 95% CI: 5.585–36.659) and in the moderate group (HR = 41.021, 95% CI: 17.588–95.678). Our findings highlight that the identified determinants may help to predict the risk of developing more severe illness among COVID-19 patients and contribute to optimising arrangement of health resources.
Introduction
The pandemic of the novel corona virus disease 2019 (COVID-19), which originally emerged in Wuhan, China in December 2019 has spread around the world [ 1 , 2 ]. As of 5 June 2020, the WHO has reported a total of 6 535 354 COVID-19 cases and 387 155 deaths globally, with an average mortality of 5.92% and the person-to-person transmission is still continuing [ 3 ]. The clinical spectrum of COVID-19 appears to be wide, ranging from asymptomatic infection to mildly, severely, critically ill cases. Mild patients present only upper respiratory tract symptoms like cough and fever, however, respiratory failure, acute respiratory distress syndrome, heart failure, septic shock and even death can be observed in patients with critical conditions [ 4 ]. Although most confirmed patients (81%) were classified as mild or moderate, 14% were severe and 5% were critical according to the largest investigation of 72 314 cases to date [ 5 ]. Accumulated evidences have indicated that older age, male, smoking, comorbidity, neutrophilia, coagulopathy, elevated D-dimer level and organ dysfunction were associated with increased risk of death from COVID-19 [ 5 – 10 ]. However, investigations of determinants of severity of COVID-19 are scarce. Early detecting cases with the potential deterioration of disease will contribute to optimising the use of limited health resources and allocating the proper care. Liang et al . developed a clinical risk score to predict the occurrence of critical COVID-19 based on severe or non-severe [ 11 ]. To our knowledge, no previous studies have been conducted to investigate the risk factors of severity of COVID-19 based on ordinal response, namely moderate, severe and critical illness. The estimation of risk factors of disease severity is therefore not very robust.
Here, we conducted a retrospective study based on COVID-19 patients from four designated hospitals in Shanghai, Hubei and Anhui provinces to describe the clinical features of COVID-19, and aimed to identify the predictors of multi-level response of severity from moderate, severe to critical illness.
Study design and participants
This multi-centre retrospective study encompassed COVID-19 patients classified as being moderately, severely and critically ill. The illness severity of COVID-19 was defined according to the Guideline on the Diagnosis and Treatment of COVID-19 by the National Health Commission (V.5) as described previously [ 12 ]. Patients were admitted to Shanghai Public Health Clinical Center, Wuhan Jinyintan Hospital and Tongji Hospital of Tongji Medical College HUST in Hubei province and Tongling Municipal People's Hospital in Anhui province from 1 January 2020 to 8 March 2020. All patients recruited in this study were laboratory-confirmed COVID-19. The study was approved by the Ethics Committees of these four hospitals, respectively. Written informed consent was waived owing to the need of rapid emergency response to this infectious disease.
Data collection
Medical records of COVID-19 patients were reviewed by the research team, and demographic, epidemiological, clinical, laboratory, treatment and outcome data were retrieved from electronic medical records using a standardised case report form. All data were cross-checked by two experienced doctors. To ascertain the medical histories or epidemiological data, which were unavailable from electronic medical records, the patients or their close relatives were interviewed by researchers. Data from the medical records were adopted if there was a discrepancy between the subjective description and the medical records.
Laboratory procedures
Method of laboratory confirmation of severe acute respiratory syndrome coronavirus 2 (SARS-CoV-2) has been described elsewhere [ 1 ]. Simply, the Chinese Center for Disease Control and Prevention (CDC) and local CDC were in charge of detecting SARS-CoV-2 in throat-swab specimens from the upper respiratory tract by real-time reverse transcription polymerase chain reaction assay (RT-PCR). The criteria of discharge included absence of fever for at least 3 days, remission of respiratory symptoms, complete improvement in bilateral lungs in chest CT, together with negative for 2 times in throat-swab samples for SARS-CoV-2 RNA at least 24 h apart.
Initial clinical laboratory examinations involved complete blood count, serum biochemical tests (including liver and kidney functions, creatine kinase, lactate dehydrogenase (LDH) and electrolytes), myocardial enzymes, D-dimer and procalcitonin (PCT). Frequency of examinations was under the discretion of treating physicians. Chest computed tomographic (CT) scans were carried out for all COVID-19 patients. Two radiologists were invited to interpret chest CT scans independently and were blinded to the severity of the patient. When disagreement arose, a third radiologist was consulted to reach a final decision.
Statistical analysis
Continuous variables were presented as median with interquartile range (IQR) and the analysis of variance (ANOVA) or Kruskal−Wallis H test were used to compare the difference among three groups as appropriate. Categorical variables were expressed as frequency with percentages, and were analysed by Pearson's χ 2 test or Fisher's exact test. Bonferroni's correction was used for pairwise comparison. All patients were divided into moderate, severe and critical illness groups. Potential predictive variables included the following case characteristics on admission: demographic and epidemiological features, comorbidity, clinical signs and symptoms, laboratory findings and chest imaging results. To explore the risk factors associated with illness severity of COVID-19, namely moderately, severely and critically ill, which means the response variable was ordinally scaled, a cumulative logit model was used to investigate the effect of predictors of COVID-19 severity. Imputation for missing variables of some patients at hospital admission was considered if missing values were less than 20%, and imputation based on the expectation−maximisation algorithm method was used to replace missing values. Before ordinal logistic regression model was fitted, continuous variables of laboratory findings were transformed into categorical variables according to their reference values. The univariate and multivariate cumulative logit models were fitted with moderate illness as the reference level. Potential predictors of severity were investigated using univariate ordinal logistic regression firstly. We further conducted a backward stepwise multivariate ordinal logistic regression analysis excluding variables which were not significant in univariate cumulative logit model. Since missing rate of 34.6% occurred in the lung imaging results and over 40% existed in urine protein and urine glucose, these variables were excluded from multivariate ordinal logistic model. The overall survival (OS) was estimated using the method of Kaplan−Meier and the log-rank test was applied to compare the survival difference among different severity illness groups. The hazard ratio with 95% confidence interval (CI) was estimated with Cox proportional hazard model. A two-sided α of less than 0.05 was considered statistically significant. All statistical analyses were conducted using SAS software (V. 9.4) (SAS Institute Inc., USA).
Demographics, laboratory findings and clinical course
As of 28 April 2020, data from 598 COVID-19 cases admitted to these four hospitals, including 400 (66.89%) moderate cases, 85 (14.21%) severe cases and 113 (18.90%) critical cases, had been collected to be incorporated into this study, of whom 79 cases had died during hospitalisation, with an average mortality of 13.21%, and 457 cases had recovered and been discharged. The remaining 62 cases were still in hospitals. The median age of the 598 patients was 57 years (IQR 42–66), ranging from 11 to 89 years, and 58.03% patients were male ( Table 1 ). At least one comorbidity was present in 51.54% of patients, with hypertension being the most frequent comorbidity (33.90%), followed by diabetes (13.18%) and cardiovascular disease (6.51%). Few cases had a current (7.53%) or former (2.57%) smoking habit. The most common symptoms on admission were fever (81.22%) and dry cough (33.63%), followed by sputum production (30.77%) and shortness of breath (26.65%). Overall, the median interval between illness onset and confirmed diagnosis was 4 days (IQR 2–7), whereas the median interval between illness onset and admission was 7 days (IQR 3–11).
Clinical characteristics and comorbidities of 598 COVID-19 patients
Variable | All ( = 598) | Illness Severity | value | ||
---|---|---|---|---|---|
Moderate ( = 400) | Severe ( = 85) | Critical ( = 113) | |||
Age (years) | 57 (42–66) | 52 (39–64)*† | 61 (49.5–67)* | 65.5 (58.25–72) | <0.0001 |
Sex | 0.067 | ||||
Male | 347/598 (58.03) | 219/400 (54.75) | 54/85 (63.53) | 74/113 (65.49) | |
Female | 251/598 (41.97) | 181/400 (45.25) | 31/85 (36.47) | 39/113 (34.51) | |
Smoking | 0.883 | ||||
Never | 525/584 (89.90) | 355/399 (88.97) | 74/85 (87.06) | 91/100 (91.00) | |
Current | 44/584 (7.53) | 33/399 (8.27) | 4/85 (4.71) | 7/100 (7.00) | |
Former | 15/584 (2.57) | 11/399 (2.76) | 2/85 (2.35) | 2/100 (2.00) | |
Symptoms | |||||
Fever (temperature ⩾37.3°C) | 454/559 (81.22) | 324/400 (81.00) | 70/85 (82.35) | 60/74 (81.08) | 0.958 |
Dry cough | 188/559 (33.63) | 120/400 (30.00)* | 43/85 (50.59) | 25/74 (33.78) | 0.001 |
Sputum production | 172/559 (30.77) | 120/400 (30.00) | 22/85 (25.88) | 30/74 (40.54) | 0.112 |
Pharyngodynia | 38/559 (6.80) | 31/400 (7.75) | 6/85 (7.06) | 1/74 (1.35) | 0.132 |
Chest pain | 28/559 (5.01) | 18/400 (4.50) | 5/85 (5.88) | 5/74 (6.76) | 0.581 |
Shortness of breath | 149/559 (26.65) | 59/400 (14.75)*† | 59/85 (69.41)* | 31/74 (41.89) | <0.0001 |
Any comorbidity | 301/584 (51.54) | 167/399 (41.85) | 40/85 (47.06) | 94/100 (94.00) | <0.0001 |
Hypertension | 198/584 (33.90) | 91/399 (22.81)*† | 31/85 (36.47)* | 76/100 (76.00) | <0.0001 |
Cardiovascular disease | 38/584 (6.51) | 25/399 (6.27) | 4/85 (4.71) | 9/100 (9.00) | 0.469 |
Diabetes | 77/584 (13.18) | 42/399 (10.53)* | 7/85 (8.24)* | 28/100 (28.00) | <0.0001 |
Carcinoma | 21/584 (3.60) | 11/399 (2.76)* | 2/85 (2.35) | 8/100 (8.00) | 0.048 |
Cerebrovascular disease | 19/584 (3.25) | 13/399 (3.26) | 0/85 (0.00) | 6/100 (6.00) | 0.052 |
COPD | 11/584 (1.88) | 7/399 (1.75) | 2/85 (2.35) | 2/100 (2.00) | 0.809 |
Others | 99/584 (16.95) | 86/399 (21.55)*† | 6/85 (7.06) | 7/100 (7.00) | <0.0001 |
Days from illness onset to diagnosis confirmed | 4 (2−7) | 4 (2−6) | 8 (5−13)* | 7 (4−11) | <0.0001 |
Days from illness onset to admission | 7 (3−11) | 6 (3−10)*† | 10 (6−14) | 10 (0−14) | <0.0001 |
COPD, chronic obstructive pulmonary disease.
Data are median (IQR) or n / total (%). P value denotes the comparison among moderate, severe and critical illness group. * and †Signify P < 0.05 for pos-hoc comparison. *Refers to comparison between the critical group and the severe group or the moderate group. †Refers to comparison between the severe group and the moderate group.
The substantial differences of laboratory findings on admission among three groups of patients were observed ( Table 2 ). The results indicated that significantly higher proportion of patients showing abnormal alanine aminotransferase (ALT) and aspartate aminotransferase (AST), and significantly higher levels of C-reactive protein, neutrophil count, serum potassium, cardiac troponin I (cTnI), myohaemoglobin, PCT, brain natriuretic peptide (BNP) and D-dimer were observed in the critically ill group and the severely ill group than in the moderately ill group, whereas, the levels of haemoglobin and serum albumin were significantly lower in critically ill group and severely ill group as compared with moderately ill group ( P < 0.05). The critical group showed a significantly higher level of platelet count, fibrinogen and serum calcium as compared with severe group and moderate group. The severe group showed a significantly lower level of lymphocyte count than the moderate group. The normal ranges of laboratory indicators are shown in Supplementary Table S1. Abnormalities on chest radiographs on admission were seen in most patients ( Table 2 ). Overall, typical findings on chest CT images were ground-glass opacity (94.37%), followed by pleural thickening (47.06%) and consolidation (35.74%). Chest CT scans showed significantly higher percentage of bilateral lungs involvement in critical group (92.45%) and severe group (95.24%) as compared with moderate group (80.07%). The median lung lobes involved in critical group (5, IQR 5–5) and severe group (5, IQR 2.75–5) were greater than those in moderate group (4, IQR 2–5).
Laboratory and chest CT findings on admission of 598 COVID-19 patients
Variable | All ( = 598) | Disease severity | value | ||
---|---|---|---|---|---|
Moderate ( = 400) | Severe ( = 85) | Critical ( = 113) | |||
Laboratory findings | |||||
C-reactive protein (mg/l) | 18.80 (5.17–60.22) | 15.40 (4.85–42.98)*† | 63.05 (18.55–159.43) | 37.00 (3.95–129.35) | <0.0001 |
White blood cell count (×10 /l) | 5.51 (4.38–7.52) | 5.22 (4.20–6.63)* | 7.03 (4.62–10.21) | 6.26 (4.91–8.76) | <0.0001 |
Neutrophil count (×10 /l) | 3.59 (2.70–5.37) | 3.40 (2.54–4.69)*† | 5.27 (3.37–8.94) | 3.68 (3.09–5.53) | <0.0001 |
Lymphocyte count (×10 /l) | 1.03 (0.69–1.48) | 1.10 (0.76–1.49)† | 0.86 (0.60–1.18) | 0.94 (0.57–1.49) | 0.002 |
<1.0 × 10 /l | 285/598 (47.66) | 166/400 (41.5) † | 58/85 (68.24) | 61/113 (53.98) | <0.0001 |
Haemoglobin (g/l) | 132 (120–143) | 134 (123–145)*† | 129 (114–138) | 127 (113–136) | <0.0001 |
Platelet count (×10 /l) | 188 (146–253) | 185 (145–238)* | 188 (144–240)* | 274 (178–338) | <0.0001 |
ALT >50 μ/l | 136/598 (22.74) | 60/400 (15.0) *† | 23/85 (27.06) * | 53/113 (46.90) | <0.0001 |
AST >40 μ/l | 174/598 (29.10) | 82/400 (20.50) *† | 33/85 (38.82) | 59/113 (52.21) | <0.0001 |
Total bilirubin (μmol/l) | 9.8 (7.5–13.8) | 9.7 (7.3–14.0) | 10.7 (8.8–14.8)* | 9.0 (7.2–13.0) | 0.025 |
Direct bilirubin (μmol/l) | 3.9 (3.1–5.6) | 4.0 (3.0–5.7) | 4.0 (3.1–5.5) | 3.9 (3.1–5.9) | 0.654 |
Albumin (g/l) | 36.94 (32.48–41.32) | 38.88 (34.55–42.40)*† | 31.90 (28.73–34.28)* | 34.05 (31.48–37.58) | <0.0001 |
Blood urea nitrogen (mmol/l) | 4.80 (3.70–5.90) | 4.75 (3.66–5.82) | 5.10 (3.80–6.35) | 4.80 (4.10–5.80) | 0.804 |
Creatinine (μmol/l) | 68.12 (55.00–81.05) | 65.51 (52.93–78.27)* | 70.30 (57.00–82.50) | 76.00 (62.25–90.50) | <0.0001 |
Urinary glucose | 0.956 | ||||
Negative | 268/325 (82.46) | 184/222 (82.89) | 48/59 (81.36) | 36/44 (81.82) | |
Positive | 57/325 (17.54) | 38/222 (17.11) | 11/59 (18.64) | 8/44 (18.18) | |
Urinary protein | 0.087 | ||||
Negative | 224/343 (65.31) | 150/222 (67.57) | 44/64 (68.75) | 30/57 (52.63) | |
Positive | 119/343 (34.69) | 72/222 (32.43) | 20/64 (31.25) | 27/57 (47.37) | |
Sodium (mmol/l) | 139 (137–141) | 139 (137–141)† | 141 (138––142)* | 139 (136–142) | 0.007 |
Potassium (mmol/l) | 3.9 (3.6–4.3) | 3.9 (3.6–4.1)*† | 4.3 (3.8–4.7) | 4.2 (3.8–4.5) | <0.0001 |
Calcium (mmol/l) | 2.07 (1.98–2.17) | 2.05 (1.97–2.16)* | 2.04 (1.94–2.14)* | 2.16 (2.08–2.25) | <0.0001 |
LDH (μ/l) | 264 (207–376) | 242 (197–326) | 345 (238–479) | 320 (249–497) | 0.767 |
Creatine kinase (μ/l) | 82 (54–151) | 79 (53–137) | 96 (55–184) | 81 (53–198) | 0.619 |
CK-MB (μ/l) | 9.97 (13.00–17.00) | 12.94 (10.41–16.31)* | 13 (10.00–18.00) | 10.00 (0.78–19.25) | 0.035 |
Myohaemoglobin (ng/ml) | 24.51 (5.76–59.80) | 13.41 (3.91–41.30)*† | 61.55 (33.10––95.70) | 67.40 (32.40–160.10) | <0.0001 |
cTnI (ng/ml) | 0.07 (0.02–5.00) | 0.02 (0.01–0.07)*† | 4.85 (2.00–15.13) | 6.49 (2.80–19.68) | <0.0001 |
PCT (μg/l) | 0.05 (0.02–0.09) | 0.03 (0.02–0.06) *† | 0.06 (0.05–0.18) | 0.08 (0.05–0.24) | <0.0001 |
ESR (mm/h) | 49 (29–79) | 47 (27–83) | 51 (35–72) | 52 (29–68) | 0.638 |
BNP (pg/ml) | 46.00 (24.00–101.00) | 36.00 (22.00–72.50)*† | 61.00 (34.00–150.00) | 106.00 (52.80–260.00) | <0.0001 |
Fibrinogen (g/l) | 4.60 (3.72–5.81) | 4.30 (3.62–5.27)* | 5.20 (3.44–6.20)* | 6.49 (4.86–11.48) | <0.0001 |
D-dimer (μg/l) | 0.61 (0.35–1.39) | 0.50 (0.32–0.99)*† | 0.98 (0.56–3.14) | 1.07 (0.58–2.65) | <0.0001 |
Chest CT findings | |||||
Lung lobes involved | 4 (2–5) | 4 (2–5)* | 5 (2.75–5) | 5 (5–5) | <0.0001 |
Bilateral lungs involved | 326/391 (83.38) | 237/296 (80.07)*† | 40/42 (95.24) | 49/53 (92.45) | 0.008 |
Consolidation | 104/391 (35.74) | 91/296 (30.74)† | 3/42 (7.14) | 10/53 (18.87) | 0.002 |
Ground-glass opacity | 369/391 (94.37) | 277/296 (93.58) | 42/42 (100.00) | 48/53 (90.57) | 0.123 |
Linear opacity | 94/391 (24.04) | 62/296 (20.94)† | 20/42 (47.62)* | 12/53 (22.64) | 0.001 |
Pleural effusion | 22/391 (5.63) | 16/296 (5.41) | 3/42 (7.14) | 3/53 (5.67) | 0.866 |
Pleural thickening | 184/391 (47.06) | 149/296 (50.34)† | 13/42 (30.95) | 22/53 (41.51) | 0.043 |
ALT, alanine aminotransferase; AST, aspartate aminotransferase. LDH, lactate dehydrogenase; CK-MB, creatine kinase isoenzymes; cTnI, cardiac troponin I; PCT, procalcitonin; ESR, erythrocyte sedimentation rate; BNP, brain natriuretic pepti.
Data are median (IQR) or n / total (%). P value denotes the comparison among moderate, severe and critical illness group. * and †Signify P < 0.05 for post-hoc comparison. *Refers to comparison between the critical group and the severe group or the moderate group. †Refers to comparison between the severe group and the moderate group.
Totally, 320 (61.07%) patients were given antivirals within 2 days after admission including lopinavir/ritonavir, arbidol, darunavir and chloroquine. In all, 369 (70.83%) patients received antibiotics and 117 (23.08%) received corticosteroids. More patients received corticosteroids in critical group and severe group as compared with the moderate group ( P < 0.05). The proportions of patients accepting high-flow nasal cannula oxygen therapy and non-invasive mechanical ventilation, respectively, in critical and severe groups were significantly higher than in mode rate group ( P < 0.05). Compared with the moderate group, the critical group and the severe group had a significantly lower rate of discharge and a higher mortality rate ( P < 0.05), as shown in Table 3 . The comparison of demographic and baseline characteristics, symptoms, laboratory parameters, lung image features, treatment and prognosis among moderately, severely and critically ill patients were shown in Tables 1 – 3 .
Treatment and prognosis of 598 COVID-19 patients
Variable | All ( = 598) | Disease severity | value | ||
---|---|---|---|---|---|
Moderate ( = 400) | Severe ( = 85) | Critical ( = 113) | |||
Administration of antiviral | 320/524 (61.07) | 229/395 (57.97)* | 42/62 (67.74) | 49/67 (73.13) | 0.032 |
Administration of antibiotics | 369/521 (70.83) | 253/398 (63.57) | 52/58 (89.66) | 64/65 (98.46) | <0.0001 |
Administration of corticosteroids | 117/507 (23.08) | 40/398 (10.05)*† | 25/51 (49.02)* | 49/58 (84.48) | <0.0001 |
Oxygen therapy | <0.0001 | ||||
Nasal cannula or no oxygen therapy | 459/583 (78.73) | 394/399 (98.75)*† | 45/79 (56.96)* | 20/105 (19.05) | |
High-flow nasal cannula | 47/583 (8.06) | 2/399 (5.01)*† | 7/79 (8.86)* | 38/105 (36.19) | |
Non-invasive mechanical ventilation (face mask) | 54/583 (9.26) | 2/399 (5.01)*† | 27/79 (24.18) | 25/105 (23.81) | |
Invasive mechanical ventilation | 23/583 (3.95) | 1/399 (2.51)* | 0/79 (0.00)* | 22/105 (20.95) | |
Prognosis | <0.0001 | ||||
Discharge | 457/576 (76.42) | 372/400 (93.00)*† | 56/85(65.88)* | 29/113 (25.66) | |
Death | 79/576 (13.21) | 6/400 (1.50)*† | 5/85 (5.88)* | 68/113(60.18) | |
Remained in hospital | 62/576 (10.37) | 22/400(5.50)*† | 24/85 (28.24) | 16/113 (14.16) | |
Secondary viral infection | 16/389 (4.11) | 1/268 (0.37)*† | 2/54 (3.70)* | 13/67 (19.40) | <0.0001 |
Secondary bacterial infection | 10/323 (3.10) | 5/266 (1.88)† | 4/40 (10.00) | 1/17 (5.88) | 0.027 |
Secondary fungal infection | 9/307 (2.93) | 8/263 (3.04) | 1/27 (3.70) | 0/17 (0.00) | 0.756 |
Length of hospital stay – days | 21 (13−39) | 20 (13−35)† | 29 (14−46) | 23 (7−40) | 0.041 |
Determinants of illness severity
Fifty-three variables on admission were successively included in the univariate ordinal logistic regression, and 35 variables were found to be associated with illness severity, including age, gender, hypertension, diabetes, interval between illness onset and diagnosis, interval between illness onset and admission, pharyngodynia, shortness of breath, early administration of antiviral, C-reactive protein, white blood cell (WBC) count, neutrophil count, lymphocyte count, haemoglobin, platelet count, ALT, AST, albumin, blood urea nitrogen, creatinine, potassium, LDH, creatine kinase, myohaemoglobin, troponin I (cTnI), PCT, erythrocyte sedimentation rate (ESR), BNP, fibrinogen, D-dimer, bilateral lungs involved, consolidation, linear opacity, pleural thickening and lung lobes involved ( Table 4 ).
Results of univariate ordinal logistic model using three levels of severity as response
Variable | Level | OR | 95% CI | value | |
---|---|---|---|---|---|
Age (years) | <40 | Ref | |||
40–69 | 1.091 | 2.978 | 1.722–5.150 | <0.0001 | |
⩾70 | 2.139 | 8.492 | 4.548–15.857 | <0.0001 | |
Gender | Male | 0.408 | 1.503 | 1.064–2.124 | 0.0207 |
Female | Ref | ||||
Smoking status | Never | Ref | |||
Current | −0.399 | 0.671 | 0.336–1.340 | 0.2581 | |
Former | −0.361 | 0.697 | 0.221–2.200 | 0.5387 | |
Hypertension | Present | 1.503 | 4.493 | 3.155–6.399 | <0.0001 |
Absent | Ref | ||||
Cardiovascular disease | Present | 0.117 | 1.124 | 0.574–2.199 | 0.7333 |
Absent | Ref | ||||
Diabetes | Present | 0.772 | 2.164 | 1.363–3.437 | 0.0011 |
Absent | Ref | ||||
Carcinoma | Present | 0.788 | 2.198 | 0.963–5.019 | 0.0614 |
Absent | Ref | ||||
COPD | Present | 0.101 | 1.107 | 0.327–3.747 | 0.8708 |
Absent | Ref | ||||
Cerebrovascular disease | Present | 0.148 | 1.159 | 0.458–2.931 | 0.7549 |
Absent | Ref | ||||
Fever | Absent | Ref | |||
Present | 0.041 | 1.042 | 0.653–1.662 | 0.8640 | |
Dry cough | Absent | Ref | |||
Present | 0.038 | 1.039 | 0.726–1.487 | 0.8353 | |
Sputum production | Absent | Ref | |||
Present | −0.175 | 0.840 | 0.577–1.222 | 0.3609 | |
Pharyngodynia | Absent | Ref | |||
Present | −0.917 | 0.400 | 0.170–0.940 | 0.0356 | |
Chest pain | Absent | Ref | |||
Present | 0.077 | 1.080 | 0.495–2.356 | 0.8459 | |
Shortness of breath | Absent | Ref | |||
Present | 1.135 | 3.112 | 2.148–4.507 | <0.0001 | |
Early administration of antiviral | Present | Ref | |||
Absent | 0.465 | 1.592 | 1.138–2.227 | 0.0066 | |
Interval between illness onset and diagnosis | 0.079 | 1.082 | 1.048–1.116 | <0.0001 | |
Interval between illness onset and admission | 0.110 | 1.116 | 1.083–1.150 | <0.0001 | |
CRP (mg/l) | >100 | 1.813 | 6.126 | 3.400–11.039 | <0.0001 |
5–100 | 0.543 | 1.722 | 1.053–2.816 | 0.0304 | |
<5 | Ref | ||||
WBC count (×10 /l) | <4 | Ref | |||
4–10 | 0.186 | 1.204 | 0.735–1.973 | 0.460 | |
>10 | 1.228 | 3.416 | 1.834–6.360 | 0.0001 | |
Neutrophil count (×10 /l) | >6.3 | 1.358 | 3.887 | 1.601–9.442 | 0.0027 |
1.8–6.3 | 0.416 | 1.516 | 0.650–3.536 | 0.3360 | |
<1.8 | Ref | ||||
Lymphocyte count (×10 /l) | <0.5 | 0.987 | 2.684 | 1.508–4.776 | 0.0008 |
0.5–1.1 | 0.449 | 1.567 | 1.096–2.239 | 0.0138 | |
⩾1.1 | Ref | ||||
Haemoglobin (g/l) | ⩾130 | Ref | |||
<130 | 0.435 | 1.545 | 1.104–2.162 | 0.0112 | |
Platelet count (×10 /l) | <125 | Ref | |||
125–350 | 0.315 | 1.370 | 0.802–2.341 | 0.2498 | |
>350 | 1.299 | 3.666 | 1.612–8.335 | 0.0019 | |
ALT –(μ/l) | >50 | 1.331 | 3.783 | 2.593–5.521 | <0.0001 |
⩽50 | Ref | ||||
AST (μ/l) | >40 | 1.227 | 3.410 | 2.388–4.871 | <0.0001 |
⩽40 | Ref | ||||
Total bilirubin (mmol/l) | >20.5 | −0.604 | 0.547 | 0.277–1.079 | 0.0817 |
⩽20.5 | Ref | ||||
Direct bilirubin (mmol/l) | >8.6 | 0.051 | 1.052 | 0.590–1.875 | 0.8626 |
⩽8.6 | Ref | ||||
Albumin (g/l) | <40 | 1.746 | 5.734 | 3.532–9.306 | <0.0001 |
⩾40 | Ref | ||||
Blood urea nitrogen (mmol/l) | >9.5 | 1.181 | 3.257 | 1.811–5.860 | <0.0001 |
⩽9.5 | Ref | ||||
Creatinine (μmol/l) | >111 | 1.293 | 3.645 | 1.927–6.895 | <0.0001 |
⩽111 | Ref | ||||
Urinary glucose | Positive | 0.084 | 1.088 | 0.599–1.976 | 0.7827 |
Negative | Ref | ||||
Urinary protein | Positive | 0.362 | 1.436 | 0.918–2.247 | 0.1133 |
Negative | Ref | ||||
Sodium (mmol/l) | >147 | 0.593 | 1.809 | 0.388–8.433 | 0.4506 |
⩽147 | Ref | ||||
Potassium (mmol/l) | <3.5 | Ref | |||
3.5–5.3 | 1.046 | 2.847 | 1.459–5.557 | 0.0022 | |
>5.3 | 1.642 | 5.164 | 1.664–16.020 | 0.0045 | |
Calcium (mmol/l) | ⩾2.55 | 0.246 | 1.279 | 0.525–3.120 | 0.5882 |
<2.55 | Ref | ||||
LDH (μ/l) | <245 | Ref | |||
⩾245 | 1.119 | 3.062 | 2.114–4.434 | <0.0001 | |
Creatine kinase (μ/l) | ⩽200 | Ref | |||
>200 | 0.594 | 1.811 | 1.211–2.708 | 0.0038 | |
CK–MB (μ/l) | ⩽24 | Ref | |||
>24 | −0.431 | 0.650 | 0.341–1.239 | 0.1905 | |
Myohaemoglobin (ng/ml) | ⩽48.8 | Ref | |||
>48.8 | 1.930 | 6.888 | 4.770–9.945 | <0.0001 | |
cTnI (ng/ml) | ⩽0.04 | Ref | |||
>0.04 | 2.571 | 13.083 | 8.077–21.189 | <0.0001 | |
PCT (μ/l) | ⩽0.05 | Ref | |||
>0.05 | 1.206 | 3.341 | 2.363–4.723 | <0.0001 | |
ESR (mm/h) | ⩽15 | 0.631 | 1.879 | 1.084–3.258 | 0.0247 |
>15 | Ref | ||||
BNP (pg/ml) | ⩽250 | Ref | |||
>250 | 1.361 | 3900 | 2.479–6.136 | <0.0001 | |
Fibrinogen (g/l) | ⩽4 | Ref | |||
>4 | 0.520 | 1.682 | 1.126–2.513 | 0.0111 | |
D-dimer (μg/l) | ⩽0.5 | Ref | |||
>0.5 | 1.266 | 3.548 | 2.414–5.214 | <0.0001 | |
Bilateral lungs involved | Yes | 1.286 | 3.618 | 1.519–8.616 | 0.0037 |
No | |||||
Consolidation | Yes | −0.977 | 0.319 | 0.201–0.704 | 0.0022 |
No | |||||
Ground-glass opacity | Yes | 0.077 | 1.080 | 0.409–2.855 | 0.8766 |
No | |||||
Linear opacity | Yes | 0.522 | 1.685 | 1.015–2.798 | 0.0437 |
No | |||||
Pleural effusion | Yes | 0.139 | 1.149 | 0.439–3.008 | 0.7765 |
No | |||||
Pleural thickening | Yes | −0.510 | 0.600 | 0.375–0.961 | 0.0334 |
No | |||||
Lung lobes involved | 0.426 | 1.531 | 1.270–1.847 | <0.0001 |
COPD, chronic obstructive pulmonary disease; CRP, C-reactive protein; WBC, white blood cell; ALT, alanine aminotransferase; AST, aspartate aminotransferase; LDH, lactate dehydrogenase; CK-MB, creatine kinase isoenzymes; cTnI, cardiac troponin I; PCT, procalcitonin; ESR, erythrocyte sedimentation rate; BNP, brain natriuretic peptid.
Except five lung image variables, 30 significant predictors of severity in univariable analysis were included in a multivariable stepwise cumulative logit model, and seven variables retained in the final model which were statistically significant independent determinants of COVID-19 illness severity ( Table 5 ). The results of multivariate model revealed that the risks of having more severe illness were 1.586 (95% CI: 0.824–3.053) and 3.419 (95% CI: 1.596–7.323) times higher among patients belonging to the age group 40–69 and 70+ years, respectively, when compared with patients of less than 40 years. Patients with hypertension had 3.372 (95% CI: 2.185–5.202) times greater risk of having worse severity of illness compared with patients without hypertension. The risk of having worse severity of illness was found higher for patients with ALT>50 μ/l (OR = 3.304; 95% CI: 2.107–5.180) when compared with those having ALT⩽50 μ/l. The risk of having worse severity of illness was found significantly higher for patients with higher cTnI (>0.04 ng/ml) than those with normal cTnI (⩽0.04 ng/ml) with OR being 7.464 (95% CI: 4.292–12.980). COVID-19 patients with myohaemoglobin>48.8 ng/ml at admission had a 2.214 (95% CI: 1.42–3.453)-fold greater risk of having worse severity of illness when comparison was made with patients having normal myohaemoglobin level. Table 5 also shows that interval between illness onset and diagnosis and interval between illness onset and admission were independent significant predictors of illness severity with OR being 1.056 (95% CI: 1.012–1.101) and 1.048 (95% CI: 1.009–1.087), respectively.
Results of multiple ordinal logistic model using three levels of severity as response
Variable | Level | OR | 95% CI | value | |
---|---|---|---|---|---|
Age (years) | <40 | Ref | |||
40–69 | 0.461 | 1.586 | 0.824–3.053 | 0.1675 | |
⩾70 | 1.229 | 3.419 | 1.596–7.323 | 0.0016 | |
Hypertension | Present | 1.215 | 3.372 | 2.185–5.202 | <0.0001 |
Absent | Ref | ||||
ALT (μ/l) | >50 | 1.195 | 3.304 | 2.107–5.180 | <0.0001 |
⩽50 | Ref | ||||
cTnI (ng/ml) | ⩽0.04 | Ref | |||
>0.04 | 2.010 | 7.464 | 4.292–12.980 | <0.0001 | |
Myohaemoglobin (ng/ml) | ⩽48.8 | Ref | |||
>48.8 | 0.795 | 2.214 | 1.420–3.453 | 0.0005 | |
Days between illness onset and diagnosis | 0.054 | 1.056 | 1.012–1.101 | 0.0111 | |
Days between illness onset and admission | 0.047 | 1.048 | 1.009–1.087 | 0.0142 |
ALT, alanine aminotransferase; cTnI, cardiac troponin I.
Post-hoc comparison of survival among patient groups with different risk factors
In the critical illness group, 68 of 113 patients (60.18%) died, as compared with five of 85 (5.88%) in the severe illness group and six out of 400 (1.50%) in the moderate illness group. The median OS was 29 days in critical group, as compared with not attainable in severe group and moderate group. The 30-day OS rates were 97.7% (95% CI: 95.5–100%), 95.3% (95% CI: 90.2–100%) and 46.4% (95% CI: 37.4–57.6%) in moderate, severe and critical groups, respectively ( P < 0.001), as shown in Figure 1 . Patients of critical illness suffered from inferior survival, as compared with patients in severe group (HR = 14.309, 95% CI: 5.585–36.659) and those in moderate group (HR = 41.021, 95% CI: 17.588–95.678), representing 14.309 times greater risk of death and 41.021 times greater risk of death when compared with severe and moderate groups, respectively. As shown in Supplementary Table S2, COVID-19 patients of older age (HR = 9.823 for ⩾70 vs. <40; HR = 3.361 for 40–69 vs. <40), comorbidity of hypertension (HR = 3.161), abnormal ALT (HR = 1.657), abnormal cTnI (HR = 2.513) or abnormal myohaemoglobin (HR = 2.671) suffered from inferior survival. Also, the Kaplan−Meier survival curves (Supplementary Figs S1–S5) demonstrated that the differences of OS with respect to stratification by these risk factors were all statistically significant ( P < 0.05).

Kaplan−Meier estimate of OS of COVID-19 patients according to severity of illness.
COVID-19 has presented an unprecedented challenge to the health-care system across the world. With the increasingly scarce health resources, mortality is the most important issue when dealing with epidemics. Early identifying potential of severe and critical patients becomes the priority in minimising the mortality and contributes to allocation of limited critical care. Previously, a risk forecasting model to predict the occurrence of critical illness among hospitalised COVID-19 patients in China has been reported by Liang et al . [ 11 ]. The response variable in Liang's model had just two levels of severe and non-severe. Actually, the mortalities of COVID-19 patients with different severity are variant. This study demonstrated the mortality of 60.18% in critical cases, followed by 5.88% in severe cases and 1.50% in moderate cases. Kaplan−Meier analysis also showed that patients of critical illness suffered from inferior survival, as compared with patients of severe illness and moderate illness, indicating that illness severity is related to the prognosis. In our opinion, more precise classification of illness severity into three levels suits the clinical spectrum of COVID-19 and the predicting model based on multi-level ordinal response variables can be more practical. The current multi-centre retrospective study identified the risk factors of illness severity among COVID-19 inpatients from four hospitals of Hubei, Anhui and Shanghai. According to the Guideline on the Diagnosis and Treatment of COVID-19 by the National Health Commission, inpatients of COVID-19 in this study were divided into moderately ill, severely ill and critically ill groups, and the ordinal logistic regression model was fitted to identify the predicators of severity of illness. Based on current data, older age, comorbidity of hypertension, elevated levels of ALT, elevated cTnI, elevated myohaemoglobin, together with prolonged interval between illness onset and diagnosis and interval between illness onset and admission were independent determinants of severity of COVID-19 and represented higher odds of worse severity of illness.
Evidence is gradually accumulating with regard to the risk factors associated with severity of COVID-19. As described previously, older age has been reported as an important independent predictor of severity in COVID-19 patients [ 5 , 6 , 11 ], which is proved by this study. Comorbidity of hypertension was found to be associated with an increased risk of death. A meta-analysis including 46 248 patients with confirmed COVID-19 indicated that those with the most severe illness were more likely to have hypertension with OR of 2.36 (95% CI: 1.46–3.83). Hypertension was reported to increase the OR for death by 3.05 (95% CI: 1.57–5.92) in patients with COVID-19 [ 4 ]. Similarly, patients with hypertension had 3.372 (95% CI: 2.185–5.202) times greater risk of developing worse severity of illness in comparison with those without hypertension in our study. The relationship between hypertension and COVID-19 may relate to the role of angiotensin converting enzyme (ACE2) [ 13 ]. As a key element in the renin–angiotensin–aldosterone system (RAAS), ACE2 is critically involved in the pathophysiology of hypertension. Studies demonstrated that inhibition of the RAAS with ACE inhibitors (ACEIs) or angiotensin II receptor blockers (ARBs) may result in a compensatory increase in tissue levels of ACE2 and poorer clinical course and prognosis, leading to suggestions that these drugs may be detrimental to COVID-19 patients [ 14 ].
It has been reported that COVID-19 had significant impact on the liver function [ 15 , 16 ]. A meta-analysis including 20 retrospective studies with 3428 COVID-19 patients revealed that higher serum levels of AST (mean difference = 8.84 U/l, 95% CI: 5.97–11.71, P < 0.001) and ALT (mean difference = 7.35 U/l, 95% CI: 4.77–9.93, P < 0.001) and lower serum levels of albumin (mean difference = −4.24 g/l, 95% CI: −6.20 to −2.28, P < 0.001) were associated with a significant increase in the severity of COVID-19 [ 17 ]. Our univariate logistic regression results also showed patients with elevated AST, ALT and decreased albumin had 3.410-, 3.783- and 5.734-fold greater risk of worse severity, which was consistent with this meta-analysis. Particularly, our multivariate ordinal regression demonstrated that ALT is an independent predictor of severity of COVID-19 patients. Since elevated liver injury indicators are strongly associated with the severity risk and subsequent death risk, the liver function should be monitored during hospitalisation. Acute myocardial injury is the most commonly described cardiovascular complication in COVID-19 [ 18 ]. The overall incidence of acute myocardial injury has been variable but roughly 8–12% of COVID-19 patients are found to develop significant elevation of cTnI [ 19 ]. The patients admitted to ICU or having severe/fatal illness have several-fold higher likelihood of cTnI elevation. Several recent studies indicated that higher concentration of cTnI and myohaemoglobin were associated with the severity and case fatality rate of COVID-19 [ 20 – 22 ]. Chen et al . reported that elevated cTnI (OR = 26.909, 95%CI: 4.086–177.226, P = 0.001) were the independent risk factors of critical disease status [ 20 ]. Han et al ., found there were statistically significant differences in the level and positive rate of cTnI and myohaemoglobin among the mild, severe and critical COVID-19 case groups [ 23 ]. Our results are in agreement with the previous studies that the elevated myocardial injury markers such as cTnI and myohaemoglobin are independent determinants of illness severity in COVID-19 patients representing negative clinical course and potentially life-threatening prognosis. In particular, cTnI is the strongest predictor of worse severity with OR being 7.464 (95% CI: 4.292–12.980). Direct myocardial injury due to viral myocarditis or the effect of systemic inflammation appears to be the most common mechanisms of acute cardiac injury. Our study also found that interval between illness onset and diagnosis and interval between illness onset and admission were independently associated with illness severity. The risk of developing worse illness severity increased by 1.056-fold and 1.048-fold for each day delay of interval between illness onset and diagnosis and interval between illness onset and admission, respectively. Chen et al . [ 24 ] found the median time from symptom onset to admission was 10 days among deceased COVID-19 patients, one day longer than that of discharged patients. Considering the limited health resources and high case volume in some regions currently, adjusted tactics and strategies should be taken to maximise the availability of and accessibility to medical service to shorten the diagnosis delay or admission delay.
Our study had several limitations. First, this is a retrospective study design, which could be subject to recall bias and selection bias. Second, not all laboratory parameters were tested in all patients, including LDH and D-dimer. Although imputation technique was used to replace missing values, their role might be underestimated in predicting illness severity. Third, due to massive loss of chest CT results, the predicting role of chest CT abnormalities could not be evaluated in this study. Its role of predicting critical illness of COVID-19 has been demonstrated by other studies. Last but not least, generalisability of our findings might be limited by the sample size, and the results need to be validated based on a much larger patient population.
In this study, we identified older age, presence of hypertension, elevated ALT, cTnI and myohaemoglobin, prolonged interval between illness onset and diagnosis and admission as the independent determinants to predict the risk of developing more severe illness among COVID-19 patients. Given the ongoing global pandemic of COVID-19, this study will contribute to early identifications of patients with high risk of developing critical illness and optimising the arrangement of health resources.
Acknowledgements
We acknowledge all health-care workers involved in the diagnosis and treatment of patients in four sites and we thank Dr Qiqi Cao and Dr Weixia Li for assistance in the analysis and interpretation of the lung image data.
Author contributions
Dr Jian Li had full access to all the data in the study and takes responsibility for the integrity of the data and the accuracy of the data analysis. Study design: Jian Li; acquisition of data: Kandi Xu, Dexiang Yang, Yun Ling, Kui Liu, Tao Bai; analysis and interpretation of data: Jian LI, Kandi Xu, Min Zhou, Zenghui Chen; funding acquisition: Min Zhou; drafting of the manuscript: Jian Li; statistical analysis: Jian Li, Kandi Xu; critical revision of the manuscript: all authors.
Financial support
This study was funded by grants from the National R&D Program of China, Shanghai Key Discipline for Respiratory Diseases, Shanghai Shenkang Hospital Development Center Clinical Science and Technology Innovation Project and Zhejiang University Special Scientific Research Fund for COVID-19 Prevention and Control.
Data availability statements
Supplementary material, conflict of interests.
We declare no competing interests.

An official website of the United States government
The .gov means it’s official. Federal government websites often end in .gov or .mil. Before sharing sensitive information, make sure you’re on a federal government site.
The site is secure. The https:// ensures that you are connecting to the official website and that any information you provide is encrypted and transmitted securely.
- Publications
- Account settings
- My Bibliography
- Collections
- Citation manager
Save citation to file
Email citation, add to collections.
- Create a new collection
- Add to an existing collection
Add to My Bibliography
Your saved search, create a file for external citation management software, your rss feed.
- Search in PubMed
- Search in NLM Catalog
- Add to Search
Ordinal logistic regression in medical research
Affiliation.
- 1 Department of Metabolic Diseases and Nutrition, Heinrich-Heine-University of Düsseldorf.
- PMID: 9429194
- PMCID: PMC5420958
Medical research workers are making increasing use of logistic regression analysis for binary and ordinal data. The purpose of this paper is to give a non-technical introduction to logistic regression models for ordinal response variables. We address issues such as the global concept and interpretation of logistic models, the model building procedure from a practical point of view, and the assessment of the model adequacy. For illustrative purposes we apply these methods to real data of a study investigating the association between glycosylated haemoglobin and retinopathy. We give some recommendations for the use and assessment of ordinal logistic regression models in medical research.
PubMed Disclaimer
Similar articles
- Risk factors for the development of micro-vascular complications of type 2 diabetes in a single-centre cohort of patients. Teliti M, Cogni G, Sacchi L, Dagliati A, Marini S, Tibollo V, De Cata P, Bellazzi R, Chiovato L. Teliti M, et al. Diab Vasc Dis Res. 2018 Sep;15(5):424-432. doi: 10.1177/1479164118780808. Epub 2018 Jun 18. Diab Vasc Dis Res. 2018. PMID: 29911415
- Diagnostic performance of glycated hemoglobin for diabetic retinopathy in non-diabetic older overweight/obese African-Americans. Okosun IS, Turbow S, McJenkin K, Monique Davis-Smith Y, Seale JP. Okosun IS, et al. Diabetes Res Clin Pract. 2016 Oct;120:124-31. doi: 10.1016/j.diabres.2016.07.021. Epub 2016 Aug 6. Diabetes Res Clin Pract. 2016. PMID: 27544907
- Ordinal logistic regression models: application in quality of life studies. Abreu MN, Siqueira AL, Cardoso CS, Caiaffa WT. Abreu MN, et al. Cad Saude Publica. 2008;24 Suppl 4:s581-91. doi: 10.1590/s0102-311x2008001600010. Cad Saude Publica. 2008. PMID: 18797732 Review.
- Using binary logistic regression models for ordinal data with non-proportional odds. Bender R, Grouven U. Bender R, et al. J Clin Epidemiol. 1998 Oct;51(10):809-16. doi: 10.1016/s0895-4356(98)00066-3. J Clin Epidemiol. 1998. PMID: 9762873
- Development of a clinical prediction model for an ordinal outcome: the World Health Organization Multicentre Study of Clinical Signs and Etiological agents of Pneumonia, Sepsis and Meningitis in Young Infants. WHO/ARI Young Infant Multicentre Study Group. Harrell FE Jr, Margolis PA, Gove S, Mason KE, Mulholland EK, Lehmann D, Muhe L, Gatchalian S, Eichenwald HF. Harrell FE Jr, et al. Stat Med. 1998 Apr 30;17(8):909-44. doi: 10.1002/(sici)1097-0258(19980430)17:8 3.0.co;2-o. Stat Med. 1998. PMID: 9595619 Review.
- Modified rankin scale is a reliable tool for the rapid assessment of stroke severity and predicting disability outcomes. Nimbvikar AA, Panchawagh S, Chavan AP, Ingole JR, Pargaonkar Y, Pai R. Nimbvikar AA, et al. J Family Med Prim Care. 2024 Mar;13(3):1085-1090. doi: 10.4103/jfmpc.jfmpc_1431_23. Epub 2024 Apr 4. J Family Med Prim Care. 2024. PMID: 38736805 Free PMC article.
- Factors affecting motivation for receiving a booster dose of the COVID-19 vaccine among Japanese university students and staff: a cross-sectional questionnaire survey. Uchida S, Uno S, Kondo M, Uwamino Y, Namkoong H, Nishimura T, Misawa K, Kashimura S, Yamato K, Ishizaka T, Nagashima K, Kitagawa Y, Hasegawa N. Uchida S, et al. Sci Rep. 2024 Apr 5;14(1):8009. doi: 10.1038/s41598-024-58603-9. Sci Rep. 2024. PMID: 38580716 Free PMC article.
- Cognitive decline, Aβ pathology, and blood-brain barrier function in aged 5xFAD mice. Nehra G, Promsan S, Yubolphan R, Chumboatong W, Vivithanaporn P, Maloney BJ, Lungkaphin A, Bauer B, Hartz AMS. Nehra G, et al. Fluids Barriers CNS. 2024 Mar 27;21(1):29. doi: 10.1186/s12987-024-00531-x. Fluids Barriers CNS. 2024. PMID: 38532486 Free PMC article.
- Associations between Urinary Concentrations of Polycyclic Aromatic Hydrocarbons and Overactive Bladder in US Adults: Data from the National Health and Nutrition Examination Survey 2005-2016. Pu S, Li Q, Tao Z, Wang S, Meng X, Wang S, Wang Z. Pu S, et al. Urol Int. 2024;108(2):137-145. doi: 10.1159/000536253. Epub 2024 Jan 12. Urol Int. 2024. PMID: 38219726 Free PMC article.
- A feasibility study on using fNIRS brain signals to recognize personal thermal sensation and thermal comfort conditions. M Sharooni P, Maerefat M, Zolfaghari SA, Dadgostar M. M Sharooni P, et al. J Expo Sci Environ Epidemiol. 2023 Oct 25. doi: 10.1038/s41370-023-00609-y. Online ahead of print. J Expo Sci Environ Epidemiol. 2023. PMID: 37880409
Publication types
- Search in MeSH
Related information
- Cited in Books
LinkOut - more resources
Full text sources.
- Europe PubMed Central
- PubMed Central
- Citation Manager
NCBI Literature Resources
MeSH PMC Bookshelf Disclaimer
The PubMed wordmark and PubMed logo are registered trademarks of the U.S. Department of Health and Human Services (HHS). Unauthorized use of these marks is strictly prohibited.
- Open access
- Published: 14 November 2011
Application of ordinal logistic regression analysis in determining risk factors of child malnutrition in Bangladesh
- Sumonkanti Das 1 &
- Rajwanur M Rahman 2
Nutrition Journal volume 10 , Article number: 124 ( 2011 ) Cite this article
58k Accesses
81 Citations
3 Altmetric
Metrics details
The study attempts to develop an ordinal logistic regression (OLR) model to identify the determinants of child malnutrition instead of developing traditional binary logistic regression (BLR) model using the data of Bangladesh Demographic and Health Survey 2004.
Based on weight-for-age anthropometric index (Z-score) child nutrition status is categorized into three groups-severely undernourished (< -3.0), moderately undernourished (-3.0 to -2.01) and nourished (≥-2.0). Since nutrition status is ordinal, an OLR model-proportional odds model (POM) can be developed instead of two separate BLR models to find predictors of both malnutrition and severe malnutrition if the proportional odds assumption satisfies. The assumption is satisfied with low p-value (0.144) due to violation of the assumption for one co-variate. So partial proportional odds model (PPOM) and two BLR models have also been developed to check the applicability of the OLR model. Graphical test has also been adopted for checking the proportional odds assumption.
All the models determine that age of child, birth interval, mothers' education, maternal nutrition, household wealth status, child feeding index, and incidence of fever, ARI & diarrhoea were the significant predictors of child malnutrition; however, results of PPOM were more precise than those of other models.
These findings clearly justify that OLR models (POM and PPOM) are appropriate to find predictors of malnutrition instead of BLR models.
Peer Review reports
Malnutrition is one of the most important causes for improper physical and mental development of children. Child malnutrition still remains a public health problem in developing countries like Bangladesh [ 1 , 2 ]. It is an underlying cause of child morbidity and mortality. Two-thirds of childhood deaths occurred due to malnutrition in Bangladesh [ 3 ]. From Bangladesh Demographic and Health Survey (BDHS) 2007, it is investigated that 43% children are stunted, and 41% are underweight in Bangladesh [ 4 ]. According to WHO, these levels of stunting and underweight are above the threshold of "very high" prevalence [ 5 ]. The level of wasting (17%) also shows that children in Bangladesh were in "serious severity" [ 4 , 5 ]. Using BDHS 2004 data, a study observed that nearly three fifths children were malnourished-either stunted, wasted or underweight [ 6 ]. The identification of factors for child malnutrition is still the interest of many researchers. Various methods are applied to uncover the factors of child malnutrition. Among them logistic regression analysis has got most preference in previous studies [ 7 – 10 ]. In most of the studies, the response variable was considered as binary (nourished and undernourished); consequently the binary logistic regression model was applied in all the cases. However, the nutrition status of a child is usually classified as nourished, moderately malnourished and severely malnourished. When the researchers are interested to find the determinants of malnutrition and severe malnutrition, two separate binary logistic regression (BLR) models are required to develop by grouping the response variable into two categories [ 7 ]. This task is tedious and cumbersome due to estimation and interpretation of more parameters. However, the researcher may consider the response variable as ordinal and may apply ordinal logistic regression model for the same purpose. A few studies have been done using ordinal logistic regression model (OLR) to identify the predictors of child undernutrition [ 11 ]. In many epidemiological and medical studies, OLR model is frequently used when the response variable is ordinal in nature [ 12 – 17 ]. The study has made an effort to identify the predictors of child malnutrition as well as severe malnutrition for under five Bangladeshi children by developing an ordinal logistic regression model.
Ordinal Logistic Regression Model
There are several occasions when the outcome variable is polychotomous. Such outcome variable can be classified into two categories-multinomial and ordinal. While the dependent variable is classified according to their order of magnitude, one cannot use the multinomial logistic regression model. A number of logistic regression models have been developed for analyzing ordinal response variables [ 12 , 18 – 24 ]. Moreover, when there is a need to take several factors into consideration, special multivariate analysis for ordinal data is the natural alternative. There are various approaches, such as the use of mixed models or another class of models, probit for example, but the ordinal logistic regression models have been widely used in most of the previous research works [ 18 , 19 , 25 – 33 ]. There are several ordinal logistic regression models such as proportional odds model (POM), two versions of the partial proportional odds model-without restrictions (PPOM-UR) and with restrictions (PPOM-R), continuous ratio model (CRM), and stereotype model (SM). The most frequently used ordinal logistic regression model in practice is the constrained cumulative logit model called the proportional odds model [ 18 , 33 – 35 ].
The POM is the most widely used in epidemiological and biomedical applications but POM leads to strong assumptions that may lead to incorrect interpretations if the assumptions are violated [ 28 ]. If the data fail to satisfy the proportional odds assumption, a valid solution is fitting a partial proportional odds model [ 36 ]. Another simple and valid approach to analyze the data is to dichotomize the ordinal response variable by means of several cut-off points and use separate binary logistic regression models for each dichotomous response variable [ 37 ]. However, Gameroff suggested that the second procedure should be avoided if possible because of the loss in statistical power and the reduced generality of the analytical solution [ 17 ].
Data and Variables
The study has utilized the nationwide data of BDHS 2004 where completed and plausible anthropometric data were available for 6005 (weighted) children [ 38 ]. Weight-for-age anthropometric index is an excellent overall indicator of a population's nutritional health status. Moreover, weight-for-age is a composite index of weight-for-height and height-for-age [ 4 ]. So the study considered only weight-for-age anthropometric index instead of weight-for-height and height-for-age to measure the children nutrition status. Child nutrition status was categorized into three groups-severely undernourished (< -3.0 Z-score), moderately undernourished (-3.0 to -2.01 Z-score) and nourished (≥-2.0 Z-Score). Thus nutrition status is an ordinal response variable grouped from a continuous variable.
Several socio-economic and demographic characteristics, maternal health and nutritional information, and incidence of child diseases are considered as the independent variables to develop the POM, PPOM, and separate BLR models. Mathematical forms of the models with some indication of application are shown in Table 1 . Age of children, birth interval, mothers' educational status, household wealth status, child feeding status, mothers' antenatal-postnatal care status, incidence of diarrhoea, ARI, and fever are considered as the independent variables in the study. These independent variables were found significant predictors of child undernutrition in several previous studies [ 7 – 10 , 39 – 43 ]. Household wealth status is evaluated from household wealth index which is constructed by NIPORT et al . [ 38 ]. Child feeding status and mothers' antenatal-postnatal care status are evaluated by constructing child feeding index and antenatal-postnatal care index respectively. Both the indices are constructed according to previous studies by Das et al . [ 9 , 10 ]. Construction procedure is not shown in this paper.
The authority of DHS maintains all kinds of ethical standards and procedures for the survey and also takes informed consent from the survey respondents before the data collection. In addition, we have obtained approval from the DHS to use the data through the website of DHS. So no ethical approval is needed for the study from any other institutions.
Model Fitting
Since the response variable "nutrition status" is ordinal in nature (grouped from continuous variable-weight-for-age anthropometric index), at first POM was formed without a careful assessment of the model adequacy. The chi-squared score test for the proportional odds assumption [ 18 , 36 ] was employed to see whether the main model assumption was violated or not. As the score test is often anticonservative (i.e., the resulting P-values are far too small) [ 13 , 24 , 36 ], we use other techniques to investigate the proportional odds assumption. We calculated single score tests for each covariate for checking whether proportional odds assumption is violated [ 24 ]. Graphical method has also been employed for checking the parallel slope assumptions for all co-variables. In addition, separate binary logistic regression analyses have been conducted as a basis for more careful analysis [ 26 ]. We dichotomized the response variable taking account of the ordering by using cumulative probabilities. The response variable is dichotomized as "at least moderate undernutrition" with two categories '0' = no undernutrition & '1' = at least moderate undernutrition and "at least severe undernutrition" with two categories '0' = no undernutrition or moderate undernutrition and '1' = at least severe undernutrition. The overall goodness-of-fit of the separate BLR models was assessed by "Hosmer and Lemeshow test" [ 33 , 44 , 45 ].
Though POM is suitable for analyzing ordinal variables arising from a continuous variable, the proportional odds assumption is satisfied seldom in practice. When this assumption is violated, a legal alternative is to develop a PPOM which allows some co-variables with proportional odds assumption to be modeled, but for the co-variables failed to perform the proportional odds assumption, it is augmented by a coefficient (γ), which is the effect linked with each j-th cumulative logit, adjusted by the other co-variables [ 33 ]. Thus, PPOM releases the constraint of having a common parameter across the response logits for all the predictors considered in the model [ 17 ]. Since both PPOM and separate binary logistic regression approaches are based on cumulative logit, the PPOM is directly comparable with separate BLR models [ 37 ]. In the same way, the formulation of the logit functions in POM and PPOM are identical (i.e. nourish vs. moderately & severely undernourish; nourish and moderately undernourish vs. severely undernourish), so overall fit of these two models are comparable [ 28 ]. So the study compared the results of the separate BLR models with that of the PPOM, and also compared POM with PPOM. The study fitted unrestricted PPOM model. STATA procedure OLOGIT and SPSS procedure PLUM with TPARALLEL option for POM, SPSS procedure LOGISTIC REGRESSION for separate BLR models [ 46 ], STATA procedure GOLOGIT2 with AUTOFIT option for PPOM [ 47 ] were employed in the study. For graphical tests of proportional odds assumption, PROC LOGISTIC procedure of SAS is used to obtain the estimated logits for at least moderate under-nutrition (logit {P[Y≤1]/P[Y = 2]}) and for at least severe undernutrition (logit {P[Y = 0]/P[Y≥1]}). To see the parallel regression assumptions, estimated logits are plotted against all categories of each explanatory variable. SPSS 17.0, STATA 11.1, and SAS 9.2 are utilized for the complex statistical analysis.
The proportion of undernourished children was 48% with 13% severely undernourished in 2004. Though both the levels reduced in 2007 (43% with 12% severely underweight), the levels are still very high [ 4 ]. The prevalence of child malnutrition according to selected background characteristics are shown in Table 2 . The proportion of severely malnourished and moderately malnourished children were found higher among the children aged 12-23 months (18% & 41%), having < 24 months birth interval (13% & 35%), illiterate (17% & 38%) and acutely malnourished mothers (18% & 40%), worst child feeding status (16% & 36%), and experienced with several diseases like diarrhea, ARI, and fever (near about 50% malnourished separately). Moreover, near about three-fourth children lived in poorest households were most vulnerable to malnutrition. All the selected independent variables were significantly associated with the children's malnutrition status (Chi-square statistics and p-values are mentioned in Table 2 ).
To identify the risk factors of child malnutrition, the study fitted POM, separate BLR models, and PPOM. At first competence of the models are described and then the results of the models are interpreted.
Proportional Odds Model
The results of the multiple POM are given in Table 3 . All the considered variables in the POM are found significant. The score test of the proportional odds assumption is found insignificant at 5% level of significance indicating the data satisfy the proportional odds assumption. However, the p-value of the score test is found small (0.144). To confirm the conclusion regarding the assumption of POM, single score tests of the proportional odds assumption for each covariate were conducted. The p-values of the single score tests are shown in the last column of Table 3 . The test results reveal that all the variables except age of children (p-value < 0.005) were found insignificant i.e., satisfy the proportional odds assumption. Without making a final decision we proceed to analyze the data using separate binary logistic regressions for the dichotomized response. Such an analysis is required to assess the correct functional form of the covariates to build models with adequate goodness-of-fit.
Separate Binary Logistic Regressions
Results of two separate binary logistic regression models are shown in Table 4 . Hosmer-Lemeshow test for both the models indicate that both the models have no lack of fit (p-value > 0.58). The regression coefficients and odds ratios in the two separate models for all the categories of each of the covariates are found homogeneous. The age of children which fails to satisfy the proportional odds assumption has the significant influence on both the models. However, significance levels varied for some covariates in the two BLR models. In the first BLR model with response variable "at least moderate undernutrition" all the variables are found significant. On the other hand, mothers' antenatal-postnatal care, incidence of ARI and diarrhea are found insignificant in the other BLR model with response variable "at least severe undernutrition". Thus the covariates show satisfactory result with some differences in significance level. Since these regression models do not consider the restriction of ordinal response and consider more parameters, we proceed to construct PPOM, which represents a joint model of the response categories [ 22 ], a powerful method based upon maximum likelihood procedures for ordinal response [ 23 ].
Partial Proportional Odds Model
The results of default GOLOGIT2 of STATA are similar to the series of binary logistic regressions and can be interpreted in the same way. The main problem with the results of both processes is that they include many more parameters than POM. These methods free all the variables from the parallel-lines constraint, even though the assumption may be violated only by one or a few of them. So the study used AUTOFIT option with GOLOGIT2 to fit partial proportional odds models, where the parallel-lines constraint is relaxed only for those variables where the assumption was not justified and parallel-lines constraint is considered for the other variables which satisfy the assumption [ 46 ]. The results are shown in Table 5 with Wald test of parallel-lines assumption. Global Wald test for the final model indicates that final model does not violate the proportional odds assumption with high p-value: 0.7943. From Table 4 and Table 5 it is clear that only 23 unique β coefficients or odds ratios need to be explained in PPOM compared to the 42 coefficients produced by separate BLR models. Results of PPOM show that all the covariates have significant influence on the response variable in both comparisons. In addition, the deviance (defined as the difference in the likelihood ratios between POM and PPOM) is chi-square = 15.03 (941.55-926.52) with 2 d.f. (23-21), favouring the PPOM as a better fit to the data than POM [ 28 ]. The pseudo R 2 of POM (0.1029) and PPOM (0.1046) also reflect the same result.
Graphical Test of Proportional Odds Assumption
The line diagrams of all the explanatory variables are shown in Figure 1 . The graphical test of proportional odds assumption indicates that the estimated average logits for all categories in the distinct variable are almost parallel in shape except the variable "age of children". The average logits of different categories for the children age did not support the parallel assumption of POM. This picture is also revealed by the individual score test.
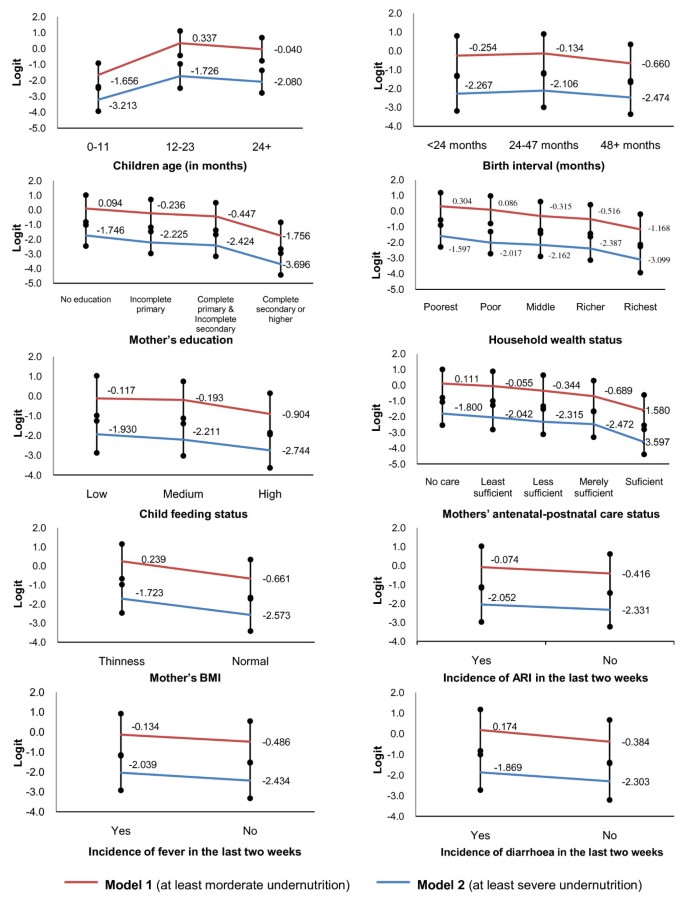
Graphical test for proportional odds assumption . Model 1 indicates the BLR model considering the response variable dichotomized as '0' = no undernutrition & '1' = at least moderate undernutrition. Model 2 indicates the BLR model considering response variable dichotomized as '0' = no undernutrition or moderate undernutrition and '1' = at least severe undernutrition.
Determinants of Child Undernutrition
In POM and PPOM, all the considered variables are found as significant predictors of child malnutrition as in previous studies. The covariates were also found significant in both the separate BLR models except antenatal-postnatal care status, incidence of diarrhoea and ARI in the 2 nd BLR model with the response variable "at least severe undernutrition". These results support the use of POM and PPOM instead of BLR models to determine the predictors of child undernutrition as well as severe undernutrition.
The results of POM reveal that the risk of having worse nutrition status were 6.53 and 5.15 times higher among the children belonging to the age group 12-23 and 24+ months respectively, when compared with the infants (Table 3 ). Since this variable violated the proportional odds assumption, this interpretation may be invalid. However, from separate BLR models and PPOM it is clear that the odds ratios for the children aged 12-23 months and 24 + months compared to infants were about 6.9 and 5.4 respectively when no undernutrition state is compared with moderate and severe undernutrition states (Table 4 & 5 ). When no undernutrition and moderate undernutrition states are compared with severe undernutrition state, the odds ratios were found about 4.2 and 3.3 respectively for children belonging to age group 12-23 and 24 + months compared to infants (Table 4 & 5 ). Since all other covariates did not violate the proportional odds assumption and PPOM performed better than POM as well as separate BLR models, the results for other covariates are described from Table 5 .
Children having birth interval < 24 and 24-47 months had 1.6 and 1.5 times greater risk of having worse nutrition status compared with the children having 48 + months birth interval (Table 5 ). The risk of having worse nutrition status was found highest for the children having mothers with no education (about 3.0 times) when compared with highly educated mothers' children. Compared to the children of the richest households, the chances of having worse nutrition status was found to increase with decrease of household wealth condition (2.03 for the children of poorest household and 1.32 for those of richer household). The risk of having poor nutrition condition was found significantly higher for the children with poor feeding practices compared to those having better feeding practices. Mothers who received no antenatal-postnatal care had 1.62 times greater risk of having malnourished children compared to those received sufficient care. The risk reduced with the increase of mothers' antenatal-postnatal care. Children belonging to acutely malnourished mothers, compared to those of nourished mothers, were 1.74 times (95% CI: 1.54-1.97) as likely to be malnourished moderately or severely. Table 5 also shows that children of acutely malnourished mothers had 1.74 times greater risk of being undernourished compared to those of nourished mothers. Children experienced with ARI, fever, and diarrhoea within last two weeks of the survey had 1.22, 1.27 and 1.28 times higher risk of being undernourished respectively when comparison is made with the children having no such problems (Table 5 ).
At first sight the POM seems to be an appropriate model for analyzing the considered data since the p-value of chi-squared score test for overall model is insignificant at 5% level of significance indicating proportional odds assumption is not violated. All of the considered variables were found significant in the POM. However, the p-value of the score test for overall model was very much small which compels to conduct single score test for each covariate. These tests show that only 'age of children' violates the vital assumption of POM which may lead invalid results. Separate BLR models also indicate the coefficients and the odds ratios for the each age categories varied in the models. Graphical test of proportional odds assumption reveals the same result. In case of all other variables, coefficients and odds ratios are not identical but almost closer. In PPOM, coefficients and odds ratios for the variable 'age of children' are almost same with the result of BLR models. However, the coefficients and odds ratios for other covariates in PPOM are slightly different compared to separate binary logistic regression models, but almost identical with those of POM. Moreover, all the variables are significant in PPOM but in separate binary logistic regression models few are insignificant.
Despite some differences in the results of the fitted models, the results of POM and PPOM are reasonably comparable with those of BLR models. The POM and PPOM have proved adequate for data analysis of child nutritional status, due to the nature of the response variable (grouped continuous variable), in addition, the parsimony and ease of interpretation. Furthermore, PPOM is fitted better for the data than POM. From the results of POM and PPOM it is clear that all the considered variables in the study are significant predictors of child malnutrition as previous research works. Moreover, these findings clearly justify that OLR models (POM & PPOM) are appropriate to find predictors of malnutrition as well as sever undernutrition instead of using two separate binary logistic regression models.
Author's information
SD , Faculty, Department of Statistics, Shahjalal University of Science & Technology (SUST), Sylhet, Bangladesh, obtained his graduation and M.S. in Statistics from the same university. His research and publications focus on the following areas: Time Series Analysis, Stochastic Model Building, Bio-Statistics, Child Health & Nutrition, and Analytic Hierarchy Process. He carried out his M.S. Thesis on Child Health and Nutrition.
RMR is working as Statistical Programmer in Shafi Consultancy Bangladesh, Sylhet, Bangladesh. He completed his graduation and M.S. in Statistics from Department of Statistics, SUST, Sylhet, Bangladesh. His Interested areas of research are Data Management, Bio-statistics, Time Series Modeling, and Child Health & Nutrition.
Abbreviations
Acute Respiratory Infections
Bangladesh Demographic and Health Survey
Binary Logistic Regression
Body Mass Index
Confidence Interval
Continuous Ratio Model
National Institute of Population Research and Training
Ordinal Logistic Regression
Partial Proportional Odds Model-With Restrictions
Partial Proportional Odds Model-Without Restrictions
Stereotype Model
Weight-for-Age Z-score
World Health Organization.
de Onis M, Frongillo EA, Blossner M: Is malnutrition declining? An analysis of changes in levels of child malnutrition since 1980. Bull Pan Am Health Organ. 2000, 78: 1222-1233.
CAS Google Scholar
ACC/SCN: Nutrition throughout the life cycle. Fourth report on the world nutrition situation. 2000, ACC/SCN in collaboration with IFPRI, Geneva, Switzerland
Google Scholar
Pelletier DL, Frongillo EA, Schroeder DG, Habicht JP: The effects of malnutrition on child mortality in developing countries. Bul World Health Org. 1995, 73: 443-448.
National Institute of Population Research and Training (NIPORT, Bangladesh), Mitra and Associates, ORC Macro: Bangladesh Demographic and Health Survey 2007. 2009, Dhaka, Bangladesh, and Calverton, Maryland USA
World Health Organization: Physical status: The use and interpretation of anthropometry. 1995, WHO technical report series 854, World Health Organization, Geneva, Switzerland
Das S, Hossain MZ, Nesa MK: Levels and trends in child malnutrition in Bangladesh. Asia Pacific Population Journal. 2009, 24 (2): 51-78.
Rajaretnam T, Hallad JS: Determinants of nutritional status of young children in India: Analysis of 1992-93 NFHS data. Demography India. 2000, 29 (2): 179-200.
Rayhan MI, Khan MSH: Factors causing malnutrition among under five children in Bangladesh. Pakistan Journal of Nutrition. 2006, 5 (6): 558-562.
Article Google Scholar
Das S, Hossain MZ: Levels and determinants of child undernutrition in Bangladesh. Pakistan Journal of Statistics. 2008, 24 (4): 301-323.
Das S, Hossain MZ, Islam MA: Predictors of child chronic malnutrition in Bangladesh. Proceedings of Pakistan Academy of Science. 2008, 45 (3): 137-155.
Tharakan CT, Suchindran CM: Determinants of child malnutrition: an intervention model for Botswana. Nutr Res. 1999, 19 (6): 843-860. 10.1016/S0271-5317(99)00045-7.
Article CAS Google Scholar
Armstrong BG, Sloan M: Ordinal regression models for epidemiologic data. Am J Epidemiol. 1989, 129: 191-204.
CAS PubMed Google Scholar
Harrell FE, Margolis PA, Gove S, Mason KE, Mulholland EK, Lehmann D, et al: Tutorial in biostatistics: Development of a clinical prediction model for an ordinal outcome: The World Health Organization ARI Multicentre Study of clinical signs and etiological agents of pneumonia, sepsis, and meningitis in young infants. Stat Med. 1998, 17: 909-944. 10.1002/(SICI)1097-0258(19980430)17:8<909::AID-SIM753>3.0.CO;2-O.
Article PubMed Google Scholar
Adeleke KA, Adepoju AA: Ordinal logistic regression model: An application to pregnancy outcomes. J Math & Stat. 2010, 6 (3): 279-285. 10.3844/jmssp.2010.279.285.
Abreu MNS, Siqueira AL, Cardoso CS, Caiaffa WT: Ordinal logistic regression models: application in quality of life studies. Cad Saúde Pública, Rio de Janeiro. 2008, 24 (4): S581-S591.
Haojie Li M, Huiman XB, Aryeh DS, Reynaldo M: Effects of early childhood supplementation on the educational achievement of women. Pediatrics. 2003, 112 (5): 1156-1162. 10.1542/peds.112.5.1156.
Gemeroff MJ: Using the proportional odds model for health-related outcomes: Why, when, and how with various SAS ® procedures. Proceedings of the Thirtieth Annual SAS Users Group International Conference: April 10-13, 2005. 2005, Paper # 205-30.
McCullagh P: Regression models for ordinal data. J R Stat Soc B. 1980, 42: 109-142.
Anderson JA: Regression and ordered categorical variables. J R Stat Soc B. 1984, 46: 1-30.
Agresti A: Tutorial on modeling ordered categorical response data. Psych Bull. 1989, 105: 290-301.
Greenland S: Alternative models for ordinal logistic regression. Stat Med. 1994, 13: 1665-1677. 10.1002/sim.4780131607.
Article CAS PubMed Google Scholar
Cox C: Location-scale cumulative odds models for ordinal data: A generalized non-linear model approach. Stat Med. 1995, 14: 1191-1203. 10.1002/sim.4780141105.
Cox C: Multinomial regression models based upon continuation ratios. Stat Med. 1997, 16: 435-441.
Scott SC, Goldberg MS, Mayo NE: Statistical assessment of ordinal outcomes in comparative studies. J Clin Epidemiol. 1997, 50: 45-55. 10.1016/S0895-4356(96)00312-5.
McCullagh P, Nelder JA: Generalized Linear Models. 1989, New York: Chapman and Hall
Book Google Scholar
Brant R: Assessing proportionality in the proportional odds model for ordinal logistic regression. Biometrics. 1990, 46: 1171-8. 10.2307/2532457.
Lee J: Cumulative logit modeling for ordinal response variables: Application in biochemical research. Compt Appl Biosci. 1992, 8: 555-562.
Ananth CV, Kleinbaum DG: Regression models for ordinal responses: A review of methods and applications. Int J Epidemiol. 1997, 26: 1323-33. 10.1093/ije/26.6.1323.
Bender R, Grouven U: Ordinal logistic regression in medical research. J R Coll Physicians Lond. 1997, 31: 546-51.
Hendrickx J: Special restrictions in multinomial logistic regression. Stata Technical Bulletin. 2000, 56: 18-26.
Walters SJ, Campbell MJ, Lall R: Design and analysis of trials with quality of life as an outcome: A practical guide. J Biopharm Stat. 2001, 11: 155-76. 10.1081/BIP-100107655.
Lall R, Campbell MJ, Walters SJ, Morgan K: A review of ordinal regression models applied on health-related quality of life assessments. Stat Methods Med Res. 2002, 11: 49-67. 10.1191/0962280202sm271ra.
Hosmer DW, Lemeshow S: Applied Logistic Regression. 2000, New York: John Wiley and Sons, 2
Agresti A: An Introduction to Categorical Analysis. 1996, New York: John Wiley and Sons Inc
Pongsapukdee V, Sukgumphaphan S: Goodness of fit of cumulative logit models for ordinal response categories and nominal explanatory variables with two-factor interaction. Silpakorn U Science & Tech J. 2007, 1 (2): 29-38.
Peterson B, Harrell FE: Partial proportional odds models for ordinal response variables. Appl Stat. 1990, 39: 205-217. 10.2307/2347760.
Bender R, Grouven U: Using binary logistic regression models for ordinal data with non-proportional odds. J Clin Epidemiol. 1998, 51 (10): 809-816. 10.1016/S0895-4356(98)00066-3.
National Institute of Population Research and Training (NIPORT, Bangladesh), Mitra and Associates, and ORC Macro: Bangladesh Demographic and Health Survey 2004. 2005, Dhaka, Bangladesh, and Calverton, Maryland USA
Bairagi R, Chowdhury MK: Socio-economic and anthropometric status and mortality of young children in rural Bangladesh. Int J Epid. 1994, 23: 1197-1281.
Ramachandran L: The effect of antenatal and natal services on pregnancy outcome and heath of the mother and child. The Journal of Family Welfare. 1989, 35 (5): 34-46.
Frongillo EA, de Onis M, Hanson KMP: Socioeconomic and demographic factors are associated with worldwide patterns of stunting and wasting of children. J Nutr. 1997, 127: 2302-9.
Dinesh K, Goel NK, Mittal P, Misra P: Influence of infant feeding practices on nutritional status of under-five children. Ind J Pediatrics. 2006, 73 (5): 417-421. 10.1007/BF02758565.
Ruel MT, Menon P: Child feeding practices are associated with child nutritional status in Latin America: Innovative uses of the Demographic and Health Surveys. J Nutr. 2002, 132: 1180-1187.
Hosmer DW, Lemeshow S: Goodness-of-fit tests for the multiple logistic regression model. Comm Stat A. 1980, 9: 1043-1069. 10.1080/03610928008827941.
Hosmer DW, Hosmer T, le Cessie S, Lemeshow S: A comparison of goodness-of-fit tests for the logistic regression modle. Stat Med. 1997, 16: 965-980. 10.1002/(SICI)1097-0258(19970515)16:9<965::AID-SIM509>3.0.CO;2-O.
Liu X: Fitting Proportional Odds Models to Educational Data in Ordinal Logistic Regression Using Stata, SAS and SPSS. Proceedings of Annual Conference of the American Educational Research Association (AERA): April. 2007, 2008, ; Chicago, IL
Williams R: Generalized ordered logit/partial proportional odds models for ordinal dependent variables. The Stata Journal. 2006, 6 (1): 58-82.
Download references
Acknowledgements
The authors wish to thank DHS [ICF Macro] and National Institute of Population Research and Training (NIPORT) [Bangladesh] providing the permission of using the nationwide data of BDHS 2004. Special thanks to the reviewers for their valuable comments and suggestions that enrich the paper.
Author information
Authors and affiliations.
Department of Statistics, Shahjalal University of Science & Technology, Bangladesh
Sumonkanti Das
Shafi Consultancy Bangladesh, Sylhet, Bangladesh
Rajwanur M Rahman
You can also search for this author in PubMed Google Scholar
Corresponding author
Correspondence to Sumonkanti Das .
Additional information
Competing interests.
The authors declare that they have no competing interests.
Authors' contributions
SD determined the study design, performed the statistical analysis, interpreted the data and drafted the typescript. RMR performed the statistical data analysis and critically reviewed the typescript. Both authors reviewed and approved the final version submitted for publication.
Authors’ original submitted files for images
Below are the links to the authors’ original submitted files for images.
Authors’ original file for figure 1
Rights and permissions.
This article is published under license to BioMed Central Ltd. This is an Open Access article distributed under the terms of the Creative Commons Attribution License ( http://creativecommons.org/licenses/by/2.0 ), which permits unrestricted use, distribution, and reproduction in any medium, provided the original work is properly cited.
Reprints and permissions
About this article
Cite this article.
Das, S., Rahman, R.M. Application of ordinal logistic regression analysis in determining risk factors of child malnutrition in Bangladesh. Nutr J 10 , 124 (2011). https://doi.org/10.1186/1475-2891-10-124
Download citation
Received : 23 January 2011
Accepted : 14 November 2011
Published : 14 November 2011
DOI : https://doi.org/10.1186/1475-2891-10-124
Share this article
Anyone you share the following link with will be able to read this content:
Sorry, a shareable link is not currently available for this article.
Provided by the Springer Nature SharedIt content-sharing initiative
- Ordinal logistic regression model
- Proportional odds model
- Partial proportional odds model
- Binary logistic regression model
- Anthropometric index
- Child malnutrition
Nutrition Journal
ISSN: 1475-2891
- General enquiries: [email protected]
Click through the PLOS taxonomy to find articles in your field.
For more information about PLOS Subject Areas, click here .
Loading metrics
Open Access
Correction: Knowledge about hypertension and associated factors among patients with hypertension in public health facilities of Gondar city, Northwest Ethiopia: Ordinal logistic regression analysis
- Maereg Wolde,
- Telake Azale,
- Getu Debalkie Demissie,
- Banchlay Addis
- Maereg Wolde,
- Telake Azale,
- Getu Debalkie Demissie,
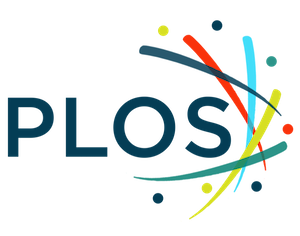
Published: June 6, 2024
- https://doi.org/10.1371/journal.pone.0305376
- Reader Comments
The fourth author’s name is spelled incorrectly. The correct name is: Banchlay Addis. The correct citation is: Wolde M, Azale T, Debalkie Demissie G, Addis B (2022) Knowledge about hypertension and associated factors among patients with hypertension in public health facilities of Gondar city, Northwest Ethiopia: Ordinal logistic regression analysis. PLoS ONE 17(6): e0270030. https://doi.org/10.1371/journal.pone.0270030 .
- View Article
- PubMed/NCBI
- Google Scholar
Citation: Wolde M, Azale T, Demissie GD, Addis B (2024) Correction: Knowledge about hypertension and associated factors among patients with hypertension in public health facilities of Gondar city, Northwest Ethiopia: Ordinal logistic regression analysis. PLoS ONE 19(6): e0305376. https://doi.org/10.1371/journal.pone.0305376
Copyright: © 2024 Wolde et al. This is an open access article distributed under the terms of the Creative Commons Attribution License , which permits unrestricted use, distribution, and reproduction in any medium, provided the original author and source are credited.

IMAGES
VIDEO
COMMENTS
The multinomial logistic regression model is a logistic regression model extended for a nominal outcome variable with more than 2 categories (14, 15). Widely used in traditional epidemiologic ...
Step 1: data preparation. First, the sample data set was imported into R, and the ordinal categorical variables ("Grade" and "Stage") in the data were rewritten as ordered factors using the factor function. The level of the outcome to be used as the baseline was selected and specified using the relevel function.
modeled using ordinal logistic regression, provided that certain assumptions are met. Ordinal logistic regression, unlike polytomous regression, takes into account any inherent ordering of the levels in the disease or outcome variable, thus making fuller use of the ordinal information. The ordinal logistic model that we shall
Abstract. Medical research workers are making increasing use of logistic regression analysis for binary and ordinal data. The purpose of this paper is to give a non-technical introduction to logistic regression models for ordinal response variables. We address issues such as the global concept and interpetation of logistic models, the model ...
Abstract. "In statistics, ordinal regression (also called "ordinal classification") is a type of regression analysis used for predicting an ordinal variable, i.e. a variable whose value exists on ...
Hence, we applied ordinal logistic regression (OLR) by assuming ordered categories of SES as low, medium, and high. Despite the fact that numerous research studies on the use of ordinal logistic regression have been performed throughout the world, there is no study that considers the ordered outcome of SES.
The univariate and multivariate ordinal logistic regression models were fitted to identify the independent predictors of illness severity. The cohort included 400 (66.89%) moderate cases, 85 (14.21%) severe and 113 (18.90%) critical cases, of whom 79 died during hospitalisation as of 28 April.
This scale has a total of 21 items, and the. final score is defined as the mean of these items, varying from 0 to 6. These scores are divided into. three categories in a new ordinal scale ...
Ordinal-level measures are very common in social science research. Researchers often analyze ordinal dependent variables using the proportional odds logistic regression model. However, this ''traditional'' method is one of many different types of logistic regression models available for the analysis of ordered response variables.
In this chapter, we discuss ordinal logistic regression (also known as the ordinal logit, ordered polytomous logit, constrained cumulative logit, proportional odds, parallel regression, or grouped continuous model), a versatile and popular method for modeling relationships between an ordinal DV and multiple IVs (Borooah, 2002; Cohen et al., 2003; DeMaris, 2004; Hoffmann, 2004; Hosmer ...
A non-technical introduction to logistic regression models for ordinal response variables, and issues such as the global concept and interpetation of logistic models, the model building procedure from a practical point of view, and the assessment of the model adequacy are addressed. Medical research workers are making increasing use of logistic regression analysis for binary and ordinal data.
The chapter concerns the most popular ordinal logistic regression, cumulative odds, because it works well with the kinds of questions communication scholars ask, and because SPSS fits this model in its Polytomous Universal Model (PLUM) procedure. It also offers instruction on how to conduct an ordinal logistic regression analysis in SPSS.
An ordinal outcome variable with three or more cate-gories can be modeled with a polytomous model, as discussed in Chapter 9, but can also be modeled using ordinal logistic regression, provided that certain assumptions are met. Ordinal logistic regression, unlike polytomous regres-sion, takes into account any inherent ordering of the
Ordinal logistic regression models are appropriate in many of these situations. This article presents a review of the proportional odds model, partial proportional odds model, continuation ratio model, and stereotype model. The fit, statistical inference, and comparisons between models are illustrated with data from a study on quality of life ...
1Traditionally, the exponent is negated in the Logistic and Generalized Logistic; we break from tradition. But, notice that the difference is only surface-deep. The important proper-ties of the Logistic loss do not change; h(z) is simply the reflection about the y-axis of the traditionally defined Logistic loss.
Features. Logistic Regression Models for Ordinal Response Variables provides applied researchers in the social, educational, and behavioral sciences with an accessible and comprehensive coverage of analyses for ordinal outcomes. The content builds on a review of logistic regression, and extends to details of the cumulative (proportional) odds ...
The ordinal logistic regression model was developed using data on 945 patients, where as the data of another 204 patients was used for temporal validation of the developed model. Out of 945 patients, females were 212 (22.4%). ... In biomedical research, in addition to frequent emergence of ordinal categorical data, ...
The binary logistic regression model estimates the odds of success or experiencing an event for the dichotomous response variable given a set of predictors. The logistic regression model can be defined as (Allison, 1999; Menard, 1995): () 11 2 2 p p ln(Y ) logit [π(x)] πx ln 1 πx αβX βX βX ′ = = − =+ + +…+ (1) In an ordinal ...
The data that support the findings of this study are available from Clinical Research Center, Ruijin Hospital, Shanghai Jiao Tong University School of Medicine. ... Before ordinal logistic regression model was fitted, continuous variables of laboratory findings were transformed into categorical variables according to their reference values ...
Abstract. Medical research workers are making increasing use of logistic regression analysis for binary and ordinal data. The purpose of this paper is to give a non-technical introduction to logistic regression models for ordinal response variables. We address issues such as the global concept and interpretation of logistic models, the model ...
Overview. Ordinal logistic regression is a statistical analysis method that can be used to model the relationship between an ordinal response variable and one or more explanatory variables. An ordinal variable is a categorical variable for which there is a clear ordering of the category levels. The explanatory variables may be either continuous ...
Background The study attempts to develop an ordinal logistic regression (OLR) model to identify the determinants of child malnutrition instead of developing traditional binary logistic regression (BLR) model using the data of Bangladesh Demographic and Health Survey 2004. Methods Based on weight-for-age anthropometric index (Z-score) child nutrition status is categorized into three groups ...
continuous variable. In this research work, final grade of students is categorized, al often treated as categorical in actual research for substantive and practical reasons. 2:1 Logistic Regression Model: To fit a binary logistic regression model, a set of regression coefficients that predict the probability of the outcome of interest are ...
The fourth author's name is spelled incorrectly. The correct name is: Banchlay Addis. The correct citation is: Wolde M, Azale T, Debalkie Demissie G, Addis B (2022) Knowledge about hypertension and associated factors among patients with hypertension in public health facilities of Gondar city, Northwest Ethiopia: Ordinal logistic regression analysis.