

How to Solve the Assignment Problem: A Complete Guide
Table of Contents
Assignment problem is a special type of linear programming problem that deals with assigning a number of resources to an equal number of tasks in the most efficient way. The goal is to minimize the total cost of assignments while ensuring that each task is assigned to only one resource and each resource is assigned to only one task. In this blog, we will discuss the solution of the assignment problem using the Hungarian method, which is a popular algorithm for solving the problem.
Understanding the Assignment Problem
Before we dive into the solution, it is important to understand the problem itself. In the assignment problem, we have a matrix of costs, where each row represents a resource and each column represents a task. The objective is to assign each resource to a task in such a way that the total cost of assignments is minimized. However, there are certain constraints that need to be satisfied – each resource can be assigned to only one task and each task can be assigned to only one resource.
Solving the Assignment Problem
There are various methods for solving the assignment problem, including the Hungarian method, the brute force method, and the auction algorithm. Here, we will focus on the steps involved in solving the assignment problem using the Hungarian method, which is the most commonly used and efficient method.
Step 1: Set up the cost matrix
The first step in solving the assignment problem is to set up the cost matrix, which represents the cost of assigning a task to an agent. The matrix should be square and have the same number of rows and columns as the number of tasks and agents, respectively.
Step 2: Subtract the smallest element from each row and column
To simplify the calculations, we need to reduce the size of the cost matrix by subtracting the smallest element from each row and column. This step is called matrix reduction.
Step 3: Cover all zeros with the minimum number of lines
The next step is to cover all zeros in the matrix with the minimum number of horizontal and vertical lines. This step is called matrix covering.
Step 4: Test for optimality and adjust the matrix
To test for optimality, we need to calculate the minimum number of lines required to cover all zeros in the matrix. If the number of lines equals the number of rows or columns, the solution is optimal. If not, we need to adjust the matrix and repeat steps 3 and 4 until we get an optimal solution.
Step 5: Assign the tasks to the agents
The final step is to assign the tasks to the agents based on the optimal solution obtained in step 4. This will give us the most cost-effective or profit-maximizing assignment.
Solution of the Assignment Problem using the Hungarian Method
The Hungarian method is an algorithm that uses a step-by-step approach to find the optimal assignment. The algorithm consists of the following steps:
- Subtract the smallest entry in each row from all the entries of the row.
- Subtract the smallest entry in each column from all the entries of the column.
- Draw the minimum number of lines to cover all zeros in the matrix. If the number of lines drawn is equal to the number of rows, we have an optimal solution. If not, go to step 4.
- Determine the smallest entry not covered by any line. Subtract it from all uncovered entries and add it to all entries covered by two lines. Go to step 3.
The above steps are repeated until an optimal solution is obtained. The optimal solution will have all zeros covered by the minimum number of lines. The assignments can be made by selecting the rows and columns with a single zero in the final matrix.
Applications of the Assignment Problem
The assignment problem has various applications in different fields, including computer science, economics, logistics, and management. In this section, we will provide some examples of how the assignment problem is used in real-life situations.
Applications in Computer Science
The assignment problem can be used in computer science to allocate resources to different tasks, such as allocating memory to processes or assigning threads to processors.
Applications in Economics
The assignment problem can be used in economics to allocate resources to different agents, such as allocating workers to jobs or assigning projects to contractors.
Applications in Logistics
The assignment problem can be used in logistics to allocate resources to different activities, such as allocating vehicles to routes or assigning warehouses to customers.
Applications in Management
The assignment problem can be used in management to allocate resources to different projects, such as allocating employees to tasks or assigning budgets to departments.
Let’s consider the following scenario: a manager needs to assign three employees to three different tasks. Each employee has different skills, and each task requires specific skills. The manager wants to minimize the total time it takes to complete all the tasks. The skills and the time required for each task are given in the table below:
The assignment problem is to determine which employee should be assigned to which task to minimize the total time required. To solve this problem, we can use the Hungarian method, which we discussed in the previous blog.
Using the Hungarian method, we first subtract the smallest entry in each row from all the entries of the row:
Next, we subtract the smallest entry in each column from all the entries of the column:
We draw the minimum number of lines to cover all the zeros in the matrix, which in this case is three:
Since the number of lines is equal to the number of rows, we have an optimal solution. The assignments can be made by selecting the rows and columns with a single zero in the final matrix. In this case, the optimal assignments are:
- Emp 1 to Task 3
- Emp 2 to Task 2
- Emp 3 to Task 1
This assignment results in a total time of 9 units.
I hope this example helps you better understand the assignment problem and how to solve it using the Hungarian method.
Solving the assignment problem may seem daunting, but with the right approach, it can be a straightforward process. By following the steps outlined in this guide, you can confidently tackle any assignment problem that comes your way.
How useful was this post?
Click on a star to rate it!
Average rating 0 / 5. Vote count: 0
No votes so far! Be the first to rate this post.
We are sorry that this post was not useful for you! 😔
Let us improve this post!
Tell us how we can improve this post?
Operations Research
1 Operations Research-An Overview
- History of O.R.
- Approach, Techniques and Tools
- Phases and Processes of O.R. Study
- Typical Applications of O.R
- Limitations of Operations Research
- Models in Operations Research
- O.R. in real world
2 Linear Programming: Formulation and Graphical Method
- General formulation of Linear Programming Problem
- Optimisation Models
- Basics of Graphic Method
- Important steps to draw graph
- Multiple, Unbounded Solution and Infeasible Problems
- Solving Linear Programming Graphically Using Computer
- Application of Linear Programming in Business and Industry
3 Linear Programming-Simplex Method
- Principle of Simplex Method
- Computational aspect of Simplex Method
- Simplex Method with several Decision Variables
- Two Phase and M-method
- Multiple Solution, Unbounded Solution and Infeasible Problem
- Sensitivity Analysis
- Dual Linear Programming Problem
4 Transportation Problem
- Basic Feasible Solution of a Transportation Problem
- Modified Distribution Method
- Stepping Stone Method
- Unbalanced Transportation Problem
- Degenerate Transportation Problem
- Transhipment Problem
- Maximisation in a Transportation Problem
5 Assignment Problem
- Solution of the Assignment Problem
- Unbalanced Assignment Problem
- Problem with some Infeasible Assignments
- Maximisation in an Assignment Problem
- Crew Assignment Problem
6 Application of Excel Solver to Solve LPP
- Building Excel model for solving LP: An Illustrative Example
7 Goal Programming
- Concepts of goal programming
- Goal programming model formulation
- Graphical method of goal programming
- The simplex method of goal programming
- Using Excel Solver to Solve Goal Programming Models
- Application areas of goal programming
8 Integer Programming
- Some Integer Programming Formulation Techniques
- Binary Representation of General Integer Variables
- Unimodularity
- Cutting Plane Method
- Branch and Bound Method
- Solver Solution
9 Dynamic Programming
- Dynamic Programming Methodology: An Example
- Definitions and Notations
- Dynamic Programming Applications
10 Non-Linear Programming
- Solution of a Non-linear Programming Problem
- Convex and Concave Functions
- Kuhn-Tucker Conditions for Constrained Optimisation
- Quadratic Programming
- Separable Programming
- NLP Models with Solver
11 Introduction to game theory and its Applications
- Important terms in Game Theory
- Saddle points
- Mixed strategies: Games without saddle points
- 2 x n games
- Exploiting an opponent’s mistakes
12 Monte Carlo Simulation
- Reasons for using simulation
- Monte Carlo simulation
- Limitations of simulation
- Steps in the simulation process
- Some practical applications of simulation
- Two typical examples of hand-computed simulation
- Computer simulation
13 Queueing Models
- Characteristics of a queueing model
- Notations and Symbols
- Statistical methods in queueing
- The M/M/I System
- The M/M/C System
- The M/Ek/I System
- Decision problems in queueing
Network flow problem
Author: Aaron Wheeler, Chang Wei, Cagla Deniz Bahadir, Ruobing Shui, Ziqiu Zhang (ChemE 6800 Fall 2020)
- 1 Introduction
- 2.1.1 The Assignment Problem
- 2.1.2 The Transportation Problem
- 2.1.3 The Shortest-Path Problem
- 2.1.4 Maximal Flow Problem
- 2.2.1 Ford–Fulkerson Algorithm
- 3.1 Formulation of the Problem
- 3.2 Solution of the Problem
- 4.1 The Minimum Cost Flow Problem
- 4.2 The Assignment Problem
- 4.3 The Shortest Path Problem
- 5 Conclusion
- 6 References
Introduction
Network flow problems arise in several key instances and applications within society and have become fundamental problems within computer science, operations research, applied mathematics, and engineering. Developments in the approach to tackle these problems resulted in algorithms that became the chief instruments for solving problems related to large-scale systems and industrial logistics. Spurred by early developments in linear programming, the methods for addressing these extensive problems date back several decades and they evolved over time as the use of digital computing became increasingly prevalent in industrial processes. Historically, the first instance of an algorithmic development for the network flow problem came in 1956, with the network simplex method formulated by George Dantzig. [1] A variation of the simplex algorithm that revolutionized linear programming, this method leveraged the combinatorial structure inherent to these types of problems and demonstrated incredibly high accuracy. [2] This method and its variations would go on to define the embodiment of the algorithms and models for the various and distinct network flow problems discussed here.
Theory, Methodology, and Algorithms
The network flow problem can be conceptualized as a directed graph which abides by flow capacity and conservation constraints. The vertices in the graph are classified into origins (source Failed to parse (SVG (MathML can be enabled via browser plugin): Invalid response ("Math extension cannot connect to Restbase.") from server "https://wikimedia.org/api/rest_v1/":): {\displaystyle X} ), destinations (sink Failed to parse (SVG (MathML can be enabled via browser plugin): Invalid response ("Math extension cannot connect to Restbase.") from server "https://wikimedia.org/api/rest_v1/":): {\displaystyle O} ), and intermediate points and are collectively referred to as nodes ( Failed to parse (SVG (MathML can be enabled via browser plugin): Invalid response ("Math extension cannot connect to Restbase.") from server "https://wikimedia.org/api/rest_v1/":): {\displaystyle N} ). These nodes are different from one another such that Failed to parse (SVG (MathML can be enabled via browser plugin): Invalid response ("Math extension cannot connect to Restbase.") from server "https://wikimedia.org/api/rest_v1/":): {\displaystyle N_i \neq X,O,\ldots N_j} . [3] The edges in the directed graph are the directional links between nodes and are referred to as arcs ( Failed to parse (SVG (MathML can be enabled via browser plugin): Invalid response ("Math extension cannot connect to Restbase.") from server "https://wikimedia.org/api/rest_v1/":): {\displaystyle A} ). These arcs are defined with a specific direction Failed to parse (SVG (MathML can be enabled via browser plugin): Invalid response ("Math extension cannot connect to Restbase.") from server "https://wikimedia.org/api/rest_v1/":): {\displaystyle (i, j)} that corresponds to the nodes they are connecting. The arcs Failed to parse (SVG (MathML can be enabled via browser plugin): Invalid response ("Math extension cannot connect to Restbase.") from server "https://wikimedia.org/api/rest_v1/":): {\displaystyle A\subseteq (i,j)} are also defined by a specific flow capacity Failed to parse (SVG (MathML can be enabled via browser plugin): Invalid response ("Math extension cannot connect to Restbase.") from server "https://wikimedia.org/api/rest_v1/":): {\displaystyle c(A)>0} that cannot be exceeded. The supply and demand of units Failed to parse (SVG (MathML can be enabled via browser plugin): Invalid response ("Math extension cannot connect to Restbase.") from server "https://wikimedia.org/api/rest_v1/":): {\displaystyle \Sigma_i u_i=0~for~i\in N} are formulated by negative and positive flow notation, and are defined such that sources equate to positive values (supply) and sinks equate to negative values (demand). Intermediate nodes have no net supply or demand. Figure 1 illustrates this general definition of the network.
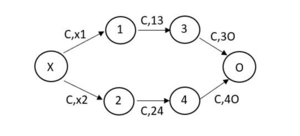
Additional constraints of the network flow optimization model place limits on the solution and vary significantly based on the specific type of problem being solved. Historically, the classic network flow problems are considered to be the maximum flow problem and the minimum-cost circulation problem, the assignment problem, bipartite matching problem, transportation problem, and the transshipment problem. [2] The approach to these problems become quite specific based upon the problem’s objective function but can be generalized by the following iterative approach: 1. determining the initial basic feasible solution; 2. checking the optimality conditions (i.e. whether the problem is infeasible, unbounded over the feasible region, optimal solution has been found, etc.); and 3. constructing an improved basic feasible solution if the optimal solution has not been determined. [3]
General Applications
The assignment problem.
Various real-life instances of assignment problems exist for optimization, such as assigning a group of people to different tasks, events to halls with different capacities, rewards to a team of contributors, and vacation days to workers. All together, the assignment problem is a bipartite matching problem in the kernel. [3] In a classical setting, two types of objects of equal amount are bijective (i.e. they have one-to-one matching), and this tight constraint ensures a perfect matching. The objective is to minimize the cost or maximize the profit of matching, since different items of two types have distinct affinity.
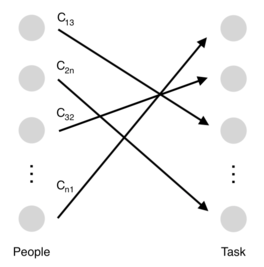
A classic example is as follows: suppose there are Failed to parse (SVG (MathML can be enabled via browser plugin): Invalid response ("Math extension cannot connect to Restbase.") from server "https://wikimedia.org/api/rest_v1/":): {\displaystyle n } people (set Failed to parse (SVG (MathML can be enabled via browser plugin): Invalid response ("Math extension cannot connect to Restbase.") from server "https://wikimedia.org/api/rest_v1/":): {\displaystyle P } ) to be assigned to Failed to parse (SVG (MathML can be enabled via browser plugin): Invalid response ("Math extension cannot connect to Restbase.") from server "https://wikimedia.org/api/rest_v1/":): {\displaystyle n } tasks (set Failed to parse (SVG (MathML can be enabled via browser plugin): Invalid response ("Math extension cannot connect to Restbase.") from server "https://wikimedia.org/api/rest_v1/":): {\displaystyle T } ). Every task has to be completed and each task has to be handled by only one person, and Failed to parse (SVG (MathML can be enabled via browser plugin): Invalid response ("Math extension cannot connect to Restbase.") from server "https://wikimedia.org/api/rest_v1/":): {\displaystyle c_{ij} } , usually given by a table, measures the benefits gained by assigning the person Failed to parse (SVG (MathML can be enabled via browser plugin): Invalid response ("Math extension cannot connect to Restbase.") from server "https://wikimedia.org/api/rest_v1/":): {\displaystyle i } (in Failed to parse (SVG (MathML can be enabled via browser plugin): Invalid response ("Math extension cannot connect to Restbase.") from server "https://wikimedia.org/api/rest_v1/":): {\displaystyle P } ) to the task Failed to parse (SVG (MathML can be enabled via browser plugin): Invalid response ("Math extension cannot connect to Restbase.") from server "https://wikimedia.org/api/rest_v1/":): {\displaystyle j } (in Failed to parse (SVG (MathML can be enabled via browser plugin): Invalid response ("Math extension cannot connect to Restbase.") from server "https://wikimedia.org/api/rest_v1/":): {\displaystyle T } ). [4] The natural objective here is to maximize the overall benefits by devising the optimal assignment pattern. A graph of the general assignment problem and a table of preference are depicted as Figure 2 and Table 2.
Figure 2 can be viewed as a network. The nodes represent people and tasks, and the edges represent potential assignments between a person and a task. Each task can be completed by any person. However, the person that actually ends up being assigned to the task will be the lone individual who is best suited to complete. In the end, the edges with positive flow values will be the only ones represented in the finalized assignment. [5]
To approach this problem, the binary variable Failed to parse (SVG (MathML can be enabled via browser plugin): Invalid response ("Math extension cannot connect to Restbase.") from server "https://wikimedia.org/api/rest_v1/":): {\displaystyle x_{ij} } is defined as whether the person Failed to parse (SVG (MathML can be enabled via browser plugin): Invalid response ("Math extension cannot connect to Restbase.") from server "https://wikimedia.org/api/rest_v1/":): {\displaystyle i } is assigned to the task Failed to parse (SVG (MathML can be enabled via browser plugin): Invalid response ("Math extension cannot connect to Restbase.") from server "https://wikimedia.org/api/rest_v1/":): {\displaystyle j } . If so, Failed to parse (SVG (MathML can be enabled via browser plugin): Invalid response ("Math extension cannot connect to Restbase.") from server "https://wikimedia.org/api/rest_v1/":): {\displaystyle x_{ij} } = 1, and Failed to parse (SVG (MathML can be enabled via browser plugin): Invalid response ("Math extension cannot connect to Restbase.") from server "https://wikimedia.org/api/rest_v1/":): {\displaystyle x_{ij} } = 0 otherwise.
The concise-form formulation of the problem is as follows [3] :
max Failed to parse (SVG (MathML can be enabled via browser plugin): Invalid response ("Math extension cannot connect to Restbase.") from server "https://wikimedia.org/api/rest_v1/":): {\displaystyle z=\sum_{i=1}^n\sum_{j=1}^n c_{ij}x_{ij}}
Subject to:
Failed to parse (SVG (MathML can be enabled via browser plugin): Invalid response ("Math extension cannot connect to Restbase.") from server "https://wikimedia.org/api/rest_v1/":): {\displaystyle \sum_{j=1}^n x_{ij}=1~~\forall i\in [1,n] }
Failed to parse (SVG (MathML can be enabled via browser plugin): Invalid response ("Math extension cannot connect to Restbase.") from server "https://wikimedia.org/api/rest_v1/":): {\displaystyle \sum_{I=1}^n x_{ij}=1~~\forall j\in [1,n] }
Failed to parse (SVG (MathML can be enabled via browser plugin): Invalid response ("Math extension cannot connect to Restbase.") from server "https://wikimedia.org/api/rest_v1/":): {\displaystyle x_{ij}=0~or~1~~\forall i,j\in [1,n] }
The first constraint captures the requirement of assigning each person to a single task. The second constraint indicates that each task must be done by exactly one person. The objective function sums up the overall benefits of all assignments.
To see the analogy between the assignment problem and the network flow, we can describe each person supplying a flow of 1 unit and each task demanding a flow of 1 unit, with the benefits over all “channels” being maximized. [3]
A potential issue lies in the branching of the network, specifically an instance where a person splits its one unit of flow into multiple tasks and the objective remains maximized. This shortcoming is allowed by the laws that govern the network flow model, but are unfeasible in real-life instances. Fortunately, since the network simplex method only involves addition and subtraction of a single edge while transferring the basis, which is served by the spanning tree of the flow graph, if the supply (the number of people here) and the demand (the number of tasks here) in the constraints are integers, the solved variables will be automatically integers even if it is not explicitly stated in the problem. This is called the integrality of the network problem, and it certainly applies to the assignment problem. [6]
The Transportation Problem
People first came up with the transportation problem when distributing troops during World War II. [7] Now, it has become a useful model for solving logistics problems, and the objective is usually to minimize the cost of transportation.
Consider the following scenario:
There are 2 chemical plants located in 2 different places: Failed to parse (SVG (MathML can be enabled via browser plugin): Invalid response ("Math extension cannot connect to Restbase.") from server "https://wikimedia.org/api/rest_v1/":): {\displaystyle M } and Failed to parse (SVG (MathML can be enabled via browser plugin): Invalid response ("Math extension cannot connect to Restbase.") from server "https://wikimedia.org/api/rest_v1/":): {\displaystyle N } . There are 3 raw material suppliers in other 3 locations: Failed to parse (SVG (MathML can be enabled via browser plugin): Invalid response ("Math extension cannot connect to Restbase.") from server "https://wikimedia.org/api/rest_v1/":): {\displaystyle F } , Failed to parse (SVG (MathML can be enabled via browser plugin): Invalid response ("Math extension cannot connect to Restbase.") from server "https://wikimedia.org/api/rest_v1/":): {\displaystyle G } , and Failed to parse (SVG (MathML can be enabled via browser plugin): Invalid response ("Math extension cannot connect to Restbase.") from server "https://wikimedia.org/api/rest_v1/":): {\displaystyle H } . The amount of materials from a supplier can be arbitrarily divided into two parts and shipped to two factories. Supplier Failed to parse (SVG (MathML can be enabled via browser plugin): Invalid response ("Math extension cannot connect to Restbase.") from server "https://wikimedia.org/api/rest_v1/":): {\displaystyle F } , Failed to parse (SVG (MathML can be enabled via browser plugin): Invalid response ("Math extension cannot connect to Restbase.") from server "https://wikimedia.org/api/rest_v1/":): {\displaystyle G } , and Failed to parse (SVG (MathML can be enabled via browser plugin): Invalid response ("Math extension cannot connect to Restbase.") from server "https://wikimedia.org/api/rest_v1/":): {\displaystyle H } can provide Failed to parse (SVG (MathML can be enabled via browser plugin): Invalid response ("Math extension cannot connect to Restbase.") from server "https://wikimedia.org/api/rest_v1/":): {\displaystyle S_1 } , Failed to parse (SVG (MathML can be enabled via browser plugin): Invalid response ("Math extension cannot connect to Restbase.") from server "https://wikimedia.org/api/rest_v1/":): {\displaystyle S_2 } , and Failed to parse (SVG (MathML can be enabled via browser plugin): Invalid response ("Math extension cannot connect to Restbase.") from server "https://wikimedia.org/api/rest_v1/":): {\displaystyle S_3 } amounts of materials respectively. The chemical plants located at Failed to parse (SVG (MathML can be enabled via browser plugin): Invalid response ("Math extension cannot connect to Restbase.") from server "https://wikimedia.org/api/rest_v1/":): {\displaystyle M } and Failed to parse (SVG (MathML can be enabled via browser plugin): Invalid response ("Math extension cannot connect to Restbase.") from server "https://wikimedia.org/api/rest_v1/":): {\displaystyle N } have the material demand of Failed to parse (SVG (MathML can be enabled via browser plugin): Invalid response ("Math extension cannot connect to Restbase.") from server "https://wikimedia.org/api/rest_v1/":): {\displaystyle D_1 } and Failed to parse (SVG (MathML can be enabled via browser plugin): Invalid response ("Math extension cannot connect to Restbase.") from server "https://wikimedia.org/api/rest_v1/":): {\displaystyle D_2 } respectively. Each transportation route, from suppliers to chemical plants, is attributed with a specific cost. This model raises the question: to keep the chemical plants running, what is the best way to arrange the material from the suppliers so that the transportation cost could be minimized?
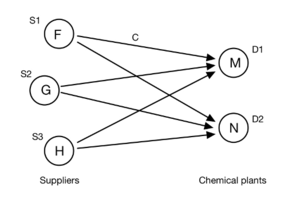
Several quantities should be defined to help formulate the frame of the solution:
Failed to parse (SVG (MathML can be enabled via browser plugin): Invalid response ("Math extension cannot connect to Restbase.") from server "https://wikimedia.org/api/rest_v1/":): {\displaystyle S_{i} } = the amount of material provided at the supplier Failed to parse (SVG (MathML can be enabled via browser plugin): Invalid response ("Math extension cannot connect to Restbase.") from server "https://wikimedia.org/api/rest_v1/":): {\displaystyle i }
Failed to parse (SVG (MathML can be enabled via browser plugin): Invalid response ("Math extension cannot connect to Restbase.") from server "https://wikimedia.org/api/rest_v1/":): {\displaystyle D_{j} } = the amount of material being consumed at the chemical plant Failed to parse (SVG (MathML can be enabled via browser plugin): Invalid response ("Math extension cannot connect to Restbase.") from server "https://wikimedia.org/api/rest_v1/":): {\displaystyle j }
Failed to parse (SVG (MathML can be enabled via browser plugin): Invalid response ("Math extension cannot connect to Restbase.") from server "https://wikimedia.org/api/rest_v1/":): {\displaystyle x_{ij} } = the amount of material being transferred from supplier Failed to parse (SVG (MathML can be enabled via browser plugin): Invalid response ("Math extension cannot connect to Restbase.") from server "https://wikimedia.org/api/rest_v1/":): {\displaystyle i } to chemical plant Failed to parse (SVG (MathML can be enabled via browser plugin): Invalid response ("Math extension cannot connect to Restbase.") from server "https://wikimedia.org/api/rest_v1/":): {\textstyle j }
Failed to parse (SVG (MathML can be enabled via browser plugin): Invalid response ("Math extension cannot connect to Restbase.") from server "https://wikimedia.org/api/rest_v1/":): {\displaystyle C_{ij} } = the cost of transferring 1 unit of material from supplier Failed to parse (SVG (MathML can be enabled via browser plugin): Invalid response ("Math extension cannot connect to Restbase.") from server "https://wikimedia.org/api/rest_v1/":): {\displaystyle i } to chemical plant Failed to parse (SVG (MathML can be enabled via browser plugin): Invalid response ("Math extension cannot connect to Restbase.") from server "https://wikimedia.org/api/rest_v1/":): {\displaystyle j }
Failed to parse (SVG (MathML can be enabled via browser plugin): Invalid response ("Math extension cannot connect to Restbase.") from server "https://wikimedia.org/api/rest_v1/":): {\displaystyle x_{ij} } Failed to parse (SVG (MathML can be enabled via browser plugin): Invalid response ("Math extension cannot connect to Restbase.") from server "https://wikimedia.org/api/rest_v1/":): {\displaystyle C_{ij} } = the cost of the material transportation from Failed to parse (SVG (MathML can be enabled via browser plugin): Invalid response ("Math extension cannot connect to Restbase.") from server "https://wikimedia.org/api/rest_v1/":): {\displaystyle i } to Failed to parse (SVG (MathML can be enabled via browser plugin): Invalid response ("Math extension cannot connect to Restbase.") from server "https://wikimedia.org/api/rest_v1/":): {\displaystyle j }
Here, the amount of material being delivered and being consumed is bound to the supply and demand constraints:
(1): The amount of material shipping from supplier Failed to parse (SVG (MathML can be enabled via browser plugin): Invalid response ("Math extension cannot connect to Restbase.") from server "https://wikimedia.org/api/rest_v1/":): {\displaystyle i } cannot exceed the amount of material available at supplier Failed to parse (SVG (MathML can be enabled via browser plugin): Invalid response ("Math extension cannot connect to Restbase.") from server "https://wikimedia.org/api/rest_v1/":): {\displaystyle i } .
Failed to parse (SVG (MathML can be enabled via browser plugin): Invalid response ("Math extension cannot connect to Restbase.") from server "https://wikimedia.org/api/rest_v1/":): {\displaystyle \sum_j^n x_{ij}\ \leq S_{i} \qquad \forall i\in I=[1,m] }
(2): The amount of material arrived at chemical plant Failed to parse (SVG (MathML can be enabled via browser plugin): Invalid response ("Math extension cannot connect to Restbase.") from server "https://wikimedia.org/api/rest_v1/":): {\displaystyle j } should at least fulfill the demand at chemical plant Failed to parse (SVG (MathML can be enabled via browser plugin): Invalid response ("Math extension cannot connect to Restbase.") from server "https://wikimedia.org/api/rest_v1/":): {\displaystyle j } .
Failed to parse (SVG (MathML can be enabled via browser plugin): Invalid response ("Math extension cannot connect to Restbase.") from server "https://wikimedia.org/api/rest_v1/":): {\displaystyle \sum_i^m x_{ij}\ \geq D_{j} \qquad \forall j\in J=[1,n] }
The objective is to find the minimum cost of transportation, so the cost of each transportation line should be added up, and the total cost should be minimized.
Failed to parse (SVG (MathML can be enabled via browser plugin): Invalid response ("Math extension cannot connect to Restbase.") from server "https://wikimedia.org/api/rest_v1/":): {\displaystyle \sum_i^m \sum_j^n x_{ij}\ C_{ij} }
Using the definitions above, the problem can be formulated as such:
min Failed to parse (SVG (MathML can be enabled via browser plugin): Invalid response ("Math extension cannot connect to Restbase.") from server "https://wikimedia.org/api/rest_v1/":): {\displaystyle \quad z = \sum_i^m \sum_j^n x_{ij}\ C_{ij} }
Failed to parse (SVG (MathML can be enabled via browser plugin): Invalid response ("Math extension cannot connect to Restbase.") from server "https://wikimedia.org/api/rest_v1/":): {\displaystyle s.t. \quad\ \sum_j^n x_{ij}\ \leq S_{i} \qquad \forall i\in I=[1,m] }
However, the problem is not complete at this point because there is no constraint for Failed to parse (SVG (MathML can be enabled via browser plugin): Invalid response ("Math extension cannot connect to Restbase.") from server "https://wikimedia.org/api/rest_v1/":): {\displaystyle x_{ij} } , and that means Failed to parse (SVG (MathML can be enabled via browser plugin): Invalid response ("Math extension cannot connect to Restbase.") from server "https://wikimedia.org/api/rest_v1/":): {\displaystyle x_{ij} } can be any number, even negative. In order for Failed to parse (SVG (MathML can be enabled via browser plugin): Invalid response ("Math extension cannot connect to Restbase.") from server "https://wikimedia.org/api/rest_v1/":): {\displaystyle x_{ij} } to make sense physically, a lower bound of zero is mandatory, which corresponds to the situation where no material was transported from Failed to parse (SVG (MathML can be enabled via browser plugin): Invalid response ("Math extension cannot connect to Restbase.") from server "https://wikimedia.org/api/rest_v1/":): {\displaystyle i } to Failed to parse (SVG (MathML can be enabled via browser plugin): Invalid response ("Math extension cannot connect to Restbase.") from server "https://wikimedia.org/api/rest_v1/":): {\displaystyle j } . Adding the last constraint will complete this formulation as such:
Failed to parse (SVG (MathML can be enabled via browser plugin): Invalid response ("Math extension cannot connect to Restbase.") from server "https://wikimedia.org/api/rest_v1/":): {\displaystyle x_{ij}\ \geq 0 }
The problem and the formulation is adapted from Chapter 8 of the book: Applied Mathematical Programming by Bradley, Hax and Magnanti. [3]
The Shortest-Path Problem
The shortest-path problem can be defined as finding the path that yields the shortest total distance between the origin and the destination. Each possible stop is a node and the paths between these nodes are edges incident to these nodes, where the path distance becomes the weight of the edges. In addition to being the most common and straightforward application for finding the shortest path, this model is also used in various applications depending on the definition of nodes and edges. [3] For example, when each node represents a different object and the edge specifies the cost of replacement, the equipment replacement problem is derived. Moreover, when each node represents a different project and the edge specifies the relative priority, the model becomes a project scheduling problem.
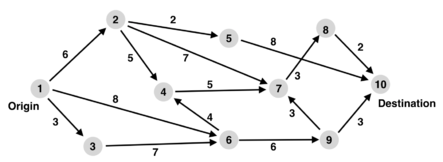
A graph of the general shortest-path problem is depicted as Figure 4:
In the general form of the shortest-path problem, the variable Failed to parse (SVG (MathML can be enabled via browser plugin): Invalid response ("Math extension cannot connect to Restbase.") from server "https://wikimedia.org/api/rest_v1/":): {\displaystyle x_{ij} } represents whether the edge Failed to parse (SVG (MathML can be enabled via browser plugin): Invalid response ("Math extension cannot connect to Restbase.") from server "https://wikimedia.org/api/rest_v1/":): {\displaystyle (i,j) } is active (i.e. with a positive flow), and the parameter Failed to parse (SVG (MathML can be enabled via browser plugin): Invalid response ("Math extension cannot connect to Restbase.") from server "https://wikimedia.org/api/rest_v1/":): {\displaystyle c_{ij} } (e.g. Failed to parse (SVG (MathML can be enabled via browser plugin): Invalid response ("Math extension cannot connect to Restbase.") from server "https://wikimedia.org/api/rest_v1/":): {\displaystyle c_{12} } = 6) defines the distance of the edge Failed to parse (SVG (MathML can be enabled via browser plugin): Invalid response ("Math extension cannot connect to Restbase.") from server "https://wikimedia.org/api/rest_v1/":): {\displaystyle (i,j) } . The general problem is formulated as below:
min Failed to parse (SVG (MathML can be enabled via browser plugin): Invalid response ("Math extension cannot connect to Restbase.") from server "https://wikimedia.org/api/rest_v1/":): {\displaystyle z=\sum_{i=1}^n \sum_{j=1}^n c_{ij}x_{ij}}
Failed to parse (SVG (MathML can be enabled via browser plugin): Invalid response ("Math extension cannot connect to Restbase.") from server "https://wikimedia.org/api/rest_v1/":): {\displaystyle \sum_{j=1}^n x_{ij} - \sum_{k=1}^n x_{ki} = \begin{cases} 1 & \text{if }i=s\text{ (source)} \\ 0 & \text{otherwise} \\ -1 & \text{if }i=t \text{ (sink)} \end{cases}}
Failed to parse (SVG (MathML can be enabled via browser plugin): Invalid response ("Math extension cannot connect to Restbase.") from server "https://wikimedia.org/api/rest_v1/":): {\displaystyle x_{ij}\geq 0~~\forall (i,j)\in E}
The first term of the constraint is the total outflow of the node i, and the second term is the total inflow. So, the formulation above could be seen as one unit of flow being supplied by the origin, one unit of flow being demanded by the destination, and no net inflow or outflow at any intermediate nodes. These constraints mandate a flow of one unit, amounting to the active path, from the origin to the destination. Under this constraint, the objective function minimizes the overall path distance from the origin to the destination.
Similarly, the integrality of the network problem applies here, precluding the unreasonable fractioning. With supply and demand both being integer (one here), the edges can only have integer amount of flow in the result solved by simplex method. [6]
In addition, the point-to-point model above can be further extended to other problems. A number of real life scenarios require visiting multiple places from a single starting point. This “Tree Problem” can be modeled by making small adjustments to the original model. In this case, the source node should supply more units of flow and there will be multiple sink nodes demanding one unit of flow. Overall, the objective and the constraint formulation are similar. [4]
Maximal Flow Problem
This problem describes a situation where the material from a source node is sent to a sink node. The source and sink node are connected through multiple intermediate nodes, and the common optimization goal is to maximize the material sent from the source node to the sink node. [3]
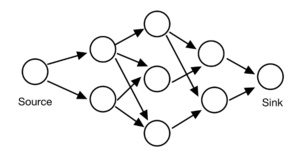
The given structure is a piping system. The water flows into the system from the source node, passing through the intermediate nodes, and flows out from the sink node. There is no limitation on the amount of water that can be used as the input for the source node. Therefore, the sink node can accept an unlimited amount of water coming into it. The arrows denote the valid channel that water can flow through, and each channel has a known flow capacity. What is the maximum flow that the system can take?
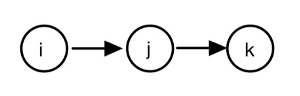
For any intermediate node Failed to parse (SVG (MathML can be enabled via browser plugin): Invalid response ("Math extension cannot connect to Restbase.") from server "https://wikimedia.org/api/rest_v1/":): {\textstyle j } in the system, it receives water from adjacent node(s) Failed to parse (SVG (MathML can be enabled via browser plugin): Invalid response ("Math extension cannot connect to Restbase.") from server "https://wikimedia.org/api/rest_v1/":): {\displaystyle i } , and sends water to the adjacent node(s) Failed to parse (SVG (MathML can be enabled via browser plugin): Invalid response ("Math extension cannot connect to Restbase.") from server "https://wikimedia.org/api/rest_v1/":): {\textstyle k } . The node Failed to parse (SVG (MathML can be enabled via browser plugin): Invalid response ("Math extension cannot connect to Restbase.") from server "https://wikimedia.org/api/rest_v1/":): {\displaystyle i } and k are relative to the node Failed to parse (SVG (MathML can be enabled via browser plugin): Invalid response ("Math extension cannot connect to Restbase.") from server "https://wikimedia.org/api/rest_v1/":): {\textstyle j } .
Failed to parse (SVG (MathML can be enabled via browser plugin): Invalid response ("Math extension cannot connect to Restbase.") from server "https://wikimedia.org/api/rest_v1/":): {\displaystyle i } = the node(s) that gives water to node Failed to parse (SVG (MathML can be enabled via browser plugin): Invalid response ("Math extension cannot connect to Restbase.") from server "https://wikimedia.org/api/rest_v1/":): {\textstyle j }
Failed to parse (SVG (MathML can be enabled via browser plugin): Invalid response ("Math extension cannot connect to Restbase.") from server "https://wikimedia.org/api/rest_v1/":): {\textstyle j } = the intermediate node(s)
Failed to parse (SVG (MathML can be enabled via browser plugin): Invalid response ("Math extension cannot connect to Restbase.") from server "https://wikimedia.org/api/rest_v1/":): {\textstyle k } = the node(s) that receives the water coming out of node Failed to parse (SVG (MathML can be enabled via browser plugin): Invalid response ("Math extension cannot connect to Restbase.") from server "https://wikimedia.org/api/rest_v1/":): {\textstyle j }
Failed to parse (SVG (MathML can be enabled via browser plugin): Invalid response ("Math extension cannot connect to Restbase.") from server "https://wikimedia.org/api/rest_v1/":): {\displaystyle x_{ij} } = amount of water leaving node Failed to parse (SVG (MathML can be enabled via browser plugin): Invalid response ("Math extension cannot connect to Restbase.") from server "https://wikimedia.org/api/rest_v1/":): {\displaystyle i } and entering node Failed to parse (SVG (MathML can be enabled via browser plugin): Invalid response ("Math extension cannot connect to Restbase.") from server "https://wikimedia.org/api/rest_v1/":): {\textstyle j } ( Failed to parse (SVG (MathML can be enabled via browser plugin): Invalid response ("Math extension cannot connect to Restbase.") from server "https://wikimedia.org/api/rest_v1/":): {\displaystyle i } and Failed to parse (SVG (MathML can be enabled via browser plugin): Invalid response ("Math extension cannot connect to Restbase.") from server "https://wikimedia.org/api/rest_v1/":): {\textstyle j } are adjacent nodes)
Failed to parse (SVG (MathML can be enabled via browser plugin): Invalid response ("Math extension cannot connect to Restbase.") from server "https://wikimedia.org/api/rest_v1/":): {\displaystyle x_{jk} } = amount of water leaving node Failed to parse (SVG (MathML can be enabled via browser plugin): Invalid response ("Math extension cannot connect to Restbase.") from server "https://wikimedia.org/api/rest_v1/":): {\textstyle j } and entering node Failed to parse (SVG (MathML can be enabled via browser plugin): Invalid response ("Math extension cannot connect to Restbase.") from server "https://wikimedia.org/api/rest_v1/":): {\textstyle k } ( Failed to parse (SVG (MathML can be enabled via browser plugin): Invalid response ("Math extension cannot connect to Restbase.") from server "https://wikimedia.org/api/rest_v1/":): {\displaystyle i } and Failed to parse (SVG (MathML can be enabled via browser plugin): Invalid response ("Math extension cannot connect to Restbase.") from server "https://wikimedia.org/api/rest_v1/":): {\textstyle k } are adjacent nodes)
For the source and sink node, they have net flow that is non-zero:
Failed to parse (SVG (MathML can be enabled via browser plugin): Invalid response ("Math extension cannot connect to Restbase.") from server "https://wikimedia.org/api/rest_v1/":): {\textstyle m } = source node
Failed to parse (SVG (MathML can be enabled via browser plugin): Invalid response ("Math extension cannot connect to Restbase.") from server "https://wikimedia.org/api/rest_v1/":): {\textstyle n } = sink node
Failed to parse (SVG (MathML can be enabled via browser plugin): Invalid response ("Math extension cannot connect to Restbase.") from server "https://wikimedia.org/api/rest_v1/":): {\displaystyle x_{in} } = amount of water leaving node Failed to parse (SVG (MathML can be enabled via browser plugin): Invalid response ("Math extension cannot connect to Restbase.") from server "https://wikimedia.org/api/rest_v1/":): {\displaystyle i } and entering sink node Failed to parse (SVG (MathML can be enabled via browser plugin): Invalid response ("Math extension cannot connect to Restbase.") from server "https://wikimedia.org/api/rest_v1/":): {\textstyle n } ( Failed to parse (SVG (MathML can be enabled via browser plugin): Invalid response ("Math extension cannot connect to Restbase.") from server "https://wikimedia.org/api/rest_v1/":): {\displaystyle i } and Failed to parse (SVG (MathML can be enabled via browser plugin): Invalid response ("Math extension cannot connect to Restbase.") from server "https://wikimedia.org/api/rest_v1/":): {\textstyle n } are adjacent nodes)
Failed to parse (SVG (MathML can be enabled via browser plugin): Invalid response ("Math extension cannot connect to Restbase.") from server "https://wikimedia.org/api/rest_v1/":): {\displaystyle x_{mk} } = amount of water leaving source node Failed to parse (SVG (MathML can be enabled via browser plugin): Invalid response ("Math extension cannot connect to Restbase.") from server "https://wikimedia.org/api/rest_v1/":): {\textstyle m } and entering node Failed to parse (SVG (MathML can be enabled via browser plugin): Invalid response ("Math extension cannot connect to Restbase.") from server "https://wikimedia.org/api/rest_v1/":): {\textstyle k } ( Failed to parse (SVG (MathML can be enabled via browser plugin): Invalid response ("Math extension cannot connect to Restbase.") from server "https://wikimedia.org/api/rest_v1/":): {\textstyle m } and Failed to parse (SVG (MathML can be enabled via browser plugin): Invalid response ("Math extension cannot connect to Restbase.") from server "https://wikimedia.org/api/rest_v1/":): {\textstyle k } are adjacent nodes)
Flow capacity definition is applied to all nodes (including intermediate nodes, the sink, and the source):
Failed to parse (SVG (MathML can be enabled via browser plugin): Invalid response ("Math extension cannot connect to Restbase.") from server "https://wikimedia.org/api/rest_v1/":): {\displaystyle C_{ab} } = transport capacity between any two nodes Failed to parse (SVG (MathML can be enabled via browser plugin): Invalid response ("Math extension cannot connect to Restbase.") from server "https://wikimedia.org/api/rest_v1/":): {\textstyle a } and Failed to parse (SVG (MathML can be enabled via browser plugin): Invalid response ("Math extension cannot connect to Restbase.") from server "https://wikimedia.org/api/rest_v1/":): {\textstyle b } ( Failed to parse (SVG (MathML can be enabled via browser plugin): Invalid response ("Math extension cannot connect to Restbase.") from server "https://wikimedia.org/api/rest_v1/":): {\textstyle a } Failed to parse (SVG (MathML can be enabled via browser plugin): Invalid response ("Math extension cannot connect to Restbase.") from server "https://wikimedia.org/api/rest_v1/":): {\displaystyle \neq } Failed to parse (SVG (MathML can be enabled via browser plugin): Invalid response ("Math extension cannot connect to Restbase.") from server "https://wikimedia.org/api/rest_v1/":): {\textstyle b } )
The main constraints for this problem are the transport capacity between each node and the material conservation:
(1): The amount of water flowing from any node Failed to parse (SVG (MathML can be enabled via browser plugin): Invalid response ("Math extension cannot connect to Restbase.") from server "https://wikimedia.org/api/rest_v1/":): {\textstyle a } to node Failed to parse (SVG (MathML can be enabled via browser plugin): Invalid response ("Math extension cannot connect to Restbase.") from server "https://wikimedia.org/api/rest_v1/":): {\textstyle b } should not exceed the flow capacity between node Failed to parse (SVG (MathML can be enabled via browser plugin): Invalid response ("Math extension cannot connect to Restbase.") from server "https://wikimedia.org/api/rest_v1/":): {\textstyle a } to node Failed to parse (SVG (MathML can be enabled via browser plugin): Invalid response ("Math extension cannot connect to Restbase.") from server "https://wikimedia.org/api/rest_v1/":): {\textstyle b } .
Failed to parse (SVG (MathML can be enabled via browser plugin): Invalid response ("Math extension cannot connect to Restbase.") from server "https://wikimedia.org/api/rest_v1/":): {\displaystyle 0\leq x_{ab} \leq C_{ab} }
(2): The intermediate node Failed to parse (SVG (MathML can be enabled via browser plugin): Invalid response ("Math extension cannot connect to Restbase.") from server "https://wikimedia.org/api/rest_v1/":): {\textstyle j } does not hold any water, so the amount of water that flows into node Failed to parse (SVG (MathML can be enabled via browser plugin): Invalid response ("Math extension cannot connect to Restbase.") from server "https://wikimedia.org/api/rest_v1/":): {\textstyle j } has to exit the node with the exact same amount it entered with.
Failed to parse (SVG (MathML can be enabled via browser plugin): Invalid response ("Math extension cannot connect to Restbase.") from server "https://wikimedia.org/api/rest_v1/":): {\displaystyle \sum_i^px_{ij}- \sum_k^r x_{jk} =0 \qquad \begin{cases} \forall i\in I=[1,p] \\ \forall j\in J=[1,q]\\ \forall k\in K=[1,r] \end{cases} }
Overall, the net flow out of the source node has to be the same as the net flow into the sink node. This net flow is the amount that should be maximized.
Using the definitions above:
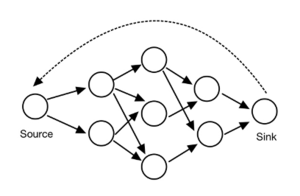
min Failed to parse (SVG (MathML can be enabled via browser plugin): Invalid response ("Math extension cannot connect to Restbase.") from server "https://wikimedia.org/api/rest_v1/":): {\displaystyle \quad z = \sum_k^r x_{uk} } (or Failed to parse (SVG (MathML can be enabled via browser plugin): Invalid response ("Math extension cannot connect to Restbase.") from server "https://wikimedia.org/api/rest_v1/":): {\displaystyle \sum_i^p x_{iv} } )
Failed to parse (SVG (MathML can be enabled via browser plugin): Invalid response ("Math extension cannot connect to Restbase.") from server "https://wikimedia.org/api/rest_v1/":): {\displaystyle s.t. \quad\ \sum_i^px_{ij}- \sum_k^r x_{jk} =0 \qquad \begin{cases} \forall i\in I=[1,p] \\ \forall j\in J=[1,q]\\ \forall k\in K=[1,r] \end{cases} }
This expression can be further simplified by introducing an imaginary flow from the sink to the source.
By introducing this imaginary flow, the piping system is now closed. The mass conservation constraint now also holds for the source and sink node, so they can be treated as the intermediate nodes. The problem can be rewritten as the following:
min Failed to parse (SVG (MathML can be enabled via browser plugin): Invalid response ("Math extension cannot connect to Restbase.") from server "https://wikimedia.org/api/rest_v1/":): {\displaystyle \quad z = x_{vu} }
Failed to parse (SVG (MathML can be enabled via browser plugin): Invalid response ("Math extension cannot connect to Restbase.") from server "https://wikimedia.org/api/rest_v1/":): {\displaystyle s.t. \quad\ \sum_i^px_{ij}- \sum_k^r x_{jk} =0 \qquad \begin{cases} \forall i\in I=[1,p] \\ \forall j\in J=[1,q+2]\\ \forall k\in K=[1,r] \end{cases} }
The problem and the formulation are derived from an example in Chapter 8 of the book: Applied Mathematical Programming by Bradley, Hax and Magnanti. [3]
Ford–Fulkerson Algorithm
A broad range of network flow problems could be reduced to the max-flow problem. The most common way to approach the max-flow problem in polynomial time is the Ford-Fulkerson Algorithm (FFA). FFA is essentially a greedy algorithm and it iteratively finds the augmenting s-t path to increase the value of flow. The pathfinding terminates until there is no s-t path present. Ultimately, the max-flow pattern in the network graph will be returned. [8]
Typically, FFA is applied to flow networks with only one source node s and one sink node t. In addition, the capacity conditions and the conservation conditions, which are two properties defining the flow, must be satisfied. [9] The capacity conditions require that each edge carry a flow that is no more than its capacity, or Failed to parse (SVG (MathML can be enabled via browser plugin): Invalid response ("Math extension cannot connect to Restbase.") from server "https://wikimedia.org/api/rest_v1/":): {\displaystyle 0\leq f(e)\leq c_{e},\forall e\in E} , where function f returns the flow on a certain edge. The conservation conditions require all nodes except the source and the sink to have a net flow of 0, or , Failed to parse (SVG (MathML can be enabled via browser plugin): Invalid response ("Math extension cannot connect to Restbase.") from server "https://wikimedia.org/api/rest_v1/":): {\displaystyle \sum_{e~into~v}f(v)= \sum_{e~out~of~v}f(v),\forall v\in V-{s,t} } .
FFA introduces the concept of residue graph based on the original graph Failed to parse (SVG (MathML can be enabled via browser plugin): Invalid response ("Math extension cannot connect to Restbase.") from server "https://wikimedia.org/api/rest_v1/":): {\displaystyle G} to allow backtracking, or pushing backward on edges that are already carrying flow. [9] The residue graph Failed to parse (SVG (MathML can be enabled via browser plugin): Invalid response ("Math extension cannot connect to Restbase.") from server "https://wikimedia.org/api/rest_v1/":): {\displaystyle G_{f} } is defined as the following:
1. Failed to parse (SVG (MathML can be enabled via browser plugin): Invalid response ("Math extension cannot connect to Restbase.") from server "https://wikimedia.org/api/rest_v1/":): {\displaystyle G_{f}} has exactly the same node set as Failed to parse (SVG (MathML can be enabled via browser plugin): Invalid response ("Math extension cannot connect to Restbase.") from server "https://wikimedia.org/api/rest_v1/":): {\displaystyle G} .
2. For each edge Failed to parse (SVG (MathML can be enabled via browser plugin): Invalid response ("Math extension cannot connect to Restbase.") from server "https://wikimedia.org/api/rest_v1/":): {\displaystyle e = (u,v)} with a nonnegative flow Failed to parse (SVG (MathML can be enabled via browser plugin): Invalid response ("Math extension cannot connect to Restbase.") from server "https://wikimedia.org/api/rest_v1/":): {\displaystyle f( e)} in Failed to parse (SVG (MathML can be enabled via browser plugin): Invalid response ("Math extension cannot connect to Restbase.") from server "https://wikimedia.org/api/rest_v1/":): {\displaystyle G} , Failed to parse (SVG (MathML can be enabled via browser plugin): Invalid response ("Math extension cannot connect to Restbase.") from server "https://wikimedia.org/api/rest_v1/":): {\displaystyle G_{f}} has the edge e with the capacity Failed to parse (SVG (MathML can be enabled via browser plugin): Invalid response ("Math extension cannot connect to Restbase.") from server "https://wikimedia.org/api/rest_v1/":): {\displaystyle c(e)_{f} = c_{e} - f(e)} , and also Failed to parse (SVG (MathML can be enabled via browser plugin): Invalid response ("Math extension cannot connect to Restbase.") from server "https://wikimedia.org/api/rest_v1/":): {\displaystyle G_f} has the edge Failed to parse (SVG (MathML can be enabled via browser plugin): Invalid response ("Math extension cannot connect to Restbase.") from server "https://wikimedia.org/api/rest_v1/":): {\displaystyle e' = (v,u)} with the capacity Failed to parse (SVG (MathML can be enabled via browser plugin): Invalid response ("Math extension cannot connect to Restbase.") from server "https://wikimedia.org/api/rest_v1/":): {\displaystyle c(e')_{f} = f(e)} .
Note that initially, the Failed to parse (SVG (MathML can be enabled via browser plugin): Invalid response ("Math extension cannot connect to Restbase.") from server "https://wikimedia.org/api/rest_v1/":): {\displaystyle G_{f} } is identical to Failed to parse (SVG (MathML can be enabled via browser plugin): Invalid response ("Math extension cannot connect to Restbase.") from server "https://wikimedia.org/api/rest_v1/":): {\displaystyle G} since there is no flow present in Failed to parse (SVG (MathML can be enabled via browser plugin): Invalid response ("Math extension cannot connect to Restbase.") from server "https://wikimedia.org/api/rest_v1/":): {\displaystyle G} .
The steps of FFA are as below. [10] Essentially, the method repeatedly finds a path with positive flow in the residue graph, and updates the flow graph and residue graph until Failed to parse (SVG (MathML can be enabled via browser plugin): Invalid response ("Math extension cannot connect to Restbase.") from server "https://wikimedia.org/api/rest_v1/":): {\displaystyle s} and Failed to parse (SVG (MathML can be enabled via browser plugin): Invalid response ("Math extension cannot connect to Restbase.") from server "https://wikimedia.org/api/rest_v1/":): {\displaystyle t} become disjoint in the residue graph.
1. Set Failed to parse (SVG (MathML can be enabled via browser plugin): Invalid response ("Math extension cannot connect to Restbase.") from server "https://wikimedia.org/api/rest_v1/":): {\displaystyle f(e) = 0, \forall e\in E} in Failed to parse (SVG (MathML can be enabled via browser plugin): Invalid response ("Math extension cannot connect to Restbase.") from server "https://wikimedia.org/api/rest_v1/":): {\displaystyle G} , and create a copy as Failed to parse (SVG (MathML can be enabled via browser plugin): Invalid response ("Math extension cannot connect to Restbase.") from server "https://wikimedia.org/api/rest_v1/":): {\displaystyle G_{f}} .
2. While there is still a Failed to parse (SVG (MathML can be enabled via browser plugin): Invalid response ("Math extension cannot connect to Restbase.") from server "https://wikimedia.org/api/rest_v1/":): {\displaystyle s, t} path Failed to parse (SVG (MathML can be enabled via browser plugin): Invalid response ("Math extension cannot connect to Restbase.") from server "https://wikimedia.org/api/rest_v1/":): {\displaystyle p} in Failed to parse (SVG (MathML can be enabled via browser plugin): Invalid response ("Math extension cannot connect to Restbase.") from server "https://wikimedia.org/api/rest_v1/":): {\displaystyle G_{f}} :
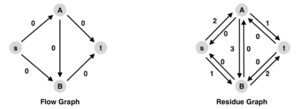
An example of running the FFA is as below. The flow graph Failed to parse (SVG (MathML can be enabled via browser plugin): Invalid response ("Math extension cannot connect to Restbase.") from server "https://wikimedia.org/api/rest_v1/":): {\displaystyle G} and residue graph Failed to parse (SVG (MathML can be enabled via browser plugin): Invalid response ("Math extension cannot connect to Restbase.") from server "https://wikimedia.org/api/rest_v1/":): {\displaystyle G_{f}} at the initial phase is depicted in Figure 8, where the number of each edge in the flow graph is the flow units on the edge, whereas it is the updated edge capacity in the residue graph.
In the residue graph, an Failed to parse (SVG (MathML can be enabled via browser plugin): Invalid response ("Math extension cannot connect to Restbase.") from server "https://wikimedia.org/api/rest_v1/":): {\displaystyle s-t} path can be found in the residue graph tracing the edge Failed to parse (SVG (MathML can be enabled via browser plugin): Invalid response ("Math extension cannot connect to Restbase.") from server "https://wikimedia.org/api/rest_v1/":): {\displaystyle s\rightarrow A\rightarrow B\rightarrow t} with the flow of two units. After augmenting the path on both graphs, the flow graph and the residue graph look like the Figure 9.
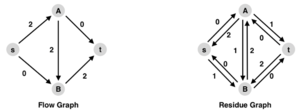
At this stage, there is still Failed to parse (SVG (MathML can be enabled via browser plugin): Invalid response ("Math extension cannot connect to Restbase.") from server "https://wikimedia.org/api/rest_v1/":): {\displaystyle s,t} -path in the residue graph Failed to parse (SVG (MathML can be enabled via browser plugin): Invalid response ("Math extension cannot connect to Restbase.") from server "https://wikimedia.org/api/rest_v1/":): {\displaystyle s\rightarrow B\rightarrow A\rightarrow t} with a flow of one unit. After augmenting the path on both graphs, the flow graph and the residue graph look like the Figure 10.
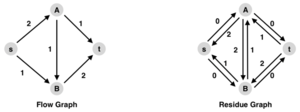
At this stage, there is no more Failed to parse (SVG (MathML can be enabled via browser plugin): Invalid response ("Math extension cannot connect to Restbase.") from server "https://wikimedia.org/api/rest_v1/":): {\displaystyle s,t} -path in the residue graph, so FFA terminates and the maximum flow can be read from the flow graph as 3 units.
Numerical Example and Solution
A Food Distributor Company is farming and collecting vegetables from farmers to later distribute to the grocery stores. The distributor has specific agreements with different third-party companies to mediate the delivery to the grocery stores. In a particular month, the company has 600 ton vegetables to deliver to the grocery store. They have agreements with two third-party transport companies A and B, which have different tariffs for delivering goods between themselves, the distributor, and the grocery store. They also have limits on transport capacity for each path. These delivery points are numbered as shown below, with path 1 being the transport from the Food Distributor Company to the transport company A. The limits and tariffs for each path can be found in the Table 2 below, and the possible transportation connections between the distributor company, the third-party transporters, and the grocery store are shown in the figure below. The distributor companies cannot hold any amount of food, and any incoming food should be delivered to an end point. The distributor company wants to minimize the overall transport cost of shipping 600 tons of vegetables to the grocery store by choosing the optimal path provided by the transport companies. How should the distributor company map out their path and the amount of vegetables carried on each path to minimize cost overall?
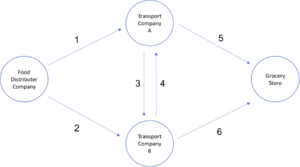
This question is adapted from one of the exercise questions in chapter 8 of the book: Applied Mathematical Programming by Bradley, Hax and Magnanti [3] .
Formulation of the Problem
The problem can be formulated as below where variables Failed to parse (SVG (MathML can be enabled via browser plugin): Invalid response ("Math extension cannot connect to Restbase.") from server "https://wikimedia.org/api/rest_v1/":): {\displaystyle x_1, x_2, x_3,..., x_6} denote the tons of vegetables carried in paths 1 to 6. The objective function stated in the first line is to minimize the cost of the operation, which is the summation of the tons of vegetables carried on each path multiplied by the corresponding tariff: Failed to parse (SVG (MathML can be enabled via browser plugin): Invalid response ("Math extension cannot connect to Restbase.") from server "https://wikimedia.org/api/rest_v1/":): {\displaystyle \sum_{i=1}^6 x_i t_i} .
Failed to parse (SVG (MathML can be enabled via browser plugin): Invalid response ("Math extension cannot connect to Restbase.") from server "https://wikimedia.org/api/rest_v1/":): {\displaystyle \begin{array}{lcl} \min z = 10x_1 + 12.5x_2 + 5x_3 + 7.5x_4 + 10x_5 + 20x_6 \\ s.t. \qquad x_5 = x_1 - x_3 + x_4 \\ \ \ \ \quad \qquad x_6 = x_2 + x_3 - x_4 \\ \ \ \ \quad \qquad x_5 + x_6 = 600 \\ \ \ \ \quad \qquad x_1 + x_2 = 600 \\ \ \ \ \quad \qquad x_1 \leq 250 \\ \ \ \ \quad \qquad x_2 \leq 450 \\ \ \ \ \quad \qquad x_3 \leq 350 \\ \ \ \ \quad \qquad x_4 \leq 200 \\ \ \ \ \quad \qquad x_5 \leq 300 \\ \ \ \ \quad \qquad x_6 \leq 500 \\ \ \ \ \quad \qquad x_1, x_2, x_3, x_4, x_5, x_6 \geq 0\\\end{array} }
The second step is to write down the constraints. The first constraint ensures that the net amount present in the Transport Company A, which is the deliveries received from path 1 and path 2 minus the transport to Transport Company B should be delivered to the grocery store with path 5. The second constraint ensures this for the Transport Company B. The third and fourth constraints are ensuring that the total amount of vegetables shipping from the Food Distributor Company and the total amount of vegetables delivered to the grocery store are both 600 tons. The constraints 5 to 10 depict the upper limits of the amount of vegetables that can be carried on paths 1 to 6. The final constraint depicts that all variables are non-negative.
Solution of the Problem
This problem can be solved using Simplex Algorithm [11] or with the CPLEX Linear Programming solver in GAMS optimization platform. The steps of the solution using the GAMS platform is as follows:
The first step is to list the variables, which are the tons of vegetables that will be transported in routes 1 to 6. The paths can be denoted as Failed to parse (SVG (MathML can be enabled via browser plugin): Invalid response ("Math extension cannot connect to Restbase.") from server "https://wikimedia.org/api/rest_v1/":): {\displaystyle x_1, x_2, x_3,..., x_6} . The objective function is the overall cost: z.
variables x1,x2,x3,x4,x5,x6,z;
The second step is to list the equations which are the constraints and the objective function. The objective function is a summation of the amount of vegetables carried in path i, multiplied with the tariff of path i for all i: Failed to parse (SVG (MathML can be enabled via browser plugin): Invalid response ("Math extension cannot connect to Restbase.") from server "https://wikimedia.org/api/rest_v1/":): {\displaystyle \sum_{i=1}^6 x_i t_i} . The GAMS code for the objective function is written below:
obj.. z=e= 10*x1+12.5*x2+5*x3+7.5*x4+10*x5+20*x6;
Overall, there are 10 constraints in this problem. The constraints 1, and 2 are equations for the paths 5 and 6. The amount carried in path 5 can be found by summing the amount of vegetables incoming to Transport Company A from path 1 and path 4, minus the amount of vegetables leaving Transport Company A with path 3. This can be attributed to the restriction that barrs the companies from keeping any vegetables and requires them to eventually deliver all the incoming produce. The equality 1 ensures that this constraint holds for path 5 and equation 2 ensures it for path 6. A sample of these constraints is written below for path 5:
c1.. x5 =e=x1-x3+x4;
Constraint 3 ensures that the sum of vegetables carried in path 1 and path 2 add to the total of 600 tons of vegetables that leave the Food Distributor Company. Likewise, the constraint 4 ensures that the sum amount of food transported in paths 5 and 6 adds up to 600 tons of vegetables that have to be delivered to the grocery store. A sample of these constraints is written below for the total delivery to the grocery store:
c3.. x5+x6=e=600;
Constraints 5 to 10 should ensure that the amount of food transported in each path should not exceed the maximum capacity depicted in the table. A sample of these constraints is written below for the capacity of path 1:
c5.. x1=l=250;
After listing the variables, objective function and the constraints, the final step is to call the CPLEX solver and set the type of the optimization problem as lp (linear programming). In this case the problem will be solved with a Linear Programming algorithm to minimize the objective (cost) function.
The GAMS code yields the results below:
x1 = 250, x2 = 350, x3 = 0, x4 = 50, x5 = 300, x6 = 300, z =16250.
Real Life Applications
Network problems have many applications in all kinds of areas such as transportation, city design, resource management and financial planning. [6]
There are several special cases of network problems, such as the shortest path problem, minimum cost flow problem, assignment problem and transportation problem. [6] Three application cases will be introduced here.

The Minimum Cost Flow Problem
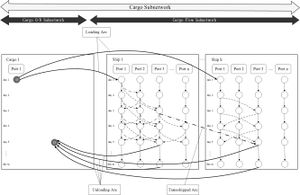
Minimum cost flow problems are pervasive in real life, such as deciding how to allocate temporal quay crane in container terminals, and how to make optimal train schedules on the same railroad line. [12]
R. Dewil and his group use MCNFP to assist traffic enforcement. [13] Police patrol “hot spots”, which are areas where crashes occur frequently on highways. R. Dewil studies a method intended to estimate the optimal route of hot spots. He describes the time it takes to move the detector to a certain position as the cost, and the number of patrol cars from one node to next as the flow, in order to minimize the total cost. [13]
Dung-Ying Lin studies an assignment problem in which he aims to assign freights to ships and arrange transportation paths along the Northern Sea Route in a manner which yields maximum profit. [14] Within this network composed of a ship subnetwork and a cargo subnetwork( shown as Figure 12 and Figure 13), each node corresponds to a port at a specific time and each arc represents the movement of a ship or a cargo. Other types of assignment problems are faculty scheduling, freight assignment, and so on.
The Shortest Path Problem
Shortest path problems are also present in many fields, such as transportation, 5G wireless communication, and implantation of the global dynamic routing scheme. [15][16][17]
Qiang Tu and his group studies the constrained reliable shortest path (CRSP) problem for electric vehicles in the urban transportation network. [15] He describes the reliable travel time of path as the objective item, which is made up of planning travel time of path and the reliability item. The group studies the Chicago sketch network consisting of 933 nodes and 2950 links and the Sioux Falls network consisting of 24 nodes and 76 links. The results show that the travelers’ risk attitudes and properties of electric vehicles in the transportation network can have a great influence on the path choice. [15] The study can contribute to the invention of the city navigation system.
Since its inception, the network flow problem has provided humanity with a straightforward and scalable approach for several large-scale challenges and problems. The Simplex algorithm and other computational optimization platforms have made addressing these problems routine, and have greatly expedited efforts for groups concerned with supply-chain and other distribution processes. The formulation of this problem has had several derivations from its original format, but its overall methodology and approach have remained prevalent in several of society’s industrial and commercial processes, even over half a century later. Classical models such as the assignment, transportation, maximal flow, and shortest path problem configurations have found their way into diverse settings, ranging from streamlining oil distribution networks along the gulf coast to arranging optimal scheduling assignments for college students amidst a global pandemic. All in all, the network flow problem and its monumental impact, have made it a fundamental tool for any group that deals with combinatorial data sets. And with the surge in adoption of data-driven models and applications within virtually all industries, the use of the network flow problem approach will only continue to drive innovation and meet consumer demands for the foreseeable future.
1. Karp, R. M. (2008). George Dantzig’s impact on the theory of computation . Discrete Optimization, 5(2), 174-185.
2. Goldberg, A. V. Tardos, Eva, Tarjan, Robert E. (1989). Network Flow Algorithms, Algorithms and Combinatorics . 9. 101-164.
3. Bradley, S. P. Hax, A. C., & Magnanti, T. L. (1977). Network Models. Applied mathematical programming (p. 259). Reading, MA: Addison-Wesley.
4. Chinneck, J. W. (2006). Practical optimization: a gentle introduction. Systems and Computer Engineering . Carleton University, Ottawa. 11.
5. Roy, B. V. Mason, K.(2005). Formulation and Analysis of Linear Programs, Chapter 5 Network Flows .
6. Vanderbei, R. J. (2020). Linear programming: foundations and extensions (Vol. 285) . Springer Nature.
7. Sobel, J. (2014). Linear Programming Notes VIII: The Transportation Problem .
8. Cormen, Thomas H.; Leiserson, Charles E.; Rivest, Ronald L.; Stein, Clifford (2001). "Section 26.2: The Ford–Fulkerson method". Introduction to Algorithms (Second ed.). MIT Press and McGraw–Hill.
9. Jon Kleinberg; Éva Tardos (2006). "Chapter 7: Network Flow". Algorithm Design. Pearson Education.
10. Ford–Fulkerson algorithm . Retrieved December 05, 2020.
11. Hu, G. (2020, November 19). Simplex algorithm . Retrieved November 22, 2020.
12. Altınel, İ. K., Aras, N., Şuvak, Z., & Taşkın, Z. C. (2019). Minimum cost noncrossing flow problem on layered networks . Discrete Applied Mathematics, 261, 2-21.
13. Dewil, R., Vansteenwegen, P., Cattrysse, D., & Van Oudheusden, D. (2015). A minimum cost network flow model for the maximum covering and patrol routing problem . European Journal of Operational Research, 247(1), 27-36.
14. Lin, D. Y., & Chang, Y. T. (2018). Ship routing and freight assignment problem for liner shipping: Application to the Northern Sea Route planning problem . Transportation Research Part E: Logistics and Transportation Review, 110, 47-70.
15. Tu, Q., Cheng, L., Yuan, T., Cheng, Y., & Li, M. (2020). The Constrained Reliable Shortest Path Problem for Electric Vehicles in the Urban Transportation Network . Journal of Cleaner Production, 121130.
16. Guo, Y., Li, S., Jiang, W., Zhang, B., & Ma, Y. (2017). Learning automata-based algorithms for solving the stochastic shortest path routing problems in 5G wireless communication . Physical Communication, 25, 376-385.
17. Haddou, N. B., Ez-Zahraouy, H., & Rachadi, A. (2016). Implantation of the global dynamic routing scheme in scale-free networks under the shortest path strategy . Physics Letters A, 380(33), 2513-2517.
- Pages with math errors
- Pages with math render errors
Navigation menu
Academia.edu no longer supports Internet Explorer.
To browse Academia.edu and the wider internet faster and more securely, please take a few seconds to upgrade your browser .
Enter the email address you signed up with and we'll email you a reset link.
- We're Hiring!
- Help Center
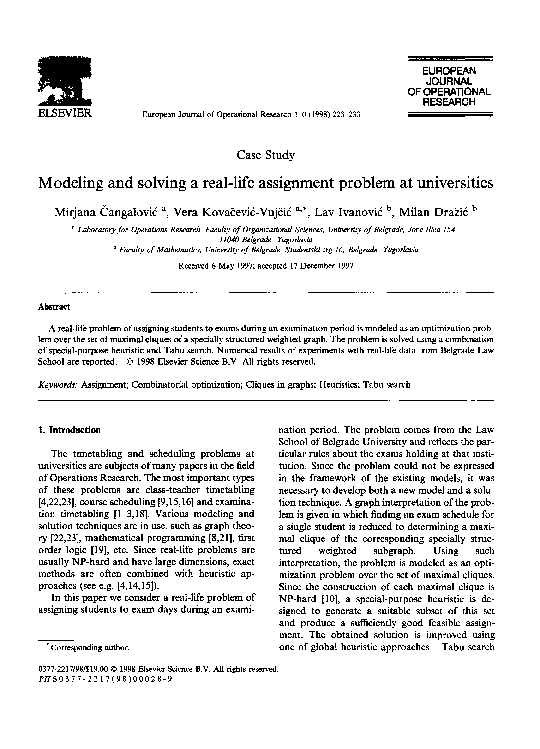
Modeling and solving a real-life assignment problem at universities

1998, European Journal of Operational Research
Related Papers
Journal of Advances in Mathematics and Computer Science
Biralatei Fawei
Combinatorial problems which have been proven to be NP-hard are faced in Higher Education Institutions and researches have extensively investigated some of the well-known combinatorial problems such as the timetabling and student project allocation problems. However, NP-hard problems faced in Higher Education Institutions are not only confined to these categories of combinatorial problems. The majority of NP-hard problems faced in institutions involve grouping students and/or resources, albeit with each problem having its own unique set of constraints. Thus, it can be argued that techniques to solve NP-hard problems in Higher Education Institutions can be transferred across the different problem categories. As no method is guaranteed tooutperform all others in all problems, it is necessary to investigate heuristic techniques for solving lesser-known problems in order to guide stakeholders or software developers to the most appropriate algorithm for each unique class of NP-hard probl...
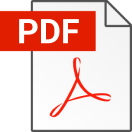
Tanzania Journal of Science
Allen Mushi
This paper reports on the design and implementation of an algorithm for the construction of an examinations timetable. The Examinations Timetabling Problem is the problem of assigning examinations and candidates to time periods and examination rooms while satisfying a set of specific constraints. Every University has a different set of constraints and structure of examinations. Generally, timetabling problems are NP-Hard and therefore very difficult to solve. However, they are of great interest due to their important practical application in educational institutions. This paper discusses a heuristic algorithm basing on the examinations timetabling at the University of Dar es salaam. The algorithm uses a Tabu Search technique, which has been successfully applied to other variations of the problem. Real life instance of the problem has been solved within reasonable time and compares with results of the previous work which used a Simulated Annealing Algorithm. It is concluded that the ...
African Journal of Science and Technology
Practice and Theory of Automated Timetabling …
Luca Di Gaspero
This paper gives a combinatorial approach to solving the student exam scheduling problem. The problem is to generate sched- ules that satisfy hard constraints while minimizing soft constraint voilations. This problem is NP-Hard. The problem is decomposed into stages that include nding stable sets, weighted bipartite match- ings, maximum o w, and pathnding in hypergraphs. We describe our method and
Examination timetabling is an important operational problem in any academic institution. The problem involves assigning examinations and candidates to time periods and examination rooms while satisfying a set of specific constraints. An increased number of student enrollments, a wider variety of courses, and the growing flexibility of students' curricula have contributed to the growing challenge in preparing examination timetables. Since examination timetabling problems differ from one institution to another, in this paper we develop and investigate the impact of a two-phase heuristic that combines Graph-Colouring and Simulated Annealing at Sokoine University of Agriculture (SUA) in Tanzania. Computational results are presented which shows great improvement over the previous work on the same problem.
elrond.informatik.tu-freiberg.de
Manar Hosny
International Journal of Metaheuristics
Meryem Cheraitia
International Journal of Computer Applications
Oluwasefunmi Arogundade
European Journal of Operational Research
RELATED TOPICS
- We're Hiring!
- Help Center
- Find new research papers in:
- Health Sciences
- Earth Sciences
- Cognitive Science
- Mathematics
- Computer Science
- Academia ©2024
Assignment Problem: Meaning, Methods and Variations | Operations Research
After reading this article you will learn about:- 1. Meaning of Assignment Problem 2. Definition of Assignment Problem 3. Mathematical Formulation 4. Hungarian Method 5. Variations.
Meaning of Assignment Problem:
An assignment problem is a particular case of transportation problem where the objective is to assign a number of resources to an equal number of activities so as to minimise total cost or maximize total profit of allocation.
The problem of assignment arises because available resources such as men, machines etc. have varying degrees of efficiency for performing different activities, therefore, cost, profit or loss of performing the different activities is different.
Thus, the problem is “How should the assignments be made so as to optimize the given objective”. Some of the problem where the assignment technique may be useful are assignment of workers to machines, salesman to different sales areas.
Definition of Assignment Problem:
ADVERTISEMENTS:
Suppose there are n jobs to be performed and n persons are available for doing these jobs. Assume that each person can do each job at a term, though with varying degree of efficiency, let c ij be the cost if the i-th person is assigned to the j-th job. The problem is to find an assignment (which job should be assigned to which person one on-one basis) So that the total cost of performing all jobs is minimum, problem of this kind are known as assignment problem.
The assignment problem can be stated in the form of n x n cost matrix C real members as given in the following table:
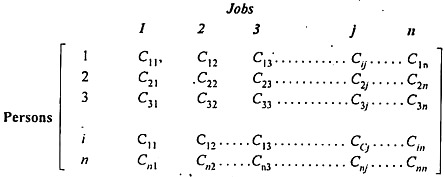
Minimizing energy consumption in a real-life classroom assignment problem
- Original Article
- Published: 07 April 2022
- Volume 44 , pages 1149–1175, ( 2022 )
Cite this article
- Raphael Medeiros Alves 1 ,
- Francisco Cunha 1 ,
- Anand Subramanian ORCID: orcid.org/0000-0002-9244-9969 2 &
- Alisson V. Brito 2
348 Accesses
3 Altmetric
Explore all metrics
This work addresses a classroom assignment problem (CAP) in the context of a large-scale Brazilian federal educational institution. In practice, such problem must be solved at the beginning of every term. Currently, the CAP arising in the referred institution is solved manually, which is not only an arduous task, but also very time-consuming, often leading to inefficient solutions. By analyzing the manual solution from an energetic perspective, one can verify that there are potential losses. For example, it is not desirable to assign classes with few students to rooms with large capacities, which in turn tend to have higher energy costs. The objective of this study is to minimize the energy consumption associated with the usage of the locations where lectures can take place, while meeting the requirements specified by the institution. To solve different versions of the problem, several scenarios were suggested and solved by a mathematical formulation of the problem based on integer linear programming. The model developed was tested on instances involving up to 3046 classes and 97 locations. All of the proposed scenarios were capable of achieving a significant reduction in energy consumption compared to the manual solution, with up to 26% of energy savings.
This is a preview of subscription content, log in via an institution to check access.
Access this article
Price includes VAT (Russian Federation)
Instant access to the full article PDF.
Rent this article via DeepDyve
Institutional subscriptions
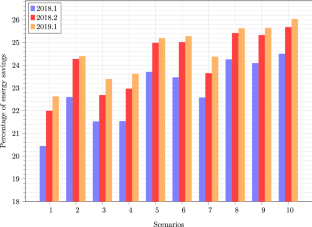
Similar content being viewed by others
An integer programming approach to curriculum-based examination timetabling.
A Course Timetabling Problem for Classroom Usage Minimization
A Mixed Integer Linear Programming Model for School Timetable in Cartagena
Data availability statement.
The datasets used in this research, as well as source codes and detailed results, are publicly available at https://github.com/francisccdn/classroom_assignment_problem .
Al-Yakoob SM, Sherali HD (2007) A mixed-integer programming approach to a class timetabling problem: a case study with gender policies and traffic considerations. Eur J Oper Res 180(3):1028–1044
Article Google Scholar
American Society of Heating R, Engineers AC (1997) ASHRAE Standard: Standards for Natural and Mechanical Ventilation. ASHRAE, Atlanta, GA
Google Scholar
Burke EK, Petrovic S (2002) Recent research directions in automated timetabling. Eur J Oper Res 140(2):266–280
Burke EK, Cowling P, Landa Silva JD, McCollum B (2001) Three methods to automate the space allocation process in UK universities. Lect Notes Comput Sci 2079:254–273
Burke EK, McCollum B, Meisels A, Petrovic S, Qu R (2007) A graph-based hyper-heuristic for educational timetabling problems. Eur J Oper Res 176(1):177–192
Burke EK, Mareček J, Parkes AJ, Rudová H (2010) Decomposition, reformulation, and diving in university course timetabling. Comput Oper Res 37(3):582–597
Carter MW (1989) A lagrangian relaxation approach to the classroom assignment problem. INFOR: Inform Syst Oper Res 27(2):230–246
Carter MW, Tovey CA (1992) When is the classroom assignment problem hard? Oper Res 40(1):S28–S39
Carter MW, Laporte G, Lee SY (1996) Examination timetabling: algorithmic strategies and applications. J Oper Res Soc 47(3):373–383. https://doi.org/10.1057/jors.1996.37
Constantino AA, Marcondes Filho W, Landa-Silva D (2010) Iterated heuristic algorithms for the classroom assignment problem. Proceedings of the 8th international conference on the practice and theory of automated timetabling - PATAT, Belfast 8:152–166
Dammak A, Elloumi A, Kamoun H (2006) Classroom assignment for exam timetabling. Adv Eng Softw 37(10):659–666
Daskalaki S, Birbas T, Housos E (2004) An integer programming formulation for a case study in university timetabling. Eur J Oper Res 153(1):117–135
Elloumi A, Kamoun H, Jarboui B, Dammak A (2014) The classroom assignment problem: complexity, size reduction and heuristics. Appl Soft Compu J 14:677–686
Faudzi S, Abdul-Rahman S, Abd Rahman R (2018) An assignment problem and its application in education domain: a review and potential path. Adv Oper Res 2018:1–19
Lemos A, Melo FS, Monteiro PT, Lynce I (2019) Room usage optimization in timetabling: a case study at universidade de lisboa. Oper Res Perspect 6:100092
Lewis R (2008) A survey of metaheuristic-based techniques for university timetabling problems. OR Spectrum 30(1):167–190
Maffray F, Preissmann M (1996) On the NP-completeness of the k-colorability problem for triangle-free graphs. Discret Math 162(1–3):313–317
Martinez-Alfaro H, Flores-Teran G (1998) Solving the classroom assignment problem with simulated annealing. SMC’98 Conference proceedings 1998 IEEE international conference on systems, man, and cybernetics 4:3703–3708
MirHassani S (2006) A computational approach to enhancing course timetabling with integer programming. Appl Math Comput 175(1):814–822
MirHassani SA, Habibi F (2013) Solution approaches to the course timetabling problem. Artif Intell Rev 39(2):133–149
Mirrazavi SK, Mardle SJ, Tamiz M (2003) A two-phase multiple objective approach to university timetabling utilising optimisation and evolutionary solution methodologies. J Oper Res Soc 54(11):1155–1166
Moran MJ, Shapiro HN, Boettner DD, Bailey MB (2010) Fundamentals of engineering thermodynamics. John Wiley & Sons
Ovalle CT, Torres JRM, Araújo CLQ, Leperqueur AS, Luna MC (2014) University course scheduling and classsroom assignment. Ing Univ Bogotá (Colombia) 18(1):59–75
Phillips AE, Waterer H, Ehrgott M, Ryan DM (2015) Integer programming methods for large-scale practical classroom assignment problems. Comput Oper Res 53:42–53
Schaerf A (1999) A survey of automated deduction. Artif Intell Rev 13:87–127
Sethanan K, Theerakulpisut S, Benjapiyaporn C (2014) Improving energy efficiency by classroom scheduling: a case study in a Thai university. Adv Mater Res 931–932:1089–1095
Song K, Kim S, Park M, Lee HS (2017) Energy efficiency-based course timetabling for university buildings. Energy 139:394–405
Thongsanit K (2014) Solving the course-classroom assignment problem for a University. Silpakorn Uni Sci Technol J 8(1):46–52
Wasfy A, Aloul F (2007) Solving the university class scheduling problem using advanced ILP techniques. IEEE GCC Conference 2007
Download references
Acknowledgements
The authors would like to thank Dr. Monica Carvalho and Dr. Teobaldo Bulhões for their valuable suggestions, as well as the anonymous reviewers for the insightful comments that helped improve the quality of the paper. This research was partially supported by Conselho Nacional de Desenvolvimento Científico e Tecnológico (CNPq), grants 307843/2018-1 and 310205/2018-2.
Author information
Authors and affiliations.
Centro de Informática, Mangabeira, Rua dos Escoteiros s/n, João Pessoa, CEP 58055-000, Brazil
Raphael Medeiros Alves & Francisco Cunha
Departamento de Sistemas de Computação, Centro de Informática, Universidade Federal da Paraíba, Rua dos Escoteiros, Mangabeira, João Pessoa, PB, 58055-000, Brazil
Anand Subramanian & Alisson V. Brito
You can also search for this author in PubMed Google Scholar
Corresponding author
Correspondence to Anand Subramanian .
Additional information
Publisher's note.
Springer Nature remains neutral with regard to jurisdictional claims in published maps and institutional affiliations.
Computing the values of \(s_j\) and \(p_j\) for each location \(j \in L\)
By using the data described in Table 9 , the estimated setup cost \(s_j\) necessary to cool location \(j \in L\) , using an air conditioner (AC), until the desirable comfort temperature is achieved can be approximately calculated according to Equations ( 21 – 23 ) (Moran et al. 2010 ).
The additional cost \(p_j\) necessary to maintain the temperature of any location \(j \in L\) during a lecture of 50 minutes considering the number of people present in j can be approximately calculated according to Equations ( 24 )–( 27 ) (American Society of Heating and Engineers 1997 ; Moran et al. 2010 ).
Gap values over time
The figures presented in this appendix show how the gap values vary over time. Overall, from Figs. 2 , 3 , 4 , 5 , 6 , 7 , 8 , 9 , 10 , and 11 , it can be clearly observed that very small gaps are achieved relatively fast, but proving optimality can be time-consuming in some cases.
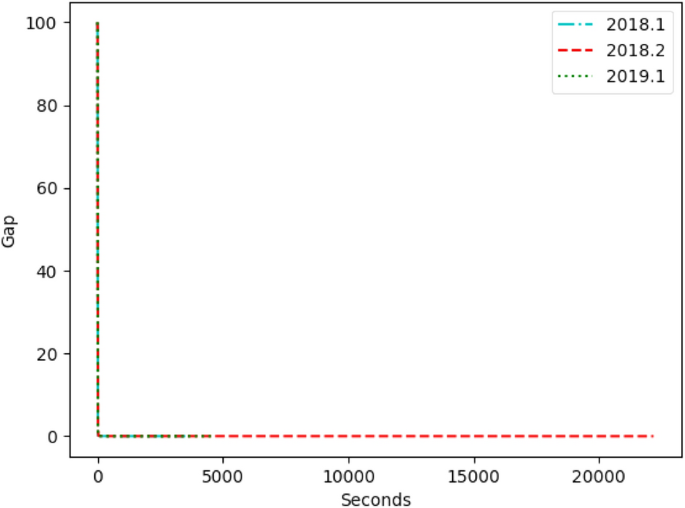
Gap value over time for scenario S1
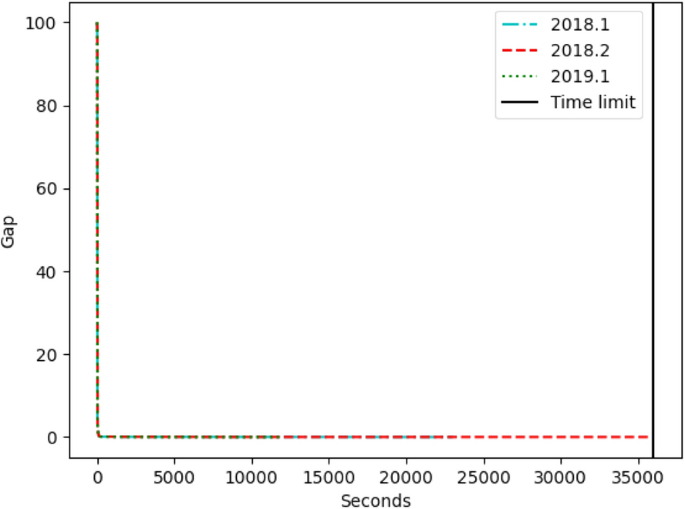
Gap value over time for scenario S2
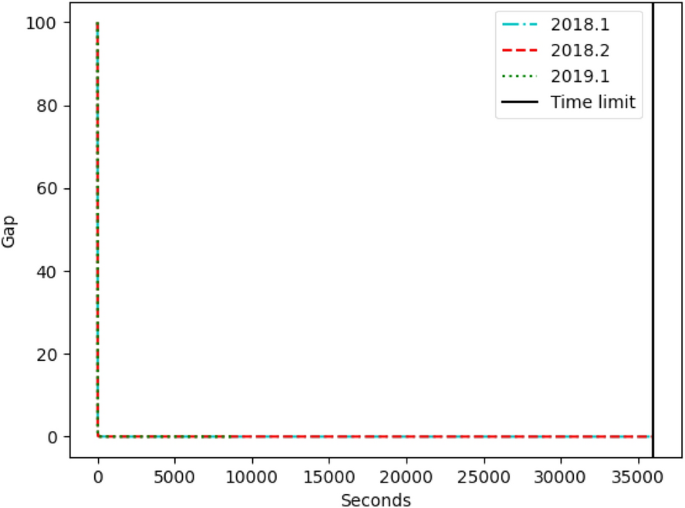
Gap value over time for scenario S3
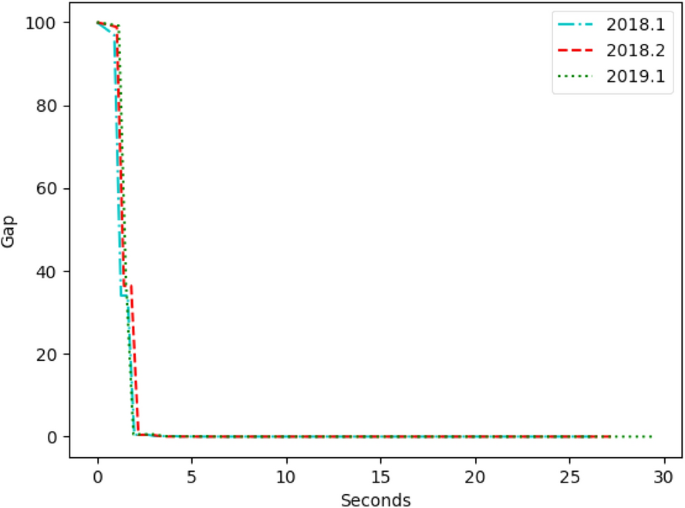
Gap value over time for scenario S4
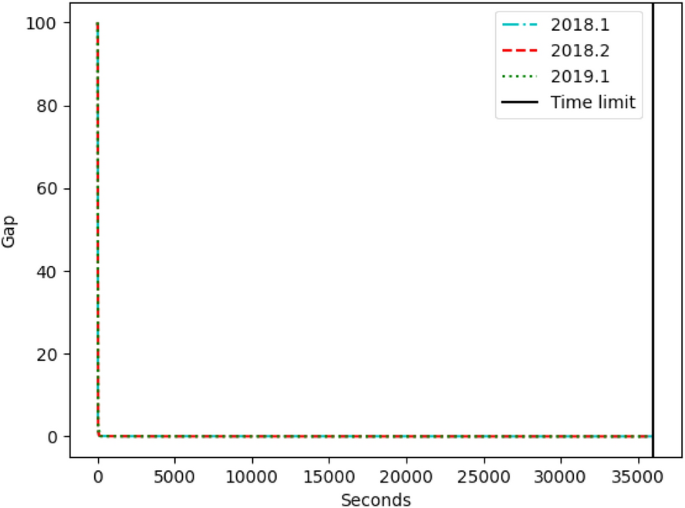
Gap value over time for scenario S5
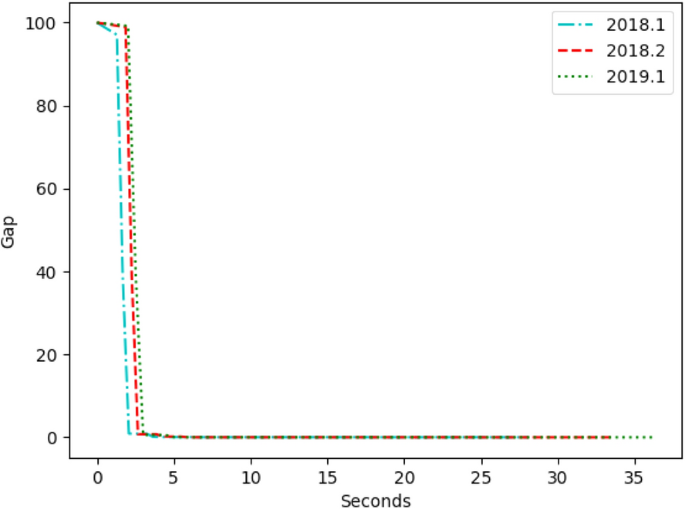
Gap value over time for scenario S6
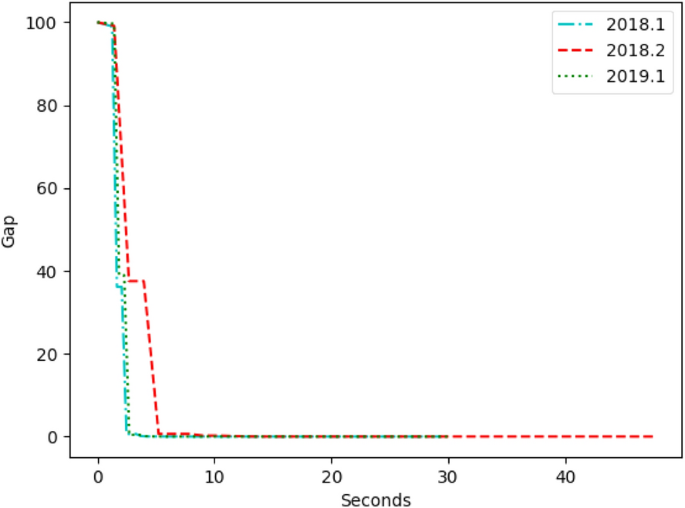
Gap value over time for scenario S7
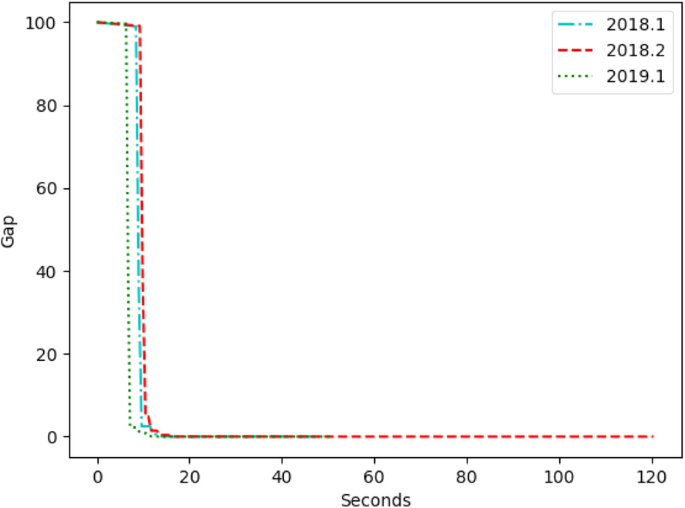
Gap value over time for scenario S8
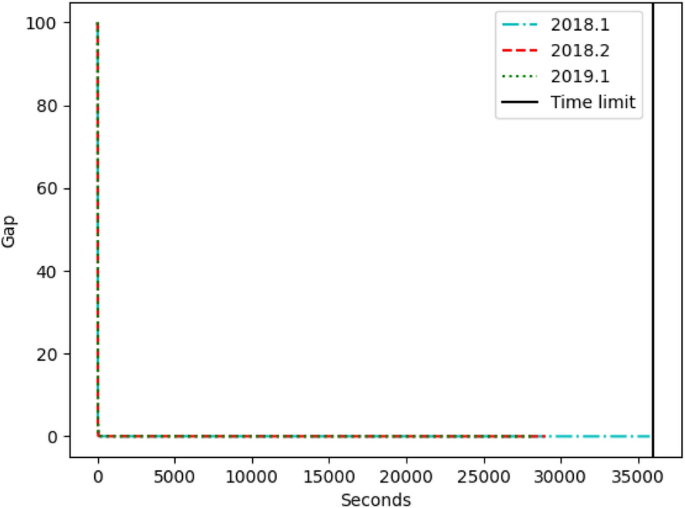
Gap value over time for scenario S9
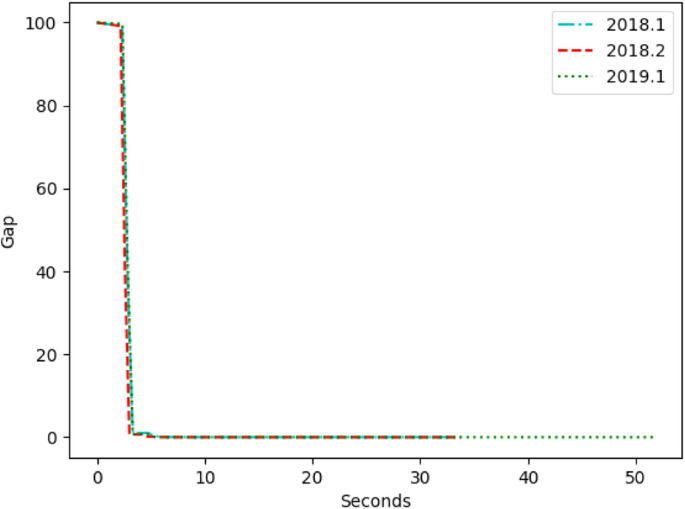
Gap value over time for scenario S10
Rights and permissions
Reprints and permissions
About this article
Alves, R.M., Cunha, F., Subramanian, A. et al. Minimizing energy consumption in a real-life classroom assignment problem. OR Spectrum 44 , 1149–1175 (2022). https://doi.org/10.1007/s00291-022-00674-z
Download citation
Received : 15 September 2020
Accepted : 28 February 2022
Published : 07 April 2022
Issue Date : December 2022
DOI : https://doi.org/10.1007/s00291-022-00674-z
Share this article
Anyone you share the following link with will be able to read this content:
Sorry, a shareable link is not currently available for this article.
Provided by the Springer Nature SharedIt content-sharing initiative
- Energy efficiency
- Optimization
- Integer programming
- Practice of OR
- Find a journal
- Publish with us
- Track your research

IMAGES
VIDEO
COMMENTS
The assignment problem is a combinatorial optimization problem that is flexible as it can be used as an approach to model any real-world problem. In fact, several components in assignment problem have been explored, for example, the constraints and solution methodology used within the education domain.
The assignment problem is a fundamental combinatorial optimization problem. In its most general form, the problem is as follows: The problem instance has a number of agents and a number of tasks. Any agent can be assigned to perform any task, incurring some cost that may vary depending on the agent-task assignment.
Applications of the Assignment Problem. The assignment problem has various applications in different fields, including computer science, economics, logistics, and management. In this section, we will provide some examples of how the assignment problem is used in real-life situations. Applications in Computer Science
The Assignment Problem: An Example A company has 4 machines available for assignment to 4 tasks. Any machine can be assigned to any task, and each task requires processing by one machine. The time required to set up each machine for the processing of each task is given in the table below. TIME (Hours) Task 1 Task 2 Task 3 Task 4 Machine 1 13 4 7 6
The Assignment Problem. Various real-life instances of assignment problems exist for optimization, such as assigning a group of people to different tasks, events to halls with different capacities, rewards to a team of contributors, and vacation days to workers. All together, the assignment problem is a bipartite matching problem in the kernel.
The assignment problem (AP) is incredibly challenging that can model many real-life problems. This paper provides a limited review of the recent developments that have appeared in the literature, meaning of assignment problem as well as solving techniques and will provide a review on a lot of research studies on different types
This paper presents a review pertaining to assignment problem within the education domain, besides looking into the applications of the present research trend, developments, and publications ...
Conclusions A difficult real-life assignment problem at the universities is modeled as a combinatorial optimi- zation problem on the corresponding weighted graph. As the problem has large dimensions, a spe- cial purpose heuristic suplemented by Tabu search is developed in order to solve it. The heuristic was successfully applied to find ...
C o n c l u s i o n s A difficult real-life assignment problem at the universities is modeled as a combinatorial optimization problem on the corresponding weighted graph. As the problem has large dimensions, a special purpose heuristic suplemented by T a b u search is developed in order to solve it. The heuristic was successfully applied to ...
The quadratic assignment problem (QAP) has considered one of the most significant combinatorial optimization problems due to its variant and significant applications in real life such as scheduling, production, computer manufacture, chemistry, facility location, communication, and other fields. QAP is NP-hard problem that is impossible to be solved in polynomial time when the problem size ...
The video covers the description of assignment problem technique, examples of real life assignment problems, characteristics of assignment problems and solvi...
This review summarizes and records a comprehensive survey regarding assignment problem within education domain, which enhances one's understanding concerning the varied types of assignment problems, along with various approaches that serve as solution. This paper presents a review pertaining to assignment problem within the education domain, besides looking into the applications of the ...
A real-life problem of assigning students to exams during an examination period is modeled as an optimization problem over the set of maximal cliques of a specially structured weighted graph. The problem is solved using a combination of special-purpose heuristic and Tabu search. Numerical results of experiments with real-life data from Belgrade ...
Equivalent Assignment Problem c(x, y) 00312 01015 43330 00110 12204 cp(x, y) 3891510 41071614 913111910 813122013 175119 8 13 11 19 13 5 4 3 0 8 9 + 8 - 13 10 Reduced costs. For x # X, y # Y, define cp(x, y) = p(x) + c(x, y) - p(y). Observation 1. Finding a min cost perfect matching with reduced costs
Course scheduling and classroom assignment problem is a common problem for all educational fields. It is an NP Hard problem. ... Güçlükol Ergin, S., Toy, A.Ö. (2023). Course Scheduling Problem and Real-Life Implementation. In: Durakbasa, N.M., Gençyılmaz, M.G. (eds) Towards Industry 5.0. ISPR 2022. Lecture Notes in Mechanical Engineering ...
Exercise 17 shows that the number of iterations is O(n2). To compare the Hungarian method to the exhaustive search method mentioned above, suppose that each iteration can be performed in one second. Then an assignment prob-lem with n = 30 can be solved in at most 302 = 900 seconds, or 15 minutes of computer time.
The problem is to find an assignment (which job should be assigned to which person one on-one basis) So that the total cost of performing all jobs is minimum, problem of this kind are known as assignment problem. The assignment problem can be stated in the form of n x n cost matrix C real members as given in the following table:
Practical Use of Assignment Problem in Real Life Suraj Kumar Sahu Guest Lecture at Model Degree College Nabarangpur, Odisha, India ABSTRACT The assignment problem is a discrete and combinatorial problem where agents are assigned to perform tasks for efficiency maximization or cost (time) minimization. Assignment Problem is a
This work addresses a classroom assignment problem (CAP) in the context of a large-scale Brazilian federal educational institution. In practice, such problem must be solved at the beginning of every term. Currently, the CAP arising in the referred institution is solved manually, which is not only an arduous task, but also very time-consuming, often leading to inefficient solutions. By ...
problem. Depending on the objective we want to optimize, we obtain the typical assignment problems. Assignment problem is an important subject discussed in real physical world we endeavor in this paper to introduce a new approach to assignment problem namely, matrix ones assignment method or MOA -method for solving wide range of problem.
ReviewArticle An Assignment Problem and Its Application in Education Domain: A Review and Potential Path SyakinahFaudzi ,1 SyarizaAbdul-Rahman ,2 andRosshairyAbdRahman 1 DecisionScienceDepartmen,SchoolofQuantitativeSciences,CollegeofArtsandSciences,UniversitiUtaraMalaysia,Malaysia
Step 1. Determine the cost table from the given problem. If the no. of sources is equal to no. of destinations, go to step 3. If the no. of sources is not equal to the no. of destination, go to step2. Step 2. Add a dummy source or dummy destination, so that the cost table becomes a square matrix.
Abstract. A novel way which uses the concept of modular arithmetic is proposed to solve Solid Assignment Problem. SAP is a special case of STP where the quantities corresponding to supply, demand ...